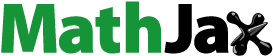
ABSTRACT
It is crucial to mangrove management by understanding its conservation efforts. This study proposed a spatiotemporal analysis model to evaluate mangrove changes in the Guangxi Beibu Gulf (GBG) and the Guangdong-Hong Kong-Macao Greater Bay Area (GBA), focusing on past, present, and future dynamics. The analysis covered mangrove loss, stability, and gain from 1990 to 2020 and provided projections for 2020 to 2035 across multiple scenarios. Then further assessed the efficacy of past mangrove conservation with the Sustainable Development Goals (SDGs) and the prospective conservation potential with the Global Mangrove Alliance Goal (GMAG) indicators. Findings indicate that by 2020, the GBG and GBA had 81.65 km² and 40.92 km² of mangroves, respectively, with increases of 31.51% and 164.09% since 1990. Projected expansions are anticipated under all three scenarios during 2020- 2035, with the highest growth projected under the trend continuation scenario, followed by ecological restoration and protection, and economic development. While mangrove protection in these regions met SDG targets 6.6.1 and 14.5.1 from 2015 to 2020, the 80% conservation milestone set by GMAG for 2030 was not achieved, necessitating the establishment of nature reserves. The study offers novel insights for sustainable governance of mangrove forests at local, regional, and global levels.
1. Introduction
Mangrove forests are typically defined as a collection of woody trees, shrubs and ferns dominated by mangrove trees which grow in tropical and subtropical intertidal zones (Duke Citation2017; Tomlinson Citation2016). Mangrove forests play vital roles in purifying seawater, sheltering land from wind and waves, sequestering and storing carbon, and conserving biodiversity, thus improving the well-being of humankind (Duke et al. Citation2014; Marois and Mitsch Citation2015; Menéndez et al. Citation2018). Due to human activities (e.g. foreshore and seabed reclamation, mudflat aquaculture, urbanization) and natural factors (e.g. climate change, windstorms), mangrove forests decreased by 3,363 km2 from 2000 to 2016 (Goldberg et al. Citation2020). According to the Global Mangrove Alliance (Citation2022), mangrove forests decreased by 5,245 km2 from 1996 to 2020 globally (Bunting et al. Citation2022; Global Mangrove Alliance Citation2022). Therefore, it is crucial to explore the past, present, and future changes in mangrove forests to better manage and protect mangrove resources.
Remote sensing, characterized by wide coverage, fast data updating, and competitively low costs, has been widely adopted for monitoring spatiotemporal changes in mangrove forests (Wang et al. Citation2019). Productive outcomes have been achieved with the use of this tool. For instance, Lorenzo, De Jesus Jr, and Jara (Citation1979), for the first time, monitored the degeneration of mangrove forest wetlands in Zamboanga city in the Philippines by analyzing Landsat MSS data. In recent years, spatiotemporal change analyses of mangroves have been conducted at different spatial scales, including large scales such as South Asia (Giri et al. Citation2015), China (Jia Citation2014), Australia (Lymburner et al. Citation2020), the United Arab Emirates (Elmahdy et al. Citation2020), as well as smaller scales (Feng et al. Citation2020; Jia, Chen, and Jiang Citation2022; Liu et al. Citation2019; Wang et al. Citation2020). However, these studies have focused mainly on spatiotemporal changes from past to present. Regarding future predictions, Sarker et al. (Citation2019) constructed a mangrove biodiversity map of the Bangladesh Sundarbans using a covariate-driven habitat model, while Pimple et al. (Citation2023) explored the main limitations and potential of remote sensing in predicting mangrove diversity. However, these studies mainly conducted the prediction under one future scenario. Recently, as an emerging new trend, multi-scenarios prediction is found to be particularly important (Qin et al. Citation2019; Veerkamp et al. Citation2020), as it can provide multiple quantitative and visual results and help decision-makers formulate more reasonable plans (Gomes et al. Citation2021). Future multi-scenario predictions on land cover (Nie et al. Citation2023), urban construction land (Li et al. Citation2022a), and wetlands (Peng et al. Citation2023) have been carried out with fruitful results. Therefore, spatial prediction models and future multi-scenario patterns can be further adopted for research on future spatiotemporal changes in mangrove forests, thus providing detailed spatial information for future recovery and protection.
In 2015, the United Nations proposed the Sustainable Development Goals (SDGs) to achieve a better and more sustainable world. Among the SDGs, SDG 6.6 and SDG 14.5 guide mangrove forest protection and the evaluation of its effectiveness (United Nations Citation2015). Current studies have shown that SDG 6.6, ‘By 2020, protect and restore water-related ecosystems, including mountains, forests, wetlands, rivers, aquifers and lakes’, and SDG 14.5, ‘By 2020, conserve at least 10% of coastal and marine areas, consistent with national and international laws and based on the best available scientific information’ (United Nations Citation2015), are closely connected with the conservation, recovery, and governance of mangrove forests (Friess et al. Citation2019; Jia et al. Citation2021; Jia et al. Citation2023). However, these two goals were set for 2020, failing to guide the future protection of mangrove forests. The Global Mangrove Alliance is a joint effort of 27 organizations, including Conservation International (CI) and The International Union for the Conservation of Nature (IUCN), that works to accelerate the revival of mangrove forests across the globe. To ensure the long-term security of mangrove forest ecosystems, the Global Mangrove Alliance released The State of the World’s Mangroves 2022 in September 2022 (Global Mangrove Alliance Citation2022) and proposed the Global Mangrove Alliance Goal for 2030 (GMAG). The GMAG attaches great importance to the conservation and recovery of mangrove forests. Hence, mangrove forests in the past and present can be quantitatively compared by using remote sensing (Jia et al. Citation2023; Wang et al. Citation2019) for further evaluating the SDGs. While for Global Mangrove Alliance Goal, it can also be evaluated by future mangrove forests simulated by spatial prediction models. A comprehensive evaluation of the SDGs and GMAG demonstrates the outcomes of previous conservation and recovery, as well as quantitative goals for future governance.
