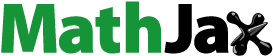
ABSTRACT
The Medium Resolution Spectral Image (MERSI) is a MODIS-like sensor aboard Fengyun-3 satellite. The first version of MERSI aerosol algorithm has been developed based on MODIS dark target (DT) algorithm, with modified models for estimating surface reflectance and an adjusted inland water masking method to release haze aerosols. This study applies MERSI DT algorithm to the global observations from the upgraded MERSI sensor (MERSI-II) on Fengyun-3D. And then, the Aerosol Optical Depth (AOD) results from the year of 2019–2020 are validated against the Aerosol Robotic Network (AERONET) data. In addition, analyses of the spatial distribution and error characteristics of MODIS and MERSI-II retrievals are presented. The overall validation demonstrates that MERSI-II retrievals perform well globally, with a correlation coefficient of 0.877 and 67.1% of matchups within the Expected Error envelope of ± (0.05 + 0.2τ), which are close to the statistic metrics of MODIS products. In addition, MERSI-II and MODIS AODs exhibit similar error trends and error dependence. Moreover, the similar global distribution characteristics of the two AODs are revealed in the retrieval performance at site and regional scales, as well as in the analysis of monthly averages. These findings indicate the success of the ported MERSI algorithm.
1. Introduction
Atmospheric aerosols play a significant role in the Earth's radiation budget and climate system by scattering and absorbing solar radiation, as well as interacting with clouds (Gautam et al. Citation2023; Ningombam et al. Citation2014; Verma et al. Citation2017). High aerosol loading can lead to air pollution, reduced visibility, and pose a threat to human health (Lelieveld et al. Citation2015; Li et al. Citation2017; Singh et al. Citation2022). Aerosol optical depth (AOD) is a critical parameter that characterizes aerosol optical properties. It is defined as the vertical integration of the aerosol extinction coefficient in the atmospheric column (Huang et al. Citation2020; Zhao et al. Citation2021). Since the highly varied distribution of aerosols, satellites are widely utilized to observe aerosols on regional to global scales, taking into account their advantages in terms of large spatial coverage (Garay et al. Citation2020; Su et al. Citation2023a).
Various types of satellite sensors are constantly emerging for observing and quantifying aerosol properties. The optical depth is traditionally retrieved from single-angle multispectral sensors such as Moderate Resolution Imaging Spectroradiometer (MODIS) and Visible Infrared Imaging Radiometer (VIIRS) (Remer, Levy, and Martins Citation2024; Su et al. Citation2022a). The multi-angle instruments (e.g. Multi-angle Imaging SpectroRadiometer (MISR) and Advanced Along-Track Scanning Radiometer (AATSR)) offer multiple angle views at the same scene, demonstrating potential in detecting aerosol types (Kuttippurath and Raj Citation2021; Limbacher, Kahn, and Lee Citation2022). Sensors with multi-angular and polarization observations (e.g. POLarizaiton and Directionality of the Earth’s Reflectance (POLDER) and GaoFen-5's Directional Polarimetric Camera (DPC)) enable the detection of detailed aerosol microphysical characteristics (Li et al. Citation2018; Pei et al. Citation2023). Geostationary sensors (e.g. Himawari-8/9's Advanced Himawari Imager (AHI), GOES-R's Advanced Baseline Imager (ABI), and Fengyun-4's Advanced Geosynchronous Radiation Imager (AGRI)) can provide aerosol information at high temporal resolution, allowing for the monitoring of diurnal cycle (Ge et al. Citation2019; Su et al. Citation2023b).
Currently, single-angle multispectral sensors, as one of the important techniques of aerosol detection, are still widely carried on various polar-orbiting and geostationary meteorological satellites. Among them, MODIS stands out as the most renowned, enabling comprehensive earth observation across various spectral bands from visible to thermal infrared. The AOD products generated by MODIS offer high precision, long-term data continuity, and global coverage, making them widely used in aerosol research (Choi et al. Citation2019; Levy et al. Citation2013; Su et al. Citation2021). However, the data record of a single sensor is too short to meet the time record length of the global Climate Data Record (CDR) (>30 years), and regional climate research requires multiple sources to derive consistent conclusions (Levy et al. Citation2015; Sogacheva et al. Citation2020). Consequently, the MODIS algorithm has been applied to different sensors, not only providing alternative aerosol products to the community but also offering the possibility of extending the aerosol record length. For instance, the Advanced Very High Resolution Radiometer (AVHRR) and Sea-viewing Wide Field-of-view Sensor (SeaWiFS) data have been reprocessed with Deep Blue (DB) and SeaWiFS Ocean Aerosol Retrieval (SOAR) algorithms, producing a long AOD time series over both land and ocean (Hsu et al. Citation2017; Sayer et al. Citation2017; Su et al. Citation2022b). VIIRS was designed to have similar capabilities as MODIS, with similar visible, near-infrared, and infrared wavelengths, similar spatial and temporal resolution, and similar physics behind the retrieval algorithms, potentially continuing the multi-decadal record of MODIS sensors (Hsu et al. Citation2019; Jackson et al. Citation2013; Levy et al. Citation2015). Additionally, geostationary meteorological satellites sensors such as AHI, ABI, and AGRI, have the spectral observation capabilities similar to MODIS, allowing the adjusted MODIS algorithm to derive high temporal resolution AOD (Gupta et al. Citation2019; Remer et al. Citation2020).
Three distinct algorithms have been developed based on MODIS, known as the Dark Target (DT) algorithm (Levy et al. Citation2007), the DB algorithm (Hsu et al. Citation2013), and the Multi-angle Implementation of Atmospheric Correction (MAIAC) algorithm (Lyapustin et al. Citation2018). The DT algorithm, as the earliest to emerge, is capable of retrieving AOD over vegetated and dark-soil land surfaces. Characterized by its ability to separate surface and atmospheric effects using empirical surface reflectance relationships, DT algorithm exhibits high portability (Gupta et al. Citation2016; Levy et al. Citation2007). Researchers have successfully applied the theoretical framework of the MODIS DT algorithm to different sensors. For instance, Levy et al. (Citation2015) applied a single MODIS-like (ML) DT algorithm on MODIS and VIIRS sensors and assessed the convergence of the derived products. The results revealed that the global offsets were nearly zero, while regional biases persisted over cloud areas and bright surfaces. Subsequently, the MODIS DT algorithm was ported to VIIRS and generated operational AOD products (Sawyer et al. Citation2020). The validation statistics of MODIS and VIIRS AOD products over land indicated that the percentages of points within the expected error envelope (within EE%) are similar, even though VIIRS exhibited a higher positive bias compared to MODIS. Gupta et al. (Citation2019) presented the outcomes of applying the MODIS DT algorithm to AHI sensor onboard geostationary satellite Himawari during May-June 2016. Their results showed a correlation coefficient (R) of 0.84 between AHI retrievals and Aerosol Robotic Network (AERONET) AOD, and 54.85% of the collocations falling within the EE envelope. The R and within EE% of MODIS products were 0.91 and 55.51% for the same stations and time period, respectively. Ge et al. (Citation2019) and Gao et al. (Citation2021) estimated AOD over China from AHI measurements using an adjusted DT algorithm. The former validated the retrievals from August 2015 to July 2016 using 12 AERONET and SONET sites, demonstrating a high correlation with an R2 of 0.81. The latter validated the AHI AOD in 2018 using 6 AERONET sites, revealing R greater than 0.75 and within EE% greater than 40% at each site.
The Fengyun-3 (FY-3) series of satellites represents the second generation of polar-orbit, sun-synchronous meteorological satellites in China (Wang et al. Citation2023). The Medium Resolution Spectral Imager (MERSI) series sensors onboard FY-3 satellite share many similarities in band design with MODIS, msking MERSI suitable for aerosol retrieval (Jin et al. Citation2021). Yang et al. (Citation2022) ported the MODIS DT algorithm to MERSI sensors and developed the first version of MERSI DT algorithm. This porting primarily involved modifications to the surface reflectance models and the inland water masking method, the latter of which enabled the retrievals of haze aerosols. The preliminary MERSI retrieval results were validated against AERONET observations over a three-month period, with results showing that 65.14% of the points fell within the EE envelope and a correlation coefficient R of 0.866. The corresponding statistical indicators for MODIS products were 72.71% and 0.925, respectively. Additionally, the correlation was 0.9 between the monthly average AOD of the two sensors. It can be seen that although the MERSI algorithm performs well globally, its retrieval accuracy still requires improvement compared to MODIS products. Accuracy evaluation of global long-term retrievals is a necessary step before algorithm optimization. Therefore, this study comprehensively validates and evaluates the retrieval results of MERSI algorithm using AERONET observations and MODIS products from 2019 to 2020. Through this work, we further identify the shortcomings of MERSI algorithm and prepare for the development of a more mature version. The paper is organized as follows: Section 2 provides a brief comparison of the MERSI and MODIS algorithms, highlighting their similarities and differences. Section 3 describes the dataset utilized, explains the matchup methodology and evaluation metrics. Section 4 presents the evaluation results of retrievals with reference to AERONET data. Further cross-comparisons are made using MODIS products from the same period. The discussion and conclusions are presented in section 5.
