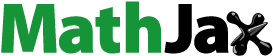
ABSTRACT
Desert locust outbreaks pose severe threats to agricultural production and ecological environments. Hence, understanding the distribution of habitat suitability is crucial for establishing effective prevention and control measures. We introduce an innovative method for analyzing the temporal and spatial variations of desert locust habitat suitability in Yemen. This method integrates ecological niche models with space–time cube analysis. First, we gathered key environmental variables that affect desert locust distribution, including vegetation and soil types, precipitation, and temperature. Subsequently, we employed the MaxEnt model to assess habitat suitability for desert locusts in Yemen for 2010 and 2013–2021. Finally, we applied the space–time cube method for spatiotemporal analysis to reveal the spatiotemporal dynamics of habitat suitability distribution. The MaxEnt model results showed that the kappa coefficients exceeded 0.46 and the area under the curve exceeded 0.75. The spatiotemporal analysis results showed that from 2010 to 2021, the Red Sea coastal plains in western Yemen exhibited the highest habitat suitability levels, with significant increases in habitat suitability in the western and central regions. This study offers a new perspective on studying the spatial and temporal characteristics of desert locust habitat suitability distribution and can provides a scientific basis for the management of desert locusts.
1. Introduction
The desert locust (Schistocerca gregaria) is widely distributed across Africa, parts of Western Asia, and Southern Asia; it is considered one of the most devastating migratory insect pests globally (Latchininsky Citation2013; Symmons and Cressman Citation2001). Under the background of global climate change, the range and severity of locust infestation are progressively escalating (Salih et al. Citation2020). Changes in environmental conditions, such as rainfall caused by tropical cyclones, can lead to the rapid reproduction of locusts and the formation of swarms, posing a considerable threat to local agriculture and animal husbandry production and seriously affecting food security, ecological security, and social stability (Anyamba et al. Citation2005; Pandey et al. Citation2021; Showler and Lecoq Citation2021; Wang et al. Citation2021). Therefore, conducting a large-scale, continuous space–time analysis of locust habitat suitability is crucial (Zhang et al. Citation2019).
Remote sensing technology, which enables the timely acquisition of large-scale Earth observation data, is widely used in studying the habitat suitability of desert locusts (Klein, Oppelt, and Kuenzer Citation2021; Latchininsky Citation2013). The rapid development of high spatiotemporal remote sensing provides a rich variety of extensive data resources for locust habitat monitoring. This condition considerably propels precise monitoring research on the complex surface environments of locust habitats and their spatiotemporal dynamic analysis (Klein et al. Citation2023).
Ecological niche models (ENMs) serve as a powerful tool for predicting the geographical distribution of species. They scientifically quantify the responses of species to environmental variables and forecast their potential distribution across geographic spaces (Elith and Leathwick Citation2009). Given their excellent ability to integrate interactions among environmental variables, the ENMs of collaborative remote sensing technology have been widely used in the study of locust habitat suitability (Rhodes and Sagan Citation2022). In their study of Moroccan locust (Dociostaurus maroccanus), Malakhov and Zlatanov (Citation2020) applied remote sensing data to construct an ecological ENM that included 74 environmental variables, such as soil, temperature, and vegetation. By using this method, they identified favorable conditions for the survival of locust egg pods. Kimathi et al. (Citation2020) utilized the MaxEnt model, which was originally developed by Phillips, Anderson, and Schapire (Citation2006; Citation2017), to analyze the relationship between globally known desert locust breeding sites and key bioclimatic and soil environmental parameters. Subsequently, they predicted potential breeding areas in East Africa.
However, the contingency of the annual distribution of locusts is typically disregarded in these methods, and a long time-series analysis of the interannual spatial and temporal changes of locust habitat suitability is lacking. The introduction of relevant spatiotemporal analyses will be beneficial (Veran et al. Citation2015). Therefore, to track the changes in habitat suitability of desert locusts in time and space, improve the monitoring of habitat suitability, and provide methodological support and data backing for locust prevention and control, we introduced the space–time cube method (Montero and Vilar Citation2014). We proposed a technique for analyzing the habitat suitability of desert locusts by combining MaXent with space–time cube. An ENM, such as MaXent, integrates the ecological niche conditions of desert locusts, aiding in more effectively predicting potential suitable areas. The space–time cube method allows tracking the trends of data changes over time and space. The combination of the two methods can help us analyze the trends of habitat suitability changes in deserts over a short period, identify key areas where habitat suitability is increasing, and generate habitat suitability mappings that consider spatiotemporal changes. This study provides a basis for scientifically valid monitoring and control measures against desert locusts to better cope with the challenges brought by these insect pests, making a positive contribution to the protection of ecological balance and agricultural production. It focuses on desert locusts in Yemen, with results validated through distribution datasets.
2. Materials and methods
2.1. Study area
Yemen () is a tropical country situated between semiarid and arid regions; it is characterized by a hot and dry climate (Al-Falahi et al. Citation2020). In recent years, the proportion of Yemeni population that relies on agrarian employment has been continually increasing, making agricultural production a primary economic source for locals (Alhawamdeh Citation2021; Bylund Citation2021). However, Yemen is perennially under the threat of desert locusts, largely affecting the agriculturally rich mountainous regions and coastal plains, which are the locations of major food production locales and densely populated areas (Alhawamdeh Citation2021).
Figure 1. Geographic location of Yemen and the distribution of desert locust occurrences in Yemen for the years 2010 and 2013–2021.

Yemen is also particularly susceptible to the effects of climate change, with agricultural production primarily reliant on rainfall brought about by the Red Sea Convergence Zone and the Intertropical Convergence Zone (Varisco Citation2019). However, increased precipitation implies a rise in the risk of desert locust outbreaks (Alhawamdeh Citation2021; Symmons and Cressman Citation2001). Simultaneously, ongoing societal unrest in Yemen poses major challenges to the effective control of desert locusts, further magnifying human-induced risk factors for locust plague outbreaks in the country (Showler and Lecoq Citation2021).
