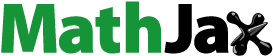
ABSTRACT
China’s massive urbanisation development will undoubtedly serve as a global reference. Due to the uncertainty of statistical data, nighttime light (NTL) data have emerged as alternative data for urbanisation evaluation. Here, the application of the Prolonged Artificial NTL Dataset of China (1984–2020) has been newly expanded. Total-value statistical, complex NTL index (CNLI), NTL concentration degree, rank-size rule, and Markov transfer matrix were used to systematically mine information about urban change from multiple perspectives. China’s urbanisation exhibited rapid growth and outwards expansion. The total percentage increase in NTL brightness and NTL area was 409.38% and 302.58%, respectively. The Yangtze River Delta urban agglomeration of East China had the fastest urbanisation (CNLI Trend = 0.0057/a, p < 0.01). Low NTL areas transitioned to medium NTL areas, and high and extremely-high NTL areas diffused to medium NTL areas. The cities in Eastern China and Southern China typically exhibited extremely-high type NTL areas, whereas other cities primarily exhibited medium NTL areas. The gaps between city sizes decreased over time (q Trend = – 0.0200/a, p < 0.01). Lowest-ranked cities exhibited the highest stability (>95%) in city size type transition. The spatiotemporal changes in NTL obtained were of great significance for monitoring urban expansion patterns, making government decisions, and quantifying China’s sustainable development.
1. Introduction
Urbanisation, which represents economic development, is the dominant form of economic development in developing countries (Montgomery Citation2008). Since 1992, China has entered a stage of comprehensive promotion of urbanisation (Fu et al. Citation2017). The speed and scale of China’s urbanisation have been unprecedented, and China’s urbanisation has undoubtedly been a major driver of global urbanisation (Chen et al. Citation2019). Urbanisation has resulted in social problems, such as air pollution, resource depletion, and environmental vulnerability, posing a major challenge to sustainable development (Dutt et al. Citation2004). Studying the spatiotemporal evolution pattern of its urbanisation in China can help to elucidate its urban development and predict future trends in urbanisation. Further, it can help in regulating the direction of urban development in terms of economic policy, land-use planning, and industry share. This is of great significance for promoting high-quality and sustainable economic development in China (Jiang et al. Citation2020; Meisner Citation2020). Monitoring these changes is closely pertinent to UN Sustainable Development Goals (SDGs), especially Goal 11 (making cities and human settlements inclusive, safe, resilient, and sustainable).
For quantitative analysis and modelling of the increasingly complex issues of urbanisation, the primary data sources are statistical data on population and socioeconomic factors and remote-sensing data (based mainly on visible light and near-infrared monitoring). Population and economic statistical data exhibit uncertainty (due to factors including difficulties in statistical standardisation, long updating cycles, and a lack of fine-scale spatial features, due to administrative boundaries). Although data such as land use/cover change (LUCC) can be extracted based on remote-sensing monitoring methods to analyse the dynamics of urbanisation (He et al. Citation2022), they are less useful for analysing large-scale urban systems and their spatial structure, and it is not possible to obtain information on the intensity of socio-economic activities related to urbanisation from such data (Yu et al. Citation2021). However, nighttime-light remote sensing, an optical remote-sensing technique capable of detecting nighttime shimmer, is gradually being widely applied in the study of urbanisation (Chen et al. Citation2019). There are two key reasons for this trend. First, remote-sensing nighttime light (NTL) data are significantly correlated with various city scale assessment indexes (that quantify aspects such as the city boundary, population, and economy). This correlation is usually monotonic and robust in time and space (Henderson et al. Citation2003; Levin and Duke Citation2012). Second, the advantages of large coverage, long-time series, rapid updating, and ease of accessibility make NTL remote-sensing images a simple but rich index for assessing the hierarchical structure of urban systems and spatial patterns, such data can be used in multi-scale spatiotemporal urbanisation research (Chen et al. Citation2019). Thus, unlike socioeconomic statistics and remote-sensing data based on surface texture features (such as LUCC data), NTL data, a spatially explicit and comprehensive index with distinct spatial features and high correlation with urban socioeconomic activity intensity, can provide different perspectives for quantitatively evaluating and understanding the spatiotemporal characteristics of urban development. In recent years, with the continuous improvement in the spatial, temporal, and spectral resolution of night-time light satellites, studies on Chinese urbanisation based on NTL remote-sensing data have entered a rapid development stage.
Most NTL-based urbanisation studies have used Defense Meteorological Satellite Program/Operational Line Scanner (DMSP/OLS) NTL data (Gao et al. Citation2021; Wei et al. Citation2019; Xu and Hu Citation2021; Xu, Lin, and Jin Citation2021) for 1992–2013 and National Polar-orbiting Partnership / Visible Infrared Imaging Radiometer Suite (NPP/VIIRS) NTL data (Ding et al. Citation2022; Luo and Li Citation2019; Ma et al. Citation2020; Pan and Hu Citation2018; Xu, Li, and Chen Citation2018) for 2012–2020. Some studies have also integrated DMSP/OLS and NPP/VIIRS data, with an integrated timeframe of 1992–2018 (Ma et al. Citation2020; You et al. Citation2021), the latest updated timeframe is 1992–2020 (Yuan et al. Citation2022). However, a standardised dataset for mutual correction of those two data types has not yet been published. These NTL datasets cover a short time span and are out-of-date, and therefore cannot be used to effectively analyse the long-term evolution of urban development in China using the latest data. Therefore, this study used the Prolonged Artificial Nighttime-light DAtaset of China (PANDA) for analysis. The PANDA dataset provided in 2021 by the National Tibetan Plateau / Third Pole Environment Data Centre was expands and optimises the integrated DMSP/OLS and NPP/VIIRS datasets, spanning the period 1984–2020 (37 years) (Zhang et al. Citation2021). This optimised, high-quality dataset can effectively capture the temporal trends of newly constructed areas. The PANDA dataset exhibits better correlations with socioeconomic indicators (surface area of built-up, GDP, and population) than all other products, indicating that it has better potential in finding NTL changes at different stages (Zhang et al. Citation2021).