2. Sensor and retrieval algorithm
2.1. MERSI-II sensor characteristics
The FY-3 series satellites are the second generation of polar-orbiting satellites developed by China. Following the successful launches of the Fengyun-3A, B, and C satellites, the Fengyun-3D (FY-3D) satellite was launched on November 15, 2017. It has an orbital altitude of 836 km and crosses the equator in an ascending orbit at the nominal local time of 13:30. FY-3D is equipped with 10 sets of advanced remote sensing instruments, with MERSI-II sensor serving as a visible infrared scanning imager. Compared to its predecessor, MERSI, MERSI-II significantly enhances performance in terms of spectral response and imaging quality. MERSI-II has a wide swath of approximately 2900 km (cross-track) × 10 km (along-track, nadir), enabling it to achieve full global coverage once a day (Xu et al. Citation2018). The sensor provides 19 reflective solar channels (0.41–2.13 μm) and 6 emissive channels (3.8–12 μm), with the ground field of view at nadir of 250 m or 1000 m, providing important observation data for clouds, aerosols, water vapor, land surface properties, and ocean color (Yang et al. Citation2020). provides the comparison of MODIS and MERSI-II band wavelengths and spatial resolution, as well as a description of the use of the MERSI-II bands in MERSI aerosol algorithm.
Table 1. MERSI-II bands versus MODIS bands, and the description of the use of MERSI-II bands in MERSI algorithm.
depicts the spectral bands of MERSI-II sensor, including blue (0.471 µm) and red (0.645 µm) bands that are sensitive to aerosol, a 2.13 µm band that is similar to the MODIS 2.11 µm band, a 1.38 µm band for cirrus cloud masking, and near-infrared (0.87, 1.64 µm) and thermal infrared (11.0 µm) bands for ice/snow and inland water masking. The table also illustrates the differences in center wavelength and spatial resolution between MERSI-II and MODIS sensors. Specifically, the shortwave infrared (SWIR) wavelength of MERSI-II (1.03 µm) is different from that of MODIS (1.24 µm). Moreover, for the bands that overlap with MODIS, the spatial resolution of the MERSI-II sensor is 250 m in the blue (0.47 µm), green (0.55 µm), and red visible bands (0.65 µm), as well as in the near-infrared (0.87 µm) and thermal infrared (10.8 µm) bands, whereas MODIS has a spatial resolution of 250 m only in the red (0.65 µm) and near-infrared (0.86 µm) bands. Additionally, MODIS features a spatial resolution of 500 m in 2.1 µm shortwave near-infrared band, while MERSI-II has a spatial resolution of 1 km in this channel.
2.2. MERSI Dark Target algorithm
The first version of the MERSI DT algorithm (name it as version 1.0 MERSI DT algorithm) is designed to be as consistent as possible with the MODIS DT algorithm to obtain comparable retrievals. The main process of the algorithm includes the construction of Look-Up Tables (LUTs), pixel masking, pixel selection and aggregation, surface reflectance estimation and aerosol retrieval. MERSI algorithm employs the same aerosol types and methodology as MODIS algorithm to create LUTs for the four bands of MERSI-II sensor (0.47, 0.55, 0.65, and 2.13 µm). However, variations occur in pixel filtering, aggregation, and surface relationships establishment due to the differences in spectral response and spatial resolution between MERSI-II and MODIS sensors (). The following part will provide a brief overview of the similarities and differences between MODIS algorithm and MERSI algorithm.
Since the DT algorithm is capable of retrieving aerosol optical properties solely over land in clear-sky conditions, it is necessary to remove clouds, inland water, and ice/snow pixels that are not suitable for retrieval (Remer et al. Citation2005). describes the pixel masking methods employed by MERSI and MODIS algorithms. Here, represents the reflectance, and λ (µm) denotes the band wavelength. STD refers to the absolute standard deviation of the reflectance within a 3 × 3 box, and MSTD represents the mean weighted standard deviation. NDVI and NDSI are normalized difference vegetation index and normalized differential snow index, respectively. The brightness temperature calculated through the 10.8 µm band is denoted as BT11.
Table 2. Pixel screening methods in MODIS and MERSI algorithms.
MODIS DT algorithm applies reflectance and variability tests (STD and MSTD) at 0.47 and 1.38 µm to filter cloud pixels (Levy et al. Citation2013). As shown in , a pixel is identified as a cloud if any of the four conditions are met. The MERSI algorithm follows the same methodology and thresholds as the MODIS algorithm for cloud pixel identification.
In the MODIS algorithm, the NDVI index, calculated using the apparent reflectance of 0.65 and 0.87 µm, is used for inland water masking. Pixels with NDVI < 0.1 are categorized as inland water (Levy et al. Citation2013). However, Yang et al. (Citation2022) discovered that NDVI values fluctuate with atmospheric conditions, with higher aerosol loading resulting in lower NDVI. Severe haze conditions could cause NDVI values to fall below 0.1, leading to misclassification of haze aerosols as inland water. To address this issue, MERSI algorithm improves the inland water masking method by utilizing the characteristics of the 2.1 µm band, which is less affected by aerosols but is strongly absorbed by water. As shown in , an additional criterion of < 0.08 is integrated into the masking process to release haze aerosols retrievals (Yang et al. Citation2022). Furthermore, this approach also demonstrates efficacy when applied to MODIS sensors (Shi et al. Citation2021).
The MODIS DT algorithm utilizes the NDSI calculated based on 0.87 and 1.24 µm apparent reflectance, along with brightness temperature thresholds to mask ice/snow (Levy et al. Citation2013). Given the absence of the 1.24 µm band in MERSI-II, the 1.64 µm band serves as a substitute following extensive testing. Additionally, the NDSI thresholds differ between the MODIS and MERSI ice/snow masking algorithms, with values of 0.01 and 0.1, respectively.
The pixels are aggregated within the 10 × 10 km box after removing pixels that are not suitable for retrieval (e.g. clouds, snow, ice, water, or bright surfaces) (Levy et al. Citation2013). It is important to note that MODIS algorithm utilizes 500 m resolution data for aggregation with 20 × 20 pixels in the 10 × 10 km box, while MERSI algorithm uses upscaled 1 km resolution data for aggregation with 10 × 10 pixels in the 10 × 10 km box. As a result, MERSI algorithm exhibits a lower signal-to-noise ratio compared to MODIS algorithm, which may negatively impact the accuracy of retrievals.
The decoupling of surface and atmospheric contributions is one of the primary challenges in aerosol retrieval algorithms. The MODIS algorithm uses surface reflectance relationships in the visible and SWIR bands to determine surface reflectance (Equation (1)). Since these relationships are empirically derived, they need to be recalculated for MERSI algorithm. The comparison of surface reflectance relationships between the two algorithms is shown in . In Equation (1), ,
, and
are the surface reflectance at 0.47, 0.65, and 2.1 μm, respectively. The parameters
,
,
, and
are the slope and intercept of the models. In , θ, θs, and NDVISWIR represent the scattering angle, solar zenith angle, and the short-wave infrared normalized difference vegetation index, respectively. Notably, the calculation of MODIS NDVISWIR is based on the apparent reflectance of 1.24 and 2.1 µm bands. However, due to the unavailability of the 1.24 µm band in MERSI, the 1.03 µm band is used as a substitute. This may result in different surface reflectance parameterization results between the MERSI and MODIS algorithms.
(1)
(1)
Table 3. The comparison of reflectance relationship models for two algorithms.
reveals significant discrepancies between the two surface parameterization models. The surface reflectance relationship between 0.65 and 2.1 µm in MERSI algorithm differs from the pattern observed in MODIS algorithm, showing fixed values of 0.68 for slope and 0.1 for intercept. Furthermore, the slope and intercept of the reflectance relationship between the 0.47 and 0.65 µm bands of the MODIS algorithm remain constant at 0.49 and 0.005, respectively. In contrast, this relationship in MERSI algorithm varies with NDVISWIR and is further adjusted based on solar zenith angle. It is noteworthy that the MERSI algorithm in this version does not specifically parameterize urban surface reflectance, which is more similar to the MODIS C6 algorithm than to C6.1 algorithm.
3. Data and methods
3.1. Data sources
3.1.1. MERSI-II retrievals
The MERSI series sensors on FY-3 have aerosol-associated bands similar to MODIS, enabling the retrieval of AOD. In this study, the version 1.0 MERSI DT algorithm was used to retrieve global land AOD from 2019 to 2020 for the MERSI-II sensor aboard the FY-3D satellite. Similar to the MODIS products, MERSI-II retrievals are accompanied by quality assurance confidence (QAC) flag values ranging from 3 to 0, representing different levels of retrieval quality. Only high-quality MERSI-II retrievals (QAC = 3) are considered in the subsequent validation.
3.1.2. MODIS AOD products
NASA's MxD04_L2 products (x = Y for Aqua satellite observations and x = O for Terra satellite observations) provide long-term and global coverage of aerosol optical depth data with a spatial resolution of 10 km (Levy et al. Citation2013). The C6.1 MYD04_L2 ‘Optical_Depth_Land_And_Ocean’ dataset covering the year 2019–2020 was selected for comparison with MERSI-II retrievals, as both Aqua and FY-3D are afternoon satellites with relatively close observation times. This dataset was filtered using QAC = 3 over land.