2.2. Data source
In general, the growth and occurrence of desert locusts are influenced by factors, such as climate, vegetation, and soil. Changes in climate exert a tremendous effect on desert locust behavior, with historical outbreaks of desert locust plague being related to climatic variations at that time, such as anomalies in temperature and precipitation (Cressman and Stefanski Citation2016; Meynard et al. Citation2017; Salih et al. Citation2020). Vegetation, as the primary food source and living space for desert locusts, plays a significant role in the spatiotemporal distribution of desert locust habitats. The state of vegetation distribution can heavily influence these factors (Cisse et al. Citation2013; Despland Citation2003; Despland and Simpson Citation2000; Gómez et al. Citation2018). In addition, female desert locusts prefer to choose warm and moist sandy soil for oviposition to reduce the difficulty of laying eggs while ensuring the required temperature and moisture for hatching (Gómez et al. Citation2018; Haque and Jaleel Citation1970; Hunter-Jones Citation1970). Therefore, on the basis of these principal factors, we selected the following seven factors as environmental variables: annual maximum normalized vegetative index (NDVI_max), annual average land surface temperature (LST), daily effective accumulated temperature (ET_daily), annual average soil moisture content (SM_mean), annual cumulative precipitation (PR), digital elevation model (DEM) data, and soil sand content (SSC).
The Google Earth Engine (GEE) is an integrated geographic information platform launched by Google. It combines data storage, analysis, and visualization. GEE offers a wealth of geospatial data and robust computational support, effectively streamlining the preprocessing of remote sensing images, enhancing analysis efficiency, and saving time and resources (Gorelick et al. Citation2017). We used GEE to obtain these environmental variables and aggregated them accordingly on an annual scale.
2.2.1. Distribution data
The distribution data of desert locusts used in this study originate from the Locust Hub of the Food and Agriculture Organization (FAO) of the United Nations. It offers free access to distribution datasets on desert locust hoppers, bands, adults, and swarms from 1985 through 2021. The Locust Hub is a pest monitoring and control platform established by FAO, with the purpose of supporting global locust plague prevention and response work. By integrating various technical means, analytical tools, and data resources, it provides decision-making support and technical guidance to experts and organizations involved in locust plague prevention and response.
2.2.2. Satellite data
The remote sensing data and products used in this study include MOD09GA and MOD11A1. MOD09GA is a land surface reflectance product with a spatial resolution of 500 m. It provides daily global surface albedo data. This data product is obtained from the Moderate Resolution Imaging Spectroradiometer (MODIS) sensor on the Terra satellite of the National Aeronautics and Space Administration (NASA). MOD11A1 is a surface temperature/emissivity data product based on MODIS with a spatial resolution of km. This product offers daily spectral radiance intensity and surface temperature data globally. The two products will be utilized to obtain NDVI_max and LST data for the study area in 2010 and from 2013 to 2021.
2.2.3. Geographic data and others
Similar to many undeveloped countries with water shortages, Yemen lacks sufficient long-term climate data records, and thus, these data can only be obtained using other means. Research conducted by Al-Falahi et al. (Citation2020) discovered that the Climate Hazards Group Infrared Precipitation with Stations (CHIRPS) dataset accurately reflects the spatial distribution of annual precipitation patterns in Yemen. The CHIRPS dataset is a global climate and precipitation dataset developed by the University of California, Santa Barbara’s Climate Hazards Center, alongside the United States Geological Survey and other partners (Funk et al. Citation2015). It is widely used in global climate change research, surface water change analysis, and natural disaster prediction and management. In the current study, we use the CHIRPS dataset to obtain annual cumulative precipitation information.
The soil moisture content data used were within the 7–28 cm () layer obtained from the land component of the fifth generation of European ReAnalysis (ERA5-Land) monthly dataset. Referring to the degree – hour calculation method by Tu et al. (Citation2014) and the temperature threshold cutoff method for the degree – day model by the University of California Integrated Pest Management (UC IMP Citationn.d.), we also used the hourly surface temperature (‘skin_temperature’ band) in the ERA5-Land dataset to calculate the daily effective accumulated temperature (ET_daily):
(1)
(1) where n is the total number of days in a year.
represents the desert locust effective accumulated temperature obtained via the DD model (Tu et al. Citation2014) on day i within a year, and it is expressed as
(2)
(2) where
is the surface temperature (°C) on day
at time
within that year. LT = 15.5°C represents the theoretical starting temperature required for desert locust egg development, and UT = 41°C denotes the highest threshold temperature for egg hatching. When temperature drops to below
or exceeds
, locust eggs cease developing (Huque and Jaleel Citation1970; Uvarov Citation1977).
The soil sand content (g/kg) used in this study is obtained from the SoilGrids250 m 2.0 dataset in the International Soil Reference and Information Center (Hengl et al. Citation2017). This dataset provides global soil information data with a spatial resolution of 250 m, including global soil texture, organic matter content, soil pH, moisture, potassium, phosphorus, and other related attribute information.
Elevation data come from the NASA digital elevation model (NASADEM) dataset with a spatial resolution of 30 m (NASA Jet Propulsion Laboratory Citation2020). To date, NASADEM is among the most advanced achievements in the field of global elevation models and is widely applied in watershed hydrology, geological research, ecosystem monitoring, and other domains.
We used the Large Scale International Boundary dataset (Geodata Citationn.d.), provided by the United States Office of Geography, as a source for international boundary data. This dataset provides high-precision international boundary data on a global scale, including information on boundaries from more than 250 countries, regions, and territories.
In addition, the MODIS annual land cover dataset MCD12Q1 (LC_Type1, Annual International Geosphere – Biosphere Program classification) with a spatial resolution of 500 m in 2010 and 2013–2021 was used in this study. The data are provided by NASA’s Earth Observation System Center and obtained through the supervised classification of MODIS Terra and Aqua reflectance data. The dataset is updated annually and has wide applications in environmental protection, natural disaster monitoring, agricultural management, urban planning, and global climate change research, among others.
2.3. Method
Through an exhaustive review of the literature, we identified the environmental variables that were necessary for studying desert locust habitat suitability and the corresponding geographic spatial datasets. These datasets were processed and normalized using ENMtools, a package in the R programming language (Warren et al. Citation2021). Subsequently, we used the MaxEnt model to explore the habitat suitability of desert locusts for each year and analyze their spatial distribution (Phillips et al. Citation2017). Finally, in conjunction with the space–time cube method, we performed a spatiotemporal integrated analysis of the temporal and spatial attributes of desert locust habitat suitability. illustrates the overall process of our method, including the interactive relationship between each dataset and model, and their application status at each step.
