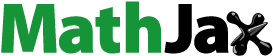
ABSTRACT
The 20-year war in Afghanistan has had a catastrophic impact on agricultural planting and food security, but the assessment was difficult due to a lack of existing statistics. We used 30m Landsat data to extract the annual planting area and built the time series to measure the condition of agriculture, and used constant nighttime light data to quantify the impact of the war. Combined with climate, Digital Elevation Model (DEM) data, etc., we quantitatively analysis the impact of the war using regression model. From a time perspective, the total planting area reached peek during some period of relative pacification, but maintained a low level of planting most time. From a spatial perspective, the main planting areas shifted from east to west. Both poppy and wheat planting have a high correlation with non-natural factor, while wheat affected more by natural conditions compared with poppy. In addition, the cultivation of wheat is limited by poppy. As a verification, we collect relative history records to explain the rationality of the result of analysis. According to the result, we reveal the adverse effects of war on agricultural planting and food security in Afghanistan and provide a reference for relevant researches on war and agriculture.
1. Introduction
The Afghanistan war is the longest war in American history and has had a severe impact on Afghanistan (Knickmeyer Citation2021). It is estimated that the war has directly caused the deaths of 241,000 people (Watson Institute Citation2021), while injuries, hunger and disease have indirectly caused the deaths of hundreds of thousands of people. In addition to casualties, the protracted war has also caused serious damage to the domestic environment; at least 2.7 million of Afghanistan’s 38 million people are refugee in exile, and 4 million refugees are displaced within Afghanistan (The UN Refugee Agency Citation2022). In addition, the war has further affected normal population growth, as Afghanistan’s poverty rate has remained high, increasing from 33.7% in 2007 to 54.5% in 2016 (World Bank Citation2017). In addition to the direct damage to farmland, the long war has disturbed people’s normal production activities, even forcing many people to migrate, making it difficult to engage in agricultural production. As a result, Afghanistan remains in a serious food crisis. In the immediate aftermath of the war in 2021, wheat production in Afghanistan was reduced by 12.18% (Index Mundi Citation2023). There is a food security crisis in 11 of the 33 provinces, and the remaining provinces are at risk. About 98% of people do not have enough to eat and wear (FEWS NET Citation2022; Gibson, Campbell, and Wynne Citation2012). This situation is expected to be difficult to improve over the next 5 years.
Analyzing the agriculture situation of in Afghanistan and planning for future development is an urgent task in order to solve the food crisis. However, the long duration and wide coverage of the Afghanistan war has led to a lack of existing statistics (Verwimp and Muñoz-Mora Citation2018). A large number of studies show that earth observation satellites can provide direct and relatively rich data sources (Atesoglu et al. Citation2020), and that remote sensing can be effectively used in crop monitoring (Yao et al. Citation2020). In 2007, E. Prins used Landsat ETM+ data to map the widespread burned villages in Sudan with high accuracy (Prins Citation2008); in 2010, John J. Sulik also used semi-automated GIS and RS techniques to assess damage in Darfur, Sudan (Nackoney et al. Citation2014). Then, in 2013, Jenet Nackoney used Landsat TM and ETM+ to monitor wartime patterns of human migration and resource use in the Democratic Republic of Congo. In 2022, Duan pointed out that the use of time series in classification significantly improves the accuracy. In 2017, Wei Jiang focused on nighttime lights, and use time series nighttime light images from the Suomi National Polar-orbiting Partnership Visible Infrared Imaging Radiometer Suite sensor (NPP-VIIRS) to assess the Yemen crisis (Jiang et al. Citation2017). In 2021, Xi-Ya Li combined daytime and nighttime satellite data and comprehensively assessed the impact of violent conflict on Syrian agriculture (Li et al. Citation2022). These studies used nighttime light (NTL) data and daytime satellite data to assess the destruction of human settlements (Cheng et al. Citation2007; Gbanie, Griffin, and Thornton Citation2018) and loss of agricultural land as indirect evidence of war conflicts.
An analysis based on Moderate-resolution Imaging Spectroradiometer (MODIS) data and the vegetation index showed that due to the high economic dependance on the drug trade, Afghanistan’s annual output of drug exceeded 90% of the world’s output even during the war (Taylor et al. Citation2010). However, the planting of food crops in Afghanistan is not optimistic due to its continuously high level of poverty and ongoing food crisis. Due to years of conflict, the planting areas in various regions are frequently disturbed, which substantially impacted production. Additionally, Afghanistan’s natural ecology limits planting to specific areas, which gradually become more stable (Akhtar et al. Citation2018).
