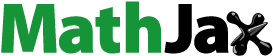
ABSTRACT
Urban Green Space (UGS) plays a crucial socio-cultural role in improving the quality of life. Assessment of quantity, quality, and accessibility determines whether the UGS are important social benefits. The travel preference of urban residents is a good indicator for the social relevance of UGS. This study integrates remote sensing and geographic big data to quantitatively analyze and evaluate the social benefits of urban green space. We propose a Green Social Benefit Index (GSBI) based on remote sensing retrieval and classification of urban green space. The GSBI is combined with the data on population mobility to investigate the influence of urban green space on residents’ behavior. The results show that urban residents prefer to move around in places with a higher quantity and quality of urban green space. The magnitude of the influence of urban green spatial features on population heat difference shows that accessibility > quantity > quality. The social benefits of urban green space are higher in middle centers compared to urban centers and city borders. The relationship between urban mobility and urban green space may suggest how to improve the distribution of green space in urban areas. This study could be a reference for the government planning department.
1. Introduction
Urban Green Space (UGS) is an integral part of urban space. It has environmental benefits such as cooling, humidifying, alleviating the urban heat island effect (Meng et al., Citation2018; Wang and Zacharias, Citation2015) and improving air quality (Derkzen, Van Teeffelen, and Verburg, Citation2015; Nowak, Crane, and Stevens, Citation2006). It can also provide a place for recreation and relaxation, reduce the mental stress of urban residents, and enhance urban livability (Deng, Citation2015; Mears et al., Citation2020). With the advancement of urbanization, urban green space is playing an increasingly important role in creating livable urban spaces and maintaining the well-being of urban residents.
The ability of urban green space to provide social benefits is influenced by its spatial pattern, accessibility, associated facilities, scale, species richness, and vegetation structure (Hwang et al. Citation2020; Kong, Yin, and Nakagoshi Citation2007; Lo and Jim Citation2010; Xu et al. Citation2016). Recent studies suggested that quantity, quality, and accessibility should be incorporated into a comprehensive assessment of urban green space distribution (De La Barrera, Reyes-Paecke, and Banzhaf Citation2016; Sathyakumar, Ramsankaran, and Bardhan Citation2020). Zhang, Xu, and Xiao (Citation2020) constructed a comprehensive and systematic method for assessing the social benefits of UGS. The results reflected the quantity of UGS by the vegetation cover within the buffer zone, the proximity of residents to UGS by the minimum path distance, and the effective and more active areas within public green spaces. You (Citation2016) conducted a study on green space supply in Shenzhen by considering quantitative indicators of green space area and green space area per capita, accessibility indicators, and the landscape pattern index. Williams et al. (Citation2020) conducted urban park metrics and equity studies for these three indicators. The park area metric is improved by using population density weighting. However, only a few studies have evaluated these three dimensions using an integrated method. Yao et al. (Citation2014) constructed an effective green equivalent to measure the real green spaces that are more enjoyable by residents. He, Wu, and Wang (Citation2020) constructed a park supply index by integrating the area, quality and spatial proximity of urban parks and evaluated the social benefits of urban parks and green spaces.
Location-based big data is a true reflection of urban residents’ activities, and studies have been conducted to apply big geographic data to urban green spatial studies. Xiao, Wang, and Fang (Citation2019) conducted a park accessibility study based on cellphone signaling data to analyze the spatiotemporal characteristics of green space accessibility and equity in Shanghai. Li et al. (Citation2017) investigated the effects of external factors such as accessibility, population density, and commercial facilities on park visitors in Beijing. It was the first study to apply APP check-in data to explore UGS utilization rates and their influencing factors. Similarly, Zhang and Zhou (Citation2018) investigated the effects of park attributes, park location, park context, and public transportation on the number of Weibo check-in visitors in parks. Fan et al. (Citation2021) proposed a park utilization index based on Baidu heat map data and studied the influence of internal factors such as park size, landscape shape index, water area, vegetation coverage, and external factors such as accessibility and surrounding facilities on park usage. From the spatial and temporal scales, mining travel big data will greatly expand the research content of urban green space and deepen the understanding of its social and humanistic benefits. Although location-based travel big data has received widespread attention, there are few studies that can quantitatively study the image of urban green space on travel traffic, and the data still faces difficulties in obtaining and processing.
The social service function of urban green space is an area of interest for scholars of urban planning and management. Many studies have used urban green space data from official databases, where the green space is only ‘public green space’ in the planning sense. It should be clarified that land partially or completely covered by grasses, trees, shrubs, and other vegetation can be classified as urban green space. They can make an important contribution to a city's function (Chen et al., Citation2017). At the same time, recent studies have shown that unmanaged ‘informal’ green spaces also have significant social functions and help to eliminate social inequities in the supply of urban green space (Hwang et al. Citation2020; Sikorska et al. Citation2020). Therefore, urban land covered by vegetation is considered a more general definition of urban green space in this study. In addition, data may be incomplete due to government management, and not refining enough geographic data on urban green space may lead to errors or even wrong results in quantitative studies (Xu et al. Citation2016). In contrast, remote sensing data have the characteristics of authenticity, providing large-scale full coverage of urban green space monitoring and mapping (Zhou, Liao, and Wang Citation2022). With the increasing spatial resolution of remote sensing data, highly heterogeneous spatial monitoring of green spaces in urban areas has been realized.