3.1.3. AERONET data
AERONET is a global network of Sunphotometers used for ground-based aerosol remote sensing. It provides spectral AOD at 15-minute intervals at various wavelengths, including 340 nm, 380 nm, 440 nm, 500 nm, 675 nm, 870 nm, and 1020 nm (Holben et al. Citation2001; Sinyuk et al. Citation2020). The AOD data provided by AERONET has very low uncertainties of approximately 0.01–0.02, making it suitable as a reference for evaluating satellite remote sensing aerosol products (Giles et al. Citation2019). This paper selected AERONET Version 3 Level 2.0 (cloud-screened and quality-assured) data from 436 sites to validate the satellite-retrieved AOD. Referring to Wei et al. (Citation2019) and Sayer et al. (Citation2019), global land AERONET sites are divided into ten regions, with their spatial distribution shown in .
Figure 1. The spatial distribution of AERONET sites. The global land is divided into ten regions, and sites covered in different regions are indicated by different colors. Region names and associated abbreviations are Eastern North America (ENA), Western North America (WNA), South America (SAM), Eurasia (EUR), North Africa / Middle East, (NAME), South Africa (SAF), North-East Asia (NEA), Indian subcontinent (IND), South-East Asia, (SEA), and Oceania (OCE).

3.2. Matchup methodology and evaluation metrics
Satellite provides instantaneous observations of specific regions, while AERONET offers frequent point measurements over time. It is essential to match satellite retrievals with AERONET measurements in both time and space prior to validation. The retrievals (QAC = 3) within a 25 km radius of the ground stations are averaged for spatial matching, and the ground-based observations within ±30 minutes of the satellite overpass time are averaged for time matching (Levy et al. Citation2010; Petrenko and Ichoku Citation2013; Remer et al. Citation2013). A valid matchup is obtained when there are at least three high-quality satellite retrievals and two AERONET observations within the matching window. Generally, we choose the aerosol loading at 550 nm for validation. Since AERONET lacks 550 nm observation band, the quadratic fit method proposed by Eck et al. (Citation1999) is chosen to interpolate the AERONET AOD value at 550 nm (Equation (2)). As shown in the Equation (2), this method utilizes multi-band (at least three) AOD observations to calculate polynomial coefficients, and then substitutes the satellite sensor bands into the polynomial to derive the corresponding AOD results.
(2)
(2) where, λ represents the wavelength of AERONET observations, τλ is the AERONET AOD value,
,
,
are the coefficients of the quadratic polynomial.
The primary metrics utilized to evaluate the accuracy of retrievals are the Pearson correlation coefficient (R), root-mean-square error (RMSE), and mean bias (MB), as defined in Equations (3)–(5). Additionally, the percentage of matching points falling within (within EE%), above (above EE%), and below (below EE%) the Expected Error envelope (EE = ± (0.05 + 0.2τ)) is computed. It is generally considered that the EE requirement is satisfied when the within EE% is higher than 2/3. The Above EE% and Below EE% can be used to simply assess the overestimation and underestimation of retrievals, respectively (Levy et al. Citation2010; Remer et al. Citation2005).
(3)
(3)
(4)
(4)
(5)
(5)
4. Validation results
4.1. Validation against AERONET measurements
4.1.1. Overall validation scatterplots
shows the overall validation scatterplots of MODIS and MERSI-II AODs compared to AERONET observations. The examination of matchup numbers, N, in (a) and (b), illustrates that the number of MERSI-II/AERONET matchups (N = 38,863) surpasses that of MODIS/AERONET matchups (N = 32,293) by approximately 20%. This disparity is primarily attributed to MERSI-II's wider swath (3000 km) and the adjustment of inland water masking method in MERSI algorithm. The stricter quality control of the MODIS algorithm also contributes to this difference. The statistical metrics presented in reveal that the MODIS products and MERSI-II retrievals demonstrate similar performance. Specifically, AODs of MODIS and MERSI-II have a good correlation coefficient of 0.909 and 0.877 with AERONET observations, respectively. Moreover, both data exhibit a large percentage of matchups falling within the expected uncertainty, with within EE% of 74.5% for MODIS and 67.1% for MERSI-II. Additionally, positive MB values (0.014 for MODIS and 0.018 for MERSI-II) and large above EE% (16.8% for MODIS and 20.1% for MERSI-II) suggest that both MODIS and MERSI-II retrievals are slightly overestimated. Aside from this, MODIS products slightly outperform MERSI-II retrievals, exhibiting lower RMSE, lower MB, and higher Within EE%.
Figure 2. The scatterplots of satellite retrieved AOD at 550 nm. Panel (a) is for Aqua MODIS and panel (b) for FY-3D MERSI-II. The red solid line, black solid line, and black dashed lines represent the linear fitting line, 1:1 line, and EE envelopes, respectively.

A comparison of the scatter distributions in (a) and (b) reveals that the scatterplot of MERSI-II shows slightly greater dispersion than that of MODIS. The MODIS algorithm uses a retrieval box of 20 × 20 pixels at a 0.5 km resolution, while the MERSI algorithm uses a retrieval box of 10 × 10 pixels at a 1 km resolution, resulting in a lower signal-to-noise ratio for MERSI algorithm compared to MODIS algorithm. The difference in signal-to-noise ratio is the main reason for the higher dispersion of MERSI-II scatterplot. Furthermore, MERSI algorithm has improved the inland water masking method, allowing for the retrievals of haze aerosols. However, this improvement may lead to the inclusion of pixels with low NDVI, such as those in high latitude snowmelt areas in the northern hemisphere and desert regions near the equator (Yang et al. Citation2022). In these cases, the applicability of surface relationship models deteriorates, resulting in the appearance of high-value scatter points in low-AOD regimes.
4.1.2. Error trends
The purpose of this section is to assess and compare the consistency of error trends between MERSI-II and MODIS AODs. In , the monthly averages of AERONER AOD, satellite AOD, and statistical metrics (R, MB, RMSE, and Within EE%) are derived from the set of matchups. Two years (2019–2020) of data are employed to draw the trend of these values over 12 months, as shown in .
Figure 3. The comparison of MODIS AOD and MERSI-II AOD error trends. Panel (a) shows the averages of AERONET AOD, panel (b) shows the averages of satellite retrieved AOD and matchup numbers, panel (c) shows the correlation coefficients R between satellite retrieved AOD and AERONE observations. panel (d) shows the mean bias of satellite retrieved AOD, panel (d) shows the RMSE of satellite retrieved AOD, and panel (d) shows the within EE%, above EE% of satellite retrieved AOD. Blue and red represent the results of MODIS and MERSI-II retrievals, respectively.

(b)–(f) reveals similar trends in the monthly averages of AOD, R, MB, RMSE and Within EE% between MERSI-II and MODIS. Specifically, the trends of both average AODs are similar across all months, with minimal divergence observed from January to March. Due to MERSI-II's wider swath and the improvement of inland water masking, MERSI-II exhibits a higher number of matchups than MODIS ((b)). Besides, the R curves for both data display a fluctuating downward trend preceding August, followed by an upward trend thereafter ((c)). In addition, the mean biases for MODIS and MERSI-II AODs fluctuate within the range of −0.025 to 0.025, with bias curves exhibiting similar tendencies ((d)). Additionally, the RMSE for both MODIS and MERSI-II datasets is notably elevated in October, potentially attributed to the increase in AOD during that month ((a), (b)). Furthermore, the Within EE% for both AODs fluctuates moderately, maintaining levels between 65% and 80% ((f)). Overall, analogous trends are observed across these metrics in different AOD datasets. The design of the MERSI algorithm draws on the theoretical foundation of the MODIS algorithm, and the similar trends in the average values of AOD, R, MB, RMSE, and Within EE% reflect the internal consistency between the two algorithms.
further indicates a slightly lower accuracy of MERSI-II retrievals compared to MODIS products, which is consistent with the overall validation results in . The correlation coefficients R and Within EE% for MERSI-II AOD are generally lower than those for MODIS AOD ((c) and (f)), while the absolute mean bias and RMSE for MERSI-II AOD are generally higher than those for MODIS AOD ((d) and (e)).
4.2. Site-scale validation and comparison
When utilizing ground-based observations to evaluate the overall performance of aerosol retrievals, the inhomogeneity distribution of site locations and the differences in data volume among sites result in the unequal weighting of sites in combined metrics. Therefore, further validations are performed at the site scale. presents the validation results for MODIS and MERSI-II AODs for sites where at least 20 matchups are found.
Figure 4. The performance of MODIS/MERSI-II retrievals at the site scale. Four indicators are displayed, i.e. R (panel (a) for MODIS AOD, panel (b) for MERSI-II AOD), MB (panel (c) for MODIS AOD, panel (d) for MERSI-II AOD), RMSE (panel (e) for MODIS AOD, panel (f) for MERSI-II AOD), and within EE% (panel (g) for MODIS AOD, panel (h) for MERSI-II AOD).

The comparison presented in reveals almost identical color representations for R, MB, RMSE, and Within EE% between MERSI-II AOD and MODIS AOD across global sites. This similarity indicates that the spatial distribution and numerical characteristics of the four statistical indicators of the MERSI AOD are similar to those of the MODIS products. On the one hand, MERSI-II retrievals perform well at sites where MODIS products demonstrate high retrieval accuracy. For instance, both AODs have good performance at sites in Eastern North America and Europe, where vegetation cover is high. As shown in , sites in these two regions show higher correlation coefficients, closer to zero bias, lower RMSE, and higher within EE%. On the other hand, MERSI-II is difficult to achieve accurate retrievals at sites where MODIS products perform weakly. For example, both MODIS and MERSI-II AODs are overestimated at sites in Eastern North America and Asia, and underestimated at sites in Africa. Moreover, some sites in Africa and Asia have low numbers of points falling within the EE envelopes, which may be attributed to Asia's high aerosol concentration and complex aerosol sources, as well as Africa’s bright surface characteristics, providing challenges to the DT algorithm. In summary, MODIS AOD and MERSI-II AOD exhibit similar spatial patterns in aerosol retrieval performance across global sites, which is consistent with the expected results when applying the DT algorithm to both sensors.
