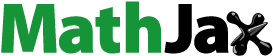
ABSTRACT
Nature reserves play a key role in the conservation of habitats, species and ecosystems in China. However, the issue of light pollution within these reserves has not received well recognition. Whereas previous studies have investigated light pollution in protected areas by remote sensing, the influence of the atmospheric scattered light from neighborhoods was ignored by using only direct light emissions. This study mapped the nighttime all-sky brightness from NPP/VIIRS data to quantify light pollution over the national nature reserves in China from 2013 to 2022. Based on the remotely sensed sky brightness, the spatio-temporal variations of sky brightness were analyzed. The mean sky brightness across all nature reserves was 0.63 mcd/m2 and the mean trend was 0.0189 mcd/m2/a, indicating obvious and accelerating light pollution. Furthermore, the sky brightness around the nature reserves was estimated and compared with the reserves. In general, the surrounding regions showed higher sky brightness and brightening trends, suggesting a certain but insufficient protective effect on the sky quality of nature reserves. This study provides a comprehensive understanding of the light pollution conditions in the national nature reserves in China and serves as a valuable reference for future assessments of light pollution using remote sensing data.
1. Introduction
Since the invention of electric light, various artificial lights have been utilized to illuminate the nocturnal environment. The introduction of artificial lighting at night provides flexibility and convenience to human activities, thereby promoting economic development and quality of life (Gaston et al. Citation2015; Sánchez de Miguel et al. Citation2022; Xu, Knudby, and Côté-Lussier Citation2018). Over the past few decades, anthropogenic light emission has dramatically increased due to the advancement of the economy and technology, specifically in the field of light-emitting diode (LED) lighting technology. From 2012 to 2016, the artificially lit area of the world has shown a continuously increasing trend at a rate of 2.2% per year (Kyba et al. Citation2017).
Despite the apparent benefits, artificial lighting also has a dark side. Excessive illumination at night has resulted in various negative environmental consequences, known as light pollution. The light pollution was initially recognized by astronomers. The widespread use of artificial lighting has brightened the night sky, diminishing the availability of darkness as an increasingly scarce resource and posing obstacles to astronomical observations. Approximately 80% of the world’s population live under light-polluted skies, and one-third are unable to see the galaxy (Falchi et al. Citation2016). Moreover, sky brightness has increased dramatically in recent years. From 2011 to 2022, the sky brightness increased at an annual rate of 9.6% according to citizen scientist observations (Kyba et al. Citation2023). The consequences of light pollution extend beyond the obscuring of the starry sky, impacting human health as well. Bright nighttime environments disrupt human circadian rhythms, leading to a range of physiological and psychological problems, including reduced sleep quality and quantity, depressed mood, metabolic disorders, and an increased incidence of cataracts and cancers (Bedrosian and Nelson Citation2013; Kohyama Citation2009; Kooijman et al. Citation2015; Navara and Nelson Citation2007; Stevens Citation2009). In addition to these human health consequences, light pollution has adverse effects on animals and plants as well. Many behavioral and physiological processes of living organisms depend on circadian rhythms, which are generally regulated by natural light sources such as the sun and moon. Artificial lighting alters the nighttime light environment, disturbing the activities of animals and plants. Studies have shown that a wide range of species, including mammals, reptiles, amphibians, birds, insects, fish, and plants, are affected by exposure to artificial light (Adams et al. Citation2021; Bassi et al. Citation2022; Bennie et al. Citation2016; Dani, Molnár, and Skribanek Citation2021; Deng et al. Citation2019; Desouhant et al. Citation2019; Kamrowski et al. Citation2012; Kumari et al. Citation2021). Raap, Pinxten, and Marcel (Citation2015) reported that light pollution had significant impacts on the sleep of free-living animals, underscoring the potential health effects of artificial light exposure. Sangma and Trivedi (Citation2023) found that light exposure affected the daily rhythms and cognitive functions of mice. Lockett et al. (Citation2022) revealed that artificial light had bottom-up effects on the eucalyptus forest food chain, impacting trees, herbivores, and various species at higher trophic levels. Viera-Pérez et al. (Citation2019) reported a significant decrease in the flower and seed production of foredune vegetation near artificial light sources compared to those far from light sources.
Accurate knowledge of the spatio-temporal variations of light pollution is of fundamental importance for its assessment and mitigation. There are two ways to monitor light pollution: in-situ observation and remote sensing. In-situ observation records environment brightness at night using devices such as illuminance meters, luminance meters, or digital cameras. Liu et al. (Citation2022) used the sky quality meter (SQM), a widely used luminance meter, to continuously monitor the urban surface nighttime light environment in Dalian, China for five years. Aguilera and González (Citation2023) implemented illuminance meters to measure the artificial light pollution within the coastal ecosystem of Coquimbo and La Serena, Dias et al. (Citation2019) employed an illuminance meter to evaluate light pollution in the natural wetlands of Brazil’s Rio Grande municipality. Fiorentin et al. (Citation2022) used SQM observations to analyze long-term trends in night sky brightness in Veneto, Italy and La Silla, Chile.
Nonetheless, in-situ observation can only provide point-scale measurements at specific locations. Due to the significant spatial difference of light, in-situ observation cannot adequately characterize the detailed distribution of light pollution. On the other hand, nighttime light remote sensing provides spatially continuous observations of surface light using sensors mounted on space-borne or aircraft-borne platforms, enabling large-scale and timely measurements of light pollution. The Defense Meteorological Satellite Program (DMSP) was the first generation of nighttime light remote sensing satellites. The Operational Linescan System (OLS) onboard DMSP can detect visible light at light, with a spatial resolution of approximately 2.7 km. DMSP/OLS data has been extensively utilized for light pollution evaluation (Butt Citation2012; Cinzano, Falchi, and Elvidge Citation2001; Han et al. Citation2014; Jiang et al. Citation2017; Kumar et al. Citation2019). However, DMSP/OLS has the limitations of coarse resolution, saturation and blooming effects. In 2011, the National Polar-orbiting Partnership (NPP) was launched, carrying the Visible Infrared Imaging Radiometer Suite (VIIRS). Compared to DMSP/OLS, NPP/VIIRS has a higher spatial resolution and a wider radiometric range, providing improved data for light pollution monitoring NPP/VIIRS data has been introduced in light pollution studies in several regions (Adelabu, Mncube, and Adagbasa Citation2022; Aksaker et al. Citation2020; Cox and Gaston Citation2023; Hu, Hu, and Huang Citation2018).