Therefore, based on the remote sensing extraction and classification of urban green space integrated with big data on population travel, this study aims to investigate the influence of urban green space on residents’ travel preferences. A scientific evaluation model of the social benefits of urban green space is constructed. The results can provide valuable references for urban green space management and further improve the social benefits of urban green space. Then we can provide a better service for urban residents.
2. Study area and data sources
2.1. Study area
Beijing is the capital of China and is located in the northern part of the North China Plain. The built-up area of Beijing has increased from 232 km2 in 1978 to 1485 km2 in 2018, and the typical concentric circle urban expansion pattern has led to a scarcity of green spaces within the main urban area. However, the population density in Xicheng District of Beijing is as high as 20,000 people per square kilometer (Beijing Municipal Bureau of Statistics Citation2021), and improving the urban habitat of Beijing has become an important issue. This study focuses on six central urban areas of Beijing (Dongcheng, Xicheng, Chaoyang, Haidian, Shijingshan, and Fengtai), which account for less than 10% of the total area of Beijing but host more than half of the city's resident population ().
2.2. Data sources and pre-processing
2.2.1. GF-6 PMS data
The GF-6 satellite is an optical remote sensing satellite in the National High Resolution Earth Observation System major project, carrying the Panchromatic/Multi-Spectral High-Resolution Camera (PMS) with an observation area of 90 km. PMS data has five spectral bands: panchromatic, blue, green, red, and near-infrared. Among them, the spatial resolution of panchromatic data is 2 m, and the spatial resolution of multispectral data is 8 m, which is an ideal data source for urban studies. In this study, 2 scenes of GF-6 data were downloaded on August 11, 2020 (scene sequence numbers 359572 and 359573) (http://www.cresda.com/CN/). Radiometric calibration, atmospheric correction, orthophoto correction, image fusion, image mosaic, and clipping are applied to the images.
Choosing the appropriate classification method for urban green space mapping is crucial, and the most suitable category may vary in different scenarios (Liao, Zhou, and Jing Citation2021). This study uses an object-oriented classification method to extract various features such as spectra, textures, and shapes of the ground objects. Based on these features, the urban vegetation can be divided into trees, shrubs, and grasses by a random forest algorithm. Based on the spectral and texture differences exhibited by different types of vegetation on images, training and testing samples for vegetation classification in the study area are prepared, with 500 training samples and 350 testing samples obtained. The spectral features selected for this study include the mean, brightness, contribution, standard deviation, standard false color HSI features, and vegetation index of the image object spectrum. The vegetation index includes the Normalized Difference Vegetation Index (NDVI), Ratio Vegetation Index (RVI), and Enhanced Vegetation Index (EVI). The shape features include boundary index, shape index, compactness, and aspect ratio. The texture features include eight Gray Level Co-occurrence Matrix (GLCM) statistics for panchromatic images. Overall, 31 features (19 spectral features, 4 shape features, and 8 texture features) are extracted from the training samples. Specifically, eCognition and ArcMap are used for image segmentation and sample collection production. The R language is used to construct a random forest model for vegetation classification.
Finally, the most important 20 features are selected for classification, with a classification accuracy of 91.7%. The spatial distribution of urban green spaces in the research area is shown in (a). The total area of the research area is 1,365.968 km2, with a vegetation area of 711.210 km2 and a vegetation coverage rate of 52.066%. Among them, grassland covers 44.513 km2, shrubs cover 429.685 km2, and trees cover 237.040 km2.
2.2.2. Population heat map
To understand the travel preferences of urban residents and thus verify the influence of the quantity, quality, and accessibility of urban green space on residents’ travel preferences, this study uses the Baidu population heat map. It collects real-time data based on geographic location information provided by smartphone users using Baidu products (e.g., Baidu Maps, Baidu Search, Baidu Weather), which reflects the population heat value at a certain spatial location. Although the Baidu population heat map does not directly show the actual population size, it has proven to be a reliable source of big data, and it can be used as a tool to dynamically depict the spatial distribution of the population in real time (Fang et al. Citation2020).
As a big data application with millions of users, the Baidu population heat map is of great value for studying the characteristics and mechanisms of urban population density spatial and temporal distribution (Li et al. Citation2019). Previous studies have shown that the urban population has similar evolutionary patterns on weekdays (from Monday to Friday), and it is also similar on rest days (from Saturday to Sunday) (Wu and Ye Citation2016). On rest days, people prefer to travel in the midday and afternoon, reaching the peak of the day at around 15:00, while on weekdays, people's activities are influenced by commuting, and there is a clear “morning peak” and “evening peak” (Shi, Xiao, and Zhan Citation2020). Recent studies have also demonstrated the validity of the Baidu population heat map on rest days and weekdays to reflect residents’ travel preferences (Fan et al., Citation2021). Therefore, this study applied the population heat data collected at 15:00 on October 16, 2020 (Friday) and October 17, 2020 (Saturday) in Beijing to reflect the free travel of residents on rest days and avoid the influence of “morning peak” and “evening peak” on population mobility.
According to the survey, the weather conditions were similar on both days, and there were no extreme weather, holidays, or special events. In terms of seasonal selection, autumn is considered the appropriate season for research. Due to the main vegetation type in Beijing being deciduous broad-leaved forests, leaves fall off in winter, and there is almost no greenery in the city. In summer, the temperature is high during the day, and people's travel preferences may be influenced by other human factors, such as going to air-conditioned shopping centers and cinemas. Spring in Beijing is relatively short, and urban vegetation and green leaves grow slowly. Compared to other seasons, autumn has a suitable climate, and people are more willing to interact with nature. In addition, the selected Friday and Saturday are two consecutive days, reducing the possibility of changes caused by other factors.