4.3. Regional-scale validation and comparison
Variations in accuracy are noted across different sites in the site-scale validation analyses, which are related to the discrepancies in aerosols and surface properties in various regions. Thus, this section focuses on assessing the regional performance of MODIS AOD and MERSI-II AOD. The aim is to provide a more in-depth validation and evaluation of AODs to help users understand the characteristics of AOD datasets within their specific study regions. As shown in , global land data are divided into ten regions, further categorized into areas dominated by low AOD (Eastern and Western North America, Eurasia, and Oceania) and areas dominated by high AOD (South America, North Africa and the Middle East, South Africa, the Indian subcontinent, North-East Asia, and South-East Asia) for the purposes of analysis (Sayer et al. Citation2014; Wei et al. Citation2019). presents the statistics metrics of MODIS and MERSI-II over different regions.
Table 4. Regional statistics of satellite-retrieved AODs.
4.3.1. Dominantly low-AOD regions
The regions of Eastern North America, Western North America, Eurasia, and Oceania are categorized as dominantly low-AOD regions. The AOD performance over Oceania is not discussed here due to its limited valid collections. Most areas in Eastern North America are characterized by vegetation cover and low AOD values, creating favorable conditions for AOD retrieval. In this region, the AOD performance is higher than the global average results. The mean bias (0.010 for MODIS, and −0.001 for MERSI-II) and RMSE (0.065 for MODIS, and 0.013 for MERSI-II) are close to zero, and the fraction of matchups agreeing with AERONET within the expected uncertainty exceeds 2/3 (79.8% for MODIS, and 68.0% for MERSI-II).
In contrast to the densely vegetated surfaces in Eastern North America, parts of Western North America are covered by bare (arid or mountainous) terrain, making AOD retrievals more challenging. For the region as a whole, both AOD datasets are overestimated, as indicated by high mean biases (0.037 for MODIS and 0.056 for MERSI-II). Similar results, i.e. the overestimation of MODSI retrievals over Western North America, were reported by Jethva, Torres, and Yoshida (Citation2019). Additionally, high correlation coefficients (0.891 for MODIS, 0.880 for MERSI-II) and more than 60.0% of matchups within the expected error range are observed in this region (70.7% for MODIS, 62.4% for MERSI-II).
AERONET sites in Eurasia are characterized by low-AOD background and largely vegetated surfaces. Among the ten regions, this region exhibits the highest data volume (9748 matchups for MODIS, 11,427 matchups for MERSI-II), and the highest fraction of points within the expected uncertainty (82.0% for MODIS, 76.0% for MERSI-II).
4.3.2. Dominantly high-AOD regions
The regions of South America, North Africa and the Middle East, South Africa, Indian subcontinent, North-East Asia, and South-East Asia are classified as dominantly high-AOD regions. These regions exhibit more complex geographical and atmospheric characteristics compared to low AOD-dominated regions, bringing challenges for satellite aerosol retrievals. In South America, the validation results of MODIS and MERSI-II AOD exhibit correlation coefficients exceeding 0.85 and the proportion of points within the EE envelope exceeding 60%. In addition, MODIS retrievals indicate a slight underestimation with a negative bias of −0.015, while MERSI-II retrievals tend to be overestimated with a positive bias of 0.026. The possible reason for this discrepancy is that we do not optimize the urban surface reflectance relationship model as in MODSI C6.1. The overestimation over urban surfaces may be more pronounced in MERSI-II retrievals (Gupta et al. Citation2016). Additionally, the high AOD pixels released by MERSI-II could contribute to an increase in positive bias (Shi et al. Citation2021).
North Africa and the Middle East are mainly covered by bare and bright surfaces, which are not suitable for the application of DT algorithms. Additionally, the remoteness of locations means that many parts of the region are not well-covered by ground measurements. Consequently, the number of matchups here is relatively small (778 for MODIS, 1131 for MERSI-II). According to , although AODs exhibit a strong correlation with AERONET (R = 0.908 for MODIS, R = 0.867 for MERSI-II), the percentage of matchups falling within the expected error is less than 52% (51.0% for MODIS, 44.6% for MERSI-II). Furthermore, both AODs are significantly underestimated, with large negative mean biases (−0.087 for MODSI, −0.088 for MERSI-II).
In South Africa, MODIS and MERSI-II AODs exhibit similar validation metrics. Although the accuracy is slightly lower than the global average, high correlation coefficients (0.843 for MODSI, 0.801 for MERSI-II) and within EE% (69.4% for MODIS, 67.5% for MERSI-II) are observed in this region. In addition, both retrievals are underestimated compared to AERONET (MB = −0.031 for MODIS, and MB = −0.038 for MERSI-II).
The Indian subcontinent has been suffering the severe air pollution problems over the past two decades, characterized by a high AOD level and complex aerosol sources (Gautam et al. Citation2021). This requires aerosol retrieval algorithms to make accurate assumptions about aerosol modeling. Previous research indicated that the Indian region exhibited strong aerosol absorption in winter and strong aerosol scattering in summer, which may differ from the model parameters in algorithms (Gautam et al. Citation2022a; Jethva et al. Citation2010). Both MODIS and MERSI-II AODs are significantly overestimated over Indian subcontinent (MB = 0.054 for MODSI, MB = 0.080 for MERSI-II), with MERSI-II showing a more pronounced overestimation. In the case of MERSI-II retrievals, the release of haze pixels is another reason for its large bias, in addition to the uncertainties caused by inaccurate aerosol model assumptions (Gautam et al. Citation2022b; Shi et al. Citation2021). Moreover, a correlation coefficient above 0.85 and within EE% above 2/3 are observed in this region.
The majority of AERONET sites in the North-East Asia region are concentrated in China, North and South Korea, with much of Mongolia, Japan, and Russia having sparser coverage due to seasonal clouds, snow, mountainous terrain, and bright desert surfaces (Sayer et al. Citation2012). As shown in , the performance of MODIS and MERSI-II AODs is comparable in this region. Both retrievals exhibit good agreement with AERONET (R = 0.895 for MODIS, R = 0.877 for MERSI-II), and high proportion of matchups falling within the EE envelope (within EE% = 66.7% for MODIS, within EE% = 63.2% for MERSI-II). Additionally, similar overestimation issues are found in the two AOD datasets, with MB values of 0.056 for MODIS retrievals and 0.057 for MERSI-II retrievals. This overestimation is consistent with the findings of previous studies by Huang et al. (Citation2019), Wang et al. (Citation2019), Wei and Sun (Citation2017), and Wei et al. (Citation2019).
In South-East Asia, AERONET sites are mainly situated in densely populated urban areas. Due to the influence of frequent cloud cover, there are few matched points here. Notably, strongest correlation coefficients are found in this region, with an R of 0.933 for MODSI AOD and 0.910 for MERSI-II AOD. This indicates that both datasets can effectively track changes in aerosol loading. Additionally, overestimation is observed in MODIS and MERSI-II AODs over South-East Asia (MB = 0.040 for MODIS, MB = 0.047 for MERSI-II).
4.4. Monthly mean AOD comparison
For the global retrievals during 2019–2020, AOD of each granule (at a resolution of 10 × 10 km, approximately 0.1° × 0.1°) is processed through image mosaics. Daily mean AOD are calculated at a resolution of 1° × 1° when at least five pixels are within a 10 × 10 grid. Additionally, monthly mean AOD are computed when there are at least three available days in a month (Sawyer et al. Citation2020). illustrates the monthly AOD of MODIS and MERSI-II, as well as the AOD difference (MERSI-II AOD minus MODIS AOD). The months of January (figure 5a1-5c1), April (figure 5a2-5c2), July (figure 5a3-5c3), and October (figure 5a4-5c4) in 2019 are chosen for display. Since the MERSI DT land algorithm is unable to retrieve AOD over the ocean and bright surface, no retrieval data is available for these regions. Overall, the series of (a) and (b) indicate that the AOD range and spatial patterns of MODIS and MERSI-II are similar in most parts of the world. Higher AOD is predominantly observed in middle or low latitude areas, notably in Africa, Indian subcontinent and Central Asia. Conversely, the AOD in Eastern North America and Europe is lower. In addition, there is a significant increase in the number of retrievals over Russia in July, which may be related to the smoke from wildfires in northeastern Russia during June-July-August (JJA), as depicted by the high AOD concentration in (b3).
Figure 5. Monthly mean AOD for 2019-2020, at 1° × 1° resolution, for MODIS retrievals (panel left), MERSI-II retrievals (panel middle), the difference (panel right, MERSI-II AOD – MODIS AOD).