Nature reserves play a key role in the preservation of habitats, species and ecosystems (Cetas and Yasué Citation2017; Soulé and Simberloff Citation1986; Sun, Sang, and Axmacher Citation2020). However, these areas are still affected by human interactions. In recent decades, human activities have drastically and extensively altered ecosystems (Venter et al. Citation2016). Among the human-induced disturbances, light pollution has profound negative effects on species and ecology, as described above. To mitigate the impact of artificial light on ecological systems, a comprehensive knowledge of light pollution within nature reserves is of great importance. Various studies have been carried out to assess the nighttime light environment in protected areas using remote sensing data. Davies et al. (Citation2016) reported widespread and increasing artificial light in the world’s marine protected areas from 1992 to 2012 based on DMSP/OLS data. Xiang and Tan (Citation2017) used DMSP/OLS data to examine light pollution in China’s protected areas from 1992 to 2012 and found that artificial light had significantly increased with apparent spatial variations. Guetté et al. (Citation2018) assessed the spatio-temporal trends of artificial light around global protected areas between 1993 and 2012 using DMSP/OLS data. The results indicated that artificial light was consistently low within the protected areas but high and increasing in the surrounding areas. Zheng et al. (Citation2021) utilized a harmonized NTL dataset that combines DMSP/OLS and DMSP-like NTL data transformed from NPP/VIIRS data to monitor light pollution in African protected areas from 1992 to 2018. They found an increasing trend of artificial light in these areas. Wang et al. (Citation2022) used the same harmonized NTL dataset to explore the spatio-temporal variations of light pollution over the Tibetan Plateau and discovered an increase in light-polluted areas from 1.2 to 82.8 thousand km2 over the period of 1992–2018. LaRoe, Holmes, and Schad (Citation2022) investigated nightlight changes around the Orbroicher Bruch Nature Reserve in Germany from 1992 to 2010 based on DMSP/OLS data and found that 96% of the area exhibited an increasing trend.
Previous studies mostly used DMSP/OLS data or harmonized NTL data that combined DMSP/OLS and DMSP-like VIIRS data. However, these datasets were limited by coarse resolution, saturation effects and low sensitivity to weak light. Moreover, these studies have primarily used the radiance or DN values of remote sensing data to qualify the nocturnal light environment. Lights do not only influence the influence immediate environment, but also have an impact on distant regions through atmospheric scattering. This phenomenon, known as sky glow, refers to the diffuse light of the night sky. While direct surface light has a limited range, scattered light can extend hundreds of kilometers and is less affected by terrain blocking (Aubé Citation2015; Gaston et al. Citation2015; Kyba and Hölker Citation2013). Only using direct light emissions neglects the impact of scattered light from surrounding areas, especially for dark reserves. In light of this consideration, this paper aims to map the nocturnal sky brightness from NPP/VIIRS nighttime light remote sensing data to characterize the light pollution variations within China’s nature reserves. Compared with previous studies that directly utilized radiometric values without considering the glow effect, our study can provide a more accurate and comprehensive understanding of the light pollution scenario across these nature reserves. Firstly, the all-sky brightness from 2013 to 2022 was estimated based on NPP/VIIRS data. Subsequently, the spatial and temporal trends of light pollution in China’s national nature reserves were evaluated to provide valuable insights into the light pollution management in these nature reserves.
2. Study area and data
2.1. Study area
In this study, the national nature reserves in China were selected as the study area. China is one of the countries with the greatest biodiversity in the world (Liu et al. Citation2003; Yu et al. Citation2022). The establishment of nature reserves serves as an effective strategy to preserve natural ecosystems, rare animals, and plants, and maintain national ecological security. According to the Regulations of the People’s Republic of China on Nature Reserves, a nature reserve an area designated by law for the special protection and management of the land, water, and sea areas where representative natural ecosystems, natural concentrated distribution areas of rare and endangered species of wild animals and plants, and natural monuments of special significance are located. Since the establishment of the first reserve (Dinghu Mountain National Nature Reserve) in 1956, China has established 474 national nature reserves (as illustrated ), forming a nature protection system with complete types, reasonable layout, rich levels and perfect functions.
The 474 national nature reserves cover a vast area of over 1013 thousand km2, accounting for 10.55% of China’s total area. The mean area of these reserves is 2154.28 km2, and the median area is 239.27 km2. This considerable difference between the median and median indicates a highly positive skewed distribution in the sizes of the reserves. Among them, the Qiangtang Nature Reserve stands as the largest one, sprawling across approximately 295 thousand km2, while Shanwang Nature Reserve is the smallest, covering an area of only 1.23 km2.
2.2. Data
The NPP/VIIRS monthly composite cloud-free nighttime light product from 2013 to 2022 was collected from the Earth Observation Group at the Colorado School of Mines (https://eogdata.mines.edu/products/vnl/). The product provides the monthly average radiance of the cloud-free VIIRS DNB band. During the production of the dataset, several processing steps have been implemented to exclude the impacts of cloud cover, solar and lunar interference, background noise, and the irrelevant features such as fires, flares and volcanoes (Elvidge et al. Citation2017). The product spans the globe from 75°N to 65°S latitude, with a spatial resolution of 15 arc-seconds (∼500 m). There are two versions of NPP/VIIRS monthly composite nighttime light data: ‘vcm’ and ‘vcmsl’. The ‘vcm’ version excludes the data contaminated by stray light, whereas the ‘vcmsl’ version includes the stray-light corrected data, offering more data coverage toward the poles at the cost of reduced quality. In this study, the ‘vcm’ version of the NPP/VIIRS data from 2013 to 2022 was employed to map nighttime sky brightness.