The raw data obtained are a series of points spaced about 100 m apart, containing latitude, longitude, and the heating value of the population shown in . By conducting kernel density analysis on Baidu's population heat data, a population heat map of the past two days can be obtained with a spatial resolution of 2 m. To obtain a more intuitive understanding of the population flow from Friday to Saturday, band operations were performed on the two graphs. The population heat value of October 17 is subtracted from the population heat value of October 16 to obtain a population difference heat map with a spatial resolution of 2 m.
2.2.3. Road network and POI data
The road network data used for accessibility metrics in this study is obtained from OpenStreetMap (OSM). OSM road network data in Shapefile vector format for China in 2020 is obtained through Geofabrik's free download server (https://download.geofabrik.de/). The data has been cropped and topologically corrected.
Point of Interest (POI) refers to all geographical entities that can be abstracted as points, containing names, categories, and precise spatial information. In this study, POIs are obtained through the Gaode Map open platform by defining the Uniform Resource Locator (URL) parameters sent by standard http requests. Finally, 312 subway stations and 3385 bus station POI data in the study area are obtained, which will be used as input for the accessibility metric in the subsequent study.
3. Research methodology
3.1. Technical route
The social benefits of urban green space depend on its quantity, quality, and accessibility. The evaluation indicators involved in this study are as follows: (1) Quantity of green space, characterized by the proportion of green space within a landscape unit (Percent of Landscape, PLAND). (2) Quality of green space, characterized by Shannon's Diversity Index (SHDI) and Aggregation Index (AI) of green space within a landscape unit. A rich landscape and a variety of green space types can provide a rich visual experience, i.e., the higher the diversity, the more likely it is to attract residents to stop and visit, and the greater its function is in beautifying the urban landscape and providing places for rest and recreation. Furthermore, a higher aggregation index indicates a more concentrated landscape structure of green space. Compared with fragmented green space patches, concentrated green spaces are more likely to be planned and managed by the government and provide residents with more prosperous social functions, such as physical exercise, sightseeing, resting, and socializing. (3) Accessibility of green space, characterized by road density (RD), number of subway stations (NSS), and number of bus stations (NBS) within the landscape unit. Based on these indices, the Green Social Benefit Index (GSBI) is proposed:
(1)
(1) The specific values of a, b, c, d, and e, i.e., the weights, will be obtained by further statistical analysis in combination with the travel examples of the residents in the study area, where a + b = 1, c + d + e = 1.
The technique and route of this study are shown in .
3.2. Window sampling
According to the distribution of urban vegetation and the needs of the study, 1 km × 1 km grid sampling is conducted in the study area, and 1149 samples are finally obtained. Each 1 km × 1 km grid serves as a landscape unit. The difference in population heat within each grid on October 17 and October 16 is extracted as the study's dependent variable. The road network density, the number of bus and subway stations, and the landscape indices PLAND, AI, and SHDI with the grid as the landscape unit () are extracted as the independent variables of the study for further statistical analysis.
3.3. Geographical detector
Quantitative analysis of the influence pattern of urban green space characteristics on residents’ travel preferences is the basis of the model construction evaluation. The traditional correlation and regression models can effectively reveal the correlation between the dependent and independent variables. However, the new methods that can detect the nonlinear relationship are recommended, considering the possible nonlinear relationship between independent and dependent variables in complex geographical phenomena.
The geographic detector is a new spatial statistical method for detecting spatially stratified heterogeneity and determining the relative importance of driving factors. Geographical detectors assume that if an environmental factor is spatially significantly consistent with the change of a geographical thing, it is decisive for the occurrence and development of the geographical thing (Zhao et al. Citation2020). This study applies the factor detection module of the geographical detector to measure the influence of the spatial characteristics of urban green space on residents’ travel preferences, which can be quantitatively described by the q-statistic:
(2)
(2) Where N and σ2 respectively represent the number of study units and the variance of Y across the study area, the overall Y has L strata (h = 1, 2,..., L), and stratum h consists of Nh units,
represents the variance of Y values within stratum h (Wang et al., Citation2016). q takes the values of [0,1], and q = 0 indicates that there is no coupling between Y and X; q = 1 indicates that Y is completely determined by the explanatory factor X; and the value of q = (0,1) indicates that X explains 100% of Y. In addition, interaction detection determines whether there is an interaction between the two factors and the strength, direction, linearity, or nonlinearity of the interaction by calculating the q value of the two factors after superposition and comparing it with the q value of the two single factors (Wang et al. Citation2010; Wang and Xu Citation2017).
According to the above principles, geographical detectors are good at analyzing type values; for continuous values, they can also be used for statistical analysis with appropriate discretization. Thus, geographical detectors can be used flexibly for qualitative and quantitative data. In addition, compared with other statistical methods, geographical detectors have the following advantages: (1) No linearity assumption, which can detect complex relationships of both linearity and nonlinearity between independent and dependent variables. (2) The results are not affected by multivariate covariance. (3) Interaction detection can measure the combined benefits of multiple influencing factors (Hu et al., Citation2020). Thus, the geographical detector method has been widely used in the fields of ecology and environment, public health, regional economy, etc. (Hu et al., Citation2022; Liu et al., Citation2021; Wan et al., Citation2019; Wu et al., Citation2020; Zhang, Xu, and Xiao, Citation2020; Zhu, Meng, and Zhu, Citation2020).