The Guangxi Beibu Gulf and Guangdong–Hong Kong–Macao Greater Bay Area, were in Guangxi and Guangdong, two provincial-level regions of China, with the largest and richest mangrove forests. According to the recent research, 95% of China's mangrove forests grow in Guangdong, Guangxi, and Hainan provinces (Jia et al. Citation2021), where Guangdong and Guangxi are the top provinces with the largest cover of mangroves (Wei et al. Citation2021). However, the gap in social and economic development between these two urban agglomerations is huge. As a developed urban agglomeration, the Guangdong-Hong Kong-Macao Greater Bay Area has a gross domestic product (GDP), population, and per capita GDP of 13 trillion yuan, 86.347 million, and 150.6 thousand yuan, respectively. In contrast, the emerging urban agglomeration of Guangxi Beibu Gulf has the GDP, population, and per capita GDP as 1.3 trillion yuan, 22.8574 million, and 56.9 thousand yuan, respectively. The big gap in between has fully justified the representativeness and importance to choose these two urban agglomerations as our study areas.
Therefore, the objectives of this study are as follows:
To propose an analytical model based on the full process chain (past–present–future) of spatiotemporal changes in mangrove forests. Using the Guangxi Beibu Gulf and Guangdong–Hong Kong–Macao Greater Bay Area as study areas, we conducted a spatiotemporal change analysis of mangrove forests from 1990 to 2020 and from 2025 to 2035 under multiple future scenarios.
To evaluate the effectiveness and future potential of mangrove forest conservation and recovery according to both the SDGs and the GMAG.
2. Materials and methods
This study uses remote sensing classification data and model simulation data to analyze the spatiotemporal changes in mangrove degradation, restoration, and stability from 1990 to 2020 and from 2020 to 2035. Based on the SDGs, the past effectiveness of mangrove protection and restoration was evaluated, and the future potential was evaluated based on the GMAG ().
2.1. Study area
The Guangxi Beibu Gulf and Guangdong-Hong Kong-Macao Greater Bay Area are two coastal urban agglomerations in southern China. As shown in , Guangxi Beibu Gulf is located between 20°54'8"–24°2'7"N and 106°33'26"–110°53'42"E, with an area of 72,319.84 km2 and a coastline of 1595 km. Guangdong-Hong Kong-Macao Greater Bay Area is located at 21°34'2"–24°23'30"N and 111°21'24"–115°25'18"E, with an area of 55,459.92 km2 and a coastline of 2377.43 km. The two areas share the similar climate and resource due to their similar geo-location, such as high temperature, abundant heat, abundant rainfall, first-class ecological quality in the country, rich in ports, coastline, oil and gas, agriculture and forestry, as well as abundant mangrove forest resources, including 18 families, 24 genera, and 27 species. Approximately 73% of the mangrove forest species in China are observed here.
Since the two urban agglomerations are in the transition zone between terrestrial and marine ecosystems, they are sensitive to climate change with a fragile ecological environment (He et al. Citation2016). During the past decades, these two regions both experienced rapid economic development (Li et al. Citation2022b). During 1990 to 2020, the construction land area has increased from 1520 km2 to 8962 km2 in the Guangdong-Hong Kong-Macao Greater Bay Area, while from 650 km2 to 2010 km2 in the Guangxi Beibu Gulf (Peng Citation2022). The rapid urbanization has led to a trend of landscape fragmentation, influencing their wetland and mangrove patterns (Guo et al. Citation2021; Zhang et al. Citation2023). As a result, the latest urbanization development projects and actions of the two areas for urban agglomerations can be found to be no longer dominated by a single socio-economic development, but gradually carrying out the construction of green space, livable space, and smart space, as can been seen in Guangxi New Urbanization Plan (2021–2035) and Guangdong New Urbanization Plan (2021–2035). As a featured green space of the two areas, it is essential to analyze the spatiotemporal change of the mangrove forests.
2.2. Data sources and processing
2.2.1. Remote sensing data and simulated data of mangrove forests
The mangrove forest data from 1990–2035 adopted in this study were contributed by Peng (Peng Citation2022; Peng et al. Citation2024). In Peng’s study, the initial mangrove sample points were generated by Global Mangrove Watch (https://data.unep-wcmc.org/datasets/45), and the Global Mangrove Watch data from 1996 to 2016 with 7 periods was spatially overlaid to generate random mangrove points in the common intersection area. Based on Landsat time series data, pixel-oriented random forest classification and object-oriented hierarchical decision tree classification were combined to classify wetlands. First, using the random forest algorithm and phenology-based features, such as median, wettest, and greenest images with spectral bands, the pixel based supervised classification was carried out to extract 6 major categories of the wetlands. Secondly, based on geometric features and auxiliary data sets, combined with the chessboard segmentation method (Zheng et al. Citation2016), a hierarchical decision tree of knowledge rules is constructed to further subdivide the large category of wetlands into mangrove forests and other 9 types of fine wetlands. This enabled us to precisely extract mangrove forests from 1990 to 2020, with an overall precision of 0.92±0.01. A spatial consistency comparison was conducted by calculating the areas of the spatial consistency between the mangrove forests datasets extracted by Peng et al. in 2020 and the Ministry of Natural Resources in 2018 (http://www.sasclouds.com/chinese/). Based on ZY-3 and GF-1 Satellites with a spatial resolution of 2 meters, the mangrove forests data from the Ministry of Natural Resources is produced by combining automatic classification, manual correction, and field verification, where the overall accuracy achieved is 98%. The results showed that the spatial consistency of the two areas was 90.8 km2, with a consistency ratio of 74.1%. From 1990 to 2020, the correlation coefficient (R2) value was between 0.674 and 0.834, and the root mean square error (RMSE) value was between 0.007 and 0.012.