Most NTL-based urbanisation studies have examined dynamic changes at a single spatial level, such as administrative division (continent, country, province, and city) or watershed. In recent decades, studies of urbanisation based on administrative division, which have focused on comparisons between continents, countries, provinces, or cities, have provided valuable insight into the characteristics of urban expansion in administrative divisions (Gong et al. Citation2020; He et al. Citation2019; Luo and Li Citation2019; Xu, Lin, and Jin Citation2021; Xu, Zang, and Na Citation2020). Studies of urbanisation based on watersheds have focused on comparing urban expansion characteristics between inner and outer watersheds, providing benchmarks and proxies for measuring human activities and their environmental impacts at the watershed scale (Huang et al. Citation2021). However, few studies have analysed urbanisation at the level of the country and its various administrative regions, or have addressed urban agglomerations of economic development from multiple perspectives. Layered analysis can help researchers to scientifically understand the overall urbanisation development trend in a country, examine the characteristics of development in each administrative region, examine how the urbanisation development differ between regions and the country as a whole, and elucidate the spatiotemporal evolution and heterogeneity of each urban agglomeration. Multilevel evaluation and analysis can provide a scientific basis for a comprehensive understanding of the dynamic evolution of urbanisation in a country and systematic study of urban changes.
There are numerous methods for studying urbanisation using NTL data. Classification of NTL brightness type is usually achieved via empirical thresholding (ET) (Elvidge et al. Citation1997; Sutton Citation1997), local optimisation thresholding (LOT) (Liu et al. Citation2012; Milesi et al. Citation2003), and brightness gradient-based thresholding (BG) (Jiang et al. Citation2020; Kamarajugedda, Mandapaka, and Lo Citation2017). Among these methods, threshold techniques using auxiliary data have been widely used owing to their simplicity, relatively high accuracy, and reliability. Total-value statistical analysis involves the study the dynamic evolution regularity of urbanisation in terms of urban expansion area, speed, and trends (Connolly Citation2018; Ma et al. Citation2012). The complex nighttime light index (CNLI) can reflect the overall urbanisation level of a region by taking into account both NTL brightness and urban NTL areas (Gao et al. Citation2015; Liu et al. Citation2012; Zheng et al. Citation2020; Zheng et al. Citation2021). Based on NTL concentration degree (i.e. the proportion of NTL per NTL type per region per year), regional urbanisation patterns can be comprehensively analysed and summarised in terms of in the distribution of NTL types (Shen Citation2020). The rank-size rule can be used to evaluate the concentration, dispersion, and balance of urban scale distribution within a region (Ding et al. Citation2022; Gao et al. Citation2015; Shaban, Kourtit, and Nijkamp Citation2020; Xu, Li, and Chen Citation2018). A Markov transfer matrix (MTM) can be used to study the type transition of different city sizes throughout the entire study period (Pu et al. Citation2009; Xu and Hu Citation2021). These methods have been widely applied in the NTL-based study of urbanisation. Nonetheless, most previous studies on urbanisation have used one or two methods for analysis and research. This study attempts to apply the research methods described earlier to comprehensively analyse China’s urbanisation. In addition, This study uses pixel-level evaluation method for NTL-based visualisation of urbanisation. Other indices, including the allometric growth model (Cheng et al. Citation2019) and the Hurst index (Xu, Lin, and Jin Citation2021), can effectively calculate urbanisation. In future, we will use these indices to study urbanisation.
The pressing need for sustainable development of cities and human settlements has made it crucial to monitor changes in urbanisation expansion patterns (Zheng et al. Citation2023). To address this, this study used China as the study area and classified PANDA NTL types via threshold techniques. Considering the multiscale aspects of China and its seven geo-administrative regions and five typical urban agglomerations, this study applied this study applied methods such as Total-value statistical analysis, CNLI, NTL concentration degree, the rank-size rule, and MTM to study trends in NTL in China from 1984 to 2020. The aim was to reveal the association between NTL and urbanisation in China, and to analyse its patterns of urban development over the past 37-year period. This study is of great practical significance because it provides a comprehensive understanding of the level of development and sustainability of urbanisation in China, and was a theoretical reference for the formulation of policies for coordinated regional development in China.
2. Study area and data sources
2.1. Study area
China is located in the eastern Asia on the west coast of the Pacific Ocean, with a land area of approximately 9.6 million km2 with provincial administrative divisions comprising four municipalities directly under the central government, 23 provinces, five administrative autonomous regions, and two special administrative regions (Xu, Lin, and Jin Citation2021) (). Based on multiple factors such as its natural geography, history, and ethnicity, China is divided into seven major geo-administrative regions: Northeast, North, Easta, South, Centra, Southwest, and Northwest. Northeast China includes Liaoning, Heilongjiang, and Jilin (provinces); North China includes Beijing and Tianjin (cities), Shanxi, Inner Mongolia, and Hebei (provinces); East China includes Shandong, Anhui, Jiangsu, Jiangxi, Zhejiang, Fujian, and Taiwan (provinces), Shanghai (city); South China includes Guangdong, Guangxi and Hainan (provinces), Hong Kong and Macao (special administrative regions); Central China includes Henan, Hubei, and Hunan (provinces); Southwest China includes Tibet, Sichuan, Guizhou and Yunnan (provinces), and Chongqing (city); and Northwest China includes: Ningxia, Qinghai, Gansu, Shaanxi, and Xinjiang (provinces) (Li et al. Citation2021; Zhou et al. Citation2014) ().
Since the twenty-first century, a group of urban agglomerations has rapidly emerged, represented by the Yangtze River Delta (YRD), the Pearl River Delta (PRD), and the Middle Reaches of the Yangtze River (MRYR). The Outline of the 14th Five Year Plan (2021–2025) indicates that it is necessary to develop and strengthen urban agglomerations and metropolitan areas, promote their integrated development, and to comprehensively develop ‘two horizontal and three vertical’ strategic urbanisation pattern. Based on the three original representative agglomerations, the Bohai Rim (BR) and the Chengdu-Chongqing urban agglomeration (CC) were added (Chen, Liu, and Sun Citation2016; Li and Liu Citation2020) ().
Table 1. Cities included in China's five major urban agglomerations.