First, the study uses Pearson correlation analysis to initially detect the direction of the independent variable effect on the dependent variables, then it uses the geographical detector to determine the extent of the independent variable effect on the dependent variables and the strength of the interaction.
3.4. Moving window method
Moving window analysis is a common multi-scale analysis method. It can count the composition and pattern characteristics of the study object within a certain size sampling window and output the corresponding raster image. The spatial pattern of the study object is reflected quantitatively in the corresponding window size. To give full play to the advantages of high-resolution remote sensing images, this study calculated an index under the framework of the moving window method to obtain spatially continuous evaluation results of the spatial social benefits of urban green space. Then the spatial heterogeneity of green space within cities is analyzed, which is conducive to the accurate identification of areas with poor quality of green space. The scale, window shape, and size of the heterogeneity analysis need to be determined before the moving window analysis. According to the total area of the study area and the distribution of urban features, this study sets the window size to 1,000 m and the moving step size to 100 m to obtain a 100 m resolution thematic map of the evaluation index. Of course, when facing different cities, the choice of grid resolution and the step size of the moving window still need to be reconsidered. Choosing the appropriate resolution is crucial.
4. Results and analysis
4.1. Pearson correlation analysis
The correlation coefficients of the population heat difference with the quantity, quality, and accessibility of the green space are shown in . The results of the correlation analysis show that the population heat values on 16 and 17, 2020, are significantly negatively correlated with the quantity and quality of green space and significantly positively correlated with accessibility. This is consistent with people's daily activities, i.e. the distribution of activity intensity of urban residents is mainly attracted by facilities such as buildings, road networks, and public transportation. Although the absolute value of population heat value in each day is negatively correlated with the quantity and quality of urban green space, the difference in population heat value between weekends and weekdays is significantly and positively correlated with it. It indicates that residents are more attracted to urban green space on weekends compared to weekdays and are more likely to be active in places with a higher quantity and quality of urban green space. In addition, quantity has a more significant impact on residents’ travels, while among the quality characteristics, aggregation has a slightly greater impact on residents’ travels than vegetation diversity.
Table 1. Pearson correlation coefficient between population and UGS quantity, quality and accessibility.
Accessibility determines the traveling convenience for residents, but in the results of the correlation analysis, the road network density, the number of subway stations and the number of bus stations are negatively correlated with the population heat difference. Analysis of the real situation shows that the commercial areas with significantly lower population heat values have high levels of road network density and the number of public transportation stations.
To investigate the relationship between population heat difference and accessibility in depth, the sampling grids can be divided into two categories: the first category with increasing population heat values (where urban residents prefer to go on weekends), and the second one with decreasing population heat values (where people prefer to go on weekdays). The obtained correlation coefficients are shown in .
Table 2. Pearson correlation coefficient between population difference and UGS accessibility.
From , when the population heat difference is greater than 0, i.e. the residents’ travels increase, the population heat difference is positively correlated with accessibility. When the population heat difference is less than 0, i.e. when the residents’ travels decrease, the population heat difference is negatively correlated with accessibility. For example, road network density, as shown in . The figure shows that the higher the accessibility of the area, the more significant the difference between the population heat value on weekends and weekdays. Further, this study calculates the correlation between the absolute value of population heat difference (population heat value on October 17 and population heat value on October 16) and the three accessibility factors. The results show that the Pearson correlation coefficients for RD, NSS, and NBS are 0.445, 0.339, and 0.421, respectively. All results are significant at the 0.001 level. Combined with the population heat difference map, it can be concluded that office areas with higher accessibility levels experience a significant decrease in population heat values after the weekdays.
4.2. Geographical detector analysis
4.2.1. Factor detection
Considering the possible nonlinear relationship between PLAND, SHDI, AI, RD, NSS, and NBS and population heat differences, the driving effects of the above six factors on residential travel are further explored by geographical detectors (factor and interaction detections). In addition, it is already known that there may be different phenomena for areas with increasing and decreasing population heat values, so separate geographical detector analyses are conducted for three sampling schemes: (1) All samples are analyzed, with a total of 1149 sample areas; (2) The samples with increased population heat values (containing samples with constant heat values) are analyzed, with a total of 654 samples, i.e. places where people prefer to go on weekends; (3) The samples with decreased population heat values (containing samples with constant heat values) are analyzed, with a total of 534 sample areas, i.e. people prefer to go on weekdays.
The results of the factor detection of population heat difference concerning the quantity, quality, and accessibility of green space are shown in . When all sample areas are analyzed, it is found that although the q-values of all six factors pass the significance test. It indicates that all six factors have a significant driving effect on population travel; the q-values are less significant. However, after distinguishing the sample areas with increasing or decreasing population heat values, the q-values are significantly increased, indicating the validity and necessity of conducting separate analyses for areas with increasing or decreasing population heat values.
Table 3. The factor detector analysis of UGS quantity, quality, and accessibility affected the population heat difference.
Under all three schemes, the three factors with high significance are the proportion of green space, road network density, and the number of bus stations. Overall, the influence of urban green space characteristics on the population heat difference is shown as accessibility > quantity > quality. Among accessibility factors, the influence of the number of bus stations is comparable to that of road network density, while the influence of the number of subway stations is the least. Among quality factors, the influence of green space aggregation is slightly more significant than that of diversity.