Future mangrove data were modeled through spatial prediction models and simulated with future scenario patterns (Peng Citation2022; Peng et al. Citation2023). First, a spatial prediction model for fine wetland types was constructed by coupling random forest regression and the CLUE-S model, and the accuracy of the model was evaluated using real remote sensing classification data from 2015 and 2020. The model has good rationality and accuracy (). Second, policy planning and socioeconomic development were considered to construct future urban agglomeration development, and the area demand for wetlands and nonwetlands under future scenarios were calculated. Finally, using the area demand of the scenario mode as a constraint, a spatial prediction model was used to simulate the spatial distribution of mangroves and other land use types from 2025 to 2035. There were three future development scenarios: the trend continuation scenario (TCS), the ecological restoration and protection scenario (ERPS), and the economic development scenario (EDS). TCS was achieved using a Markov chain. As the TCS considers the proximity of time, it was used to deduce the land use area required for 2025–2035 based on the land use change of mangrove forests and other land use types. In the ERPS, the study area emphasizes the conservation of the ecological environment and resources. The future structural design of land use areas aims to maximize the value of ecosystem services and ecological benefits. The EDS, in contrast, seeks to maximize economic development. Future structures are designed to serve socioeconomic development instead, targeting the maximization of economic benefits.
Table 1. Accuracy evaluation of the simulation results.
2.2.2. Data for mangrove forest reserves
The data adopted in this study were from national and provincial reserves, Ramsar sites, national wetland parks, national marine parks, and representative international wetlands, as shown in . Resources include the National Forestry and Grassland Administration of China (http://www.forestry.gov.cn/), the Forestry Bureau of Guangxi Zhuang Autonomous Region (http://lyj.gxzf.gov.cn), the Forestry Bureau of Guangdong Province (http://lyj.gd.gov.cn/), official websites of Ramsar site protection (https://www.ramsar.org/), and relevant documents released by the government. These data were used for calculating the protection rate of mangroves.
2.3. Methods
2.3.1. Type of spatial variation
To reveal the spatial variation in mangrove forests and non-mangrove forests, this study constructed diagrams for 1990–2000, 2000–2010, 2010–2020, and three scenarios from 2020–2035 to show the spatial variation in mangrove forests and further identified areas that were stable, gain, and loss, respectively. Implementation Steps: First, spatial mangrove data for the years 1990, 2000, 2010, 2020, and 2035 were overlaid using ArcMap software. Subsequently, the Raster Calculator was utilized to perform code conversion calculations for ‘stable’, ‘gain’, and ‘loss’. The types of spatial variation were defined as follows. LUm refers to the location of a given pixel. If the pixel does not move, it is regarded as ‘stable’; if other types of land transfer to mangrove forests, then it is called ‘gain’; if mangrove forests change to other land types, then it is called ‘loss’.
(1)
(1)
(2)
(2)
(3)
(3) where LUm and LUi represent mangrove forest and other types of land use, respectively.
2.3.2. Methods for SDGs evaluation
According to SDG 6.6 ‘By 2020, protect and restore water-related ecosystems, including mountains, forests, wetlands, rivers, aquifers and lakes’ and SDG 6.6.1 ‘Change in the extent of water-related ecosystems over time’, this study set 2015 (SDGs released year) and 2020 as the base year and target year, respectively, and examined the variation in the area of mangrove forest wetlands during this period.
(4)
(4) where
represents SDG 6.6.1 and
and
represent the areas of mangrove forest wetlands in 2015 and 2020, respectively.
Based on SDG 14.5 ‘By 2020, conserve at least 10 percent of coastal and marine areas, consistent with national and international laws and based on the best available scientific information’ and SDG 14.5.1 ‘Coverage of protected areas in relation to marine areas,’ this study calculated the percentage of mangrove forests protected in 2020 in the study area, i.e. the ratio of mangrove forests in protected areas to the total area of mangrove forests.
(5)
(5) where
represents the indicator for SDG 614.5.1 and
and
represent the total area of mangrove forest wetlands and the area of mangrove forest wetlands in protected areas in 2000, respectively.
2.3.3. Methods for Global Mangrove Alliance Goal evaluation
The Global Mangrove Alliance Goal set several targets: ‘By 2030: halting loss, restoring losses, and doubling protection. Doubling protection refers to the area of mangrove forests that are managed in protected areas or that have equivalent levels of protection, increasing from 40% to 80%.’ This study calculated the percentage of mangrove forests protected in 2030 in the study area, that is, the ratio of mangrove forests in protected areas to the total area of mangrove forests.
(6)
(6) where
represents the Global Mangrove Alliance Goal indicator and
and
represent the total area of mangrove forest wetlands and the area of mangrove forests in protected areas in 2030, respectively.
3. Results
3.1. Distribution of mangrove forests in 2020
The areas of mangrove forests in the Guangxi Beibu Gulf and Guangdong–Hong Kong–Macao Greater Bay Area were 81.65 and 40.92 km2, respectively; that is, the area of mangrove forests in the Guangxi Beibu Gulf was approximately twice that of the Guangdong–Hong Kong–Macao Greater Bay Area.
Mangrove forests were observed in most areas along the coastline of the Guangxi Beibu Gulf (). The regions with large mangrove forest areas included Pearl Bay (10.01 km2), the Maowei Sea (4.97 km2), the Dafeng River (10.45 km2), Lianzhou Bay (10.32 km2), Tieshan Harbor (8.65 km2), and the Dandou Sea (5.78 km2). The mangrove forests in these regions accounted for 61% of the total area of mangrove forests in the Guangxi Beibu Gulf. Other mangrove forests were sparsely distributed in the Beilun River estuary, Dongxi Bay, Jingu River, Xicun Harbor, and Yingluo habitat.
Figure 3. Distribution of mangrove forests in the Guangxi Beibu Gulf and Guangdong–Hong Kong–Macao Greater Bay Area in 2020.