2.3.1. Preprocessing of distribution data
We extracted distribution data for Yemen from the years 2010 and 2013–2021 from the distribution datasets. Data from 2011–2012 were sparse, and thus, not considered. Distribution data retrieved from different periods within the same year may fall within the same environmental variable grid or be located at the same position, leading to data redundancy. Redundant data can interfere with the MaxEnt model results, and they also waste storage space and computing resources. To eliminate data redundancy, we used ENMtools to remove redundant data distributed on the same grid, saving the processed data as a CSV file.
2.3.2. Selection of environmental variables
The data input of ENM requires consistent spatial range and resolution, and thus, preprocessing is required. To enhance the accuracy of the results, removing habitats where desert locusts cannot possibly survive beforehand is essential. Using ArcGIS Pro v3.0.1, the SDMtoolbox (Brown, Bennett, and French Citation2017), and land classification data from MODIS MCD12Q1, we created a land use mask for data from different sources. Areas unsuitable for desert locust survival, such as permanent water bodies, forests, and urban construction lands, were masked out. To ensure consistent spatial resolution and scope among various environmental variables, we resampled the masked environmental variables to a uniform 1 km spatial resolution. Finally, by using the Yemen national borders obtained from LSIB, we separately cropped the environmental variables. In this manner, we could eliminate the land types that desert locusts are unlikely to inhabit, more accurately predicting the potential distribution areas of locusts.
When using ENM, paying attention to the issue of collinearity among environmental variables is also important. In the modeling process, highly collinear environmental variables can lead to unstable model estimates, increased difficulty in interpretation, and even affect the predictive ability of the model. To avoid strong collinearity among environmental variables, we used ENMtools to calculate the Pearson coefficients among the seven environmental variables. We only retained variables with lower correlations to other factors. In general, a Pearson coefficient value of 0.80 or above indicates a strong collinearity relationship between two variables (Kimathi et al. Citation2020). Therefore, variables with a Pearson coefficient of < 0.80 will serve as input for the model. Ultimately, we selected NDVI_max, SM_mean, PR, ET_daily, and SSC as input for the MaxEnt model.
2.3.3. Annual habitat suitability analysis
In the current study, the MaxEnt model constructed based on biomod2 (Thuiller et al. Citation2016) was used to obtain the annual distribution of desert locust habitat suitability. The biomod2 package is a package for ENM applications; it enables the treatment of a range of methodological uncertainties in models and the examination of species – environment relationships (Thuiller et al. Citation2016). The MaxEnt model was first proposed by ecologists Phillips, Anderson, and Schapire (Citation2006). The model is based on ecological niche theory, which states that species are restricted by environmental variables, employs machine learning technology, and models and predicts ecological niches by establishing probability density functions of species’ ecological niche distributions.
The MaxEnt model should not be treated as a black box and used only with default settings. This approach cannot guarantee optimal results, and thus, fine-tuning the necessary parameters is crucially important. In the MaxEnt model, the two most significant parameters are feature class (FC) and regularization multiplier (RM) (Morales, Fernández, and Baca-González Citation2017).
Accordingly, we used combinations of FC (L, LQ, H, LQH, and LQHPT) and RM (the value range is set from 0.5 to 5, with a step length of 0.5), resulting in 50 different models, with parameter tuning performed using ENMeval (Kass et al. Citation2021). R package’s ENMeval provides a flexible framework that allows users to choose diverse models and test different parameter combinations, automatically finding the optimal model and parameters. Notably, the MaxEnt model constructed using biomod2 is implemented by applying the Java version of MaxEnt (v3.4.3). Hence, to ensure consistency with the version of the MaxEnt model used in biomod2, we must use the same model to replace the default MaxEnt model used in ENMeval, which is located in the source files of the R package dismo (Hijmans et al. Citation2017). That is, MaxEnt in the dismo package was replaced.
To assess the performance of the models, we utilized the Akaike information criterion (AIC) as the evaluation criterion for the MaxEnt model (Sakamoto, Ishiguro, and Kitagawa Citation1986). AIC is a commonly used model selection criterion that considers the goodness of fit and complexity of models, helping users select the most appropriate one. By calculating the AIC for the 50 different parameter combinations of the MaxEnt models, we can identify the optimal model on the given dataset, enhancing the accuracy and performance of ecological niche modeling.
We can obtain the desert habitat suitability results for each year by using the selected environmental variables as input for the optimal models in each year. To ensure the reliability of the results, the model for each year was run 25 times to obtain 25 separate MaxEnt models. In addition, we used the area under the curve (AUC) of the receiver operator characteristic curves, the kappa coefficient, and true skill statistics (TSS) as validation metrics for the models. The kappa coefficient, TSS, and AUC are used to evaluate the performance of classifiers. However, the kappa coefficient and TSS measure the consistency and accuracy of classifier predictions with a value range of [−1, 1], while the AUC serves as a metric for assessing the discrimination ability of a classifier. This condition reflects the model’s ability to correctly classify positive and negative samples with a value range of [0, 1]. In accordance with historical experience (Allouche, Tsoar, and Kadmon Citation2006; Cohen Citation1960; Fielding and Bell Citation1997; Hanley and McNeil Citation1982; Monserud and Leemans Citation1992; Swets Citation1988), the three aforementioned evaluation indicators can be classified as shown in .
Table 1. Classification of evaluation indicator levels.
Furthermore, we utilized the previously generated 25 separate MaxEnt models. Using the model’s AUC value as a threshold, models with an AUC more significant than 0.7 were selected for further fusion to obtain the final ENM. With regard to the choice of a fusion strategy, we adopted a weighted average approach. The weights are determined using the following formula:
(3)
(3) where wj represents the weight of the jth model, AUCj is the AUC value of the jth model, and h is the number of models with AUC greater than 0.7. The final habitat suitability can be calculated as
(4)
(4) where yi is the habitat suitability value of the ith grid, wj is the weight of the jth model, and xij is the habitat suitability value of the jth model in the ith grid.
The purpose of this step was to enhance prediction accuracy and stability based on multiple high-quality models. The final fused ENM was used to acquire the annual suitability distribution results for desert locust habitats in Yemen.
2.3.4. Spatiotemporal variation analysis
We used the space–time cube method to further explore the spatiotemporal changes of desert locust suitability in Yemen. First, we constructed a space–time cube based on the results of the distribution of the suitability of desert locust habitats in Yemen in Section 2.3.3. A space–time cube refers to a virtual 3D data structure created using spatiotemporal data, such that effective time – space analysis and visualization can be performed (). The X and Y planes represent a 2D surface of an area, while the Z-axis represents time.