Considering the high impact of stray light in summer at high latitudes (Qiu et al. Citation2017), the NPP/VIIRS images from May to September were excluded. Due to the relatively low radiance at night and instrument noise, there are a small number of negative values in the NPP/VIIRS NTL product. These negative pixel values were assigned to zero. Previous studies have shown that NPP/VIIRS monthly data are subject to seasonal effects that mostly attributable to the change in vegetation and snow cover (Levin Citation2017). The annual radiance was calculated on a pixel basis using the median composite method to reduce the seasonal effect. Finally, the data were converted to the Albers equal-area conic projection with a resolution of 500 m.
3. Methods
3.1. Sky brightness mapping
Nighttime all-sky brightness is calculated based on the neighborhood annulus method presented by Duriscoe et al. (Citation2018), which is based on the relationship between sky glow brightness and the distance from the observer to the source of upward light.
The anthropogenic sky light pollution ratio is determined by aggregating the contributions of upward radiance from each NPP/VIIRS pixel within a radius of 300 km:
(1)
(1) where ALR is the anthropogenic sky light pollution ratio, c is a constant (according to the empirical fitting based on ground observations and the weighted sums of NPP/VIIRS radiance at the resolution of 450 m, the constant c is 567.72), n is the number of pixels within the 300-km radius, ri is the VIIRS radiance of pixel i (unit: nW/cm2/sr), di is the distance from pixel i to the center pixel (unit: km), wi is the distance-dependent weight of pixel i, calculated as:
(2)
(2)
ALR is the ratio of anthropogenic sky brightness to natural background sky brightness. The natural mean all-sky brightness is considered as 0.25 mcd/m2. Therefore, the calculated ALR can be converted to nighttime all-sky brightness:
(3)
(3) where NASB is the nighttime all-sky brightness (unit: mcd/m2).
3.2. Sky brightness spatio-temporal analysis
The mean sky brightness over the 10-year period of the 474 national nature reserves was calculated to assess the degree of light pollution. To eliminate the large difference of the areas of different reserves, the mean sky brightness of the individual reserves was calculated and compared. The annual mean sky brightness for all nature reserves was calculated on a reserve basis to illustrate the overall dynamics of light pollution across these nature reserves.
The temporal change of the nighttime sky brightness of the national nature reserves was also quantitatively analyzed. Theil–Sen median method (Sen Citation1968; Theil Citation1992), a robust non-parametric regression analysis method, was employed to discover the temporal changes of nighttime sky brightness within the nature reserves. Compared with traditional ordinary least squares regression, the Theil–Sen method is robust against outliers (Sen Citation1968), which helps to minimize the potential influence of residual fires and flares on the analysis.
The idea of the Theil–Sen method is to calculate all the slopes between each pair of data points and choose the median as the slope (Equation (4)). A positive slope signifies an upward trend, whereas a negative slope indicates a downward trend.
(4)
(4) where β is the trend (unit: mcd/m2/a), NASBi and NASBj are the nighttime all-sky brightness of year i and j, respectively (unit: mcd/m2).
To test the significance of the sky brightness change trends, the Mann–Kendall method was employed. It is a non-parametric test that does not require the data to be normally distributed (Mann Citation1945; Kendall Citation1975), and has been extensively implemented in detecting temporal changes in environmental, climatic, and hydrological datasets. The test statistic S is calculated as (Equation (5)):
(5)
(5)
where n is the length of the time series, NASBi and NASBj are the nighttime sky brightness of year i and j, respectively (unit: mcd/m2).
Lastly, the test statistic Z value is determined by standardizing S:
(6)
(6)
where Z is the significance test statistics, n is the length of the time series, m is the number of ties in the time series; ti is the number of input values inside the ties i.
A positive Z value indicates an increasing trend of the time series, whereas a negative Z value suggests a decreasing trend. The critical test statistical values corresponding to confidence levels of 90%, 95%, and 99% are 1.64, 1.96, and 2.58, respectively. Consequently, a value below 1.64 is considered statistically insignificant, while
values above 1.64, 1.96, and 2.58 denote statistically significant at 90%, 95%, and 99% confidence levels, respectively.
Light pollution is a multifaceted issue that is caused not only by locally emitted light but also by the scattered light from neighbors. The influence of artificial light sources outside the nature reserves cannot be ignored (Zheng et al. Citation2021). In order to assess the influence of the surrounding areas on the sky brightness over nature reserves, the annual mean sky brightness and the changing trend within the surrounding areas were calculated and compared with those within reserves. Given that the influence distance of surface artificial lights on sky brightness usually does not exceed 300 km, 300 km was set as the maximum distance of the buffer zones around the natural reserves. Within the 300-km buffer zones, three surrounding belts were identified: 0–50 km buffer zone (Belt1), 50–150 km buffer zone (Belt2), and 150–300 km buffer zone (Belt3). If the buffer belt of a nature reserve overlaps with other reserves, the overlapping area is excluded from the buffer belt. By comparing the mean values and trends of the sky brightness between the nature reserves and their corresponding buffer belts, the protect effect of the nature reserves and assess the influence of surrounding areas on the reserves were analyzed.
To reveal the spatial clustering characteristics of the light pollution within the nature reserves, the local indicators of spatial association (LISA) statistic was introduced to quantitatively evaluate the local autocorrelation of the sky brightness. The LISA method detects local spatial associations and identifies local clusters. Based on LISA analysis, the cluster maps of the 10-year mean value and the trend of the sky brightness of the nature reserves were generated for aggregation analysis.
4. Results
4.1. Remote sensed sky brightness
Based on the annual nighttime all-sky brightness derived from NPP/VIIRS data, the 10-year mean sky brightness during 2013–2022 were calculated (). The sky brightness over China showed a large spatial difference. In general, the eastern sky was brighter than the western sky and the southern sky was brighter than the northern sky. Urban areas had a sky brightness over 1.7 mcd/m2, while the outer suburbs had a sky brightness below 1.0 mcd/m2. Because most of the nature reserves are located far away from urban areas, the sky in these nature reserves was noticeably darker than in urban areas.