As shown in the series of (a) and (b), MERSI-II AOD is generally higher than MODIS AOD in high-AOD regions. The positive sign in high AOD conditions in (c) further illustrates this discrepancy. The improvement of the inland water masking method may account for this, as the improved method enables the retrieval of haze aerosols, resulting in higher AOD values for MERSI-II at high AOD loading. In addition, MERSI-II is capable of achieving more aerosol retrievals, particularly in eastern China and Central Asia, where frequent and intense natural aerosol pollution often occurs. The ability of the MERSI algorithm to retrieve haze aerosols contributes to a larger number of AOD in these areas. The wider swath of the MERSI-II sensor is also a contributing factor. However, discrete high-value points are observed in MERSI-II result in the desert region near the equator. This may be due to the poor applicability of the surface relationship model to low NDVI points, which are introduced by the adjusted inland water masking method. Furthermore, the pattern shown in (c) is uniform across the four months, with higher AOD observed in MERSI-II. This is consistent with the results obtained in , that is, MERSI-II retrievals has a larger positive bias compared to MODIS product.
4.5. Error dependence analysis
The validation and comparison conducted above have revealed similarities in the characteristics of MODIS and MERSI-II retrievals, including error trends, performance at site and regional scales, and monthly mean AOD values. However, the overestimation of MERSI-II AOD is more severe than that of MODIS AOD. This section analyzes the dependence of aerosol loading, view geometry, surface types, NDVISWIR, and surface reflectance on AOD retrieval errors. The purpose is to compare the effects of different factors on AOD uncertainty and explain the higher mean bias in MERSI-II retrievals.
4.5.1. Dependence on aerosol loading
As described by Remer et al. (Citation2005), and Levy et al. (Citation2010), the uncertainty of AOD retrievals at low aerosol concentration primarily comes from inaccuracies in surface characterization, while the selected aerosol model properties become dominant as the aerosol concentration increases. MODIS and MERSI-II AODs are categorized into 11 intervals with an interval of 0.02 according to AERONET observations to evaluate the retrieval performance under different aerosol loadings. The retrieval biases and the proportion of points in agreement within DT algorithm’s EE envelope are calculated across varying aerosol loading, as shown in .
Figure 6. Retrieval biases and within EE% of MODIS (panel a) and MERSI-II (panel b) AODs as a function of aerosol loading. Mean and 1-σ of AOD bias are represented by red points and blue vertical lines, respectively. The green bar represents within EE%, and the black dashed lines indicate the EE envelope of ± (0.05 + 0.2τ).

Overall, the performance of MODIS and MERSI-II AODs decreases as aerosol loading increases, exhibiting an upward trend in mean bias and a downward trend in Within EE%. Notably, both MODIS and MERSI-II retrievals are overestimated at high aerosol loading, with a larger positive bias in MERSI-II retrievals. Particularly, in bins where the ground-based AOD exceeds 0.8, the bias of MERSI-II AOD rises significantly, showing a pronounced overestimation. The overestimation at low AOD loading is possibly due to the underestimation of surface reflectance, while the overestimation at high AOD loading may be attributed to the inaccurate assumption of aerosol model. The MERSI algorithm provides more retrievals (e.g. haze) over high-AOD regions, where the assumed aerosol model may deviate from the actual situation. The increase of high-value AOD retrievals is accompanied by the introduction of high errors, contributing to the notable positive mean bias in MERSI-II retrievals under high AOD conditions.
4.5.2. Dependence on view geometry
Geometric parameters such as solar zenith angle (SZA), view zenith angle (VZA), and scattering angle (SCA) can affect the atmospheric transmission and scattering effects, consequently affecting the accuracy of AOD retrieval. Therefore, as shown in , SZA and VZA are divided into 12 bins at 5° intervals, and SCA is divided into 9 bins at 10° intervals to examine the dependence of AOD bias and Within EE% on view geometry.
Figure 7. As , except binned as a function of view geometry, panel (a, b) is for SZA, panel (c, d) is for VZA, panel (e, f) is for SCA.

As shown in , the variation patterns of the mean bias, 1-σ bias, and the percentage of points within the error envelope are nearly mirrored for the two AOD datasets across various angles. It can be seen that the effects of SZA, VZA, and SCA on the errors of MODIS and MERSI-II AODs are almost the same. Specifically, both MODIS and MERSI-II AOD are overestimated when SZA < 20°. The percentage of points within the error envelope gradually increases with higher SZA, indicating improved performance for both datasets ((a) and (b)). The retrieval errors of MODIS and MERSI-II AODs show minimal dependency on the VZA, as the mean bias, 1-σ, and within EE% remain relatively stable across view zenith angles ((c) and (d)). However, the retrieval accuracy gradually decreases with increasing scattering angle, as indicated by the decline in Within EE% and the rise in positive mean ((e) and (f)). Moreover, an obvious positive bias is observed in MERSI-II AOD when SCA > 150°, which needs to be addressed in future algorithm.
4.5.3. Dependence on surface types
The accuracy of AOD retrieval varies across different surface types (Sun et al. Citation2022; Wang et al. Citation2019). Consequently, this section undertakes further analysis to investigate the impact of different land cover types on retrieval errors. The classification of land cover types is accomplished using the MCD12Q1 product, which incorporates five global land cover classification systems. We focus on the 17 land cover categories defined by the International Geosphere-Biosphere Programme (IGBP) (Friedl et al. Citation2010). presents the percentage distribution of MODIS and MERSI-II matchups across the 17 categories. Subsequently, 17 categories were reclassified into broader types, namely ‘Forest,’ ‘Grassland,’ ‘Cropland,’ ‘Urban,’ and ‘Bare land’ to simplify the analysis (Wang et al. Citation2019). illustrates the dependency of aerosol retrieval errors on these five surface types.
Table 5. Percentage of MODIS and MERSI-II retrievals in 17 land cover types.
As can be seen in (a) and (b), both datasets perform well over ‘Forest’ and ‘Grassland’ types (mean bias < 0.02, Within EE% > 68%), while performing poorly over ‘Bare land’ type (mean bias > 0.05, Within EE% < 50%). The DT algorithm was originally designed for vegetated land surfaces, thus the ‘Forest’ and ‘Grassland’ surface types provide favorable conditions for retrieval, facilitating the generation of high-precision results. Conversely, the high reflectance of bare land presents numerous challenges to DT algorithms, resulting in great uncertainty in retrieved AOD (Levy et al. Citation2007). Notably, MERSI-II retrievals over urban surface type exhibit a significant positive bias (MBMERSI-II = 0.040). In MODIS C6.1, Gupta et al. (Citation2016) developed a new surface parameterization model that incorporated urban percentage along with NDVISWIR and scattering angle to mitigate inaccuracies in retrievals over urban regions. However, the version 1.0 MERSI-II algorithm has yet to address this issue due to limited data accumulation, resulting in the pronounced overestimation of MERSI-II AOD over urban surfaces.
4.5.4. Dependence on NDVISWIR
NDVISWIR is a crucial parameter utilized by the DT algorithm for estimating surface reflectance In aerosol free conditions, NDVISWIR is highly correlated with regular NDVI, with higher values indicating more active vegetation (Levy et al. Citation2007). It should be noted that due to the lack of a 1.24 µm band in MERSI-II, the bands used to calculate NDVISWIR are different for the two sensors. Specifically, MODIS defines NDVISWIR as a function of 1.24 and 2.1 µm, while MERSI-II defines it as a function of 1.03 and 2.1 µm. Therefore, it is necessary to analyze the impact of NDVISWIR on retrieval errors. We divide NDVISWIR into 12 bins with an interval of 0.05 and statistically analyze the AOD bias distribution in each bin to illustrate the impact of NDVISWIR on retrieval accuracy. The results are presented in .
Surprisingly, despite the differences in the definition of NDVISWIR, the biases and within EE% of MERSI-II and MODIS AODs exhibit extremely similar trends across NDVI bins, both showing a gradual improvement in retrieval performance with increasing NDVISWIR. For regions characterized by dormant or sparse vegetation (NDVISWIR ≤ 0.2), AODs exhibit a positive bias exceeding 0.25 and a Within EE% below 65%. In regions with moderate vegetation (0.2 < NDVISWIR ≤ 0.5), both AODs show improved performance, as demonstrated by higher within EE% values and reduced positive bias. Additionally, in densely vegetated areas (NDVISWIR > 0.5), MODIS and MERSI-II AODs achieve good retrieval accuracy, with Within EE% exceeding 80% and mean bias approaching zero.
4.5.5. Dependence on surface reflectance
The major challenge in satellite remote sensing aerosol retrieval is to separate the total observed reflectance into atmospheric and surface contributions. The scattering characteristics of aerosol particles brighten dark surfaces, making it easier for the DT algorithm to acquire aerosol signals (Levy et al. Citation2013). However, aerosol information is relatively weak over bright surfaces, and estimation errors in reflectance can significantly impact retrieval accuracy (Hsu et al. Citation2013). Given the different surface relationship models employed by MODIS and MERSI algorithms, it is crucial to examine the effect of different surface reflectance on AOD retrieval errors, as illustrated in .
Generally, the regularities of retrieval errors with various surface reflectance are similar for the two datasets. Algorithms tend to produce accurate aerosol retrievals at low surface reflectance (surface reflectance ≤ 0.10), with MODIS and MERSI-II AODs showing a bias close to 0 and a Within EE% exceeding 2/3. However, the aerosol signal may be submerged at higher surface reflectance (surface reflectance > 0.10), making AOD retrieval more challenging. In such cases, the Within EE% displays a gradually decreasing trend as surface reflectance increases for both retrievals, indicating a decline in the quality of AOD datasets. Notably, an obvious positive mean bias was observed in MERSI-II AOD when the surface reflectance exceeds 0.16, suggesting that the retrieval accuracy of MERSI algorithm needs improvement under high reflectance conditions.