To track the changing trend of desert locust habitat suitability distribution in Yemen over time and space, we conducted an emerging hot spot analysis (ArcGIS Pro Citationn.d.-b; Montero and Vilar Citation2014). This method performs a cross-analysis of data from the spatial and temporal dimensions in the space–time cube, calculating the Getis – Ord (Gi*) statistic for each pixel in the dataset (Ahmadi et al. Citation2022). It allows us to obtain the distribution and trends of emerging hot spots, with categories that include new, persistent, intensifying, consecutive, diminishing, sporadic, oscillating, and historical hot or cold spots (ArcGIS Pro Citationn.d.-a) (). Through emerging hot spot analysis, we can capture hot spot areas within a region where the suitability of desert locusts in Yemen changes.
Table 2. Emerging spatiotemporal hot spot analysis patterns (ArcGIS Pro Citationn.d.-a).
In addition, we conducted a time series clustering analysis to further explore the spatiotemporal patterns of desert locust suitability in Yemen. The time clustering tool in ArcGIS Pro offers three clustering methods: similar values over a period, the same change trends, and similar repetition patterns. In the current study, we cluster based on similar values over a period. On the basis of the values from the clustering, we classified this feature class into levels, obtaining classifications for the suitability of desert locust habitats in Yemen.
3. Results
3.1. Importance of environmental variables
To explore which environmental variables contribute the most to the MaxEnt model, we determined the importance of environmental variables for each annual model (). The greatest contributor to the model over several years was PR, followed by ET_daily, while SSC made the least contribution. The two factors that contributed the most to the model in 2014 and 2016 were ET_daily and NDVI_max, while for 2019, the factors were PR and NDVI_max. Apart from these years, the two greatest contributing factors across all remaining years are PR and ET_daily. This result suggests that among the five key factors (i.e. temperature, vegetation, precipitation, soil moisture content, and soil sand content), precipitation and temperature are the two most critical factors that determine whether a region is suitable for desert locusts.
3.2. Suitability distribution analysis
The kappa coefficients, TSS, and AUC values for the models of each year are not entirely consistent. The kappa coefficient ranges from 0.465 to 0.792, TSS from 0.435 to 0.848, and AUC from 0.757 to 0.961 (). Referring to the rating levels in , the models that exhibited great performance based on the kappa coefficient and TSS were those from 2010, 2014, 2015, and 2017. Those that demonstrated good performance were from 2013, 2016, 2020, and 2021. Meanwhile, the models from 2018 and 2019 only presented fair performance. For the AUC metric, the models from 2010, 2014, and 2015 were rated excellent, while the models from all the other years were rated as good. Considering the overall results of the three evaluation metrics, except for the models from 2018 and 2019, the models from all the other years achieved good performance. In 2018–2019, given the unusual climate conditions and the lack of effective monitoring, a significant proliferation of desert locusts was reported in Yemen, causing widespread damage (Salih et al. Citation2020; Showler and Lecoq Citation2021). This situation resulted in an extraordinary amount of anomalous distribution data (), leading to poorer model performance compared with the other years. Although the models from 2018 and 2019 cannot be considered great, they achieved fair ratings for the kappa coefficient and TSS and a good rating for AUC.
Table 3. Best models and their major parameters for analyzing the suitability of desert locusts in Yemen in 2010 and 2013–2021.
The results of desert locust suitability distribution in Yemen () exhibit similarities and differences among different years. In particular, the distribution of suitability in 2010, 2014, and 2015 is similar, indicating that habitat suitability for desert locusts at the same location tends to be close in these years with a certain consistency. The suitability distributions of 2016, 2017, 2020, and 2021 also share some comparable characteristics. However, the patterns seen in 2013, 2018, and 2019 are clearly different from those in other years. This result corresponds to the geographical spread of locust infestations in those 3 years ((b, g, and h)). In all the years, areas along the Red Sea coastline in western Yemen exhibit the highest suitability for desert locust survival, implying that this region has comparatively stable suitable conditions across various years. By contrast, the plateau and inland desert areas in western Yemen have relatively lower suitability, possibly because the environmental conditions in these regions are less favorable to desert locust sustenance.
3.3. Spatiotemporal dynamic analysis
Desert locust populations and their distribution fluctuate significantly () under the influences of rainfall and high temperature (Cisse et al. Citation2013; Cressman and Stefanski Citation2016; Haque and Jaleel Citation1970; Latchininsky and Sivanpillai Citation2010; Roffey and Magor Citation2003). Among all the years mentioned, the distribution data were least abundant in 2010 and 2015 ((a and d)) and concentrated on the western plains of Yemen. Between 2018 and 2020 ((g–i)), a large-scale outbreak of desert locusts occurred, and their distribution spanned nearly the entire country of Yemen. By 2021 ((j)), desert locust population started to decline gradually, with distribution patterns resembling those of 2013 ((b)), 2014 ((c)), and 2016 ((e)). The differences in distribution between years indicate variability in habitat suitability for desert locusts in Yemen, as illustrated in .
To explore these spatiotemporal distribution differences, we conducted a spatiotemporal integrated analysis by using a space–time cube. We obtained the results shown in through an emerging hot spot analysis. This analysis shows that new hot spots are predominantly situated in the western San'ā Province, eastern Raimah, and western Al-Mahwit. The findings indicate an elevated habitat suitability in these provinces in recent years. Continuous, persistent, and intensifying hot spots are primarily observed along the Red Sea coast within the provinces of Hajjah, Al-Hudaydah, and Ta'izz, suggesting that these areas have historically exhibited high habitat suitability. In addition, the oscillating hot spots in Ma'rib, Al-Jawf, and the eastern part of the Red Sea coastal provinces indicate certain fluctuations in the habitat suitability of desert locusts in these regions. By contrast, northern Al-Jawf, eastern Hadramawt, northern Shabwah, and highland provinces, such as Ibb, are identified as key areas for consecutive and persistent cold spots, indicating that these locales consistently manifest low desert locust habitat suitability. We also noted that the southern areas of Hadramawt province and its neighboring provinces are key regions of diminishing cold spots, indicating a decreasing trend in habitat suitability for desert locusts in these locales. In the past decade, a significant increase in locust habitat suitability has been noted in the western and central regions of Yemen, particularly in Al-Hudaydah and Hajjah ().