2.2. Data sources
2.2.1. NTL dataset
PANDA (1984–2020) was calculated by Zhang et al. (Citation2021) at Tsinghua University in 2021 using a NTL convolution long short-term memory neural network (ConvLSTM), and was published by the National Tibetan Plateau Data Centre (http://data.tpdc.ac.cn/; accessed on 21 October 2021), with a 30 arcsec (approximately 1 km) spatial resolution. The ConvLSTM neural network was used to generate the PANDA dataset. The calculations were performed in integers by enlarging the digital number (DN) of the NTL data from DMSP/OLS and NPP/VIIRS after mutual calibration by 100 times in order to ensure the calculation accuracy and efficiency. Therefore, The DN range of PADNA data released by the official data centre was zero to 6300 (Zhang et al. Citation2021), which is a dimensionless quantity. Model evaluation comparing this dataset and the original image reveals an RMSE was 0.73, a coefficient of determination (R2) of 0.95, and the pixel slope of 0.99, indicating that the data quality of the generated product was high (Zhang et al. Citation2021). Socioeconomic indicators, such as the area of built-up, GDP, population size, correlate better with the PANDA dataset than with other products, suggesting that the PANDA has a better potential to find different NTL changes at different stages (Zhang et al. Citation2021). Multiple data sources – the impervious surface data (1991–2020, Remote Sensing Centre of Wuhan University), total population value, and GDP statistics (1984–2020; National Bureau of Statistics of China on China Statistical Yearbook) – were used to verify the reliability of PANDA data (Supplementary Figure A1–2).
2.2.2. Administrative division data
Administrative division data were obtained from geographic information system data (scale, 1:1 million), based on 2021, from the National Basic Geographic Information Centre (https://ngcc.cn/ngcc/; accessed on 20 September 2023).
2.2.3. Land cover dataset
Land cover dataset was obtained from the Remote Sensing Centre of Wuhan University in China, published by the Zenodo database in Europe (https://zenodo.org/records/8176941/; accessed on 23 March 2023). This dataset was processed uniformly using GEE on Landsat remote sensing images, with a spatial resolution of 30 m, the temporal resolution of 1 year, and the time range from 1991 to 2020. Land cover types include nine types: cropland, forest, shrubs, grasslands, water, snow/ice, wasteland, impervious surface (Yang and Huang Citation2021).
2.2.4. Population dataset
The population dataset was obtained from the NASA’s Socioeconomic Data and Applications Centre (SEDAC) (https://www.worldpop.org/; accessed on 5 March 2022; unit, people per square kilometre; spatial resolution, 1 km), and included annual data from 2000 to 2020.
2.2.5. Gross national product (GDP) dataset
The gross national product (GDP) dataset was obtained from the Geographic Remote Sensing Ecological Network Platform (https://www.gisrs.cn/; accessed 23 December 2023; unit, million yuan per square kilometre; spatial resolution, 1 km), and included seven periods: 1990, 1995, 2000, 2005, 2010, 2015, and 2020.
2.2.6. China statistical yearbook data
Statistical yearbook data were obtained from the National Bureau of Statistics of China (https://www.stats.gov.cn/sj/ndsj/; accessed on 23 January 2024). Population and GDP data from the China Statistical Yearbook data (1984–2020) were used for data cross-validation.
3. Methods
NTL data is spatially and temporally explicit with respect to the magnitude of socioeconomic activity related to urban development; it therefore provides a new means for investigating urban patterns and urbanisation processes. This NTL-based study examines urbanisation mainly in three respects. First, NTL was classified by type according to brightness gradient. The total-value statistics method and NTL concentration degree analysis were used to dynamically study the proportions and change characteristics between the types, in order to reveal the regularity of urbanisation development. Second, based on the impervious surface (from the Land cover data), the urban area was extracted via threshold segmentation of the NTL, together with the CNLI (which can simultaneously consider both NTL brightness [NTLB] and NTL area [NTLA]), were used to reflect long-term changes in urbanisation. Third, in different administrative regions, the rank-size rule method was used to analyse the distribution and evolution of city size in each region, and the MTM was used to analyse type transitions in different city sizes throughout the research period. This study is crucial for gaining a deeper understanding of China’s urbanisation over the past few decades.
illustrates the technical procedure used in this study. This involved fives steps. First, PANDA DN values were corrected by dividing them by 100, so that the NTL values range from zero to 63. Second, using the Jenks natural breaks method (Economic and Social Research Institude Citation2007), the NTL data were classified into four types: low, medium, high, and extremely-high, and NTL classification images were obtained. Third, the NTL data were extracted: Using provincial administrative vector boundary data, mask data were extracted for the seven geo-administrative regions, and using municipal administrative district vector boundary data, mask data were extracted for the five major urban agglomerations. Based on these mask data, mask extraction was performed on the PANDA dataset to obtain seven datasets: (i) The ‘NTL dataset of China’ (1984–2020); (ii) The ‘NTL Classification dataset of China’ (1984–2020); (iii) The ‘NTL dataset of the Seven Geo-administrative Regions’ (1984–2020); (iv) ‘NTL Classification dataset of Seven Geo-administrative Regions’ (1984–2020); (v) The ‘NTL dataset of five urban agglomerations’ (1984–2020); (vi) The ‘NTL Classification dataset of five urban agglomerations’ (1984–2020); and (vii) The ‘NTL dataset of 370 Cities in China’ (1984–2020). Fourth, NTLB and NTLA were calculated for each dataset via the total-value statistics method, NTL concentration degree analysis, and CNLI. Urban development scales were analysed using the rank-size rule and MTM analysis. Finally, each result was visualised and analysed.
3.1. Relationship between NTL classification and urbanisation
Jenks natural breaks method was specifically developed for the use in analysis of geographic data, and has emerged as a standard geographic classification algorithm (Economic and Social Research Institude Citation2007). The classification is achieved by maximise the variance between classes mean and minimise the variance within classes mean (Jenks Citation1967). Based on the similarity between auxiliary data indexes such as population size, GDP, and LUCC data and NTL data in terms of changes in spatial distribution, Jenks natural-breaks method was used to classify the average NTL DN values for 1984–2020 as low (0, 4); medium [4, 16); high [16, 37); and extremely-high [37, 63]. A unified classification standard was used to classify the 37 years of PANDA data. Unified standardised classification can avoid the problem of the same NTL values in different areas being classified as different NTL types, and increases the comparability of NTL types in different cities. Beijing, the Chinese capital, is used as an example. Considering the accessibility of different long-term socioeconomic variables, spatial distribution maps of population density, GDP, and Land cover data for 2000, 2005, 2010, 2015, and 2020 were compared with the results of NTL classification for the same period () to illustrate the relationships between NTL classification images and urbanisation and to conduct verification.