4.2. Sky brightness variations of nature reserves
Give the significant variations in size among the nature reserves, the mean sky brightness of all 474 reserves was calculated by arithmetic averaging the 10-year mean sky brightness of each nature reserve. The resulting mean sky brightness for all reserves was 0.63 mcd/m2, which is 2.5 times brighter than the natural background sky (0.25 mcd/m2), suggesting a substantial level of light pollution within these nature reserves. shows the histogram of the 10-year mean sky brightness of the nature reserves. The histogram was also calculated on the reserve basis to avoid the effects of the large differences in reserve sizes. The mean sky brightness of the nature reserves exhibited an obvious right-skewed distribution, signifying that a majority of the reserves had sky brightness clustered toward the darker end, while a minority exhibited high sky brightness. Notably, Xiamen Valuable and Rare Marine Species Nature Reserve exhibited the brightest sky, with a mean sky brightness of 8.06 mcd/m2. It was followed by Neilingding-Futian Nature Reserve, Shenhuwan Submarine Paleoforest Remains Nature Reserve and Zhujiangkou Chinese White Dolphin Nature Reserve, with mean sky brightness values of 5.28, 4.25, and 4.01 mcd/m2, respectively. These bright nature reserves are located in close proximity to coastal cities, rendering them more susceptible to urban lights. Conversely, Huanglianshan Nature Reserve showcased the darkest sky, with a mean sky brightness of 0.36 mcd/m2. Following closely were Yading Nature Reserve, Haizishan Nature Reserve and Mangkam Yunnan Snub-nosed Monkey Nature Reserve, each with a mean sky brightness value of 0.37 mcd/m2. These dark reserves are situated in sparsely populated mountainous regions of southwest China, thereby experiencing less impact from artificial lighting.
illustrates the temporal variations of the annual mean sky brightness across the 474 nature reserves from 2013 to 2022. It is evident that the annual mean sky brightness demonstrated an obvious increasing trend, with the lowest value recorded at 0.34 mcd/m2 in 2016 and the highest value at 0.56 mcd/m2 in 2022. The Sen’s slope, which represents the annual trend, was found to be 0.0189 mcd/m2/a, which was statistically significant at the 99% confidence level. Taken the baseline sky brightness of 0.39 mcd/m2 in 2013, the sky brightness within the nature reverses increased by 4.90% per year. These findings highlight a notable overall brightening trend of the sky over China’s nature reserves during the past decade. In addition, to explore the underlying reasons behind the lowest sky brightness in 2016, we analyzed the temporal variations of annual precipitation across China from 2013 to 2022 based on the ERA5-land reanalysis dataset. Notably, 2016 recorded the highest annual precipitation during the 10 years. High precipitation led to higher soil moisture, which in turn reduced the surface albedo. As a result, the surface reflected light was lower compared to other years, resulting in a relatively lower sky brightness.
Figure 4. Temporal variations of annual mean sky brightness over nature reserves in China from 2013 to 2022. Shaded areas indicate a 95% confidence level.

To get a comprehensive understanding of the spatial and temporal variations of nighttime sky brightness across the nature reserves, the Thiel–Sen’s slope regression and Mann–Kendall significance test were performed on the pixel-by-pixel basis. (a,b) presents the spatial distribution of the sky brightness change rate and significance level across the 474 nature reserves from 2013 to 2022. The majority of nature reserves exhibited increasing trends in sky brightness higher than 0.016 mcd/m2/a at the 95% confidence level. The brightening trend was observed in an area spanning 1012 thousand km2, accounting for 99.96% of the total area of the national nature reserves. Notably, the eastern nature reserves showed higher brightening trends in sky brightness compared to the western reserves, suggesting that bright reserves trended to experience faster brightening than dark reserves. (c) shows the histogram depicting the annual trends of sky brightness of the nature reserves from 2013 to 2022. All 474 reserves showed a positive trend in sky brightness, with the majority falling below 0.04 mcd/m2/a. Among the 474 national nature reserves, 27, 286, and 155 reserves exhibited significant increases in sky brightness at confidence levels of 90%, 95%, and 99%, respectively. These findings indicate that a significant brightening occurred in most of the national nature reserves over the past decade. Xiamen Valuable and Rare Marine Species Nature Reserve exhibited the highest brightening trend of 0.39 mcd/m2/a, followed by Datian Nature Reserve, Mashan Nature Reserve, Neilingding-Futian Nature Reserve and Shenhuwan Submarine Paleoforest Remains Nature Reserve, with trends of 0.35, 0.26, 0.21 and 0.20 mcd/m2/a, respectively.
Figure 5. Spatial distribution of the annual trend of sky brightness (a) and the significance level of the trend (b) over the national nature reserves in China. Histogram of the sky brightness annual trend (c) and the statistical result of the significance levels and (d) of the national nature reserves in China.

4.3. Comparison between the sky brightness within nature reserves and surroundings
The 10-year mean value and the annual trend of the sky brightness were also calculated for each of the 474 national nature reserves’ corresponding three buffer belts to compare them with values within the reserves. presents the box plots illustrating the mean sky brightness and annual trend within the reserves and the surrounding belts. It is noteworthy that the buffering belts exhibited obviously higher sky brightness than the reserves. Furthermore, as the buffer belts expanded, the sky brightness increased. Specifically, Belt3 had higher sky brightness than Belt2, and Belt2 displayed higher sky brightness compared to Belt1. The difference between Belt3 and Belt2 was smaller than that between Belt2 and Belt1. This suggests that distant buffer belts had higher sky brightness but the difference reduced with distance. Notably, the annual trend of sky brightness showed a similar spatial characteristic to the mean sky brightness. The sky brightness trend within the reserves was also lower than that within the surrounding belts. Specifically, Belt 3 and Belt 2 exhibited higher trends compared to Belt1.
Figure 6. Box plots of the mean value (a) and trend (b) of the all-sky brightness of the nature reserves and their surrounding belts. These two boxplots were also calculated on the reserve basis, not on the pixel basis.