The mangrove forests in the Guangdong–Hong Kong–Macao Greater Bay Area were relatively concentrated (), occurring mainly in Zhenhai Bay (12.05 km2, 29%), Qi’ao Island (5.00 km2, 12%), and Shenzhen Bay (8.59 km2, 21%), which represented 63% of the total area. Other mangrove forests were distributed sporadically in Yinhu Bay, the Pearl River Estuary, Dapeng Bay, and Xunliao Bay.
3.2. Spatiotemporal changes in mangrove forests
3.2.1. Temporal changes in mangrove forests
This study calculated the variations in the areas of mangrove forests in the two coastal urban agglomerations during 1990–2020 and 2020–2035 (). The Guangxi Beibu Gulf experienced an overall increasing trend in the area of mangrove forests from 62.08 km2 in 1990 to 81.65 km2 in 2020, for an increase of 19.56 km2 (31.51%). It experienced only a small reduction during 1995–2000. From 2020–2035, the Guangxi Beibu Gulf showed increasing trends in the area of mangrove forests under all three future scenarios, with it increasing the most under the TCS, followed by the ERPS and EDS. By 2035, the areas of mangrove forest under the TCS, ERPS, and EDS will be 122.26, 110.69, and 99.50 km2, respectively, and their increase rates are 50%, 36%, and 22%, respectively, compared to those in 2020. The Guangdong–Hong Kong–Macao Greater Bay Area also exhibited an overall increase in the area of mangrove forests during the first 30 years, with only a slight decrease from 1995–2005. The area of mangrove forests increased from 15.49 km2 in 1990 to 40.92 km2 in 2020, and the percentage increase reached 164.09%. The area of mangrove forests under the three future scenarios in the Guangdong–Hong Kong–Macao Greater Bay Area also demonstrated increasing trends, among which the area increased the most under the TCS, followed by the ERPS and EDS. By 2035, the mangrove forest area will reach 61.54, 59.95, and 52.21 km2, respectively, with percentage increases of 50%, 44%, and 28%, respectively.
In summary, the mangrove forests in both urban agglomerations showed increasing trends from 1990 to 2000, and the variation in the Guangdong–Hong Kong–Macao Greater Bay Area was greater than that in the Guangxi Beibu Gulf. Increasing trends can be observed under the three future scenarios for the two coastal agglomerations. The TCS showed the greatest variation, followed by the ERPS and EDS.
3.2.2. Spatial changes in mangrove forests
As shown in , in the Guangxi Beibu Gulf, the mangrove forests located in the Beilun River estuary, Pearl Bay, Tieshan Harbor, and Dandou Sea remained stable from 1990–2020. However, the mangrove forests in the Maowei Sea, Dafeng River, and Lianzhou Bay decreased first and then increased during the same period. The loss of mangrove forests was observed from 1990–2000 in the Maowei Sea and Dafeng River, followed by a significant increase after 2000. First, mangrove forests in Lianzhou Bay expanded toward the sea, and the newly grown areas were characterized as large patches. During 2010–2020, the mangrove forests in Lianzhou Bay increased, similar to those in the Maowei Sea and Dafeng River. In 2020–2035, mangrove forests under the three future scenarios will experience a stable or stably increasing trend, with the stable area larger than the gain area.
Figure 5. Spatial distribution of stable, gain, and loss areas of mangrove forests in the Guangxi Beibu Gulf and Guangdong–Hong Kong–Macao Greater Bay Area in 1990–2020 and 2020–2035.

indicates that during 1990–2000, the mangrove forests in the Shenzhen Bay core area of the Guangdong–Hong Kong–Macao Greater Bay Area were stable overall but exhibited an obvious string-like gain pattern toward the coastline and a sparse loss trend toward the inland areas. The Zhenhai and Yinhu harbors experienced a loss first, followed by a gain. The mangrove forests on Qi’ao Island increased to a relatively large scale and finally stabilized. Specifically, 1) both the Zhenhai and Yinhu harbors, located in the western and central parts of the study area, experienced linear degradation during 1990–2000; 2) the most serious degradation in the study area occurred during 2000–2010, especially in Jin Bay, located in the center of the Guangdong–Hong Kong–Macao Greater Bay Area. 3) Mangrove forests in the Guangdong–Hong Kong–Macao Greater Bay Area increased significantly during 2000–2020. For example, in 2000–2010, the mangrove forests in Zhenhai Bay continued to grow, those in Yinhu Harbor started to grow and recover, and those in northwestern Qi’ao Island also showed an increasing trend; in 2010–2020, mangrove forests continuously increased in Zhenhai Bay, those in Yinhu Bay started to increase and recover, those on Qi’ao Island showed a ring-like expansion northward and westward, while mangrove forests in Shenzhen Bay showed a linear increase along the coastline toward the north and south.
As shown in , the mangrove forests in protected areas, such as the Beilun River estuary, Pearl Bay, Dandou Sea, and Shenzhen Bay, tend to be more stable. Thus, improving the mangrove forest inventory in protected areas is highly important for the sustained growth of mangrove forests. Mangrove forests grew in large patches in an oceanward direction from the coastline, such as in Lianzhou Bay and Shenzhen Bay ().
3.3. Evaluation of the effectiveness of the conservation and recovery of mangrove forests based on the SDGs
The mangrove forests in the Guangxi Beibu Gulf increased by 11.80 km2 from 69.85 km2 in 2015 to 81.65 km2 in 2020, with a rate of 16.89%; the mangrove forests in the Guangdong–Hong Kong–Macao Greater Bay Area increased by 7.70 km2 from 33.22 km2 in 2015 to 40.92 km2 in 2020, with a rate of 23.19%. The variations in mangrove forests in both the Guangxi Beibu Gulf and Guangdong–Hong Kong–Macao Greater Bay Area reached the standard for SDG 6.6.1.
In 2020, 36.74 and 25.24 km2 of mangrove forests in the Guangxi Beibu Gulf and Guangdong–Hong Kong–Macao Greater Bay Area, respectively, were protected, with conservation rates of 45% and 62%, respectively. Thus, the conservation rates in both study areas reached the target of 10%, as stated in SDG 14.5.1.