Figure 3. Comparative verification of different data types for Beijing (2000–2020). a. Population density; b. GDP; c. Land cover; d. Nighttime light type.

Comparative analysis of the different datasets revealed that areas without NTL and areas with low NTL were generally underdeveloped mountainous areas, with a population density of approximately 0–200 people/km2 and GDP density of approximately 0–17 million/km2, and the Land cover types were mostly forest and grassland; those with medium NTL were generally rural with some development, with a population density of mostly 200–825 people/km2 and GDP density of 0–70 million/km2, and the land cover types were mostly interlaced agricultural and impervious surface; areas with high NTL were generally better developed areas around towns and cities, with a population density of mostly 825–2340 people / km2 and GDP of 17–400 million / km2, and the land cover types mostly built-up areas and areas of intersecting agricultural and impervious surface; areas with extremely-high NTL were generally the most developed urban centre areas, with a population density of mostly 2340–86,000 people/km2 and GDP of 400–14,000 million/km2, and the Land cover types were mostly built-up areas.
Based on the different types (low, medium, high, and extremely-high) of NTL during the study period, the corresponding population and GDP data were extracted. Correlations between NTL type and population and GDP data were analysed. The coefficients of the correlations between low NTL and population and GDP were 0.85 and 0.74; between medium NTL and population and GDP, 0.79 and 0.64; between high NTL and population and GDP, 0.81 and 0.67; and between extremely-high NTL and population and GDP, 0.87 and 0.84, respectively. These correlations were significant at the 90% significance level. The impervious surface area of land cover data were compared with those exhibiting extremely-high NTL: the difference between the percentage of these areas was ≤2.76%. Using Jenks natural breaks method and the unified standard, the four types of NTL were classified and long-term spatiotemporal changes in each type were calculated. In this way, the NTL types were compared, providing a detailed description of the dynamic changes in urbanisation.
3.2. Total-value statistical analysis
NTL values were obtained for each pixel of study area, and the total NTL values were calculated separately for each year in each area for 1984–2020, as follows (Burrough Citation2001):
(1)
(1) where n is the total number of pixels in the calculated region and xi is the DN value of the ith pixel in the region.
Based on the results of Equation (1), growth K and growth rate k of NTL were calculated, as follows:
(2)
(2)
(3)
(3) where Lm is the total value of NTL in a region in year m and Lm – 1 is the total value of NTL in the same region in year m – 1.
3.3. CNLI
CNLI is a novel indicator for measuring urbanisation development. CNLI is the product of the average relative NTL intensity in the region after noise removal and the proportional NTLA in that region (Zheng et al. Citation2020), as follows (Gao et al. Citation2015):
(4)
(4)
(5)
(5)
(6)
(6) where I is the average relative NTL intensity; S is the proportional NTLA; DNi is the DN of pixel i in the region; DNm is the maximum DN of pixel i in the region (DNm is 63 in this study); P is the threshold of DN after noise removal via threshold technique (Liu et al. Citation2012), which was used to determine the optimal threshold value for identifying urban NTL area in different regions (Supplementary Table A1). Considering China’s vast size and the substantial diversity of its geographical environment and economic development, for each of the seven major geo-administrative regions in China, the optimal thresholds were determined for each period in which the urban areas extracted using NTL data best matched the ancillary data (Gao et al. Citation2015); in this calculation, Ni is the number of pixels with DN of i in the region; N is the total number of pixels in the region; and NL is the number of pixels in the region with a non-zero DN.
3.4. Ntl concentration degree
The concentration of urbanisation is expressed as the proportion of the urban population to the total population, with a higher proportion leading to a higher concentration. In this study, NTL was used as a proxy for urban population data, and the NTL concentration degree (i.e. the proportion of NTL per NTL type per region per year) was calculated.
(Shen Citation2020), as follows:
(7)
(7) where zi is the NTL concentration degree with a certain type in the region in year i,
is the total of NTL with a certain type in region j within the region in year i, and
is the total of NTL in the region in year i. Urbanisation was calculated based on population data, to determine the proportion of the urban population in each city relative to the total population. It is difficult to accurately determine the threshold for urban NTL when calculating urbanisation using NTL data. The NTL concentration degrees of different types were analysed by calculating the percentage of NTL at different types in a certain region to the total amount of NTL. The high type NTL concentration indicated a high urbanisation development and rapid urbanisation process in the region; conversely, low type NTL concentration indicated a relatively low level of urbanisation, with the majority of people still living in rural or relatively underdeveloped areas. In terms of time, by comparing the changes in the concentration degree of different NTL types in a certain region, the dynamic changes in urbanisation development in that region could be obtained. In terms of space, by comparing the differences in the NTL concentration degree between regions, the urbanisation development characteristics of each region can be identified. Therefore, the different types of NTL concentration degree could be used as one of the indexes to measure the urbanisation development process.
3.5. The rank-size rule
The ‘rank-size’ rule examines the size distribution of an urban system based on the relationship between city size and rank. Many studies within and across borders have shown that the rank-size rule is the most comprehensive in portraying the size distribution of cities. Here, the urban NTL data were used instead of population data to examine the size distribution of the urban system (Ding et al. Citation2022; Xu, Li, and Chen Citation2018), as follows (Kundak and Dkmeci Citation2018; Shaban, Kourtit, and Nijkamp Citation2020):
(8)
(8) Following logarithmic transformation,
(9)
(9) where Ri is the rank of the i-th city of the region; K is a constant; Pi is the NTL size of the ith city of the region in descending rank of city size; and q is the Zipf index, which reflects the overall characteristics of the urban distribution. If q = 1, it indicates that the urban distribution in the region is optimal, and that the number and proportion of cities at different classes in the region are the most reasonable; this relationship is known as Zipf’s law distribution; If 0 < q < 1, it indicates that the city size distribution is relatively dispersed, with a lower number of large-sized cities and a higher number of small and medium-sized cities with relatively good development (known as a second-place distribution); If q > 1, it indicates that the city size distribution is more centralised, with the large-size cities grow faster than small-size cities, and that the influence of the largest city is greater, (known as a first-place distribution).
For each geo-administrative region, linear regression was performed using year as the independent variable and q value as the dependent variable, as follows:
(10)
(10) where t is the year; a and b are regression coefficients. If a > 0, it means that the urban development trend in the region during the period 1984–2020 is biased in favour of large-sized cities; if a < 0, it means that small- and medium-sized cities in the region are growing faster and are more concentrated; and if a = 0, large-, medium-, and small-sized cities in the region are growing at the same rate.