By conducting a time-series clustering analysis on the space–time cube, we obtained the final classification of desert locust suitability levels in Yemen (). On the basis of cluster averages ((b)), we dissected suitability into five distinct levels.
Figure 9. (a) Time-series clustering results. (b) Average values of analytical variables at each time step for each cluster.

Very high suitability, regions that are exceedingly conducive to the survival and multiplication of generations of desert locusts.
High suitability, areas that are conducive to the survival and reproduction of generations of desert locusts.
Moderate suitability, zones where potential adequacy exists catering to the survival and reproduction of desert locust populations.
Low suitability, regions where the likelihood for an accommodative environment that supports desert locust generation is comparably low.
Unsuitable, locales that rae particularly inhospitable for the life cycle and propagation of any form of desert locust colonies.
The results reveal that the coastal regions of Yemen are primarily conducive to desert locusts. In particular, the Red Sea coasts of Hajjah, Al-Hudaydah, and Ta'izz Provinces, and the areas along the Gulf of Aden are identified as areas of very high or high suitability. The provinces of Ma'rib, Al-Jawf, and Hadramawt primarily constitute areas with moderate suitability. Low suitability regions are predominantly found in the eastern and northern parts of Al-Jawf, northern Hadramawt, and northern Shabwah. Unsuitable regions include the Western plateau, Southern plateau, inland desert zones, and a major part of Yemen’s east and southern Hadramawt, collectively accounting for the largest area among all the classifications.
4. Discussion
Traditionally, the analysis of locust populations and their habitats is largely based on technical means, such as ground surveys based on expert experience and biological models that integrate expert knowledge. These methods typically require high professional background and suffer from the problems of high cost and poor representativeness (Cressman Citation2013; Latchininsky and Sivanpillai Citation2010; Sword, Lecoq, and Simpson Citation2010). Therefore, the relatively economical high-resolution latest suitability map can help improve monitoring efficiency and focus on key breeding areas (Cressman Citation2013; Klein et al. Citation2023). ENM, as a commonly used habitat suitability analysis tool, has been proven to be effective in the management of locusts (Kimathi et al. Citation2020; Klein et al. Citation2022). However, conducting large-scale and long-term studies on dynamic changes is beneficial for formulating effective pest control and management strategies (Veran et al. Citation2015). Therefore, this study builds on the ENM model, incorporating spatiotemporal integrated analysis. Compared with conventional ENM model research, this method offers several advantages and innovations. We focus on the spatiotemporal analysis of suitability, employing emerging hot spot analysis and time-series clustering analysis to reveal the spatial distribution patterns and temporal trends of desert locust habitat suitability. By identifying persistent hot spot areas, monitoring and control resources can be deployed preferentially, while identifying cold spot areas helps exclude regions of relatively low risk. Time-series clustering analysis avoids the use of empirical numerical divisions to habitat suitability levels and considers long-term changes in habitat suitability, making the results more scientifically rigorous. Furthermore, the trends in habitat suitability revealed by this research are significant for predicting future habitat changes and formulating long-term management strategies.
This study found that the reliability of the MaxEnt model is more related to the distribution of desert locust data rather than quantity. Years with relatively concentrated data, such as 2010 and 2015, usually have higher reliability, while years with dispersed data distribution, such as 2018 and 2019, typically exhibit weaker reliability. However, the favorable validation indices of the multiyear MaxEnt model (kappa > 0.46, TSS > 0.43, AUC > 0.75) demonstrate that the constructed MaxEnt model possesses strong discriminative ability. The variable importance of the model indicates that the spatiotemporal variation in the suitability distribution of desert locust habitats is attributed to changes in climate, particularly variations in precipitation and temperature. This finding is consistent with the conclusions of existing research (Meynard et al. Citation2017; Salih et al. Citation2020). Climate warming may lead to a geographical shift in the temperature range preferred by desert locusts, while changes in precipitation patterns directly affect the moisture conditions of their breeding grounds (Cressman and Stefanski Citation2016; Haque and Jaleel Citation1970; Hunter-Jones Citation1970; Roffey and Magor Citation2003). Through emerging hot spot analysis, we obtained some interesting information that traditional ENM analysis methods do not provide. For example, suitability in Al-Hudaydah province has significantly increased over the past decade, indicating that it is becoming increasingly favorable for the survival of desert locusts. This finding may be related to the rise in temperature in Al-Hudaydah Province (Iraqi and Abdallah Citation2022). The results of the time-series clustering analysis indicate that our study can distinctly differentiate between suitable and unsuitable areas for desert locusts. Regions of medium to high suitability are primarily distributed along the coasts of the Red Sea, the Gulf of Aden, and the Arabian Sea, and inland areas, such as Al-Jawf, Marib, Shabwah, Hadramaut, and Al-Mahrah, which are the typical breeding areas in Yemen (Alhawamdeh Citation2021; Symmons and Cressman Citation2001). The above results suggest that our proposed research method can provide references for desert locust management under the background of global climate change, and thus, it has profound implications for protecting the food supply chain, maintaining ecological balance, and promoting sustainable development.
Although this study offers some unique insights, it also has certain limitations. The effectiveness of clustering results from the space–time cube is contingent upon the output from the MaxEnt model. Moreover, establishing a space–time cube necessitates at least 10 time steps, and thus, the methodology proposed in this work can only be applied to studies with sufficiently abundant time-series data. In addition, while building our annual habitat suitability models, we leaned exclusively on the MaxEnt model. Utilizing a single model may have some limitations. Supplementing it with other ENMs, such as generalized linear models, may potentially yield more scientifically robust and accurate predictive results. Regarding environmental variable selection, we did not consider the effect of human activities on desert locust habitats. Soil information was only limited to soil sand content and moisture, i.e. factors that influencing the seasonal migration of desert locusts, such as wind direction, were not considered. In our future research, we plan to use a combination of multiple ENMs and incorporate more variables, such as human activities, into our considerations.
5. Conclusion
Desert locusts are short-lived insects that exist in enormous numbers and pose severe threats to agriculture and the economy. To explore the potential habitat distribution of desert locusts in Yemen, we employed an ENM, namely, MaxEnt, and a space–time cube method to analyze their suitable areas and distribution changes. When using the MaxEnt model to predict suitable areas for desert locusts, we incorporated several environmental variables, such as vegetation, climate, and soil, along with the daily effective accumulated temperature calculated based on the degree day model for our study. Thereafter, we constructed a space–time cube of the suitability of desert locusts in Yemen. Through emerging hot spot analysis and time-series clustering, we analyzed changes in habitat suitability for desert locusts across temporal and spatial dimensions. Through these analyses, we produced a distribution map of Yemen desert locust suitability that fully considers spatiotemporal changes. The results suggest that the regions along the Red Sea coast represent the most conducive environments for the sustenance of desert locust populations in Yemen. These research findings can offer significant references for formulating management and control strategies for desert locusts.