4.2.2. Interaction detection
The results of the interaction detection between population heat difference and green space quantity, quality, and accessibility are shown in , demonstrating the strength of 15 sets of interactions for the six explanatory variables under the three sampling schemes. The q-values of the interactions are shown in a white and red gradient, with more red representing higher q-values. Those shown in bold are nonlinearly enhanced, and the rest are two-factor enhanced, indicating that the explanatory power of the two-factor superposition is stronger than that of the single factor. Similar to the factor detection results, under the three sampling scenarios, the interaction of the six explanatory variables on the change in population heat value is greater in the decreasing areas, followed by the increasing areas, while the interaction is the smallest when all samples are analyzed together. The number of bus stations has a higher q value in the factor detection, and the interaction with the other 5 factors also has a higher q value. The 3 sets of interactions with the highest q values are all found in the decreasing sample areas, and the highest is AI ∩ NBS with a q value of 0.534, i.e. the interaction between bus station density and green space aggregation explains 53.4% of the change in population heat value, followed by q(SHDI∩NBS) = 0.473 and q(PLAND∩NBS) = 0.446.
Table 4. The interaction detector analysis of UGS quantity, quality, and accessibility affected the population heat difference.
4.3. GSBI metrics and analysis
The above results provide a reference for determining the importance of urban space quality and accessibility in the GSBI index. The GSBI calculation formula can be determined as
(3)
(3) Before calculating GSBI, all indices are normalized. The calculation results of the constructed Green Social Benefit Index (GSBI) based on the moving window method are shown in (a). The GSBI shows a spatially aggregated distribution pattern, with lower GSBI values in the urban centers and edges and higher values in the middle circles. The histogram of GSBI frequency distribution for the whole study area is shown in (b). The minimum value of GSBI is 0, the maximum value is 141.425, the median is 24.682, the mean value of the whole study area is 28.073, and the standard deviation is 18.101. The histogram of GSBI frequency distribution is skewed to the left. Although the maximum GSBI value reaches 141.425, the occurrence frequency is higher for 10–30.
The statistical results of GSBI for each district are shown in . The mean values of GSBI in Dongcheng District, Xicheng District, Haidian District, Chaoyang District, Fengtai District, and Shijingshan District are 30.927, 28.027, 29.821, 26.720, 26.292, and 32.153, respectively. It is worth noting that although the maximum value of GSBI in Shijingshan District is only 99.737, the smallest among the six districts, the average value of GSBI in Shijingshan District is 32.153 at maximum. The maximum value of GSBI for the whole study area occurs in Dongcheng District, and the Moran index and standard deviation of Dongcheng District are also the highest among the six districts, indicating that a distinct hot spot may appear in Dongcheng District.
Table 5. Statistical results of GSBI in six districts of Beijing’s central urban area.
5. Discussion
To evaluate the performance of the GSBI model, seven representative 1 km × 1 km sample areas of the study area are selected as samples for the analysis. shows (1) the location of the sample areas, (2) the detailed landscape of the sample areas, and (3) the values of PLAND, SHDI, AI, RD, NSS, NBS, and GSBI that were calculated in each sample area, where PLAND, SHDI, AI, RD, NSS, and NBS are normalized values.
Sample (a) is in the northwestern part of the study area, where the proportion of green space landscape is 100% and the aggregation is also high; however, Shannon diversity is low, thus making the quality and quantity of UGS mismatch. More importantly, the density of the road network, the number of bus stations, and the number of subway stations in the area are all 0, resulting in an accessibility of 0. Therefore, as shown in Equation 2, GSBI is the product of the quantity, quality, and accessibility of UGS, and a completely inaccessible UGS cannot be effectively used by urban residents, making it difficult to bring the corresponding social benefits into play. Hence, the GSBI of the area is 0.
Samples (b and c) are respectively located in Beijing Botanical Garden and Chaoyang Park, the former in the northwestern part of the study area and the latter in the eastern part of the study area, closer to the urban center. The GSBI values of both regions are greater than 100, and the quantity and quality of UGS are comparable, but sample (c) has better accessibility. Thus, its GSBI is slightly greater than that of sample (b). Sample (d) is a commercial and office area located near China World Trade Center in Chaoyang District, and the quantity of UGS is much less than samples (a–c), but the area maintains a relatively high quality of UGS. In addition, accessibility in this area is also high, with the number of subway stations within 1 km2 reaching three and an NSS score of 0.75; thus, sample (d) still exceeds the average GSBI in the study area with only 20% PLAND.
Sample (e–g) are all residential areas but have different building distribution characteristics. From left to right, the building height increases, and the density decreases. Sample (e) is an alley area in the city's center with a UGS ratio of about 5%. Although the area has a more developed road network and a certain number of subway and bus stations, the quantity of UGS is still a prerequisite for UGS to be socially effective, and the low PLAND results in a GSBI of only 5.023. Samples in (f and g) are residential and school areas with a low quantity and quality of UGS. But sample (g) has a slightly higher proportion and aggregation of UGS than sample (f), with a low number of bus stations but two subway stations, so the overall GSBI is slightly higher.
Through the above analysis, the reason for the poor social benefits of green space in the urban periphery is that the accessibility level is too low to serve urban residents effectively. The reason for the poor social benefits of green space in the urban center is that the landscape ratio is too low to provide high-quality green space. In this regard, it is recommended to explore and develop green space in the city's suburbs to effectively provide green space for urban residents. In addition, for the relatively compact urban space in the center, the landscape ratio of green space should be maintained as much as possible. Its landscape pattern should be improved to provide green space with a more aggregated distribution and a more diverse vegetation structure.