3.6. MTM analysis
MTM analysis can be used to study the long-term dynamics of cities of different sizes throughout the study period, and has been widely used to study the evolution of urban population size, land area, and GDP. The total NTL effectively characterises city size. This paper proposes combining MTM analysis with total NTL analysis to study the mechanisms of city-size evolution. Based on the proportion of the average of total NTLB in all cities in every year, cities were classified into the following four types (Pu et al. Citation2009; Xu and Hu Citation2021): Type I, with total NTLB < 50% of the average of NTLB of all cities; Type II, where total NTLB was 50–100% of the average total NTLB of all cities; Type III, where total NTLB was 100–200% of the average total NTLB of all cities; and Type IV, with total NTLB > 200% of the average total NTLB of all cities, as follows (Pu et al. Citation2009; Xu and Hu Citation2021):
(11)
(11)
(12)
(12) where Rj,t is the type of jth city in year t; Mt is the average total NTLB for all cities in year t; N is the number of cities; and Lj,t is the total NTLB for the jth city in year t.
There are 16 types of transfers between different size classes: (Ⅰ, Ⅱ, Ⅲ, Ⅳ): Ⅰ→Ⅰ, Ⅰ→Ⅱ, Ⅰ→Ⅲ, Ⅰ→Ⅳ, →Ⅰ, Ⅱ→Ⅱ, Ⅱ→Ⅲ, Ⅱ→Ⅳ, Ⅲ→Ⅰ, Ⅲ→Ⅱ, Ⅲ→Ⅲ, Ⅲ→Ⅳ, Ⅳ→Ⅰ, Ⅳ→Ⅱ, Ⅳ→Ⅲ, and Ⅳ→Ⅳ. First, the number of transitions from year t to year t + 1 (t = 1984, 1985, 1986, … , 2019) was calculated for each city. The total number of transfers, and the probability of each type of transfer from 1984 to 2020, were counted for the 370 cities nationwide. Finally, the MTM for the size evolution of the 370 cities was obtained.
Similarly, using a time interval of six years, the total number and probability of each transfer type from year t to year t + 6 (t = 1984, 1990, 1996, … , 2014) were calculated for the 370 cities, generating an MTM for the city-size evolution of six sets of 370 cities.
4. Results and analyses
4.1. Statistical analysis of NTL data
Based on the PANDA NTL dataset (1984–2020), changes in total NTLB and NTLA were analysed, revealing the urbanisation patterns of China, its seven major geo-administrative regions, and its five major urban agglomerations over the 37-year period.
4.1.1. Analysis of NTL in China
Trends in total NTLB and NTLA, with absolute growth and growth rate, are shown in .
NTLB in China exhibited an increasing trend, with a growth rate of 79.61 × 104/a (p < 0.01), and total percentage increase of 409.38% (growth of 2604.66 × 104) (a–c). The 37-year time-series can be divided into three periods. The first is 1984–2003, during which China’s NTLB development fluctuated and increased slowly, with a growth of 210.91 × 104 (growth rate of 63.24 × 104/a; p < 0.01). The second is 2003–2012, during which there were two peaks, first in 2003–2004, when total NTLB percentage increased by 17.84% (followed by a 3.43% decrease in 2004–2005), and the second in 2010–2011, when total NTLB percentage increased by 11.74% (followed by a 8.30% decrease in 2011–2012). During these two peaks, total NTLB first increased and then decreased, with no significant growth. In the third period, 2012–2020, total NTLB first increased and then decreased, with a slight overall increase. After a significant 17.74% percentage increase from 2018 to 2019, there was a significant 7.98% percentage decrease from 2019 to 2020, with a clear peak in 2019. Compared to the period before 2000, urbanisation development was cyclical after 2000, exhibiting an ‘increase–decrease–steady increase’ pattern.
NTLA in China had also exhibited a stable increase over the 37-year period, growing at 6.73 × 104 km2/a (p < 0.01; total percentage increase, 302.58%, i.e. 246.34 × 104 km2 (d–f). NTLA and NTLB exhibited similar trends, such as a significant decrease in 1992, 2009, and 2017 and a significant increase in 2020, 2004, 2010, and 2019. The Pearson correlation coefficient between NTLA and NTLB was 0.98 (p < 0.01), indicating when NTLB was increasing, there was relatively strong urban development. Simultaneously, the urban area expanded and human nighttime activities expanded, causing an increase in NTLA.
The trends in each type of NTLB and NTLA, along with absolute growth and growth rate, are shown in .
Medium, high, and extremely-high type NTLB all increased, whereas low type NTLB first increased and then decreased (a–c). The percentage increase in low type NTLB was 116.17 × 104 (a 153.82% increase, reflecting growth of 2.58 × 104/a; p < 0.05). The increase in medium type NTLB was slow and fluctuating until 2003, after which it accelerated, with an increase of 1291.13 × 104 (227.34%), and the increase in medium type NTLB was largest over the 37-year period. High and extremely-high type NTLB exhibited little change before 2000, then increased at an accelerating rate, with extremely-high type NTLB increasing faster. The increases in high and extremely-high types NTLB were 425.16 × 104 (156.37%) and 772.19 × 104 (225.93%), respectively.
There were significant differences in the trends of the various NTLA and NTLB types (d–f). China has a vast territory, and not all areas have achieved good development. Most of China exhibits no NTLB or low NTLB. Low type NTLA increased slowly in an ‘increase–decrease–increase’ pattern, and was overtaken in 2006 by the rapidly increasing medium NTLA, while high and extremely-high type NTLA increased slowly. Over the 37-year period, China’s urban NTLA has developed according to a model in which development spreads from wealthy areas into other areas. The development of higher type NTLA promoted the transition of the surrounding lower NTL areas into higher NTL areas. Influenced by the development of built-up areas, the total increases in high and extremely-high types NTLA were 16.74 × 104 km2 and 15.89 × 104 km2, respectively, less than those of medium and low types NTLA. The medium type NTLA developed mainly via development of the low type NTL area and diffusion of the high and extremely-high types NTL areas, exhibiting the largest increase in NTLA (168.97.74 × 104 km2; 262.02%). Although the low type NTLA was second only to the medium type NTLA, at 44.74 × 104 km2, its rate and percentage increase were lowest, owing to its natural environment and geographic location.