Disclosure statement
No potential conflict of interest was reported by the author(s).
Data availability statement
The data that support the findings of this study are openly available in LSIB 2017 at https://geonode.state.gov/layers/catalog:geonode:LSIB, ERA5-Land Monthly Aggregated at https://doi.org/10.24381/cds.68d2bb30
, MOD09GA at https://doi.org/10.5067/MODIS/MOD09GA.061 , MCD12Q1 at https://doi.org/10.5067/MODIS/MCD12Q1.061 , CHIRPS at https://doi.org/10.1038/sdata.2015.66 , NASADEM at https://doi.org/10.5067/MEaSUREs/NASADEM/NASADEM_HGT.001 .Additional information
Funding
References
- Ahmadi, Hamed, Meysam Argany, Abolfazl Ghanbari, and Maryam Ahmadi. 2022. “Visualized Spatiotemporal Data Mining in Investigation of Urmia Lake Drought Effects on Increasing of PM10 in Tabriz Using Space-Time Cube (2004-2019).” Sustainable Cities and Society 76 (January): 103399. https://doi.org/10.1016/j.scs.2021.103399.
- Al-Falahi, Ali Hamoud, Naeem Saddique, Uwe Spank, Solomon H. Gebrechorkos, and Christian Bernhofer. 2020. “Evaluation the Performance of Several Gridded Precipitation Products Over the Highland Region of Yemen for Water Resources Management.” Remote Sensing 12 (18): 2984. https://doi.org/10.3390/rs12182984.
- Alhawamdeh, Ashraf (FAOYE). 2021. “Yemen Desert Locust Response Project: Pest and Pesticides Management Plan (PPMP).” 88.
- Allouche, Omri, Asaf Tsoar, and Ronen Kadmon. 2006. “Assessing the Accuracy of Species Distribution Models: Prevalence, Kappa and the True Skill Statistic (TSS).” Journal of Applied Ecology 43 (6): 1223–1232. https://doi.org/10.1111/j.1365-2664.2006.01214.x.
- Anyamba, Assaf, Jennifer Small, Compton Tucker, Keith Cressman, Timothy Love, and Kenneth J. Linthicum. 2005. “Remote Sensing of Eco-Climatic Conditions Associated with the 2004 Desert Locust Outbreak in Northwest and Sahelian Africa.” Pecora 16 Symp. Global Priorities in Land Remote Sensing.
- ArcGIS Pro. n.d.-a. “How Emerging Hot Spot Analysis Works—ArcGIS Pro | Documentation.” Accessed June 27, 2023. https://pro.arcgis.com/en/pro-app/latest/tool-reference/space-time-pattern-mining/learnmoreemerging.htm.
- ArcGIS Pro. n.d.-b. “How Time Series Clustering Works—ArcGIS Pro | Documentation.” Accessed June 27, 2023. https://pro.arcgis.com/en/pro-app/latest/tool-reference/space-time-pattern-mining/learnmoretimeseriesclustering.htm.
- Brown, Jason L., Joseph R. Bennett, and Connor M. French. 2017. “SDMtoolbox 2.0: The next Generation Python-Based GIS Toolkit for Landscape Genetic, Biogeographic and Species Distribution Model Analyses.” PeerJ 5 (December): e4095. https://doi.org/10.7717/peerj.4095.
- Bylund, Emma. 2021. “Dynamics of Net Primary Production and Food Availability in the Aftermath of the 2004 and 2007 Desert Locust Outbreaks in Niger and Yemen.” Master Thesis in Geographical Information Science 3.
- Cisse, Sory, Saïd Ghaout, Ahmed Mazih, Mohamed Abdallahi Ould Babah Ebbe, Ahmed Salem Benahi, and Cyril Piou. 2013. “Effect of Vegetation on Density Thresholds of Adult Desert Locust Gregarization from Survey Data in Mauritania.” Entomologia Experimentalis et Applicata 149 (2): 159–165. https://doi.org/10.1111/eea.12121.
- Cohen, Jacob. 1960. “A Coefficient of Agreement for Nominal Scales.” Educational and Psychological Measurement 20 (1): 37–46. https://doi.org/10.1177/001316446002000104.
- Cressman, Keith. 2013. “Role of Remote Sensing in Desert Locust Early Warning.” Journal of Applied Remote Sensing 7 (1): 075098. https://doi.org/10.1117/1.JRS.7.075098.
- Cressman, Keith, and Robert Stefanski. 2016. “Weather and Desert Locusts.” World Meteorological Organization and Food and Agriculture Organization of the United Nations: Rome, Italy.
- Despland, E. 2003. “Fractal Index Captures the Role of Vegetation Clumping in Locust Swarming.” Functional Ecology 17 (3): 315–322. https://doi.org/10.1046/j.1365-2435.2003.00728.x.
- Despland, E., and S. J. Simpson. 2000. “The Role of Food Distribution and Nutritional Quality in Behavioural Phase Change in the Desert Locustt.” Animal Behaviour 59 (3): 643–652. https://doi.org/10.1006/anbe.1999.1335.
- Elith, Jane, and John R. Leathwick. 2009. “Species Distribution Models: Ecological Explanation and Prediction Across Space and Time.” Annual Review of Ecology, Evolution, and Systematics 40 (1): 677–697. https://doi.org/10.1146/annurev.ecolsys.110308.120159.
- Fielding, Alan H., and John F. Bell. 1997. “A Review of Methods for the Assessment of Prediction Errors in Conservation Presence/Absence Models.”.
- Funk, Chris, Pete Peterson, Martin Landsfeld, Diego Pedreros, James Verdin, Shraddhanand Shukla, Gregory Husak, et al. 2015. “The Climate Hazards Infrared Precipitation with Stations—a New Environmental Record for Monitoring Extremes.” Scientific Data 2 (1): 150066. https://doi.org/10.1038/sdata.2015.66.