Current research primarily focuses on the wartime period before 2010 (Beck Citation2003; de Beurs and Henebry Citation2008). However, a comprehensive summary of the entire 20-year Afghanistan war is needed. Considering the long-term and complex geographical environment, existing researches suggests the inclusion of nighttime light, precipitation and Digital Elevation Model (DEM) data in the assessment of the Afghanistan war. Furthermore, in order to address the food crisis, it is essential to gain an understanding of the cultivation of major crops in Afghanistan. Therefore, in this study, we assess the impact of the war in Afghanistan from 2001 to 2021 on the planting area of major food crops (wheat) and cash crops (poppy). 30 m-resolution images offered by Landsat 5/7/8 satellite, level-2, collection-2 (which means the images have undergone radiometric correction and geometric correction), were used to create a sample dataset of main crops. The annual planting area of crops on a national scale was then extracted using a random forest model. Satellite-derived nighttime light changes were used to reflect the intensity of war across the country, particularly in urban areas. Regression analysis was used to quantitatively analyze the contributions of precipitation and war conflict to cultivated land change by. Additionally, historical reports on Afghanistan war are selected to verify and explain the attribution.
2. Materials and methods
2.1. Data
2.1.1. Landsat remote sensing data
For classification between 2000 and 2011, Landsat 7 Level 2 Collection 2 Tier 1 data was used, while Landsat 8 Level 2 Collection 2 Tier 1 data was used for 2012–2021. The Landsat 7 and Landsat 8 satellites have a revisit period of 16 days. The dataset is geometrically corrected to L2 (L2TP), and atmospheric correction is performed using the Landsat Ecosystem Disturbance Adaptive Processing System (LEDAPS) and Land Surface Reflectance Code (LASRC), which includes cloud, shadow, water, and snow masks. The product includes four visible and near-infrared bands, two shortwave infrared bands, and one thermal infrared band. Both the visible and infrared bands have a resolution of 30 m. Images from Landsat7 have the issue of striping, while we use the method offered by Google, using Landsat5 data to fill them. All images have undergone cloud removal processing, using the mask function offered by GEE. As a result, we obtained 1275 Landsat5 and 2508 images from 2000 to 2011, 3128 Landsat8 images and 3397 Landsat7 images from 2012 to 2021 (Wulder et al. Citation2019).
2.1.2. Nighttime light data
The two most commonly used NTL data sources were selected for this study. The first source is the annual synthesis of Defense Meteorological Satellite Program/Operational Linescan System (DMSP/OLS) images. These images measure global nighttime light data from 1992 to 2013, with a spatial resolution of 30 arc seconds. This product provides a stable light band that records global persistent lighting. The second data source is the annual synthetic image of the visible infrared imaging radiometer component on the NPP/VIIRS. We use the V2 version, which measures global nighttime light data from 2012 to the present. The product records the annual average embedded nighttime lights by synthesizing the average field, with a spatial resolution of 15 arc seconds. To construct the time series, we resample the data to maintain a consistent scale and use the NTL data from 2012, which is available for both products, to achieve alignment (He et al. Citation2014).
2.1.3. Geographic data
The precipitation data used in this study are sourced from the fifth generation of the ECMWF atmospheric reanalysis of the global climate (ERA5) reanalysis dataset, produced by the European Centre for Medium Range Weather Forecasts (ECMWF). It has a resolution of 0.5°, and contains 137 layers of atmospheric data. The precipitation data for the year is based on the monthly average data from July of the previous year to June, considering that the phenological periods of poppy and wheat in Afghanistan are approximately from March to June.
The DEM data, with a resolution of 10 m, are obtained from the University of Texas database.
2.2. Methods
The experiment mainly includes three parts plus a verification. The mainly process of experiment is presented below and the verification is presented in Section 3.2.2 ().
Figure 1. Overview chart. (a) Nighttime light time series extraction. (b) Planting area time series extraction. (c) Regression analysis. (d) Further analysis.

2.2.1. Remote sensing classification
Based on the major crop types and calendar in Afghanistan, the vegetation growth window was set from March to June (Tiwari et al. Citation2020), and a sampling interval of 15 days was used. For the study area, 400 true colors images were selected at each time point from the Landsat 7 Enhanced Thematic Mapper Plus (ETM+) sensor Level 2, Collection 2, Tier 1 dataset, as well as from the Landsat 8 Operational Land Imager (OLI) and Thermal Infrared Sensor (TIRS) Level 2, Collection 2, Tier 1 dataset. Seven features were used in the mapping of cropland, including the red band (R), near-infrared band (NIR), shortwave infrared band (SWIR) (Tiwari et al. Citation2020; Welle et al. Citation2005), as well as the normalized difference vegetation index (NDVI) (Hestir et al. Citation2008; Park et al. Citation1997), normalized difference water index (NDWI) (Brown, Steckler, and Anderson Citation1994; Vrindts, De Baerdemaeker, and Ramon Citation2002) and slope calculated from DEM data (Hao et al. Citation2019). Statistical metrics were derived from Landsat data for both the growing and non-growing seasons for each year from 2000 to 2021.