The fact that the surrounding areas exhibited brighter sky brightness and higher change rates compared to the nature reserves suggests a strong influence of the surroundings on the reserves’ sky quality. We explored the relationship between the size of the reserves’ area and the degree of light pollution. The 474 national nature reserves were divided into three size levels based on their areas: small (area < 100 km2), medium (100 km2 < area < 1000 km2) and large (area > 1000 km2). The box plots of the mean sky brightness and trends of these three groups of reserves were shown in . It is evident that small reserves showed obviously higher sky brightness and variability than medium and large reserves. The trend of sky brightness exhibited similar characteristics to the mean sky brightness. Smaller reserves had a much greater likelihood of experiencing rapid increases in nighttime sky brightness.
Figure 7. Box plots of the mean value (a) and trend (b) of the sky brightness over the nature reserves of different area levels.

shows the spatial cluster maps of the mean value and trend of sky brightness of the 474 nature reserves during 2013–2022. In general, The LISA maps of the mean value and trend of sky brightness showed similar spatial patterns. The spatial agglomeration type was mostly significant high–high and low–low reserves, and there were few low–high and high–low clustering reserves, suggesting that the high-value agglomeration and low-value agglomeration were prominent. The high–high clustering reserves of the mean value and trend of sky brightness were concentrated in the eastern coastal areas, which were characterized by high-developed economy. In contrast, the low–low clustering reserves were mainly located in less-developed northeast and western regions. The cluster maps indicated that the nighttime sky brightness was
5. Discussion
Artificial light at night has been demonstrated to have substantial impacts on ecological systems. To mitigate the detrimental effects of light pollution, one of the priority initiatives is to preserve natural darkness in nature reserves. Several remote sensing have investigated light pollution in protected areas, including some conducted in China. However, most of these studies were based on DMSP/OLS or DMSP-like NTL data, which suffer from limitations of coarse resolution, saturation and blooming effects. NPP/VIIRS is a more advanced and accurate night light remote sensing data that is suitable for light pollution monitoring. This study employed NPP/VIIRS NTL data to evaluate the spatio-temporal variations of the nighttime light environment in China’s national nature reserves, which can provide a more comprehensive, accurate and detailed knowledge of the light pollution conditions. Furthermore, previous studies commonly directly used DN values or radiances to indicate the light pollution levels, which only reflect surface brightness, but cannot reflect the diffuse glow of the sky. Our study estimated all-sky brightness to quantify light pollution, enabling a comprehensive characterization of the ambient brightness level.
Our results reveal that China’s national nature reserves have experienced significant and worsening light pollution in the past decade, which is a noteworthy phenomenon. Despite the success of these nature reserves in conserving biodiversity and other natural resources, light pollution has not been effectively controlled due to its non-traditional nature as an environmental pollutant. In addition, the sky brightness and its increasing rate were lower within the reserves compared to the surrounding areas, aligning with the concept of ‘dark islands’ that indicated by Guetté et al. (Citation2018). This suggests that the nature reserves were less exposed to artificial light than outside, highlighting the positive effect of these nature reserves in restricting human activities. The establishment of nature reserves plays a role in protecting them from the disturbance of human activities to some extent. The surrounding areas, particularly the distant buffer belts, showed high increasing rate of sky brightness, indicating their exposure to high levels of artificial light. Surface artificial lights not only influence the nocturnal light environment where they are located but also influence the sky brightness over considerable distances through scattered light. The areas surrounding the nature reserves exhibited both higher sky brightness and higher change rates compared to the reserves themselves, suggesting that there are more artificial lights in these peripheral regions. Therefore, it is important to recognize the non-negligible influence that these surrounding areas exert on the nighttime light environment within the reserves. Moreover, specific phototropic species within the nature reserves may be lured out of reserves, where the bright surroundings function as ecological light traps (Guetté et al. Citation2018). The scientific management of lighting in the vicinity of the nature reserves also needs attention.
Light pollution is an emerging environmental problem alongside rapid technology and economy advancements. There remains a lack of sufficient knowledge about light pollution among both the government and the general public. In China, the detrimental effects of light pollution on the natural environment have not been well examined. Considering the light pollution situation of natural reserves, further studies are necessary to have a clear understanding on the impacts of light pollution on wildlife and ecological system. Moreover, it is imperative to establish a long-term observation network dedicated to monitoring light pollution and to implement national laws and regulations on the prevention and management of light pollution. This will contribute to the development of a healthy and sustainable nighttime light environment that strikes an optimal balance between ecological protection and economic development.
The study also comes with some limitations that should be acknowledged. Firstly, due to the lack of ground measurements of sky brightness in China’s national nature reserves, the remotely sensed nighttime all-sky brightness in this study was not validated. Secondly, while LED light sources have become increasingly popular due to their energy-saving and long-lasting advantages, it is important to recognize that the NPP/VIIRS DNB band does not cover the blue light spectrum, making it unable to fully capture the peak blue light emission of LED sources. Consequently, the monitoring of light pollution induced by LEDs based on NPP/VIIRS data may be incomplete. The replacement of old lamps (such as low-pressure lamps) with new LED ones may not be efficiently quantified by NPP/VIIRS, even though the emissions of new lamps are much higher than old ones. As a result, the increase of light pollution within national reserves could potentially be underestimated. In the future, the development of multispectral NTL data, such as SDGSAT-1 and JL1-3B, could provide a better characterization of light pollution in the blue band.
6. Conclusions
In this study, we investigated the light pollution of China’s national nature reserves from 2013 to 2022 based on NPP/VIIRS nighttime remote sensing data. The annual nighttime all-sky brightness was mapped using a neighborhood annulus method. Then the spatio-temporal variations of sky brightness across the 474 national nature reserves were analyzed to evaluate the light pollution. The mean sky brightness across all nature reserves was measured at 0.63 mcd/m2, indicating an overall presence of light pollution within the reserves. The sky brightness varied greatly among different nature reserves. Xiamen Valuable and Rare Marine Species Nature Reserve exhibited the highest sky brightness of 8.06 mcd/m2, while Huanglianshan Nature Reserve had the lowest sky brightness of 0.36 mcd/m2. Most of the nature reserves showed significant increasing trends in sky brightness, with a mean annual trend of 0.0189 mcd/m2/a, corresponding to a brightening rate of 4.90% per year. Notably, compared with the nature reserves, the surrounding areas showed higher sky brightness and more pronounced increasing trends. The results indicate that the nature reserve has a certain but insufficient protective effect on the sky quality. Therefore, it is crucial to pay close attention to the artificial lights both in and around the nature reserves. This study contributes to a comprehensive understanding of the light pollution conditions within China’s national nature reserves and presents a valuable template for remote sensing-based light pollution assessment in other regions.