5. Conclusions
The MERSI series sensors aboard the FY-3 satellite include aerosol bands similar to those used in MODIS DT algorithm. Yang et al. (Citation2022) developed the version 1.0 MERSI DT algorithm on the theoretical basis of MODIS DT algorithm. This study presents a detailed evaluation of MERSI-II retrievals from 2019 to 2020 using AERONET observations and MODIS products. The main conclusions are as follows.
Overall, the validation statistics for the MERSI-II AOD are similar to those of the MODIS products when compared to the AERONET data, with correlation coefficients above 0.85 and the proportion of points falling within the expected error envelope exceeding 2/3. Additionally, the error characteristics of MODIS and MERSI-II AOD are generally consistent, with similar trends in R, mean bias, RMSE, and within EE%. The dependence of both errors on multiple factors, including aerosol loading, view geometry, surface types, NDVISWIR, and surface reflectance, is also comparable. Particularly, the error dependence on view geometry and NDVISWIR is nearly mirrored.
The validation and comparison of MODIS and MERSI-II AODs are conducted at site and regional scales. For global sites, MODIS and MERSI-II retrievals exhibit similar spatial patterns in terms of consistency with AERONET. MERSI-II retrievals are more effective at sites where MODIS products perform well, and less effective at sites where MODIS product perform weakly. Due to the complexity of global surface and atmospheric systems, satellite aerosol retrieval accuracy varies significantly across different regions. Both data perform well over densely vegetated Eastern North America and Eurasia, but poorly over sparsely vegetated North Africa and the Middle East. Moreover, significant overestimation is observed over the Indian subcontinent, North-East Asia, and South-East Asia. In addition to the similar performance at the site and regional scales, MERSI-II AOD and MODIS AOD also demonstrate good consistency in the range and spatial distribution of monthly mean AOD.
In summary, MERSI-II retrievals demonstrate good accuracy compared to AERONET and exhibit excellent similarity with MODIS products, indicating that the ported MERSI algorithm is successful. However, we observe that the dispersion of MERSI-II validation scatterplot is larger than that of the MODIS validation scatterplot. This difference may be attributable to the lower signal-to-noise ratio caused by using 1 km resolution data for aggregation in MERSI algorithm. Furthermore, there are some high-value scatter points in monthly averages of MERSI-II. This is due to the introduction of low NDVI points by the adjusted inland water masking method, resulting in poor applicability of the surface relationship model. These aspects require further optimization in future work.
Another significant finding is the more pronounced overestimation in MERSI-II retrievals compared to MODIS products. Further analysis revealed that there are two main reasons for this phenomenon. Firstly, the version 1.0 MERSI-II algorithm does not modify the surface parameterization scheme for urban areas, and thus the bias in MERSI-II retrievals includes the bias caused by inaccurate urban surface reflectance. Therefore, it is necessary to improve the accuracy of urban retrievals by updating the urban reflectance models in subsequent versions of algorithm. Secondly, MERSI algorithm changes the inland water masking method for the retrievals of haze aerosols. However, this change also brings new biases, which arise mainly from inaccurate aerosol models, resulting in significant overestimation at high aerosol loading. Enhancing the accuracy of haze retrievals in the future can be achieved by updating aerosol models and refining the assumption about aerosol layer height.
The evaluation presented in this paper serves not only as a reference for refining MERSI algorithm, but also as a guide for algorithm design for sensors that replicate the crucial spectral and spatial capabilities of MODIS. It is expected that the next version of the MERSI algorithm will optimize the surface relationship model and aerosol characteristic assumptions to further improve retrieval accuracy.
Acknowledgements
We would like to express our deepest gratitude to National Satellite Meteorological Centre (NSMC) for providing MERSI-II data, to AERONET for providing ground-based observations, and to Level-1 and Atmosphere Archive & Distribution System Distributed Active Archive Center (LAADS DAAC) for distributing MODIS aerosol products.
Disclosure statement
No potential conflict of interest was reported by the author(s).
Additional information
Funding
References
- Choi, M., H. Lim, J. Kim, S. Lee, T. F. Eck, B. N. Holben, M. J. Garay, et al. 2019. “Validation, Comparison, and Integration of GOCI, AHI, MODIS, MISR, and VIIRS Aerosol Optical Depth Over East Asia During the 2016 KORUS-AQ Campaign.” Atmospheric Measurement Techniques 12 (8): 4619–4641. https://doi.org/10.5194/amt-12-4619-2019.
- Eck, T. F., B. N. Holben, J. S. Reid, O. Dubovik, A. Smirnov, N. T. O'Neill, I. Slutsker, and S. Kinne. 1999. “Wavelength Dependence of the Optical Depth of Biomass Burning, Urban, and Desert Dust Aerosols.” Journal of Geophysical Research: Atmospheres 104 (D24): 31333–31349. https://doi.org/10.1029/1999JD900923.
- Friedl, M. A., D. Sulla-Menashe, B. Tan, A. Schneider, N. Ramankutty, A. Sibley, and X. Huang. 2010. “MODIS Collection 5 Global Land Cover: Algorithm Refinements and Characterization of New Datasets.” Remote Sensing of Environment 114 (1): 168–182. https://doi.org/10.1016/j.rse.2009.08.016.
- Gao, L., L. Chen, J. Li, C. Li, and L. Zhu. 2021. “An Improved Dark Target Method for Aerosol Optical Depth Retrieval Over China from Himawari-8.” Atmospheric Research 250: 105399. https://doi.org/10.1016/j.atmosres.2020.105399.
- Garay, M. J., M. L. Witek, R. A. Kahn, F. C. Seidel, J. A. Limbacher, M. A. Bull, D. J. Diner, et al. 2020. “Introducing the 4.4 km Spatial Resolution Multi-Angle Imaging SpectroRadiometer (MISR) Aerosol Product.” Atmospheric Measurement Techniques 13 (2): 593–628. https://doi.org/10.5194/amt-13-593-2020.
- Gautam, S., J. Elizabeth, A. S. Gautam, K. Singh, and P. Abhilash. 2022b. “Impact Assessment of Aerosol Optical Depth on Rainfall in Indian Rural Areas.” Aerosol Science and Engineering 6 (2): 186–196. https://doi.org/10.1007/s41810-022-00134-9.
- Gautam, S., A. S. Gautam, K. Singh, and E. J. James. 2021. “Investigations on the Relationship Among Lightning, Aerosol Concentration, and Meteorological Parameters with Specific Reference to the Wet and Hot Humid Tropical Zone of the Southern Parts of India.” Environmental Technology & Innovation 22: 101414. https://doi.org/10.1016/j.eti.2021.101414.
- Gautam, S., L. Hens, A. S. Gautam, and L. Shao. 2023. “Introduction to the Special Issue ‘Aerosol Optical Depth, Aerosols, and Precipitation’.” Geological Journal 58 (12): 4299–4301. https://doi.org/10.1002/gj.4874.
- Gautam, A. S., K. Singh, M. Sharma, S. Gautam, A. Joshi, and S. Kumar. 2022a. “Classification of Different Sky Conditions Based on Solar Radiation Extinction and the Variability of Aerosol Optical Depth, Angstrom Exponent, Fine Particles Over Tehri Garhwal, Uttarakhand, India.” Mapan 38 (1): 21–36. https://doi.org/10.1007/s12647-022-00533-w.
- Ge, B., Z. Li, L. Liu, L. Yang, X. Chen, W. Hou, Y. Zhang, D. Li, and L. Li. 2019. “A Dark Target Method for Himawari-8/AHI Aerosol Retrieval: Application and Validation.” IEEE Transactions on Geoscience and Remote Sensing 57 (1): 381–394. https://doi.org/10.1109/TGRS.2018.2854743.
- Giles, D. M., A. Sinyuk, M. G. Sorokin, J. S. Schafer, A. Smirnov, I. Slutsker, T. F. Eck, et al. 2019. “Advancements in the Aerosol Robotic Network (AERONET) Version 3 Database – Automated Near-Real-Time Quality Control Algorithm with Improved Cloud Screening for Sun Photometer Aerosol Optical Depth (AOD) Measurements.” Atmospheric Measurement Techniques 12 (1): 169–209. https://doi.org/10.5194/amt-12-169-2019.
- Gupta, P., R. C. Levy, S. Mattoo, L. A. Remer, R. E. Holz, and A. K. Heidinger. 2019. “Applying the Dark Target Aerosol Algorithm with Advanced Himawari Imager Observations During the KORUS-AQ Field Campaign.” Atmospheric Measurement Techniques 12 (12): 6557–6577. https://doi.org/10.5194/amt-12-6557-2019.
- Gupta, P., R. C. Levy, S. Mattoo, L. A. Remer, and L. A. Munchak. 2016. “A Surface Reflectance Scheme for Retrieving Aerosol Optical Depth Over Urban Surfaces in MODIS Dark Target Retrieval Algorithm.” Atmospheric Measurement Techniques 9 (7): 3293–3308. https://doi.org/10.5194/amt-9-3293-2016.