Figure 2. Extraction of crop planting area in 2000. (a) The time series of true color image in April, May and June; (b) the result for cultivation area of wheat and poppy in Afghanistan; (c) the origin true color image over cropland; and (d) the result of extraction.

A supervised classification approach was employed to map annual productive cropland in Afghanistan. The training dataset was built through visual interpretation, following the method officially released by The United Nations Office on Drugs and Crime (UNODC) (). The phenological period of poppy and wheat in Afghanistan is approximately from March to June. Leaves typically begin to grow in March every year, and are at their most luxuriant in April. In May, the poppy begins to be harvested while the wheat matures. Then in June, poppy and wheat are both harvested. To ensure the representativeness and accuracy of the samples, we mainly start from two perspectives: I. the training samples need to involve as much cultivated land as possible, covering mountains, plains and other terrain, to ensure the diversity of samples. II. It is necessary to refer to the time series in the process of drawing samples when the colors are easily confused. Land cover types including but not limited to poppy and wheat, should be divided into other plants, bare land and water based on the actual situation. Each year, 40–50 samples were marked for wheat, poppy and other plants, while 20–30 samples were marked for bare land and water areas are marked with 20–30 samples due to their distinct characteristics.
The random forest (RF) method was chosen as the classification algorithm for its demonstrated effectiveness in extracting crop planting areas (Pal Citation2005; Yan et al. Citation2021). The classifier was trained using Google Earth Engine, and the trained RF model was used to create time series data on the planting areas of major crops. The dataset was divided into a training set and a validation set in a 7:3ratio. The accuracy of the training model on the validation set was over 90% ().
Table 1. Classification accuracy.
2.2.2. NTL quantitative war impact model
Existing research suggests that in conflict areas, NTLs are typically negatively correlated with conflicts in the region, and severe conflicts can lead to a decline in NTLs (Xu et al. Citation2023). In addition, this study uses multisource data for cross-checking, including news reports, areas controlled by each regime, and other relevant information.
The cross-examination results indicate a general increase in NTL data values in Afghanistan, although there were some decreases in certain stages. International assistance has continuously improved the power and lighting systems in Afghanistan. However, relevant news reports on the war in Afghanistan suggest a negative correlation between nighttime light values and military or other activities. According to , the war began in 2001, but due to Afghanistan’s poor development, the impact on nighttime light data was not significant, with a decrease of less than 0.01. The second time nighttime light data values fell was in 2002, when the US-led army launched Operation Mori as the decisive battle of the first stage. The Taliban’s resurgence in 2005 and subsequent surge in terrorist attacks from 2006 to 2008 resulted in a decline of nighttime light data values to varying degrees. In 2010, the United States increased troop number and launched an attack on Helmand Province, a Taliban stronghold. In 2015, the Taliban further increased their attacks, including bombings of the parliament building and airport in Kabul. These two violent events resulted in a substantial decline in nighttime light data values. In 2017, the US military dropped the most powerful non-nuclear bomb on an Al Qaeda gathering, causing infrastructure damage and a subsequent decline in nighttime light data values. The use of noctilucent data to quantitatively measure the impact of war is significant.
2.2.3. Cropland change attribution
The alteration in crop planting area during the war in Afghanistan is primarily attributed to natural and non-natural factors. Numerous natural factors such as precipitation, evapotranspiration and temperature can affect the area of productive farmland. Precipitation in particular, has high variability and can be directly measured. Non-natural factors, such as labor transfer, irrigation infrastructure, agricultural markets, and changes in regime policies are also affected by war. The change in planting area during the war in Afghanistan can be attributed to the impact of precipitation and conflict. It is important to note that this conclusion is based on evidence from relevant studies and the current situation in Afghanistan.
The linear regression model was chosen for the study. The model was established the at the national level ().
(1)
(1)
refers to the planting area of each major crop in Afghanistan in a given year,
refers to precipitation and
refers to nighttime light value across the whole country for that year,
is a fixed value representing the time effect, and
is the error term. Through the comparison from two dimensions, we can compare the different effects of the same crop by two factors and the different effects of the same factor on two crops, in order to analyze the planting situation of major crops in Afghanistan.
Table 2. Regression coefficient of nation model diagram.