Acknowledgements
The authors would like to thank the Earth Observation Group (EOG) of Colorado School of Mines for providing NPP/VIIRS data, and Dr Alejandro Sánchez de Miguel of the University of Exeter for his kind help in mapping nighttime sky brightness. The authors would also like to thank the anonymous reviewers and the editors for their valuable comments on the manuscript.
Disclosure statement
No potential conflict of interest was reported by the author(s).
Data availability statement
The data that support the findings of this study are available from the corresponding author upon reasonable request.
Additional information
Funding
References
- Adams, C. A., E. Fernández-Juricic, E. M. Bayne, and C. C. St. Clair. 2021. “Effects of Artificial Light on Bird Movement and Distribution: A Systematic Map.” Environmental Evidence 10 (37): 1–28. https://doi.org/10.1186/s13750-021-00246-8.
- Adelabu, S., Z. Mncube, and E. Adagbasa. 2022. “Utilising VIIRS DNB Nighttime Light Data Together with Landsat Daytime Data to Assess Changes in Light Pollution in Ethekwini Metropolitan Municipality (EMM).” The International Archives of the Photogrammetry, Remote Sensing and Spatial Information Sciences XLIII-B4-2022 (43): 463–470. https://doi.org/10.5194/isprs-archives-XLIII-B4-2022-463-2022.
- Aguilera, M. A., and M. G. González. 2023. “Urban Infrastructure Expansion and Artificial Light Pollution Degrade Coastal Ecosystems, Increasing Natural-to-Urban Structural Connectivity.” Landscape and Urban Planning 229: 104609. https://doi.org/10.1016/j.landurbplan.2022.104609.
- Aksaker, N., S. K. Yerli, Z. Kurt, M. Bayazit, A. Aktay, and M. A. Erdoğan. 2020. “A Case Study of Light Pollution in France.” Astrophysics and Space Science 365 (9): 153. https://doi.org/10.1007/s10509-020-03869-4.
- Aubé, M. 2015. “Physical Behaviour of Anthropogenic Light Propagation into the Nocturnal Environment.” Philosophical Transactions of the Royal Society B: Biological Sciences 370 (1667): 20140117. https://doi.org/10.1098/rstb.2014.0117.
- Bassi, A., O. P. Love, S. J. Cooke, T. R. Warriner, C. M. Harris, and C. L. Madliger. 2022. “Effects of Artificial Light at Night on Fishes: A Synthesis with Future Research Priorities.” Fish and Fisheries 23 (3): 631–647. https://doi.org/10.1111/faf.12638.
- Bedrosian, T. A., and R. J. Nelson. 2013. “Influence of the Modern Light Environment on Mood.” Molecular Psychiatry 18 (7): 751–757. https://doi.org/10.1038/mp.2013.70.
- Bennie, J., T. W. Davies, D. Cruse, and K. J. Gaston. 2016. “Ecological Effects of Artificial Light at Night on Wild Plants.” Journal of Ecology 104 (3): 611–620. https://doi.org/10.1111/1365-2745.12551.
- Butt, M. J. 2012. “Estimation of Light Pollution Using Satellite Remote Sensing and Geographic Information System Techniques.” GIScience & Remote Sensing 49 (4): 609–621. https://doi.org/10.2747/1548-1603.49.4.609.
- Cetas, E. R., and M. Yasué. 2017. “A Systematic Review of Motivational Values and Conservation Success in and Around Protected Areas.” Conservation Biology 31 (1): 203–212. https://doi.org/10.1111/cobi.12770.
- Cinzano, P., F. Falchi, and C. D. Elvidge. 2001. “The First World Atlas of the Artificial Night Sky Brightness.” Monthly Notices of the Royal Astronomical Society 328 (3): 689–707. https://doi.org/10.1046/j.1365-8711.2001.04882.x.
- Cox, D. T., and K. J. Gaston. 2023. “Global Erosion of Terrestrial Environmental Space by Artificial Light at Night.” Science of The Total Environment 904: 166701. https://doi.org/10.1016/j.scitotenv.2023.166701.
- Dani, M., P. Molnár, and A. Skribanek. 2021. “The Sensitivity of Herbaceous Plants to Light Pollution.” Acta Universitatis de Carolo Eszterházy Nominatae. Sectio Biologiae 46: 173–181. https://doi.org/10.33041/ActaUnivEszterhazyBiol.2021.46.173.
- Davies, T. W., J. P. Duffy, J. Bennie, and K. J. Gaston. 2016. “Stemming the Tide of Light Pollution Encroaching into Marine Protected Areas.” Conservation Letters 9 (3): 164–171. https://doi.org/10.1111/conl.12191.
- Deng, K., B. Zhu, Y. Zhou, Q. Chen, T. Wang, J. Wang, and J. Cui. 2019. “Mate Choice Decisions of Female Serrate-Legged Small Treefrogs Are Affected by Ambient Light Under Natural, but Not Enhanced Artificial Nocturnal Light Conditions.” Behavioural Processes 169: 103997. https://doi.org/10.1016/j.beproc.2019.103997.
- Desouhant, E., E. Gomes, N. Mondy, and I. Amat. 2019. “Mechanistic, Ecological, and Evolutionary Consequences of Artificial Light at Night for Insects: Review and Prospective.” Entomologia Experimentalis et Applicata 167 (1): 37–58. https://doi.org/10.1111/eea.12754.
- Dias, K. S., E. S. Dosso, A. S. Hall, A. P. Schuch, and A. M. Tozetti. 2019. “Ecological Light Pollution Affects Anuran Calling Season, Daily Calling Period, and Sensitivity to Light in Natural Brazilian Wetlands.” The Science of Nature 106 (46): 1–10. https://doi.org/10.1007/s00114-019-1640-y.