- Geodata. n.d. “Large Scale International Boundaries.” Geonode.State.Gov. Accessed May 20, 2023. https://geonode.state.gov/layers/catalog:geonode:LSIB.
- Gómez, Diego, Pablo Salvador, Julia Sanz, Carlos Casanova, Daniel Taratiel, and Jose Luis Casanova. 2018. “Machine Learning Approach to Locate Desert Locust Breeding Areas Based on ESA CCI Soil Moisture.” Journal of Applied Remote Sensing 12 (03): 1. https://doi.org/10.1117/1.JRS.12.036011.
- Gorelick, Noel, Matt Hancher, Mike Dixon, Simon Ilyushchenko, David Thau, and Rebecca Moore. 2017. “Google Earth Engine: Planetary-scale geospatial analysis for everyone.” Remote Sensing of Environment 202: 18–27. http://dx.doi.org/10.1016/j.rse.2017.06.031.
- Hanley, James A., and Barbara J. McNeil. 1982. “The Meaning and Use of the Area Under a Receiver Operating Characteristic (ROC) Curve.” Radiology 143 (1): 29–36. https://doi.org/10.1148/radiology.143.1.7063747.
- Haque, H., and M. A. Jaleel. 1970. Effect of Soil Salinityon the Development of Eggs of the Desert Locust (Schistocerca Gregaria Forsk.). FAO: Desert Locust Project Progress report.
- Hengl, Tomislav, Jorge Mendes de Jesus, Gerard B. M. Heuvelink, Maria Ruiperez Gonzalez, Milan Kilibarda, Aleksandar Blagotić, Wei Shangguan, et al. 2017. “SoilGrids250m: Global Gridded Soil Information Based on Machine Learning.” PLoS One 12 (2): e0169748. https://doi.org/10.1371/journal.pone.0169748.
- Hijmans, Robert J., Steven Phillips, John Leathwick, Jane Elith, Maintainer Robert, and J. Hijmans. 2017. “Package ‘Dismo’.” Circles 9 (1): 1–68.
- Hunter-Jones, Philip. 1970. “The Effect of Constant Temperature on Egg Development in the Desert Locust Schistocerca Gregaria (Forsk.).” Bulletin of Entomological Research 59 (4): 707–718. https://doi.org/10.1017/S0007485300003722.
- Huque, Heshamul, and M. A. Jaleel. 1970. “Temperature-Induced Quiescence in the Eggs of the Desert Locust1.” Journal of Economic Entomology 63 (5): 1398–1400. https://doi.org/10.1093/jee/63.5.1398.
- Iraqi, Abkar A., and Abdallah M. Abdallah. 2022. “Analysis of Long-Term Climatic Changes at Al-Hodeidah-Yemen During the Period Between 1985 and 2019.” Theoretical and Applied Climatology 150 (3–4): 1067–1081. https://doi.org/10.1007/s00704-022-04178-2.
- Kass, Jamie M., Robert Muscarella, Peter J. Galante, Corentin L. Bohl, Gonzalo E. Pinilla-Buitrago, Robert A. Boria, Mariano Soley-Guardia, and Robert P. Anderson. 2021. “ENMeval 2.0: Redesigned for Customizable and Reproducible Modeling of Species’ Niches and Distributions.” Methods in Ecology and Evolution 12 (9): 1602–1608. https://doi.org/10.1111/2041-210X.13628.
- Kimathi, Emily, Henri E. Z. Tonnang, Sevgan Subramanian, Keith Cressman, Elfatih M. Abdel-Rahman, Mehari Tesfayohannes, Saliou Niassy, et al. 2020. “Prediction of Breeding Regions for the Desert Locust Schistocerca Gregaria in East Africa.” Scientific Reports 10 (1): 11937. https://doi.org/10.1038/s41598-020-68895-2.
- Klein, Igor, Natascha Oppelt, and Claudia Kuenzer. 2021. “Application of Remote Sensing Data for Locust Research and Management—A Review.” Insects 12 (3): 233. https://doi.org/10.3390/insects12030233.
- Klein, Igor, Soner Uereyen, Christina Eisfelder, Vladimir Pankov, Natascha Oppelt, and Claudia Kuenzer. 2023. “Application of Geospatial and Remote Sensing Data to Support Locust Management.” International Journal of Applied Earth Observation and Geoinformation 117 (March): 103212. https://doi.org/10.1016/j.jag.2023.103212.
- Klein, Igor, Sietse van der Woude, Frederic Schwarzenbacher, Nadiya Muratova, Bart Slagter, Dmitry Malakhov, Natascha Oppelt, and Claudia Kuenzer. 2022. “Predicting Suitable Breeding Areas for Different Locust Species – A Multi-Scale Approach Accounting for Environmental Conditions and Current Land Cover Situation.” International Journal of Applied Earth Observation and Geoinformation 107 (March): 102672. https://doi.org/10.1016/j.jag.2021.102672.
- Latchininsky, Alexandre V. 2013. “Locusts and Remote Sensing: A Review.” Journal of Applied Remote Sensing 7 (1): 075099. https://doi.org/10.1117/1.JRS.7.075099.
- Latchininsky, Alexandre V., and Ramesh Sivanpillai. 2010. “Locust Habitat Monitoring and Risk Assessment Using Remote Sensing and GIS Technologies.” In Integrated Management of Arthropod Pests and Insect Borne Diseases, edited by Aurelio Ciancio, and K. G. Mukerji, 163–188. Dordrecht: Springer Netherlands. https://doi.org/10.1007/978-90-481-8606-8_7.
- Malakhov, Dmitry V., and Boris V. Zlatanov. 2020. “An Ecological Niche Model for Dociostaurus Maroсcanus, Thunberg, 1815 (Orthoptera, Acrididae): The Nesting Environment and Survival of Egg-Pods.” Biosis:Biological Systems 1 (1): 08–24. https://doi.org/10.37819/biosis.001.01.0048.
- Meynard, Christine N., Pierre-Emmanuel Gay, Michel Lecoq, Antoine Foucart, Cyril Piou, and Marie-Pierre Chapuis. 2017. “Climate-Driven Geographic Distribution of the Desert Locust During Recession Periods: Subspecies’ Niche Differentiation and Relative Risks Under Scenarios of Climate Change.” Global Change Biology 23 (11): 4739–4749. https://doi.org/10.1111/gcb.13739.