3. Result and discussion
3.1. Overview of main crop in Afghanistan during wartime
Throughout the war in Afghanistan (2000–2021), the average annual wheat planting area was 0.61 Mha, while the poppy area was 2.17 Mha. The coefficient of variation for poppy cultivation area was 0.6297, compared to 1.4029 for wheat. The wheat planting area was typically less than 0.60 Mha, with the smallest area recorded in 2018 at only 36 Kha, and the largest in 2015 at 3.31 Mha. Poppy cultivation has consistently remained at a high level. The smallest area for poppy cultivation was recorded in 2001, at only 0.15 Mha, while the highest point was reached in 2015 with 4.93 Mha. The data indicates that the area used for planting poppy was three times that of area used for planting wheat. Additionally, the coefficient of variation for poppy is less than half that of wheat.
Both wheat and poppy planting areas in Afghanistan exhibited significant fluctuations, following a similar trend. As illustrated in and , wheat planting decreased sharply in 2001 and continued to decline until 1 year after 2003, and reached a low point in 2005 again. Subsequently, wheat planting remained low until 2010, after which it began to increase the wheat planting area then reached its peak in 2015. However, in just 3 years, wheat planting reached a new low point that continued until the end of the war in 2021. Poppy planting also shows a periodicity, as it also suffered during the middle of each stage, but it recovered more quickly than wheat. The illustrates that the area of poppy planting was at its lowest level at the end of the war, but increased during the middle of each stage (2001, 2005, 2010, 2018), before decreasing again at the end of the subsequent stage of the war. Crop planting reached a high level but was unable to be maintained due to the war. In particular, wheat planting remained at a low level for most of the time.
Figure 4. The planting conditions of Afghanistan 2000–2021. (a) Shows the frequency of main crop cultivating, which is defined by the number of years a pixel of map used as cropland. (b) The overall cultivation area of wheat and poppy in Afghanistan 2000–2021. (c) Annual cultivation area change compared to the previous year.

Figure 5. Annual productive cropland area for each region between 2000 and 2021. Afghanistan is officially divided into seven administrative regions, the line charts show cropland area change time series of each region.

shows that the western region has the largest average planting area for poppy and wheat, while the southern region has the smallest. The central region has the highest variation coefficient for poppy, while the southwestern region has the highest for wheat. The lowest variation coefficient for poppy is found in the southwestern region, while the lowest for wheat is in the eastern region. Crop planting in Afghanistan is mainly concentrated in the west and northeast, with scattered planting in other areas.
Figure 6. Average productive cropland area in each province and the trend of change. (a) The amount of annual wheat planting area distribution in each province. (b) The data maps the change trade of annual wheat planting area, which is measured by the result of linear regression of area-year. (c) The area of poppy. (d) The trade of poppy.

The wheat planting area in the west has significantly increased after 2010, while in the east, the area is smaller with insignificant increase or even decline. Furthermore, the wheat growing areas in Afghanistan have shown a gradual trend of low yield to high yield. illustrates that provinces with less wheat planting area also exhibit a downward trend. The distribution of poppy planting shows similarities with concentration in the western region and more planting in Badakhshan province in North-East region. However, there are significant gaps in poppy planting between the east and west. Additionally, the poppy planting area generally increased after 2010 in the west, while in the east it remained at a lower level than 2003 until now. The planting area shows a trend of transferring from northeast to southwest ().
Table 3. Average productive cropland area in each province and the trend of change.
3.2. Attribution of major productive agricultural crops
3.2.1. Regression analysis of influencing factors
The climate in Afghanistan is primarily continental. Therefore, agricultural production is theoretically dependent on precipitation, excluding war factors. The model considers precipitation and nighttime lights measured using nighttime light as the two factors. The regression model of the original data indicates significance, while the standardized data shows the relative relationship between features. The results demonstrate the different cultivation situations of the major crops ().
Table 4. Regression coefficient of nation model diagram.
Regarding wheat, precipitation is a significant factor that restricts planting during wartime, with the exception of the considerable impact of war itself (Sharma, Sonder, and Sika Citation2015). The statistics indicate that the P value of nighttime lights is less than 0.01, while that of precipitation is less than 0.005, demonstrating the substantial impact of both natural and war factors. Furthermore, based on the standardized data, the precipitation estimate is 1.03206, while of the estimate for nighttime lights is 0.17867. This suggests that nature has a more significant impact on wheat cultivation, even during times of war. In other words, although war disrupts wheat cultivation, natural features also severely limit wheat production. Wheat planting in Afghanistan is equally affected by war while adhering to natural crop planting rules. Due to limited natural conditions, improving crop cultivation in Afghanistan remains challenging after the war (Akhtar et al. Citation2018; Kawasaki et al. Citation2012).