- Holben, B. N., D. Tanré, A. Smirnov, T. F. Eck, I. Slutsker, N. Abuhassan, W. W. Newcomb, et al. 2001. “An Emerging Ground-Based Aerosol Climatology: Aerosol Optical Depth from AERONET.” Journal of Geophysical Research: Atmospheres 106 (D11): 12067–12097. https://doi.org/10.1029/2001JD900014.
- Hsu, N. C., M. J. Jeong, C. Bettenhausen, A. M. Sayer, R. Hansell, C. S. Seftor, J. Huang, and S. C. Tsay. 2013. “Enhanced Deep Blue Aerosol Retrieval Algorithm: The Second Generation.” Journal of Geophysical Research: Atmospheres 118 (16): 9296–9315. https://doi.org/10.1002/jgrd.50712.
- Hsu, N. C., J. Lee, A. M. Sayer, N. Carletta, S.-H. Chen, C. J. Tucker, B. N. Holben, and S.-C. Tsay. 2017. “Retrieving Near-Global Aerosol Loading Over Land and Ocean from AVHRR.” Journal of Geophysical Research: Atmospheres 122 (18): 9968–9989. https://doi.org/10.1002/2017JD026932.
- Hsu, N. C., J. Lee, A. M. Sayer, W. Kim, C. Bettenhausen, and S. C. Tsay. 2019. “VIIRS Deep Blue Aerosol Products Over Land: Extending the EOS Long-Term Aerosol Data Records.” Journal of Geophysical Research: Atmospheres 124 (7): 4026–4053. https://doi.org/10.1029/2018JD029688.
- Huang, G., Y. Chen, Z. Li, Q. Liu, Y. Wang, Q. He, T. Liu, et al. 2020. “Validation and Accuracy Analysis of the Collection 6.1 MODIS Aerosol Optical Depth Over the Westernmost City in China Based on the Sun-Sky Radiometer Observations From SONET.” Earth and Space Science 7 (3). ;https://doi.org/10.1029/2019EA001041.
- Huang, Y., B. Zhu, Z. Zhu, T. Zhang, W. Gong, Y. Ji, X. Xia, et al. 2019. “Evaluation and Comparison of MODIS Collection 6.1 and Collection 6 Dark Target Aerosol Optical Depth Over Mainland China Under Various Conditions Including Spatiotemporal Distribution, Haze Effects, and Underlying Surface.” Earth and Space Science 6 (12): 2575–2592. https://doi.org/10.1029/2019EA000809.
- Jackson, J. M., H. Liu, I. Laszlo, S. Kondragunta, L. A. Remer, J. Huang, and H. Huang. 2013. “Suomi-NPP VIIRS Aerosol Algorithms and Data Products.” Journal of Geophysical Research: Atmospheres 118 (22): 12673–12689. https://doi.org/10.1002/2013JD020449.
- Jethva, H., S. K. Satheesh, J. Srinivasan, and R. C. Levy. 2010. “Improved Retrieval of Aerosol Size-Resolved Properties from Moderate Resolution Imaging Spectroradiometer Over India: Role of Aerosol Model and Surface Reflectance.” Journal of Geophysical Research Atmospheres 115 (D18): D18213. https://doi.org/10.1029/2009JD013218.
- Jethva, H., O. Torres, and Y. Yoshida. 2019. “Accuracy Assessment of MODIS Land Aerosol Optical Thickness Algorithms Using AERONET Measurements Over North America.” Atmospheric Measurement Techniques 12 (8): 4291–4307. https://doi.org/10.5194/amt-12-4291-2019.
- Jin, S., M. Zhang, Y. Ma, W. Gong, C. Chen, L. Yang, X. Hu, et al. 2021. “Adapting the Dark Target Algorithm to Advanced MERSI Sensor on the FengYun-3-D Satellite: Retrieval and Validation of Aerosol Optical Depth Over Land.” IEEE Transactions on Geoscience and Remote Sensing 59 (10): 8781–8797. https://doi.org/10.1109/TGRS.2020.3021021.
- Kuttippurath, J., and S. Raj. 2021. “Two Decades of Aerosol Observations by AATSR, MISR, MODIS and MERRA-2 Over India and Indian Ocean.” Remote Sensing of Environment 257: 112363. https://doi.org/10.1016/j.rse.2021.112363.
- Lelieveld, J., J. S. Evans, M. Fnais, D. Giannadaki, and A. Pozzer. 2015. “The Contribution of Outdoor air Pollution Sources to Premature Mortality on a Global Scale.” Nature 525: 367–371. https://doi.org/10.1038/nature15371.
- Levy, R. C., S. Mattoo, L. A. Munchak, L. A. Remer, A. M. Sayer, F. Patadia, and N. C. Hsu. 2013. “The Collection 6 MODIS Aerosol Products Over Land and Ocean.” Atmospheric Measurement Techniques 6 (11): 2989–3034. https://doi.org/10.5194/amt-6-2989-2013.
- Levy, R. C., L. A. Munchak, S. Mattoo, F. Patadia, L. A. Remer, and R. E. Holz. 2015. “Towards a Long-Term Global Aerosol Optical Depth Record: Applying a Consistent Aerosol Retrieval Algorithm to MODIS and VIIRS-Observed Reflectance.” Atmospheric Measurement Techniques 8 (10): 4083–4110. https://doi.org/10.5194/amt-8-4083-2015.
- Levy, R. C., L. A. Remer, R. G. Kleidman, S. Mattoo, C. Ichoku, R. Kahn, and T. F. Eck. 2010. “Global Evaluation of the Collection 5 MODIS Dark-Target Aerosol Products Over Land.” Atmospheric Chemistry and Physics 10 (21): 10399–10420. https://doi.org/10.5194/acp-10-10399-2010.
- Levy, R. C., L. A. Remer, S. Mattoo, E. F. Vermote, and Y. J. Kaufman. 2007. “Second-Generation Operational Algorithm: Retrieval of Aerosol Properties Over Land From Inversion of Moderate Resolution Imaging Spectroradiometer Spectral Reflectance.” Journal of Geophysical Research: Atmospheres 112 (D13): D13211. https://doi.org/10.1029/2006jd007811.
- Li, Z., J. Guo, A. Ding, H. Liao, J. Liu, Y. Sun, T. Wang, et al. 2017. “Aerosol and Boundary-Layer Interactions and Impact on Air Quality.” National Science Review 4 (6): 810–833. https://doi.org/10.1093/nsr/nwx117.
- Li, Z., W. Hou, J. Hong, F. Zheng, D. Luo, J. Wang, X. Gu, and Y. Qiao. 2018. “Directional Polarimetric Camera (DPC): Monitoring Aerosol Spectral Optical Properties Over Land from Satellite Observation.” Journal of Quantitative Spectroscopy & Radiative Transfer 218: 21–37. https://doi.org/10.1016/j.jqsrt.2018.07.003.
- Limbacher, J. A., R. A. Kahn, and J. Lee. 2022. “The New MISR Research Aerosol Retrieval Algorithm: A Multi-Angle, Multi-Spectral, Bounded-Variable Least Squares Retrieval of Aerosol Particle Properties Over Both Land and Water.” Atmospheric Measurement Techniques 15 (22): 6865–6887. https://doi.org/10.5194/amt-15-6865-2022.
- Lyapustin, A., Y. Wang, S. Korkin, and D. Huang. 2018. “MODIS Collection 6 MAIAC Algorithm.” Atmospheric Measurement Techniques 11 (10): 5741–5765. https://doi.org/10.5194/amt-11-5741-2018.
- Ningombam, S. S., S. P. Bagare, A. K. Srivastava, B. J. Sohn, H.-J. Song, and E. Larson. 2014. “Aerosol Radiative Forcing Over a High-Altitude Station Merak, in the Trans-Himalayan Region During Advection of Anthropogenic Events from the Indo-Gangetic Plain.” Atmospheric Environment 98: 253–259. https://doi.org/10.1016/j.atmosenv.2014.08.061.
- Pei, X., L. Yang, W. Ji, X. Cheng, and X. Lu. 2023. “Global Validation and Comparison of MODIS DT and POLDER-3 GRASP Aerosol Products Over Ocean.” Atmospheric Research 281: 106480. https://doi.org/10.1016/j.atmosres.2022.106480.
- Petrenko, M., and C. Ichoku. 2013. “Coherent Uncertainty Analysis of Aerosol Measurements from Multiple Satellite Sensors.” Atmospheric Chemistry and Physics 13 (14): 6777–6805. https://doi.org/10.5194/acp-13-6777-2013.
- Remer, L. A., Y. J. Kaufman, D. Tanré, S. Mattoo, D. A. Chu, J. V. Martins, R. R. Li, et al. 2005. “The MODIS Aerosol Algorithm, Products, and Validation.” Journal of the Atmospheric Sciences 62 (4): 947–973. https://doi.org/10.1175/JAS3385.1.
- Remer, L. A., R. C. Levy, and J. V. Martins. 2024. “Opinion: Aerosol Remote Sensing Over the Next 20 Years.” Atmospheric Chemistry and Physics 24 (4): 2113–2127. https://doi.org/10.5194/acp-24-2113-2024.
- Remer, L. A., R. C. Levy, S. Mattoo, D. Tanré, P. Gupta, Y. Shi, V. Sawyer, et al. 2020. “The Dark Target Algorithm for Observing the Global Aerosol System: Past, Present, and Future.” Remote Sensing 12 (18): 2900. https://doi.org/10.3390/rs12182900.