4.1.2. Analysis of NTL in the seven major geo-administrative regions of China
Supplementary Figure A3 presents changes in the total NTLB and NTLA in the seven major geographical administrative regions, while shows the spatiotemporal variation in NTLA in these regions.
Figure 6. Spatiotemporal variation in the nighttime light area in the seven major geographical administrative regions, 1984–2020.

The trends in NTLB in the seven major geo-administrative regions and in China overall were similar, fluctuating and increasing (Supplementary Figure A3). The percentage increases in Northwest and Southwest China were relatively high, at 1202.57% and 720.87%, respectively, although the increase was less than 300 × 104. The increase in East China was the largest, this was followed by the increases in North, Northeast, Central, South, Northwest, and Southwest China.
NTLA increased gradually in the seven geo-administrative regions (Supplementary Figure A3; ). As of 2020, the NTLA in East China still ranked first, followed by that of Northeast China, North China, Northwest China, Central China, Southwest China, and South China. The NTLA of East China increased fastest, at 49.80 × 104 km2/a. NTLA percentage increased the most in Southwest China (by 956%).
Supplementary Figure A4 presents changes in each type of NTLB and NTLA in the seven major geographical administrative regions of China, while shows the spatiotemporal transitions in NTL type in these regions.
Figure 7. Spatiotemporal transition of nighttime light type in the seven major geographical administrative regions, 1984–2020.

The trends in the various types of NTLB in the seven major geo-administrative regions and in China overall were similar, although the characteristics of each type of NTLB is different (Supplementary Figure A4). The extremely-high type NTLB in East China exhibits a significant increasing trend from 2001 onward, and the development of extremely-high type NTLB was extremely rapid. The trends in the various NTLB types in North China are consistent with those in China overall, but there was no significant difference between the trends in high and extremely-high types NTLB in this region. In Northeast, Central, Northwest, and Southwest China, NTLB in medium type was much higher than that in the low, high, and extremely-high types. The NTLB of low, high, and extremely-high types increased relatively slowly in those regions. In South China, NTLB in extremely-high type was highest, followed by the medium, high, and low types, with significant differences among the various types of NTLB. Over the past 37 years, urban development in the seven major geo-administrative regions was mainly focused in medium type NTLB areas.
In the seven major geo-administrative regions, the surface area without NTL declined, with the largest decrease in Southeast China, followed by East, Central, South, Southwest, and Northwest China (Supplementary Figure A4; ). Owing to geographic constraints, low type NTLA increased to varying degrees, with the greatest increase being in Southwest China, at 578%; medium, high, and extremely-high types NTLA increased most significantly in West China. NTL type transitions occurred most in the areas without NTL, followed by areas with low type NTL. The overall transition probability from the low type NTL to high and extremely-high types NTL was less than 4%, while that in the southwestern and northwestern regions was <1%.
4.1.3. Analysis of NTL in the five major urban agglomerations of China
Supplementary Figure A5 presents changes in the total NTLB and NTLA in the five major urban agglomerations, while shows the spatiotemporal variation in NTLA in these regions.
Figure 8. Spatiotemporal variation in nighttime light area in the five major urban agglomerations of China, 1984–2020.

The trends in NTLB in the five major urban agglomerations were similar to those in China overall and in the seven major geo-administrative regions, with three peaks in the 37-year period (in 2004, 2011, and 2019; Supplementary Figures A5). The increase in the proportion of NTLB in the CC urban agglomeration was the largest, at 1337.85%, although its total increase was smallest, at 99.96 × 104. The BR urban agglomeration exhibited the largest total increase in NTLB, at 501.26 × 104 (growth rate, 4.95 × 104 /a; p < 0.05). This urban agglomeration exhibited the highest total NTLB; over time, the gap in NTLB between it and the YRD urban agglomeration, ranked second in terms of total NTLB, gradually increased. The total NTLB of the other three major urban agglomerations was highest in the PRD urban agglomeration, followed by the MRYR urban agglomeration, and was lowest in the CC urban agglomeration.
The NTLA of the five major urban agglomerations increased overall; NTLA growth was faster in the BR and MRYR urban agglomerations, and in the other three agglomerations, NTLA growth rate was <0.5 × 104 km2/a (Supplementary Figures A5; ). BR-agglomeration NTLA growth was fastest (at 31.66 × 104 km2/a), far exceeding that of the other four agglomerations. In the MRYR and CC urban agglomerations, the proportional growth in NTLA was higher, with increases of 575.64% and 864.54%, respectively, and the increases were continuous. In the YRD, PRD, and BR agglomerations, NTLA increased fastest from 1984 to 1995, then gradually stabilised and increased more slowly.
Supplementary Figure A6 presents changes in each type of NTLB and NTLA in the five major urban agglomerations of China, while shows the spatiotemporal transitions in NTL type in these regions.
Figure 9. Spatiotemporal transition of nighttime light type in the five major urban agglomerations of China 1984–2020.

The trends in the various NTLB types in the five major urban agglomerations were similar to those in China overall and in the seven major geo-administrative regions: NTLB in medium, high, and extremely-high types increased, whereas low type NTLB increased then decreased (Supplementary Figure A6). However, owing to their different geographic locations, the changes in NTLB differed greatly between the urban agglomerations. The extremely-high type NTLB in the YRD and PRD urban agglomerations was predominant. The total increase in extremely-high NTLB in the YRD agglomeration was 173.01 × 104 (almost 1110.50%). The low, medium, and high types NTLB were consistently stable in the PRD urban agglomeration, whereas extremely-high type NTLB was the largest and exhibited a significant increase rate (75.13 × 104/a). In the BR urban agglomeration, the change in medium type NTLB was largest (at 261.13 × 104), followed by extremely-high, then high, and finally low type NTLB. The MRYR and CC urban agglomerations exhibited similar trends in NTLB, with much greater growth in medium type NTLB than in low, high, and extremely-high types NTLB. However, owing to their low baseline values, the increases in the various types NTLB were lower in the MRYR and CC urban agglomerations than in the other urban three agglomerations.