The regression analysis for poppy cultivation in Afghanistan reveals a significant positive correlation with the level of economic development, as measured by the nighttime light level. However, there is little or no relationship between poppy cultivation and precipitation, as indicated by the non-significant P value of 0.23 and t value of −1.249 for precipitation and significant P value of 0.000103 for nighttime light. The data indicates a significant correlation between poppy planting and development in Afghanistan. Poppy is the primary cash crop on which Afghanistan depends, and it is more susceptible to economic factors than natural conditions. In other words, Afghanistan had to allocate sufficient resources to poppy planting to overcome adverse environmental conditions and ensure basic overheads, particularly for the army.
Wheat planting is limited by various factors, including poppy cultivation, in addition to natural and non-natural factors. The estimate of nighttime lights for wheat is 0.17867, while the estimate for poppy is 0.5379, which is triple that of wheat. This suggests that poppy is more affected by non-natural factors than wheat. Furthermore, Afghanistan has shown a preference for investing in poppy planting over wheat, despite facing a severe food crisis (Kienberger et al. Citation2017). This highlights the country’s reliance on opium poppy cultivation for economic growth. While it is encouraging that Afghanistan has redirected resources toward wheat and other food crops following the Taliban’s ban on opium poppy cultivation, it is crucial for the country to explore alternative forms of economic development beyond the drug trade.
3.2.2. Time series analysis explaining for attribution
The Afghanistan war can be divided into four stages. The first stage was from 2001 to the beginning of 2002, during which the Taliban was expelled from their base areas in Hilmand and Kandahar provinces. This resulted in serious damage to crop planting in 2001 (Akresh, Verwimp, and Bundervoet Citation2011), particularly in the west and south which are the main production areas for opium poppy. The second stage began in 2003, and was marked by intensive terrorist attacks, with the Taliban targeting Afghanistan’s infrastructure. Poppy cultivation increased after 2002, while wheat production remained low until 2004 when foreign aid was provided to help Afghanistan rebuild (Goodhand Citation2002). Following the Taliban’s resurgence in 2005, they carried out frequent terrorist attacks until 2010. The US increased the number of troops to combat the Taliban, and wheat planting began to pick up after 2011. However, following the international coalition’s attacks on the Taliban, poppy planting in Afghanistan arrived a new low point. The third stage began in 2009, with the US sending more troops to Afghanistan. A change in countermeasures occurred with the US military planning to focus more on protecting people from insurgents rather than trying to kill a large number of militants. During this stage, both wheat and poppy planting in Afghanistan experienced a rebound. In the west, the planting area was notably higher. Following the formal end of North Atlantic Treaty Organization (NATO)’s combat mission in 2015, the wheat planting area reached its peak. However, in 2016, the fourth stage began with a Taliban counterattack. They increased their attacks, bombing the parliament building and airport in Kabul, and carrying out multiple suicide bombings. The crop planting area in Afghanistan Experienced a decline once again, reaching a new low point in 2018 due to the breakdown of peace negotiations between Taliban and the US, leading to further conflict. The difference is that the Taliban gained the upper hand. Since then, wheat planting has not recovered, while poppy planting has remained at a high level until 2021, when the Taliban launched an all-out counterattack and eventually gained leadership in Afghanistan.
Wheat planting was destroyed more serious by war activities than poppy. During wartime, poppy cultivation was more prevalent than wheat cultivation during wartime, especially for Taliban, as shown in . The area controlled by the Taliban saw less destruction of poppy planting compared to wheat planting. This is due to the important role of drug trade in Afghanistan’s economic income (Goodhand Citation2005). Afghanistan imposes a tax of 10% to 20% on poppy cultivation and trade, which generated an income of $40 million in 1999 and has since increased to $1.4 billion in 2021, covering 29% of the entire agricultural sector’s value in 2021. This has led to a preference for poppy cultivation to ensure high levels of drug production. Furthermore, due to poverty resulting from the conflict, more people have returned to poppy cultivation. In other words, the war has inadvertently contributed to the growth of poppy cultivation. The regression results indicate the positive impact of planting, which, when combined with the historical context, suggests a certain degree of association between poppy planning and Taliban activities. This may explain why poppy planting was concentrated in the west. The central and eastern regions have less arable land and more urban areas, making them unsuitable for crop planting. As a result, wheat planting area has declined. In contrast, the west and the northwest regions have large areas for poppy cultivation due to their relatively superior natural conditions. Additionally, the presence of the NATO garrison in close proximity to Kabul has had significant impact. As demonstrated in , the area used for poppy planting decreased after NATO’s arrival. However, the southwest region, which was controlled by the Taliban has one of the highest growth rates and the widest areas for poppy planting, despite the similar unsuitable natural conditions. In conclusion, shows that poppy planting is concentrated in areas controlled by the Taliban and the Northern Alliance, whereas less planting occurs in areas controlled by the Afghanistan government and the international coalition (Piazza Citation2012).