4. Discussion
4.1. Evaluation of the conservation and recovery potential of mangrove forests based on the Global Mangrove Alliance Goal
We introduced the Global Mangrove Alliance Goal in detail in Section 2.3.2. According to our calculations, the mangrove forests in the Guangxi Beibu Gulf and Guangdong–Hong Kong–Macao Greater Bay Area increased by 12.47 and 17.14 km2, respectively, from 1995 to 2020. The spatial variations show a stable/increasing trend during 2020–2030, which indicates that the goal of halting and restoring losses has been achieved. If the current number and range of protected areas are maintained, the conservation rates of mangrove forests in the Guangxi Beibu Gulf may further increase from 45% to 56%, 54%, and 50% under the TCS, ERPS, and EDS, respectively, and those in the Guangdong–Hong Kong–Macao Greater Bay Area may increase from 62% to 67%, 65%, and 62%, respectively, during 2020–2030. However, these two areas failed to meet the 80% conservation rate required by the Global Mangrove Alliance Goal. Nature reserves have been shown to contribute significantly to mangrove protection (Jia et al. Citation2018; Wei et al. Citation2021). Therefore, to achieve the protection rate of 80% for the GMAG, more protected areas should be established in addition to recovery and planting. On the one hand, new protection areas, such as reserves or natural parks, could be established in areas with concentrated mangrove forest resources but under inadequate protection. On the other hand, integrating and optimizing current nature reserves is a good choice. For instance, mangrove forest wetlands outside protected areas could be included in the nearest area.
The future multiscenario simulations used in this study could anticipate the challenges faced by mangrove conservation and restoration in the study area and quantify the disparities between mangrove protection rates and the targeted protection rate. Moreover, proactive measures to expand protected areas can be proposed in advance.
4.2. Possible policy impacts
Policies collectively influence the conservation and restoration of mangroves in the study area at the global, national, and local levels (). At the global level, although the SDGs and the Global Mangrove Alliance may not directly intervene in the mangrove management of the study area, they guide national management strategies. For instance, following the introduction of the SDGs in 2015, China promptly released the ‘National Plan on Implementation of the 2030 Agenda for Sustainable Development’ in 2016, emphasizing the ‘protection and restoration of wetland ecosystems’ (http://newyork.fmprc.gov.cn/wjb_673085/zfxxgk_674865/gknrlb/tywj/zcwj/201610/t20161012_9869225.shtml). Consequently, the protection of mangrove wetlands has become a crucial aspect of China's efforts to implement the 2030 Agenda for Sustainable Development. Since the Global Mangrove Alliance published the ‘2022 Global Mangrove Status Report’, it has garnered widespread attention from government departments, social organizations, and other stakeholders.
At the national level, in recent years, China has released and implemented a series of legal policies and projects related to mangrove conservation (), providing legal safeguards for the high-quality development of mangrove wetland protection and specifying protection tasks for regions. In 2020, the Ministry of Natural Resources and the National Forestry and Grassland Administration (Citation2020) of China formulated The Special Action Plan for Mangrove Conservation and Recovery (2020–2025). The action plan states ‘to implement ecological recovery in suitable areas, but carry out nurture, improvement, and transformation of the previous ones to new ones in the degraded areas to increase the mangrove forests and further enhance their quality and functions. The targeted newly built and recovering areas of mangrove forests by 2025 will be 18,800 hectares – 9,050 and 9,750 hectares for the two types of areas, respectively.’
At the local level, policies are more specific, involving detailed research, planning, and verification of suitable areas and species for conservation and restoration. This directly impacts local mangrove conservation and restoration efforts. The local government of Guangxi, where the Guangxi Beibu Gulf is situated, released the Regulations of Guangxi Zhuang Autonomous Region on the Conservation of Mangrove Resources, and this is the first time that China has promulgated specialized laws in this regard. The Forestry Bureau of Guangxi Zhuang Autonomous Region (Citation2021) announced the Guangxi Mangrove Resources Conservation Plan (2020–2030), in which the Forestry Bureau proposed a goal; that is, by 2025, Guangxi will build 10 km2 and recover 35 km2 of mangrove forests. Since September 2022, 4.57 and 14.55 km2 of mangrove forests have been planted and recovered in the Guangxi Beibu Gulf, respectively. Comprehensive management of mangrove forests and the coastal zone of the Fengjiajiang River Basin of Beihai has been selected as one of the best nature-based solutions by the IUCN.
Guangdong Province, where the Guangdong–Hong Kong–Macao Greater Bay Area is located, has also issued similar documents. For instance, the Wetland Conservation and Recovery Implementation Plan of Guangdong Province was released in 2017. The Regulations on Wetland Conservation of Guangdong Province were revised and approved in 2020. The Implementation Plan of the Special Action Plan for Mangrove Conservation and Recovery of Guangdong Province was printed and distributed in 2021. The Specialized Planning on Mangrove Conservation and Recovery of Guangdong Province (Citation2023) proposed the following goal: ‘By 2025, Guangdong will plant 55 km2 and recover 25 km2 of mangrove forests and establish 4 demonstration areas with mangrove forests over 1,000,000 mu (1 ha = 15 mu)’. As of June 2023, Guangdong had planted 17 km2 and recovered 18 km2 of mangrove forests (https://www.sohu.com/a/683556391_161795).
Therefore, because the trend continuation scenario followed the trends observed in mangroves from 2015 to 2020, and coincidentally, both the Guangxi Beibu Gulf and Guangdong–Hong Kong–Macao Greater Bay Area have in recent years implemented highly proactive policies for mangrove creation and restoration and the growth rate of mangroves has been significant, reaching 16.89% and 23.19%, respectively, the highest growth rate among the three scenarios was observed for the trend continuation scenario. In this study, without considering the rise in sea level (Gilman et al. Citation2006), mangroves in the three scenarios from 2020 to 2035 remained stable and experienced rapid growth, with the growth area exceeding the stable area.