- Duriscoe, D., A. Sharolyn, L. Christian, and B. Kimberly. 2018. “A Simplified Model of All-Sky Artificial Sky Glow Derived from VIIRS Day/Night Band Data.” Journal of Quantitative Spectroscopy and Radiative Transfer 214: 133–145. https://doi.org/10.1016/j.jqsrt.2018.04.028.
- Elvidge, C. D., K. Baugh, M. Zhizhin, F. C. Hsu, and T. Ghosh. 2017. “VIIRS Night-Time Lights.” International Journal of Remote Sensing 38 (21): 5860–5879. https://doi.org/10.1080/01431161.2017.1342050.
- Falchi, F., P. Cinzano, D. Duriscoe, C. C. M. Kyba, C. D. Elvidge, K. Baugh, B. A. Portnov, N. A. Rybnikova, and R. Furgoni. 2016. “The New World Atlas of Artificial Night Sky Brightness.” Science Advances 2 (6): e1600377, 1–25. https://doi.org/10.1126/sciadv.1600377.
- Fiorentin, P., R. Binotto, S. Cavazzani, A. Bertolo, S. Ortolani, and I. Saviane. 2022. “Long-Time Trends in Night Sky Brightness and Ageing of SQM Radiometers.” Remote Sensing 14 (22): 5787. https://doi.org/10.3390/rs14225787.
- Gaston, K. J., S. Gaston, J. Bennie, and J. Hopkins. 2015. “Benefits and Costs of Artificial Nighttime Lighting of the Environment.” Environmental Reviews 23 (1): 14–23. https://doi.org/10.1139/er-2014-0041.
- Guetté, A., L. Godet, M. Juigner, and M. Robin. 2018. “Worldwide Increase in Artificial Light At Night Around Protected Areas and Within Biodiversity Hotspots.” Biological Conservation 223: 97–103. https://doi.org/10.1016/j.biocon.2018.04.018.
- Han, P., J. Huang, R. Li, L. Wang, Y. Hu, J. Wang, and W. Huang. 2014. “Monitoring Trends in Light Pollution in China Based on Nighttime Satellite Imagery.” Remote Sensing 6 (6): 5541–5558. https://doi.org/10.3390/rs6065541.
- Hu, Z., H. Hu, and Y. Huang. 2018. “Association Between Nighttime Artificial Light Pollution and Sea Turtle Nest Density Along Florida Coast: A Geospatial Study Using VIIRS Remote Sensing Data.” Environmental Pollution 239: 30–42. https://doi.org/10.1016/j.envpol.2018.04.021.
- Jiang, W., G. He, T. Long, C. Wang, Y. Ni, and R. Ma. 2017. “Assessing Light Pollution in China Based on Nighttime Light Imagery.” Remote Sensing 9 (2): 135. https://doi.org/10.3390/rs9020135.
- Kamrowski, R. L., C. Limpus, J. Moloney, and M. Hamann. 2012. “Coastal Light Pollution and Marine Turtles: Assessing the Magnitude of the Problem.” Endangered Species Research 19 (1): 85–98. https://doi.org/10.3354/esr00462.
- Kendall, M. G. 1975. Rank Correlation Methods. London: Griffin.
- Kohyama, J. 2009. “A Newly Proposed Disease Condition Produced by Light Exposure During Night: Asynchronization.” Brain and Development 31 (4): 255–273. https://doi.org/10.1016/j.braindev.2008.07.006.
- Kooijman, S., R. van den Berg, A. Ramkisoensing, M. R. Boon, E. N. Kuipers, M. Loef, T. C. M. Zonneveld, et al. 2015. “Prolonged Daily Light Exposure Increases Body Fat Mass Through Attenuation of Brown Adipose Tissue Activity.” Proceedings of the National Academy of Sciences 112 (21): 6748–6753. https://doi.org/10.1073/pnas.1504239112.
- Kumar, P., S. Rehman, H. Sajjad, B. R. Tripathy, M. Rani, and S. Singh. 2019. “Analyzing Trend in Artificial Light Pollution Pattern in India Using NTL Sensor’s Data.” Urban Climate 27: 272–283. https://doi.org/10.1016/j.uclim.2018.12.005.
- Kumari, R., V. Verma, N. Kronfeld-Schor, and M. Singaravel. 2021. “Differential Response of Diurnal and Nocturnal Mammals to Prolonged Altered Light-Dark Cycle: A Possible Role of Mood Associated Endocrine, Inflammatory and Antioxidant System.” Chronobiology International 38 (11): 1618–1630. https://doi.org/10.1080/07420528.2021.1937200.
- Kyba, C. C. M., YÖ Altıntaş, C. E. Walker, and M. Newhouse. 2023. “Citizen Scientists Report Global Rapid Reductions in the Visibility of Stars from 2011 to 2022.” Science 379 (6629): 265–268. https://doi.org/10.1126/science.abq7781.
- Kyba, C. C. M., and F. Hölker. 2013. “Do Artificially Illuminated Skies Affect Biodiversity in Nocturnal Landscapes?” Landscape Ecology 28 (9): 1637–1640. https://doi.org/10.1007/s10980-013-9936-3.
- Kyba, C. C. M., T. Kuester, A. S. de Miguel, K. Baugh, A. Jechow, F. Hölker, J. Bennie, C. D. Elvidge, K. J. Gaston, and L. Guanter. 2017. “Artificially Lit Surface of Earth at Night Increasing in Radiance and Extent.” Science Advances 3 (11): 1–8. https://doi.org/10.1126/sciadv.1701528.
- LaRoe, J., C. M. Holmes, and T. Schad. 2022. “Nightlight Intensity Change Surrounding Nature Reserves: A Case Study in Orbroicher Bruch Nature Reserve, Germany.” Remote Sensing 14 (16): 3876. https://doi.org/10.3390/rs14163876.
- Levin, N. 2017. “The Impact of Seasonal Changes on Observed Nighttime Brightness from 2014 to 2015 Monthly VIIRS DNB Composites.” Remote Sensing of Environment 193: 150–164. https://doi.org/10.1016/j.rse.2017.03.003.
- Liu, M., X. Liu, B. Zhang, Y. Li, T. Luo, and Q. Liu. 2022. “Analysis of the Evolution of Urban Nighttime Light Environment Based on Time Series.” Sustainable Cities and Society 78: 103660. https://doi.org/10.1016/j.scs.2021.103660.