It should be noted that owing to the different urban structures, levels, and structures of urban green in different cities, different conclusions may be drawn. The impact of accessibility on public travel varies in cities with an uneven distribution of greenery, such as circular, strip-shaped, star-shaped cities, etc. However, for cities with abundant and evenly distributed greenery, accessibility may be relatively less important. The study provides an effective research approach and method that can be applied in multiple cities if data allows. Different index distributions may be obtained in different urban patterns, which will be further implemented in future research.
The study also has certain limitations that need to be addressed in the future:
In this study, the quantitative analysis of UGS landscape pattern is to some extent influenced by classification accuracy. In addition, owing to the limitations of remote sensing automatic classification technology, it is difficult to identify the situation of mixed planting of trees, shrubs, and grasses and distinguish tree species. In future research, multiple sample areas can be selected and newer data, such as street scenes, can be considered for fine-grained studies supplemented by ground surveys.
Although this study provides a research method for the social benefits of urban green space, more time and regions are still needed to carry out adaptive research. Conducting research on different types of cities may lead to more interesting conclusions. In future research, it is important to further divide Chinese cities into multiple types and systematically study the impact of urban green space on public travel, as well as its differences in different types of cities.
Due to recent research emphasizing the importance of urban green space quality and accessibility relative to quantity, this study adopts the UGS social benefit evaluation framework based on quantity, quality, and accessibility. However, the quality of green space is a subjective concept. Due to data limitations, this study has tried to use landscape indices to indicate the diversity and maintenance level of green space but has not yet explored the richness and hierarchy of green configuration. In future research, the perspective of vertical observation can be increased through street view data.
6. Conclusion
Urban vegetation is an important carrier for enhancing the livability of cities. This study explores the influence of urban green space characteristics on residents’ travel preferences based on geographical detectors. A scientific and objective evaluation model of the social benefits of urban green space is established based on quantitative analysis results, which has practical significance for urban green space management and planning. The main results and conclusions of this study are as follows:
Correlation analysis shows that although the distribution of activity intensity among urban residents is mainly attracted by buildings, road networks, public transportation, and other facilities, urban residents are more attracted to urban green space on weekends than on weekdays and prefer to be active in places with a higher quantity and quality of urban green space.
The results of the geographical detector show that the degree of influence of urban green space characteristics on population heat difference is accessibility > quantity > quality. For accessibility, the influence of the number of bus stations is comparable to that of road network density, while the influence of the number of subway stations is the smallest. For quality, the influence of green space aggregation is slightly greater than that of diversity.
The results of the GSBI evaluation show that the social benefits of urban green space in urban centers and edges are poor, and the middle circle is high. Through example analysis, the social benefits of urban edge green space are poor, mainly because the accessibility level is too low and it is difficult to serve urban residents effectively. The social benefits of urban center green space are poor, mainly because the landscape proportion is too low and it is challenging to provide high-quality green space.
Reasonable green space construction contributes to better urban planning and brings benefits to people when traveling and resting. Especially in some old alleyway areas of the city center, more attention should be paid to green construction. This study can provide a reference for planners when renovating old areas and planning urban routes.
Acknowledgements
This work was financially supported by Hainan Provincial Natural Science Foundation of China(423CXTD390); National Natural Science Foundation of China (42171357); FY-3 Lot 03 Meteorological Satellite Engineering Ground Application System Ecological Monitoring and Assessment Application Project (Phase I) (ZQC-R22227); Youth Innovation Promotion Association CAS (2023139).
Disclosure statement
No potential conflict of interest was reported by the author(s).
Data availability statement
The data that support the findings of this study are available from the corresponding author upon reasonable request.
Additional information
Funding
References
- Beijing Statistics Bureau. 2021. Beijing Statistical Yearbook. Beijing: China Statistics Press (Chinese).
- Chen, Bin, Zhen Nie, Ziyue Chen, and Bing Xu. 2017. “Quantitative Estimation of 21st-century Urban Greenspace Changes in Chinese Populous Cities.” Science of The Total Environment 609:956–965. https://doi.org/10.1016/j.scitotenv.2017.07.238.
- De La Barrera, Francisco, Sonia Reyes-Paecke, and Ellen Banzhaf. 2016. “Indicators for Green Spaces in Contrasting Urban Settings.” Ecological Indicators 62:212–219. https://doi.org/10.1016/j.ecolind.2015.10.027.
- Deng, Chengbin. 2015. “Integrating Multi-source Remotely Sensed Datasets to Examine the Impact of Tree Height and Pattern Information on Crimes in Milwaukee, Wisconsin.” Applied Geography 65:38–48. https://doi.org/10.1016/j.apgeog.2015.10.005.
- Derkzen, Marthe L., Astrid J. A. Van Teeffelen, and Peter H. Verburg. 2015. “REVIEW: Quantifying Urban Ecosystem Services Based on High-resolution Data of Urban Green Space: An Assessment for Rotterdam, the Netherlands.” Edited by Sarah Diamond. Journal of Applied Ecology 52 (4): 1020–1032. https://doi.org/10.1111/1365-2664.12469.
- Fan, Zhengxi, Jin Duan, Yin Lu, Wenting Zou, and Wenlong Lan. 2021. “A Geographical Detector Study on Factors Influencing Urban Park Use in Nanjing, China.” Urban Forestry & Urban Greening 59:126996. https://doi.org/10.1016/j.ufug.2021.126996.
- Fang, Lei, Jinliang Huang, Zhenyu Zhang, and Vilas Nitivattananon. 2020. “Data-driven Framework for Delineating Urban Population Dynamic Patterns: Case Study on Xiamen Island, China.” Sustainable Cities and Society 62:102365. https://doi.org/10.1016/j.scs.2020.102365.