4. Conclusion
This study constructed a time series of major crop plantings in Afghanistan from 2000 to 2021 at multiple spatial scales, showing the evolution and changes in crop plantings during the war in Afghanistan using Landsat remote sensing. Contributed a quantitative attribution model to analyze the attribution of changes in the area under major crops in Afghanistan.
Wheat planting in Afghanistan has always been at a low level of development with significant fluctuations, which is the main reason for the food crisis. Wheat planting area in Afghanistan experienced an upward trend from 2015 to 2017, during which it was relatively stable. However, with the resumption of the war, it has returned to the planting levels of 20 years ago. Wheat area in Afghanistan is concentrated in the western region and less concentrated in the central and eastern regions. Wheat cultivation tended to be centralized and, under the influence of the war, has moved to areas with high levels of cultivation. Conversely, poppy cultivation in Afghanistan remained at high levels and was less disrupted by the war. Poppy planting was mainly concentrated in the southwest region controlled by the Taliban and the northern region controlled by the Northern Alliance. By the end of the war, the planting area showed an upward trend. The central and eastern regions, where NATO troops were mainly deployed, there was less poppy cultivation and the change in planting area showed a downward trend.
The war had a severe impact on crop cultivation in Afghanistan, particularly wheat. However, international aid has significantly improved the level of infrastructure in Afghanistan, which has also benefited agricultural development. After the Taliban took power, they pledged to ban poppy cultivation. So it is time to develop the country’s agriculture by fully utilizing its resources and finding a method of economic development that will help solve the food crisis.
5. List of abbreviations
Disclosure statement
No potential conflict of interest was reported by the author(s).
Data availability statement
The data that support the findings of this study are openly available on Google Earth Engine at https://code.earthengine.google.com, where can get the data by reference key words ‘LANDSAT/LE07/C02/T1_L2’, ‘LANDSAT/LT05/C02/T1_L2’ and ‘LANDSAT/LC08/C02/T1_L2’.
Additional information
Funding
References
- Akhtar, Fazlullah, Usman Khalid Awan, Bernhard Tischbein, and Umar Waqas Liaqat. 2018. “Assessment of Irrigation Performance in Large River Basins under Data Scarce Environment—A Case of Kabul River Basin, Afghanistan.” Remote Sensing 10 (6): 972. https://doi.org/10.3390/rs10060972.
- Akresh, Richard, Philip Verwimp, and Tom Bundervoet. 2011. “Civil War, Crop Failure, and Child Stunting in Rwanda.” Economic Development and Cultural Change 59 (4): 777–810. https://doi.org/10.1086/660003.
- Atesoglu, Ayhan, Emre Aktürk, Aliakbar Rasouli, Serdar Erpay, and Halil Baris Ozel. 2020. “Monitoring of Land-Cover/Land-Use Changes in Syria by Involving the Collect Earth Methodology Approach.” Fresenius Environmental Bulletin 29 (12): 11032–11041.
- Beck, R. A. 2003. “Remote Sensing and GIS as Counterterrorism Tools in the Afghanistan War: A Case Study of the Zhawar Kili Region.” The Professional Geographer 55 (2): 170–179. https://doi.org/10.1111/0033-0124.5502005.
- Brown, R. B., J. P. Steckler, and G. W. Anderson. 1994. “Remote Sensing for Identification of Weeds in No-Till Corn.” Transactions of the ASAE 37 (1): 297–302. https://doi.org/10.13031/2013.28084.
- Cheng, Liyu, Yi Zhou, Litao Wang, Shixin Wang, and Cong Du. 2007. “An estimate of the city population in China using DMSP night-time satellite imagery.” IEEE International Geoscience and Remote Sensing Symposium 691–694. https://doi.org/10.1109/IGARSS.2007.4422890.
- de Beurs, K. M., and G. M. Henebry. 2008. “War, Drought, and Phenology: Changes in the Land Surface Phenology of Afghanistan since 1982.” Journal of Land Use Science 3 (2–3): 95–111. https://doi.org/10.1080/17474230701786109.
- FEWS NET. 2022. “Conflict and Poor Agricultural Production Expected to Drive Deteriorating Food Security in Afghanistan.” https://fews.net/central-asia/afghanistan/food-security-outlook/june-2021.
- Gbanie, Solomon Peter, Amy L. Griffin, and Alec Thornton. 2018. “Impacts on the Urban Environment: Land Cover Change Trajectories and Landscape Fragmentation in Post-War Western Area, Sierra Leone.” Remote Sensing 10 (1): 129. https://doi.org/10.3390/rs10010129.
- Gibson, Glen R., James B. Campbell, and Randolph H. Wynne. 2012. “Three Decades of War and Food Insecurity in Iraq.” Photogrammetric Engineering & Remote Sensing 78 (8): 885–895. https://doi.org/10.14358/PERS.78.8.895.