4.3. Study strengths and limitations
This study proposes an analytical model based on the full process chain (past–present–future) of spatiotemporal changes in mangrove forests. Compared to traditional monitoring of past mangrove changes and recent mangrove future predictions (Sarker et al. Citation2019; Pimple et al. Citation2023), the primary innovation of this study lies in predicting the future trends of mangroves under three different scenarios and coupling the assessment of mangrove protection with the integration of the SDGs and GMAG. The trend continuation scenario, the ecological restoration and protection scenario, and the economic development scenario consider local natural conditions, economic development, and government measures from different perspectives. These scenarios provide developmental reference models for the future restoration, protection, and management of mangroves. In addition, this study considers both the SDGs (target year: 2020) and the Global Mangrove Alliance Goal (target year: 2030). It evaluates the effectiveness of mangrove forest conservation in the past. Additionally, it assesses the potential for future conservation and recovery of mangrove forests according to the Global Mangrove Alliance Goal. Based on these results, we can establish effective measures to consolidate the outcomes of conservation.
This study has several limitations. First is the limitation of the data, which are extracted based on Landsat images (30 m×30 m per pixel), which is not good for extracting scattered small patches of mangrove forests, but the data still have good accuracy. Second, the limitations of future mangrove simulations, such as parameter settings, may affect the results, and the model itself may have uncertainties. Nevertheless, our results provide a good reference for future monitoring and evaluation. Third, in terms of target evaluation, the interaction between indicators cannot be ignored in terms of their impact on mangrove forest restoration and protection. Currently, only some indicators are selected for evaluation, and in the future, comprehensive indicators, such as SDG 15 ‘Sustainable Land Ecosystems’ related to forests, SDG 13 ‘Climate Action’ related to mangrove blue carbon, and SDG 11 ‘Sustainable Cities and Communities’ relevant to urban agglomerations, should be evaluated in combination with local planning to provide more targeted and intelligent services.
5. Conclusions
The mangrove forests in both the Guangxi Beibu Gulf and Guangdong–Hong Kong–Macao Greater Bay Area showed an increasing trend from 1990–2020, which benefited from the positive protection policies of the national and local governments of China. From 2020 to 2035, the trends under all three scenarios increase. The mangrove growth area is the largest under the trend continuation scenario, followed by the ecological restoration and protection scenario and then the economic development scenario. By evaluating the SDGs and Global Mangrove Alliance Goal, it was found that previous conservation measures have achieved great success. However, future work still faces difficulties and challenges. One of the recommended measures is to establish new nature reserves, integrate and enlarge the current reserves, and gradually increase the proportion of mangrove forests in nature reserves to provide substantial conservation of mangrove forests located outside traditional protection areas.
The spatiotemporal analysis model encompassing the past, present, and future scenarios, as well as the assessment method combining the SDGs and Global Mangrove Alliance Goal effectiveness and potential employed in this study, is applicable for monitoring, analyzing, and evaluating mangrove changes in other global regions. It can support mangrove management and conservation efforts worldwide.
CRediT authorship contribution statement
Ziyan Ling: Methodology, Software, Data curation, writing–original draft. Weiguo Jiang: Conceptualization, review, and editing. Project administration. Kaifeng Peng: Methodology, Data curation. Ze Zhang: Writing-review. Zhifeng Wu: Writing-review. Shiquan Zhong: Resources. Aiping Chu: Writing–Review Zhijie Xiao: Data curation. Ziyu Sun: Data curation.
Disclosure statement
No potential conflict of interest was reported by the author(s).
Data availability statement
The authors were unable to specify which data were used.
Additional information
Funding
References
- Bunting, P., A. Rosenqvist, L. Hilarides, R. M. Lucas, N. Thomas, T. Tadono, T. A. Worthington, M. Spalding, N. J. Murray, and L.-M. Rebelo. 2022. “Global Mangrove Extent Change 1996– 2020: Global Mangrove Watch Version 3.0.” Remote Sensing 14: 3657. https://doi.org/10.3390/rs14153657.
- Duke, N. 2017. “Mangrove Floristics and Biogeography Revisited: Further Deductions from Biodiversity hot Spots, Ancestral Discontinuities, and Common Evolutionary Processes.” In Mangrove Ecosystems: A Global Biogeographic Perspective: Structure, Function, and Services, edited by V. H. Rivera-Monroy, S. Y. Lee, E. Kristensen, and R. R. Twilley, 17–53. Cham, CH: Springer International Publishing. https://doi.org/10.1007/978-3-319-62206-4_2.
- Duke, N., I. Nagelkerken, T. Agardy, S. Wells, H. Van Lavieren, and M. Huxham. 2014. “The Importance of Mangroves to People: A Call to Action.” Cambridge: United Nations Environment Programme World Conservation Monitoring Centre (UNEP-WCMC). http://researchrepository.napier.ac.uk/Output/429439.
- Elmahdy, S. I., T. A. Ali, M. M. Mohamed, F. M. Howari, M. Abouleish, and D. Simonet. 2020. “Spatiotemporal Mapping and Monitoring of Mangrove Forests Changes from 1990 to 2019 in the Northern Emirates, UAE Using Random Forest, Kernel Logistic Regression and Naive Bayes Tree Models.” Frontiers in Environmental Science 8: 102. https://doi.org/10.3389/fenvs.2020.00102.
- Feng, Z., G. Tan, J. Xia, C. Shu, P. Chen, M. Wu, and X. Wu. 2020. “Dynamics of Mangrove Forests in Shenzhen Bay in Response to Natural and Anthropogenic Factors from 1988 to 2017.” Journal of Hydrology 591: 125271. https://doi.org/10.1016/j.jhydrol.2020.125271.
- The Forestry Bureau of Guangxi Zhuang Autonomous Regions. 2021. Guangxi Mangrove Forests Resource Protection Plan (2020–2030). Nanning, Guangxi, China: The Forestry Bureau of Guangxi Zhuang Autonomous Regions.