- He, Sanwei, Yilin Wu, and Lei Wang. 2020. “Characterizing Horizontal and Vertical Perspectives of Spatial Equity for Various Urban Green Spaces: A Case Study of Wuhan, China.” Frontiers in Public Health 8:10. https://doi.org/10.3389/fpubh.2020.00010.
- Hu, Die, Qingyan Meng, Uwe Schlink, Daniel Hertel, Wenxiu Liu, Maofan Zhao, and Fengxiang Guo. 2022. “How do Urban Morphological Blocks Shape Spatial Patterns of Land Surface Temperature over Different Seasons? A Multifactorial Driving Analysis of Beijing, China.” International Journal of Applied Earth Observation and Geoinformation 106:102648. https://doi.org/10.1016/j.jag.2021.102648.
- Hu, Die, Qingyan Meng, Linlin Zhang, and Ying Zhang. 2020. “Spatial quantitative analysis of the potential driving factors of land surface temperature in different “Centers” of polycentric cities: A case study in Tianjin, China.” Science of The Total Environment 706: 135244. https://doi.org/10.1016/j.scitotenv.2019.135244.
- Hwang, Yun Hye, Ivan Kurniawan Nasution, Deepika Amonkar, and Amy Hahs. 2020. “Urban Green Space Distribution Related to Land Values in Fast-growing Megacities, Mumbai and Jakarta–Unexploited Opportunities to Increase Access to Greenery for the Poor.” Sustainability 12 (12): 4982. https://doi.org/10.3390/su12124982.
- Kong, Fanhua, Haiwei Yin, and Nobukazu Nakagoshi. 2007. “Using GIS and Landscape Metrics in the Hedonic Price Modeling of the Amenity Value of Urban Green Space: A Case Study in Jinan City, China.” Landscape and Urban Planning 79 (3–4): 240–252. https://doi.org/10.1016/j.landurbplan.2006.02.013.
- Li, Jingang, Jianwei Li, Yangzi Yuan, and Guifang Li. 2019. “Spatiotemporal Distribution Characteristics and Mechanism Analysis of Urban Population Density: A Case of Xi’an, Shaanxi, China.” Cities 86:62–70. https://doi.org/10.1016/j.cities.2018.12.008.
- Li, Fangzheng, Fen Zhang, Xiong Li, Peng Wang, Junhui Liang, Yuting Mei, Wenwen Cheng, and Yun Qian. 2017. “Spatiotemporal Patterns of the Use of Urban Green Spaces and External Factors Contributing to their Use in Central Beijing.” International Journal of Environmental Research and Public Health 14 (3): 237. https://doi.org/10.3390/ijerph14030237.
- Liao, Yiming, Qi Zhou, and Xuanqiao Jing. 2021. “A comparison of Global and Regional Open Datasets for Urban Greenspace Mapping.” Urban Forestry & Urban Greening 62:127132. https://doi.org/10.1016/j.ufug.2021.127132.
- Liu, Wenxiu, Qingyan Meng, Mona Allam, Linlin Zhang, Die Hu, and Massimo Menenti. 2021. “Driving Factors of Land Surface Temperature in Urban Agglomerations: A Case Study in the Pearl River Delta, China.” Remote Sensing 13 (15): 2858. https://doi.org/10.3390/rs13152858.
- Lo, Alex Y.H., and C. Y. Jim. 2010. “Differential Community Effects on Perception and Use of Urban Greenspaces.” Cities 27 (6): 430–442. https://doi.org/10.1016/j.cities.2010.07.001.
- Mears, Meghann, Paul Brindley, Anna Jorgensen, and Ravi Maheswaran. 2020. “Population-level Linkages between Urban Greenspace and Health Inequality: The Case for Using Multiple Indicators of Neighbourhood Greenspace.” Health & Place 62:102284. https://doi.org/10.1016/j.healthplace.2020.102284.
- Meng, Qingyan, Linlin Zhang, Zhenhui Sun, Fei Meng, Liang Wang, and Yunxiao Sun. 2018. “Characterizing Spatial and Temporal Trends of Surface Urban Heat Island Effect in an Urban Main Built-up Area: A 12-year Case Study in Beijing, China.” Remote Sensing of Environment 204:826–837. https://doi.org/10.1016/j.rse.2017.09.019.
- Nowak, David J., Daniel E. Crane, and Jack C. Stevens. 2006. “Air Pollution Removal by Urban Trees and Shrubs in the United States.” Urban Forestry & Urban Greening 4 (3–4): 115–123. https://doi.org/10.1016/j.ufug.2006.01.007.
- Sathyakumar, Vasu, Raaj Ramsankaran, and Ronita Bardhan. 2020. “Geospatial Approach for Assessing Spatiotemporal Dynamics of Urban Green Space Distribution among Neighbourhoods: A Demonstration in Mumbai.” Urban Forestry & Urban Greening 48:126585. https://doi.org/10.1016/j.ufug.2020.126585.
- Shi, Peipei, Yinghui Xiao, and Qingming Zhan. 2020. “A Study on Spatial and Temporal Aggregation Patterns of Urban Population in Wuhan City Based on Baidu Heat Map and POI Data.” International Review for Spatial Planning and Sustainable Development 8 (3): 101–121. https://doi.org/10.14246/irspsda.8.3_101.