- Goodhand, Jonathan. 2002. “Aiding Violence or Building Peace? The Role of International Aid in Afghanistan.” Third World Quarterly 23 (5): 837–859. https://doi.org/10.1080/0143659022000028620.
- Goodhand, J. 2005. “Frontiers and Wars: The Opium Economy in Afghanistan.” Journal of Agrarian Change 5 (2): 191–216. https://doi.org/10.1111/j.1471-0366.2005.00099.x.
- Hao, Wang, Qin Lai-An, Jing Xu, He Feng, Tan Feng-Fu, and Hou Zai-Hong. 2019. “Research of Identification of Papaver Based on Spectral Analysis.” Chinese Journal of Quantum Electronics 36 (2): 151–155.
- He, Chunyang, Qun Ma, Zhifeng Liu, and Qiaofeng Zhang. 2014. “Modeling the Spatiotemporal Dynamics of Electric Power Consumption in Mainland China Using Saturation-Corrected DMSP/OLS Nighttime Stable Light Data.” International Journal of Digital Earth 7 (12): 993–1014. https://doi.org/10.1080/17538947.2013.822026.
- Hestir, E. L., S. Khanna, M. E. Andrew, M. J. Santos, J. H. Viers, J. A. Greenberg, and S. L. Ustin. 2008. “Identification of Invasive Vegetation Using Hyperspectral Remote Sensing in the California Delta Ecosystem.” Remote Sensing of Environment 112 (11): 4034–4047. https://doi.org/10.1016/j.rse.2008.01.022.
- Index Mundi. 2023. “Afghanistan Wheat Production by Year.” https://www.indexmundi.com/agriculture/?country=af&commodity=wheat&graph=production.
- Jiang, W., G. He, T. Long, and H. Liu. 2017. “Ongoing Conflict Makes Yemen Dark: From the Perspective of Nighttime Light.” Remote Sensing 9: 798. https://doi.org/10.3390/rs9080798.
- Kawasaki, Shinji, Fumio Watanabe, Shinji Suzuki, Ryuzo Nishimaki, and Satoru Takahashi. 2012. “Current Situation and Issues on Agriculture of Afghanistan.” Journal of Arid Land Studies 22 (1): 345–348.
- Kienberger, Stefan, Raphael Spiekermann, Dirk Tiede, Irmgard Zeiler, and Coen Bussink. 2017. “Spatial Risk Assessment of Opium Poppy Cultivation in Afghanistan: Integrating Environmental and Socio-Economic Drivers.” International Journal of Digital Earth 10 (7): 719–736. https://doi.org/10.1080/17538947.2016.1250828.
- Knickmeyer, Ellen. 2021. “Costs of the Afghanistan War, in Lives and Dollars.” AP News. https://apnews.com/article/middle-east-business-afghanistan-43d8f53b35e80ec18c130cd683e1a38f.
- Li, Xi-Ya, Xi Li, Ziying Fan, Li Mi, Tarek Kandakji, Zhen Song, Deren Li, and Xiao-Peng Song. 2022. “Civil War Hinders Crop Production and Threatens Food Security in Syria.” Nature Food 3: 38–46. https://doi.org/10.1038/s43016-021-00432-4.
- Nackoney, Janet, Giuseppe Molinario, Peter Potapov, Svetlana Turubanova, Matthew C. Hansen, and Takeshi Furuichi. 2014. “Impacts of Civil Conflict on Primary Forest Habitat in Northern Democratic Republic of the Congo, 1990–2010.” Biological Conservation 170: 321–328. https://doi.org/10.1016/j.biocon.2013.12.033.
- Pal, M. 2005. “Random Forest Classifier for Remote Sensing Classification.” International Journal of Remote Sensing 26 (1): 217–222. https://doi.org/10.1080/01431160412331269698.
- Park, R. S., F. J. Gordon, R. E. Agnew, R. J. Barnes, and R. W. J. Steen. 1997. “The Use of Near Infrared Reflectance Spectroscopy on Dried Samples to Predict Biological Parameters of Grass Silage.” Animal Feed Science and Technology 68 (3–4): 235–246. https://doi.org/10.1016/S0377-8401(97)00055-2.
- Piazza, James A. 2012. “The Opium Trade and Patterns of Terrorism in the Provinces of Afghanistan: An Empirical Analysis.” Terrorism and Political Violence 24 (2): 213–234. https://doi.org/10.1080/09546553.2011.648680.
- Prins, E. 2008. “Use of Low Cost Landsat ETM+ to Spot Burnt Villages in Darfur, Sudan.” International Journal of Remote Sensing 29 (4): 1207–1214. https://doi.org/10.1080/01431160701730110.