The main trends observed in the five major urban agglomerations were transitions from no or low type NTL to the higher types NTL (), with medium type NTLA increasing fastest, and high and extremely-high types NTLA increasing slowly. The area without NTL in each urban agglomeration declined significantly. The low type NTLA of the YRD, PRD, and the BR urban agglomerations decreased significantly. The BR urban agglomeration exhibited the greatest increase in NTLA for all types, with the largest being the increase in medium type NTLA, at 32.68 × 104 km2. The percentage increases in the various types NTLA were similar to total the NTLA for each types, with the largest being in the CC urban agglomeration, where extremely-high type NTLA exhibited the largest increase (1985.98%).
4.2. Analysis of CNLI
To measure the urbanisation development of the research areas, three sets of CNLI values were calculated using NTL data for China, its seven major geo-administrative regions, and its five major urban agglomerations. Line graphs were generated for analysis ().
Figure 10. Trends in CNLI in China, its seven major geo-administrative regions, and five major urban agglomerations, 1984–2020.

CNLI increased in China (a), and CNLI and NTLB exhibited very similar fluctuations in each year. Therefore, CNLI provides a potential indicator to measure urbanisation development. Overall, Chinese city development exhibited a fluctuating increasing trend, changing at 9.11 × 10–14/a (p < 0.01).
CNLI increased in the seven major geo-administrative regions, similarly to the overall Chinese trend (b). The CNLI trends in the various geo-administrative regions exhibited a clear sequence, with East China having the largest baseline CNLI and increase in CNLI, followed by South, Central, Northeast, and North China. In Southwest and Northwest China, the baseline CNLI was close to 0, and CNLI increased extremely slow; the final CNLI of Southwest China was slightly higher than that of Northwest China.
The CNLI trends for the five major urban agglomerations generally increase, similarly to those of China and the major geo-administrative regions (c). The CNLI trends for the different urban agglomerations exhibited a clear sequence, being largest for PRD, followed by BR, MRYR, and lowest for CC.
4.3. Data analysis of nighttime light concentration degree
To further understand the urbanisation of the study areas, NTL concentration degree was calculated and analysed ().
Figure 11. Nighttime light concentration degrees by various types in China, for the seven major geo-administrative regions, and five major urban agglomerations, 1984–2020.

During the 37-year study period, NTL in China was concentrated primarily in medium type NTL areas, and the proportion in medium type NTL areas increased over time (a). The proportion in extremely-high type NTL areas increased gradually from 2000 onward. No significant change was observed in the proportion in high type NTL areas, and that in low type NTL areas gradually decreased.
The NTL concentration degree in the seven major geo-administrative regions differed significantly from those in China overall (b–h). In East China before 2002, NTL was concentrated primarily in medium type NTL areas, while after 2002, it was concentrated in medium and extremely-high type NTL areas. NTL in North, Northeast, Central, Southwest, and Northwest China was concentrated primarily in the medium type NTL areas throughout the study period. NTL in South China was concentrated primarily in extremely-high type NTL areas.
The concentration degree of the various NTL types in the five major urban agglomerations differed substantially from those in China overall and in the seven major geographical administrative regions (i–m). Before 2002, in the YRD urban agglomeration, NTL was concentrated primarily in medium type NTL areas, while after 2002, it was concentrated primarily in extremely-high type NTL areas. Simultaneously, the proportion of low type NTL decreased continuously, reaching 1.69% in 2020. In the PRD urban agglomeration, NTL was concentrated primarily in extremely-high type NTL areas, at 39.06% in 1984 and increasing to 58.03% in 2020. The NTL concentration degree were similar in the BR, MRYR, and the CC urban agglomerations: NTL was concentrated primarily in medium type NTL areas, with high type NTL areas accounting for approximately 20% of NTL on average.
4.4. Data analysis of the rank-size rule
The evolution of city size distribution in China, its seven major geo-administrative regions, and its five major urban agglomerations (1984–2020) were calculated and analysed using the rank-size rule (; all findings were significant at p < 0.01).
Figure 12. Trends in the q-value in China, its seven major geo-administrative regions, and its five major urban agglomerations from (1984–2020).

In China, the q-value declined continuously (at – 0.02/a) from 1984 onward, transitioning from greater than 1 to less than 1 in 2001 and reaching 0.7898 in 2020. This indicates that the urbanisation gap in China gradually decreased (a).
In the seven major geo-administrative regions, the q-value declined slowly over the 37-year period, indicating that the gap in city size gradually decreased in these regions (b). Of the seven regions, South China exhibited the best spatial distribution of city size (which exhibited a Zipf’s distribution). Central China, East China, and Southwest China transitioned from having a first-place distribution to a second-place distribution in 1992, 1993, and 2003, respectively, with their q-values declining continuously. For Northwest China, the distribution of city size consistently reflects a first-place distribution, although its q-value decreased significantly (at −0.021/a), indicating that although small- and medium-sized cities in this region continued to grow, large cities remained more influential. The city size gap declined fastest in Southwest China, followed by South, Northwest, East, Central, North, and Northeast China.
In the five major urban agglomerations, over the 37-year study period, the q-value declined slowly, revealing a narrowing of the gap in city sizes and a more uniform distribution of cities (c). The YRD and PRD urban agglomerations transitioned from first-place to second-place distributions in 1987 and 1994, respectively, and their q-values declined continuously. The BR and the MRYR urban agglomerations were in second-place distributions and that the gap in city size was decreasing slowly. The CC urban agglomeration was in first-place distribution, although the gap in city size had been decreasing.
4.5. Data analysis of the Markov transition matrix
The results of MTM analysis largely depend on the period of the transition matrices (Sakamoto and Islam Citation2008). Therefore, using NTL data, this analysis was performed via a static MTM (SMTM) calculation with a period of 36 years (1984–2020), and via a dynamic MTM (DMTM) calculation with a period of 6 years (1984–1990, 1990–1996, 1996–2002, 2002–2008, 2008–2014, 2014–2020), for 370 Chinese cities (Types I–IV). Calculation of the DMTM and SMTM was carried out simultaneously, to compare their results and to elucidate long-term dynamic changes in city size in China for both the entire period (1984–2020) and the six different time periods (, ). The Markov transition matrix of China’s city size types based on NTL in this study had passed the time homogeneity test and the Markov test (the result can be found in the Supplemental data).
Table 2. Type transitions for 370 cities in China (1984–2020).