- Sikorska, Daria, Edyta Łaszkiewicz, Kinga Krauze, and Piotr Sikorski. 2020. “The Role of Informal Green Spaces in Reducing Inequalities in Urban Green Space Availability to Children and Seniors.” Environmental Science & Policy 108:144–154. https://doi.org/10.1016/j.envsci.2020.03.007.
- Wan, Jia, Junping Yan, Xiaomeng Wang, Ziqiang Liu, Hui Wang, and Ting Wang. 2019. “Spatial-Temporal Pattern and Its Influencing Factors on Urban Tourism Competitiveness in City Agglomerations Across the Guanzhong Plain.” Sustainability 11 (23): 6743. https://doi.org/10.3390/su11236743.
- Wang, Jinfeng, Xinhu Li, George Christakos, Yilan Liao, Tin Zhang, Xue Gu, and Xiaoying Zheng. 2010. “Geographical Detectors-based Health Risk Assessment and its Application in the Neural Tube Defects Study of the Heshun Region, China.” International Journal of Geographical Information Science 24 (1): 107–127. https://doi.org/10.1080/13658810802443457.
- Wang, Jingfeng, and Chendong Xu. 2017. “Geodetector: Principle and prospective.” Acta Geographica Sinica 72 (1): 116–134 (Chinese). https://doi.org/10.11821/dlxb201701010.
- Wang, Yupeng, and John Zacharias. 2015. “Landscape Modification for Ambient Environmental Improvement in Central Business Districts – A Case from Beijing.” Urban Forestry & Urban Greening 14 (1): 8–18. https://doi.org/10.1016/j.ufug.2014.11.005.
- Wang, Jinfeng, Tonglin Zhang, and Bojie Fu. 2016. “A measure of spatial stratified heterogeneity.” Ecological Indicators 67: 250–256. https://doi.org/10.1016/j.ecolind.2016.02.052.
- Williams, Tim G, Tom M. Logan, Connie T Zuo, Kevin D Liberman, and Seth D Guikema. 2020. “Parks and Safety: A Comparative Study of Green Space Access and Inequity in Five US Cities.” Landscape and Urban Planning 201:103841. https://doi.org/10.1016/j.landurbplan.2020.103841.
- Wu, Yafei, Ke Hu, Yaofeng Han, Qilin Sheng, and Ya Fang. 2020. “Spatial Characteristics of Life Expectancy and Geographical Detection of its Influencing Factors in China.” International Journal of Environmental Research and Public Health 17 (3): 906. https://doi.org/10.3390/ijerph17030906.
- Wu, Zhiqiang, and Zhongnan Ye. 2016. “Research on Urban Spatial Structure Based on Baidu Heat Map: A Case Study on the Central City of Shanghai.” City Planning Review 40 (4): 33–40 (Chinese). https://doi.org/10.11819/cpr20160407a.
- Xiao, Yang, De Wang, and Jia Fang. 2019. “Exploring the Disparities in Park Access through Mobile Phone Data: Evidence from Shanghai, China.” Landscape and Urban Planning 181:80–91. https://doi.org/10.1016/j.landurbplan.2018.09.013.
- Xu, Liyan, Hong You, Dihua Li, and Kongjian Yu. 2016. “Urban Green Spaces, Their Spatial Pattern, and Ecosystem Service Value: The Case of Beijing.” Habitat International 56:84–95. https://doi.org/10.1016/j.habitatint.2016.04.005.
- Yao, Liang, Jingru Liu, Rusong Wang, Ke Yin, and Baolong Han. 2014. “Effective Green Equivalent—A Measure of Public Green Spaces for Cities.” Ecological Indicators 47:123–127. https://doi.org/10.1016/j.ecolind.2014.07.009.
- You, Heyuan. 2016. “Characterizing the Inequalities in Urban Public Green Space Provision in Shenzhen, China.” Habitat International 56:176–180. https://doi.org/10.1016/j.habitatint.2016.05.006.
- Zhang, XiangXue, ChengDong Xu, and GeXin Xiao. 2020. “Spatial Heterogeneity of the Association between Temperature and Hand, Foot, and Mouth Disease Risk in Metropolitan and Other Areas.” Science of The Total Environment 713:136623. https://doi.org/10.1016/j.scitotenv.2020.136623.
- Zhang, Sai, and Weiqi Zhou. 2018. “Recreational Visits to Urban Parks and Factors Affecting Park Visits: Evidence from Geotagged Social Media Data.” Landscape and Urban Planning 180:27–35. https://doi.org/10.1016/j.landurbplan.2018.08.004.
- Zhao, Xuan, Jianjun Liu, Hongke Hao, and Yanzheng Yang. 2020. “Quantifying the Spatial Heterogeneity and Driving Factors of Aboveground Forest Biomass in the Urban Area of Xi’an, China.” ISPRS International Journal of Geo-Information 9 (12): 744. https://doi.org/10.3390/ijgi9120744.
- Zhou, Qi, Yiming Liao, and Jue Wang. 2022. “Mapping Global Urban Greenspace: An Analysis Based on Open Land-cover Data.” Urban Forestry & Urban Greening 74:127638. https://doi.org/10.1016/j.ufug.2022.127638.
- Zhu, Lijun, Jijun Meng, and Likai Zhu. 2020. “Applying Geodetector to Disentangle the Contributions of Natural and Anthropogenic Factors to NDVI Variations in the Middle Reaches of the Heihe River Basin.” Ecological Indicators 117:106545. https://doi.org/10.1016/j.ecolind.2020.106545.