- Friess, D. A., T. T. Aung, M. Huxham, C. Lovelock, N. Mukherjee, and S. Sasmito. 2019. “SDG 14: Life Below Water–Impacts on Mangroves.” Sustainable Development Goals. Cambridge: Cambridge University Press, 445, 445–481.
- Gilman, E., H. Van Lavieren, J. Ellison, V. Jungblut, L. Wilson, F. Areki, G. Brighouse, et al. 2006. Pacific Island Mangroves in a Changing Climate and Rising Sea-UNEP Regional Seas Reports and Studies No. 179. Nairobi, Kenya: United Nations Environment Programme.
- Giri, C., J. Long, S. Abbas, R. M. Murali, F. M. Qamer, B. Pengra, and D. Thau. 2015. “Distribution and Dynamics of Mangrove Forests of South Asia.” Journal of Environmental Management 148: 101–111. https://doi.org/10.1016/j.jenvman.2014.01.020.
- Global Mangrove Alliance. 2022. The State of the World's Mangroves 2022. New York, NY, USA: Global Mangrove Alliance.
- Goldberg, L., D. Lagomasino, N. Thomas, and T. Fatoyinbo. 2020. “Global Declines in Human-Driven Mangrove Loss.” Global Change Biology 26: 5844–5855. https://doi.org/10.1111/gcb.15275.
- Gomes, E., M. Inácio, K. Bogdzevič, M. Kalinauskas, D. Karnauskaitė, and P. Pereira. 2021. “Future Land-Use Changes and Its Impacts on Terrestrial Ecosystem Services: A Review.” Science of The Total Environment 781: 146716. https://doi.org/10.1016/j.scitotenv.2021.146716.
- Guangdong Provincial Department of Natural Resources, Guangdong Provincial Forestry Bureau. 2023. Special Plan for Mangrove Protection and Restoration in Guangdong Province. Guangzhou, Guangdong, China: Guangdong Provincial Department of Natural Resources, Guangdong Provincial Forestry Bureau.
- Guo, H., Y. Cai, Z. Yang, Z. Zhu, and Y. Ouyang. 2021. “Dynamic Simulation of Coastal Wetlands for Guangdong-Hong Kong-Macao Greater Bay Area Based on Multi-Temporal Landsat Images and FLUS Model.” Ecological Indicators 125: 107559. https://doi.org/10.1016/j.ecolind.2021.107559.
- He, C., D. Zhang, Q. Huang, and Y. Zhao. 2016. “Assessing the Potential Impacts of Urban Expansion on Regional Carbon Storage by Linking the LUSD-Urban and InVEST Models.” Environmental Modelling & Software 75: 44–58. https://doi.org/10.1016/j.envsoft.2015.09.015.
- Jia, M. 2014. Remote Sensing Analysis of Dynamic Changes of Chinese Mangrove Forest from 1973 to 2013. PhD dissertation. Northeast Institute of Geography and Agricultural Ecology, Chinese Academy of Sciences.
- Jia, K., S. Chen, and W. Jiang. 2022. “Long Time-Series Remote Sensing Monitoring of Mangrove Forests in the Guangdong-Hong Kong-Macao Greater Bay Area.” Natl. Remote Sens. Bull 26: 1096–1111. https://doi.org/10.11834/jrs.20221451.
- Jia, M., Z. Wang, D. Mao, C. Huang, and C. Lu. 2021. “Spatial-temporal Changes of China’s Mangrove Forests Over the Past 50 Years: An Analysis Towards the Sustainable Development Goals (SDGs).” Chinese Science Bulletin 66: 3886–3901. https://doi.org/10.1360/TB-2020-1412.
- Jia, M., Z. Wang, D. Mao, C. Ren, K. Song, C. Zhao, C. Wang, X. Xiao, and Y. Wang. 2023. “Mapping Global Distribution of Mangrove Forests at 10-m Resolution.” Science Bulletin 68 (12): 1306–1316. https://doi.org/10.1016/j.scib.2023.05.004.
- Jia, M., Z. Wang, Y. Zhang, D. Mao, and C. Wang. 2018. “Monitoring Loss and Recovery of Mangrove Forests During 42 Years: The Achievements of Mangrove Conservation in China.” International Journal of Applied Earth Observation and Geoinformation 73: 535–545. https://doi.org/10.1016/j.jag.2018.07.025.
- Li, G., C. Fang, Y. Li, Z. Wang, S. Sun, S. He, W. Qi, et al. 2022a. “Global Impacts of Future Urban Expansion on Terrestrial Vertebrate Diversity.” Nature Communications 13 (1): 1628. https://doi.org/10.1038/s41467-022-29324-2.
- Li, C., M. K. Ng, Y. Tang, and T. Fung. 2022b. “From a ‘World Factory’to China’s Bay Area: A Review of the Outline of the Development Plan for the Guangdong-Hong Kong-Macao Greater Bay Area.” Planning Theory & Practice 23 (2): 310–314. https://doi.org/10.1080/14649357.2021.1958539.
- Liu, K., L. Peng, X. Li, M. Tan, and S. Wang. 2019. “Monitoring the Inter-Annual Change of Mangroves Based on the Google Earth Engine.” Journal of Geo-information Science 21 (5): 731–739. https://doi.org/10.12082/dqxxkx.2019.180354.
- Lorenzo, E. N., B. R. De Jesus Jr, and R. S. Jara. 1979. “Assessment of Mangrove Forest Deterioration in Zamboanga Peninsula, Philippines, Using Landsat MSS Data.” Thirteenth International Symposium on Remote Sensing of Environment 106 (11): 166A–167A.
- Lymburner, L., P. Bunting, R. Lucas, P. Scarth, I. Alam, C. Phillips, C. Ticehurst, and A. Held. 2020. “Mapping the Multi-Decadal Mangrove Dynamics of the Australian Coastline.” Remote Sensing of Environment 238: 111185. https://doi.org/10.1016/j.rse.2019.05.004.