- Monserud, Robert A., and Rik Leemans. 1992. “Comparing Global Vegetation Maps with the Kappa Statistic.” Ecological Modelling 62 (4): 275–293. https://doi.org/10.1016/0304-3800(92)90003-W.
- Montero, Pablo, and José A. Vilar. 2014. “TSclust: An R Package for Time Series Clustering.” Journal of Statistical Software 62 (1): 1–43. https://doi.org/10.18637/jss.v062.i01.
- Morales, Narkis S., Ignacio C. Fernández, and Victoria Baca-González. 2017. “MaxEnt’s Parameter Configuration and Small Samples: Are We Paying Attention to Recommendations? A Systematic Review.” PeerJ 5 (March): e3093. https://doi.org/10.7717/peerj.3093.
- NASA JPL. 2020. “NASADEM Merged DEM Global 1 Arc Second V001.” (data set). doi:10.5067/MEASURES/NASADEM/NASADEM_HGT.001.
- Pandey, Meena, Binita Suwal, Preeti Kayastha, Gresha Suwal, and Dipak Khanal. 2021. “Desert Locust Invasion in Nepal and Possible Management Strategies: A Review.” Journal of Agriculture and Food Research 5 (September): 100166. https://doi.org/10.1016/j.jafr.2021.100166.
- Phillips, Steven J., Robert P. Anderson, Miroslav Dudík, Robert E. Schapire, and Mary E. Blair. 2017. “Opening the Black Box: An Open-Source Release of Maxent.” Ecography 40 (7): 887–893. https://doi.org/10.1111/ecog.03049.
- Phillips, Steven J., Robert P. Anderson, and Robert E. Schapire. 2006. “Maximum Entropy Modeling of Species Geographic Distributions.” Ecological Modelling 190 (3–4): 231–259. https://doi.org/10.1016/j.ecolmodel.2005.03.026.
- Rhodes, Kristen, and Vasit Sagan. 2022. “Integrating Remote Sensing and Machine Learning for Regional-Scale Habitat Mapping: Advances and Future Challenges for Desert Locust Monitoring.” IEEE Geoscience and Remote Sensing Magazine 10 (1): 289–319. https://doi.org/10.1109/MGRS.2021.3097280.
- Roffey, J., and J. I. Magor. 2003. “Desert Locust Technical Series—Desert Locust Popualation Dynamics Parameters.” Desert Locust Field Reserch Stations (FAO): Rome, Italy, 35.
- Sakamoto, Yosiyuki, Makio Ishiguro, and Genshiro Kitagawa. 1986. “Akaike Information Criterion Statistics.” Dordrecht, The Netherlands: D. Reidel 81 (10.5555): 26853.
- Salih, Abubakr A. M., Marta Baraibar, Kenneth Kemucie Mwangi, and Guleid Artan. 2020. “Climate Change and Locust Outbreak in East Africa.” Nature Climate Change 10 (7): 584–585. https://doi.org/10.1038/s41558-020-0835-8.
- Showler, Allan T., and Michel Lecoq. 2021. “Incidence and Ramifications of Armed Conflict in Countries with Major Desert Locust Breeding Areas.” Agronomy 11 (1): 114. https://doi.org/10.3390/agronomy11010114.
- Swets, John A. 1988. “Measuring the Accuracy of Diagnostic Systems.” Science, New Series 240 (4857): 1285–1293.
- Sword, Gregory A., Michel Lecoq, and Stephen J. Simpson. 2010. “Phase Polyphenism and Preventative Locust Management.” Journal of Insect Physiology 56 (8): 949–957. https://doi.org/10.1016/j.jinsphys.2010.05.005.
- Symmons, P. M., and K. Cressman. 2001. “Desert Locust Guidelines: Biology and Behaviour.” FAO, Rome, 1–42.
- Thuiller, Wilfried, Damien Georges, Robin Engler, Frank Breiner, Maintainer Damien Georges, and Contact Wilfried Thuiller. 2016. “Package ‘Biomod2.’” Species Distribution Modeling within an Ensemble Forecasting Framework.
- Tu, Xiongbing, Zhihong Li, Jie Wang, Xunbing Huang, Jiwen Yang, Chunbin Fan, Huihui Wu, Qinglei Wang, and Zehua Zhang. 2014. “Improving the Degree-Day Model for Forecasting Locusta Migratoria Manilensis (Meyen) (Orthoptera: Acridoidea).” PLoS One 9 (3): e89523. https://doi.org/10.1371/journal.pone.0089523.
- UC IPM. n.d. “Degree-Days: About Degree-Days.” Accessed January 9, 2024. https://ipm.ucanr.edu/WEATHER/ddconcepts.html#Cutoff.
- Uvarov, Boris. 1977. Grasshoppers and Locusts. A Handbook of General Acridology Vol. 2. Behaviour, Ecology, Biogeography, Population Dynamics. London: Centre for Overseas Pest Research.
- Varisco, Daniel. 2019. “Pumping Yemen Dry: A History of Yemen’s Water Crisis.” Human Ecology 47 (3): 317–329. https://doi.org/10.1007/s10745-019-0070-y.
- Veran, Sophie, Stephen J. Simpson, Gregory A. Sword, Edward Deveson, Sylvain Piry, James E. Hines, and Karine Berthier. 2015. “Modeling Spatiotemporal Dynamics of Outbreaking Species: Influence of Environment and Migration in a Locust.” Ecology 96 (3): 737–748. https://doi.org/10.1890/14-0183.1.
- Wang, Lei, Wen Zhuo, Zhifang Pei, Xingyuan Tong, Wei Han, and Shibo Fang. 2021. “Using Long-Term Earth Observation Data to Reveal the Factors Contributing to the Early 2020 Desert Locust Upsurge and the Resulting Vegetation Loss.” Remote Sensing 13 (4): 680. https://doi.org/10.3390/rs13040680.
- Warren, Dan L., Nicholas J. Matzke, Marcel Cardillo, John B. Baumgartner, Linda J. Beaumont, Michael Turelli, Richard E. Glor, et al. 2021. “ENMTools 1.0: An R Package for Comparative Ecological Biogeography.” Ecography 44 (4): 504–511. https://doi.org/10.1111/ecog.05485.
- Zhang, Long, Michel Lecoq, Alexandre Latchininsky, and David Hunter. 2019. “Locust and Grasshopper Management.” Annual Review of Entomology 64 (1): 15–34. https://doi.org/10.1146/annurev-ento-011118-112500.