- Liu, J., Z. Ouyang, S. L. Pimm, P. H. Raven, X. Wang, H. Miao, and N. Han. 2003. “Protecting China’s Biodiversity.” Science 300 (5623): 1240–1241. https://doi.org/10.1126/science.1078868.
- Lockett, M. T., R. Rasmussen, S. K. Arndt, G. R. Hopkins, and T. M. Jones. 2022. “Artificial Light at Night Promotes Bottom-up Changes in a Woodland Food Chain.” Environmental Pollution 310: 119803. https://doi.org/10.1016/j.envpol.2022.119803.
- Mann, H. B. 1945. “Nonparametric Tests Against Trend.” Econometrica 13 (3): 245–259. https://doi.org/10.2307/1907187.
- Navara, K. J., and R. J. Nelson. 2007. “The Dark Side of Light at Night: Physiological, Epidemiological, and Ecological Consequences.” Journal of Pineal Research 43 (3): 215–224. https://doi.org/10.1111/j.1600-079X.2007.00473.x.
- Qiu, S., S. Xi, C. Y. Cao, S. Uprety, and W. H. Wang. 2017. “Assessment of Straylight Correction Performance for the VIIRS Day/Night Band Using Dome-C and Greenland Under Lunar Illumination.” International Journal of Remote Sensing 38 (21): 5880–5898. https://doi.org/10.1080/01431161.2017.1338786.
- Raap, T., R. Pinxten, and E. Marcel. 2015. “Light Pollution Disrupts Sleep in Free-Living Animals.” Scientific Reports 5 (1): 13557. https://doi.org/10.1038/srep13557.
- Sánchez de Miguel, A., J. Bennie, E. Rosenfeld, S. Dzurjak, and K. J. Gaston. 2022. “Environmental Risks from Artificial Nighttime Lighting Widespread and Increasing Across Europe.” Science Advances 8 (37): 1–9. https://doi.org/10.1126/sciadv.abl6891.
- Sangma, J. T., and A. K. Trivedi. 2023. “Light at Night: Effect on the Daily Clock, Learning, Memory, Cognition, and Expression of Transcripts in Different Brain Regions of Rat.” Photochemical & Photobiological Sciences 22 (10): 2297–2314. https://doi.org/10.1007/s43630-023-00451-z.
- Sen, P. K. 1968. “Robustness of Some Nonparametric Procedures in Linear Models.” The Annals of Mathematical Statistics 39 (6): 1913–1922. https://doi.org/10.1214/aoms/1177698021.
- Soulé, M. E., and D. Simberloff. 1986. “What Do Genetics and Ecology Tell Us About the Design of Nature Reserves?” Biological Conservation 35 (1): 19–40. https://doi.org/10.1016/0006-3207(86)90025-X.
- Stevens, R. G. 2009. “Light-at-Night, Circadian Disruption and Breast Cancer: Assessment of Existing Evidence.” International Journal of Epidemiology 38 (4): 963–970. https://doi.org/10.1093/ije/dyp178.
- Sun, S., W. Sang, and J. C. Axmacher. 2020. “China’s National Nature Reserve Network Shows Great Imbalances in Conserving the Country’s Mega-Diverse Vegetation.” Science of The Total Environment 717: 137159, 1–8. https://doi.org/10.1016/j.scitotenv.2020.137159.
- Theil, H. 1992. “A Rank-Invariant Method of Linear and Polynomial Regression Analysis.” In Henri Theil’s Contributions to Economics and Econometrics, edited by Baldev Raj and Johan Koerts, Vol. 23, 345–381. Advanced Studies in Theoretical and Applied Econometrics. Dordrecht: Springer Netherlands. https://doi.org/10.1007/978-94-011-2546-8_20.
- Venter, O., E. W. Sanderson, A. Magrach, J. R. Allan, J. Beher, K. R. Jones, H. P. Possingham, et al. 2016. “Sixteen Years of Change in the Global Terrestrial Human Footprint and Implications for Biodiversity Conservation.” Nature Communications 7 (1): 12558. https://doi.org/10.1038/ncomms12558.
- Viera-Pérez, M., L. Hernández-Calvento, P. A. Hesp, and A. Santana-del Pino. 2019. “Effects of Artificial Light on Flowering of Foredune Vegetation.” Ecology 100 (5): 1–10. https://doi.org/10.1002/ecy.2678.
- Wang, Y., C. Lv, X. Pan, Z. Liu, P. Xia, C. Zhang, and Z. Liu. 2022. “Spatiotemporal Patterns of Light Pollution on the Tibetan Plateau over Three Decades at Multiple Scales: Implications for Conservation of Natural Habitats.” Remote Sensing 14 (22): 5755. https://doi.org/10.3390/rs14225755.
- Xiang, W., and M. Tan. 2017. “Changes in Light Pollution and the Causing Factors in China’s Protected Areas, 1992–2012.” Remote Sensing 9 (10): 1026–1031. https://doi.org/10.3390/rs9101026.
- Xu, Y., A. Knudby, and C. Côté-Lussier. 2018. “Mapping Ambient Light at Night Using Field Observations and High-Resolution Remote Sensing Imagery for Studies of Urban Environments.” Building and Environment 145: 104–114. https://doi.org/10.1016/j.buildenv.2018.09.002.
- Yu, C., Y. Gao, C. Wang, T. Chen, J. Wang, and Q. Lu. 2022. “‘Targets-Plans-Decision’: A Framework to Identify the Size of Protected Areas Based on the Balance of Ecological Protection and Economic Development.” Journal of Environmental Management 304: 114302. https://doi.org/10.1016/j.jenvman.2021.114302.
- Zheng, Z., Z. Wu, Y. Chen, G. Guo, Z. Cao, Z. Yang, and F. Marinello. 2021. “Africa’s Protected Areas Are Brightening at Night: A Long-Term Light Pollution Monitor Based on Nighttime Light Imagery.” Global Environmental Change 69: 102318. https://doi.org/10.1016/j.gloenvcha.2021.102318.