- Marois, D. E., and W. J. Mitsch. 2015. “Coastal Protection from Tsunamis and Cyclones Provided by Mangrove Wetlands–a Review.” International Journal of Biodiversity Science, Ecosystem Services & Management 11 (1): 71–83. https://doi.org/10.1080/21513732.2014.997292.
- Menéndez, P., I. J. Losada, M. W. Beck, S. Torres-Ortega, A. Espejo, S. Narayan, P. Díaz-Simal, and G. M. Lange. 2018. “Valuing the Protection Services of Mangroves at National Scale: The Philippines.” Ecosystem Services 34: 24–36. https://doi.org/10.1016/j.ecoser.2018.09.005.
- Ministry of Natural Resources of the People’s Republic of China, National Forestry and Grassland Administration. 2020. Special Action Plan for Mangrove Conservation and Restoration (2020–2025). Beijing, China: Ministry of Natural Resources of the People’s Republic of China, National Forestry and Grassland Administration.
- Nie, W., B. Xu, F. Yang, Y. Shi, B. Liu, R. Wu, W. Lin, H. Pei, and Z. Bao. 2023. “Simulating Future Land use by Coupling Ecological Security Patterns and Multiple Scenarios.” Science of The Total Environment 859: 160262. https://doi.org/10.1016/j.scitotenv.2022.160262.
- Peng, K. 2022. “Remote Sensing Classification of Wetlands in Coastal Urban Agglomerations and Simulation of Future Spatial Changes: A Case Study of Guangdong-Hong Kong-Macao Greater Bay Area and Guangxi Beibu Gulf.” PhD dissertation. Beijing.: Beijing Normal University.
- Peng, K., W. Jiang, P. Hou, Z. Wu, and T. Cui. 2024. “Detailed Wetland-Type Classification Using Landsat-8 Time-Series Images: A Pixel-and Object-Based Algorithm with Knowledge (POK).” GIScience & Remote Sensing 61 (1): 2293525. https://doi.org/10.1080/15481603.2023.2293525.
- Peng, K., W. Jiang, X. Wang, P. Hou, Z. Wu, and T. Cui. 2023. “Evaluation of Future Wetland Changes Under Optimal Scenarios and Land Degradation Neutrality Analysis in the Guangdong-Hong Kong-Macao Greater Bay Area.” Science of The Total Environment 879: 163111. https://doi.org/10.1016/j.scitotenv.2023.163111.
- Pimple, U., D. Simonetti, R. Peters, U. Berger, E. Podest, and V. Gond. 2023. “Enhancing Monitoring of Mangrove Spatiotemporal Tree Diversity and Distribution Patterns.” Land Degradation & Development 34 (5): 1265–1282. https://doi.org/10.1002/ldr.4537.
- Qin, K., J. Liu, L. Yan, and H. Huang. 2019. “Integrating Ecosystem Services Flows into Water Security Simulations in Water Scarce Areas: Present and Future.” Science of the Total Environment 670: 1037–1048. https://doi.org/10.1016/j.scitotenv.2019.03.263.
- Sarker, S. K., R. Reeve, N. K. Paul, and J. Matthiopoulos. 2019. “Modelling Spatial Biodiversity in the World's Largest Mangrove Ecosystem—The Bangladesh Sundarbans: A Baseline for Conservation.” Diversity and Distributions 25: 729–742. https://doi.org/10.1111/ddi.12887.
- Tomlinson, P. B. 2016. The Botany of Mangroves (2nd ed.). Cambridge University Press. https://doi.org/10.1017/CBO9781139946575.
- United Nations. 2015. Transforming Our World: The 2030 Agenda for Sustainable Development. New York, NY, USA: United Nations.
- Veerkamp, C. J., R. W. Dunford, P. A. Harrison, M. Mandryk, J. A. Priess, A. M. Schipper, E. Stehfest, and R. Alkemade. 2020. “Future Projections of Biodiversity and Ecosystem Services in Europe with Two Integrated Assessment Models.” Regional Environmental Change 20: 1–14. https://doi.org/10.1007/s10113-020-01685-8.
- Wang, L., M. Jia, D. Yin, and J. Tian. 2019. “A Review of Remote Sensing for Mangrove Forests: 1956–2018.” Remote Sensing of Environment 231: 111223. https://doi.org/10.1016/j.rse.2019.111223.
- Wang, Z., K. Liu, L. Peng, J. Cao, Y. Sun, Y. Qian, and S. Y. Shi. 2020. “Analysis of Mangrove Annual Changes in Guangdong Province During 1986-2018 Based on Google Earth Engine.” Tropical Geography 40 (5): 881–892. https://doi.org/10.13284/j.cnki.rddl.003268.
- Wei, S., Y. Lin, L. Wan, G. Lin, Y. Zhang, and H. Zhang. 2021. “Developing a Grid-Based Association Rules Mining Approach to Quantify the Impacts of Urbanization on the Spatial Extent of Mangroves in China.” International Journal of Applied Earth Observation and Geoinformation 102: 102431. https://doi.org/10.1016/j.jag.2021.102431.
- Zhang, Z., W. Jiang, K. Peng, Z. Wu, Z. Ling, and Z. Li. 2023. “Assessment of the Impact of Wetland Changes on Carbon Storage in Coastal Urban Agglomerations from 1990 to 2035 in Support of SDG15. 1.” Science of The Total Environment 877: 162824. https://doi.org/10.1016/j.scitotenv.2023.162824.
- Zheng, X. Y., Y. Wang, M. Y. Gan, J. Zhang, L. M. Teng, K. Wang, Z. Q. Shen, and L. Zhang. 2016. “Discrimination of Settlement and Industrial Area Using Landscape Metrics in Rural Region.” Remote Sensing 8 (10): 845. https://doi.org/10.3390/rs8100845.