- Remer, L. A., S. Mattoo, R. C. Levy, and L. A. Munchak. 2013. “MODIS 3 km Aerosol Product: Algorithm and Global Perspective.” Atmospheric Measurement Techniques 6 (7): 1829–1844. https://doi.org/10.5194/amt-6-1829-2013.
- Sawyer, V., R. C. Levy, S. Mattoo, G. Cureton, Y. Shi, and L. A. Remer. 2020. “Continuing the MODIS Dark Target Aerosol Time Series with VIIRS.” Remote Sensing 12 (2): 308. https://doi.org/10.3390/rs12020308.
- Sayer, A. M., N. C. Hsu, C. Bettenhausen, M. J. Jeong, B. N. Holben, and J. Zhang. 2012. “Global and Regional Evaluation of Over-Land Spectral Aerosol Optical Depth Retrievals from SeaWiFS.” Atmospheric Measurement Techniques 5 (7): 1761–1778. https://doi.org/10.5194/amt-5-1761-2012.
- Sayer, A. M., N. C. Hsu, J. Lee, N. Carletta, S. H. Chen, and A. Smirnov. 2017. “Evaluation of NASA Deep Blue/SOAR Aerosol Retrieval Algorithms Applied to AVHRR Measurements.” Journal of Geophysical Research: Atmospheres 122 (18): 9945–9967. https://doi.org/10.1002/2017JD026934.
- Sayer, A. M., N. C. Hsu, J. Lee, W. V. Kim, and S. T. Dutcher. 2019. “Validation, Stability, and Consistency of MODIS Collection 6.1 and VIIRS Version 1 Deep Blue Aerosol Data Over Land.” Journal of Geophysical Research: Atmospheres 124 (8): 4658–4688. https://doi.org/10.1029/2018JD029598.
- Sayer, A. M., L. A. Munchak, N. C. Hsu, R. C. Levy, C. Bettenhausen, and M.-J. Jeong. 2014. “MODIS Collection 6 Aerosol Products: Comparison Between Aqua's e-Deep Blue, Dark Target, and “Merged” Data Sets, and Usage Recommendations.” Journal of Geophysical Research: Atmospheres 119 (24): 13965–13989. https://doi.org/10.1002/2014JD022453.
- Shi, Y. R., R. C. Levy, L. Yang, L. A. Remer, S. Mattoo, and O. Dubovik. 2021. “A Dark Target Research Aerosol Algorithm for MODIS Observations Over Eastern China: Increasing Coverage While Maintaining Accuracy at High Aerosol Loading.” Atmospheric Measurement Techniques 14 (5): 3449–3468. https://doi.org/10.5194/amt-14-3449-2021.
- Singh, R., V. Singh, A. S. Gautam, S. Gautam, M. Sharma, P. S. Soni, K. Singh, and A. Gautam. 2022. “Temporal and Spatial Variations of Satellite-Based Aerosol Optical Depths, Angstrom Exponent, Single Scattering Albedo, and Ultraviolet-Aerosol Index Over Five Polluted and Less-Polluted Cities of Northern India: Impact of Urbanization and Climate Change.” Aerosol Science and Engineering 7 (1): 131–149. https://doi.org/10.1007/s41810-022-00168-z.
- Sinyuk, A., B. N. Holben, T. F. Eck, D. M. Giles, I. Slutsker, S. Korkin, J. S. Schafer, et al. 2020. “The AERONET Version 3 Aerosol Retrieval Algorithm, Associated Uncertainties and Comparisons to Version 2.” Atmospheric Measurement Techniques 13 (6): 3375–3411. https://doi.org/10.5194/amt-13-3375-2020.
- Sogacheva, L., T. Popp, A. M. Sayer, O. Dubovik, M. J. Garay, A. Heckel, N. C. Hsu, et al. 2020. “Merging Regional and Global Aerosol Optical Depth Records from Major Available Satellite Products.” Atmospheric Chemistry and Physics 20 (4): 2031–2056. https://doi.org/10.5194/acp-20-2031-2020.
- Su, X., M. Cao, L. Wang, X. Gui, M. Zhang, Y. Huang, and Y. Zhao. 2023a. “Validation, Inter-Comparison, and Usage Recommendation of Six Latest VIIRS and MODIS Aerosol Products Over the Ocean and Land on the Global and Regional Scales.” Science of the Total Environment 884: 163794. https://doi.org/10.1016/j.scitotenv.2023.163794.
- Su, X., L. Wang, M. Cao, L. Yang, M. Zhang, W. Qin, Q. Cao, Y. Yang, and L. Li. 2023b. “Fengyun 4A Land Aerosol Retrieval: Algorithm Development, Validation, and Comparison With Other Datasets.” IEEE Transactions on Geoscience and Remote Sensing 61: 1–16. https://doi.org/10.1109/tgrs.2023.3330544.
- Su, X., L. Wang, X. Gui, L. Yang, L. Li, M. Zhang, W. Qin, et al. 2022a. “Retrieval of Total and Fine Mode Aerosol Optical Depth by an Improved MODIS Dark Target Algorithm.” Environment International 166: 107343. https://doi.org/10.1016/j.envint.2022.107343.
- Su, X., L. Wang, M. Zhang, W. Qin, and M. Bilal. 2021. “A High-Precision Aerosol Retrieval Algorithm (HiPARA) for Advanced Himawari Imager (AHI) Data: Development and Verification.” Remote Sensing of Environment 253: 112221. https://doi.org/10.1016/j.rse.2020.112221.
- Su, X., Y. Wei, L. Wang, M. Zhang, D. Jiang, and L. Feng. 2022b. “Accuracy, Stability, and Continuity of AVHRR, SeaWiFS, MODIS, and VIIRS Deep Blue Long-Term Land Aerosol Retrieval in Asia.” Science of the Total Environment 832: 155048. https://doi.org/10.1016/j.scitotenv.2022.155048.
- Sun, K., Y. Gao, B. Qi, and Z. Yu. 2022. “The Influence of Underlying Land Cover on the Accuracy of MODIS C6.1 Aerosol Products – A Case Study Over the Yangtze River Delta Region of China.” Remote Sensing 14 (4): 938. https://doi.org/10.3390/rs14040938.
- Verma, S., D. Prakash, A. K. Srivastava, and S. Payra. 2017. “Radiative Forcing Estimation of Aerosols at an Urban Site Near the Thar Desert Using Ground-Based Remote Sensing Measurements.” Aerosol and Air Quality Research 17 (5): 1294–1304. https://doi.org/10.4209/aaqr.2016.09.0424.
- Wang, Q., S. Li, J. Yang, D. Zhou, and G. Song. 2023. “A High-Precision Aerosol Retrieval Algorithm for FY-3D MERSI-II Images.” Environment International 173: 107841. https://doi.org/10.1016/j.envint.2023.107841.
- Wang, Y., Q. Yuan, T. Li, H. Shen, L. Zheng, and L. Zhang. 2019. “Evaluation and Comparison of MODIS Collection 6.1 Aerosol Optical Depth Against AERONET Over Regions in China with Multifarious Underlying Surfaces.” Atmospheric Environment 200: 280–301. https://doi.org/10.1016/j.atmosenv.2018.12.023.
- Wei, J., Z. Li, Y. Peng, and L. Sun. 2019. “MODIS Collection 6.1 Aerosol Optical Depth Products Over Land and Ocean: Validation and Comparison.” Atmospheric Environment 201: 428–440. https://doi.org/10.1016/j.atmosenv.2018.12.004.
- Wei, J., and L. Sun. 2017. “Comparison and Evaluation of Different MODIS Aerosol Optical Depth Products Over the Beijing-Tianjin-Hebei Region in China.” IEEE Journal of Selected Topics in Applied Earth Observations and Remote Sensing 10 (3): 835–844. https://doi.org/10.1109/JSTARS.2016.2595624.
- Xu, N., X. Niu, X. Hu, X. Wang, R. Wu, S. Chen, L. Chen, et al. 2018. “Prelaunch Calibration and Radiometric Performance of the Advanced MERSI II on FengYun-3D.” IEEE Transactions on Geoscience and Remote Sensing 56 (8): 4866–4875. https://doi.org/10.1109/TGRS.2018.2841827.
- Yang, L., X. Hu, H. Wang, X. He, P. Liu, N. Xu, Z. Yang, and P. Zhang. 2022. “Preliminary Test of Quantitative Capability in Aerosol Retrieval Over Land From MERSI-II Onboard Fengyun-3D.” National Remote Sensing Bulletin 26 (5): 923–940. https://doi.org/10.11834/jrs.20210286.
- Yang, Z., P. Zhang, S. Gu, X. Hu, S. Tang, L. Yang, N. Xu, et al. 2020. “Capability of Fengyun-3D Satellite in Earth System Observation.” Journal of Meteorological Research 33 (6): 1113–1130. https://doi.org/10.1007/s13351-019-9063-4.
- Zhao, H., K. Gui, Y. Ma, Y. Wang, Y. Wang, H. Wang, Y. Zheng, et al. 2021. “Climatological Variations in Aerosol Optical Depth and Aerosol Type Identification in Liaoning of Northeast China Based on MODIS Data from 2002 to 2019.” Science of the Total Environment 781: 146810. https://doi.org/10.1016/j.scitotenv.2021.146810.