- Sharma, Rajiv, Kai Sonder, and G. Sika. 2015. “Potential Impact of Climate Change Trends on Wheat Production and Mitigation Strategies in Afghanistan.” Journal of Agricultural Science 7 (4): 40.
- Taylor, J. C., T. W. Waine, G. R. Juniper, D. M. Simms, and T. R. Brewer. 2010. “Survey and Monitoring of Opium Poppy and Wheat in Afghanistan: 2003–2009.” Remote Sensing Letters 1 (3): 179–185. https://doi.org/10.1080/01431161003713028.
- Tiwari, Varun, Mir A. Matin, Faisal M. Qamer, Walter Lee Ellenburg, Birendra Bajracharya, Krishna Vadrevu, Begum Rabeya Rushi, and Waheedullah Yusafi. 2020. “Wheat Area Mapping in Afghanistan Based on Optical and SAR Time-Series Images in Google Earth Engine Cloud Environment.” Frontiers in Environmental Science 8, https://doi.org/10.3389/fenvs.2020.00077.
- UNHCA (The UN Refugee Agency). 2022. “Afghanistan Refugee Crisis Explained.” https://www.unrefugees.org/news/afghanistan-refugee-crisis-explained.
- Verwimp, P., and J. C. Muñoz-Mora. 2018. “Returning Home after Civil War: Food Security and Nutrition among Burundian Households.” The Journal of Development Studies 54 (6): 1019–1040. https://doi.org/10.1080/00220388.2017.1311407.
- Vrindts, E., J. De Baerdemaeker, and H. Ramon. 2002. “Weed Detection Using Canopy Reflection.” Precision Agriculture 3 (1): 63–80. https://doi.org/10.1023/A:1013326304427.
- Watson Institute. 2021. “Human Cost of Post-9/11 Wars: Direct War Deaths in Major War Zones, Afghanistan & Pakistan (Oct. 2001–Aug. 2021); Iraq (March 2003–Aug. 2021); Syria (Sept. 2014–May 2021); Yemen (Oct. 2002–Aug. 2021) and Other Post-9/11 War Zones, Cost of War.” https://watson.brown.edu/costsofwar/figures/2021/WarDeathToll.
- Welle, Roland, Willi Greten, Thomas Müller, Gary Weber, and Hartwig Wehrmann. 2005. “Application of Near Infrared Spectroscopy On-Combine in Corn Grain Breeding.” Journal of Near Infrared Spectroscopy 13 (2): 69–75. https://doi.org/10.1255/jnirs.459.
- World Bank. 2017. “Poverty in Afghanistan: Results Based on ALCS 2016–17.” © World Bank. https://www.google.com.hk/url?sa=t&rct=j&q=&esrc=s&source=web&cd=&ved=2ahUKEwihlsGrzKX5AhXIHIgKHfgADGsQFnoECAUQAQ&url=http%3A%2F%2Fdocuments1.worldbank.org%2Fcurated%2Fen%2F451111535402851523%2Fpdf%2FAUS0000426-REVISED-ALCS-Poverty-Chapter-upload-v2.pdf&usg=AOvVaw31wpFpS0_YnQABoAW6vDnc.
- Wulder, Michael A., Thomas R. Loveland, David P. Roy, Christopher J. Crawford, Jeffrey G. Masek, Curtis E. Woodcock, Richard G. Allen, et al. 2019. “Current Status of Landsat Program, Science, and Applications.” Remote Sensing of Environment 225: 127–147. https://doi.org/10.1016/j.rse.2019.02.015.
- Xu, Jinwen, Yi Qiang, Heng Cai, and Lei Zou. 2023. “Power Outage and Environmental Justice in Winter Storm Uri: An Analytical Workflow Based on Nighttime Light Remote Sensing.” International Journal of Digital Earth 16 (1): 2259–2278. https://doi.org/10.1080/17538947.2023.2224087.
- Yan, Shuai, Xiaochuang Yao, Dehai Zhu, Diyou Liu, Lin Zhang, Guojiang Yu, Bingbo Gao, Jianyu Yang, and Wenju Yun. 2021. “Large-Scale Crop Mapping from Multi-Source Optical Satellite Imageries Using Machine Learning with Discrete Grids.” International Journal of Applied Earth Observation and Geoinformation 103: 102485. https://doi.org/10.1016/j.jag.2021.102485.
- Yao, X., G. Li, J. Xia, J. Ben, Q. Cao, L. Zhao, Y. Ma, L. Zhang, and D. Zhu. 2020. “Enabling the Big Earth Observation Data via Cloud Computing and DGGS: Opportunities and Challenges.” Remote Sensing 12 (1): 62. https://doi.org/10.3390/rs12010062.