The numbers of transitions and transition probabilities (all >90%) were obviously much larger between cities of the same type than between cities of different types in SMTM (; a). For the same city type, the transition probabilities of Type I cities exhibited the greatest stability. Transitions between city types primarily occurred in two situations, first via type increases: the transitions I→II, II→III, and III→IV had transition probabilities of 3.45%, 4.76%, and 2.73%, respectively; and second, via type decreases: transitions II → I, III → II, IV → III had transition probabilities of 3.85%, 4.29%, and 5.04%, respectively. These findings indicate that higher types of city size were prone to regression. The transition probabilities were higher for decreasing city size type than for increasing city size type. Cities with transitioning from big city size to small city size during the research period are listed in Supplementary Table A2.
During the 37-year period, the number of same-type transitions for Types I and IV decreased continuously in DMTM (;b–g). In contrast, the number of same-type transitions between Types II and III increased continuously. This indicates that the smallest and largest sized cities were both becoming medium sized cities. To some extent, the conclusion based on the DMTM confirms that obtained using the SMTM. The DMTM analysis also revealed that some urban types experienced repeated transitions over several years.
5. Discussion and conclusions
5.1. Discussions
Over the past three decades, remotely sensed NTL images have become a global icon depicting the changing nightscape in a spatially explicit manner (Zheng et al. Citation2023). NTL help researchers to understand urbanisation. NTL areas can be used to represent the spatial extent of urbanisation, and NTL intensity can also reflect the dynamic principles governing the development of differently city sizes.
5.1.1. NTL-based comparative analysis of Chinese urbanisation
Previously, NTL-based urbanisation level studies in China relied primarily on the DMSP/OLS (Gao et al. Citation2021; Wei et al. Citation2019; Xu and Hu Citation2021; Xu, Lin, and Jin Citation2021) and NPP/VIIRS (Ding et al. Citation2022; Luo and Li Citation2019; Ma et al. Citation2020; Pan and Hu Citation2018; Xu, Li, and Chen Citation2018) datasets, and the longest study period was 1992–2020 (Yuan et al. Citation2022). The PANDA dataset used here has a time series of 37 years (1984–2020), providing up-to-date information on the Chinese urbanisation.
Using PANDA data, this study provides updated information on the dynamic evolution of urbanisation in China, drawing extensively on NTL-based urbanisation studies. While its findings are consistent with those of prior studies, it also offers new insights. First, In terms of the data rationality, Ma et al. (Citation2012) verified the quantitative relationship between DMSP/OLS NTL data and indicators such as urban population, GDP, and the extent of built-up area at the local scale for over 200 cities in China, demonstrating the rationality of NTL as an urbanisation indicator. Consistent with those findings, our analysis of PANDA data revealed a significant linear correlation between NTL, urban population, and impervious surface area, and a significant nonlinear correlation with GDP. Second, In terms of the spatiotemporal evolution of urbanisation: using Pareto regression and the rank-clock method. Huang et al. (Citation2015) and Gao et al. (Citation2017) analysed DMSP/OLS data to study city-size evolution of Chinese cities (1992–2008) and urban agglomeration (1992–2015), respectively, finding that cities became more evenly distributed over time. In our study, the ‘rank-size’ rule method was used to analyse urbanisation. In addition to finding that the urban gap size in China decreased over time (leading to more even urbanisation), it was found that the distribution of city sizes in Northeast China remained almost unchanged during the 37-year period. Relevant measures are therefore urgently needed to promote coordinated development among cities in Northeast China. Using NTL data for 1992–2013, Shen (Citation2021) constructed an index measuring urban concentration, concluding that the trend in the evolution of China’s urban system is towards flattening. Our study, however, reveals that for 1984–2020, China’s NTL first increased, then decreased, stabilised, and ultimately increased; its urbanisation has passed the flattening period, and its urban concentration is increasing and is developing three-dimensionally. Yang et al. (Citation2021) and Jiang et al. (Citation2020) based on corrected-integrated DMSP/OLS and NPP/VIIRS NTL data (1992–2018) and NPP/VIIRS NTL data (2013–2016), respectively, by defining shrinking cities, calculating and identifying China's shrinking cities, and obtained the percentage of shrinking cities in China as 23.39% and 27.88%, respectively. However, in our study, MTM analysis of all prefecture-level cities in China (1984–2020) revealed that the transition probability of city size types from large to small was 13.18%. The above two methods analyse the city size degradation in China from the spatial and temporal perspectives, respectively. Third, In terms of the spatial structure of urbanisation, we observed that the marked growth of high-NTLB areas in large cities caused medium-NTLB areas to shift to the periphery, while low-NTLB areas were significantly reduced in size, becoming fragmented and patchy owing to natural constraints such as the topography and coastline. In small and medium-sized cities, low-NTLB areas mostly continued to move towards the periphery or even expand. Urban expansion is primarily influenced by the natural environment and is not limited to administrative boundaries (Huang et al. Citation2021).
There is a need for ongoing study of new and effective urbanisation-evaluation methods based on the latest NTL dataset. This will elucidate China’s urbanisation in recent decades and reveal new valuable insights.
5.1.2. Urbanisation in China
Urbanisation is defined as a complex natural, cultural, and economic geographical phenomenon, where high-density population agglomerations, intensive infrastructure construction, and frequent economic activity exist in similar but changing forms over a huge range of scales (Bettencourt Citation2013). It is usually described in terms of population, economic, infrastructure, and NTL urbanisation (Bai, Shi, and Liu Citation2014). This study compared and analysed the China’s urbanisation using data on GDP, population, impervious surface, and NTL ().
Globally, China has experienced the largest transformation of its population from rural to city dwellers and from rural areas to cities, and the speed of these transformations has been staggering (Chen et al. Citation2019). China’s urbanisation has progressed with strong momentum, the data characteristics are exhibited in three growth categories: linear, sublinear and superlinear (Bettencourt et al. Citation2007). Over the past 37-year period, GDP increased 139.95-fold (exhibiting superlinear growth of exponential model); population growth reached 135.32% (exhibiting sublinear growth of power law model); NTLA growth reached 302.58% (exhibiting fluctuating linear growth); and for 1991–2020, the extent of impervious surface area increased by 229.00% (exhibiting significant linear growth). The speed and scale of urbanisation in China are unprecedented (Chen et al. Citation2019; Gao et al. Citation2015; Huang et al. Citation2015; Liu et al. Citation2012; Ma et al. Citation2012; Xu and Hu Citation2021). China’s urbanisation process has unique attributes, that depict a huge picture with its own characteristics.
5.1.3. Reasons for spatial differences in China’s urbanisation
The spatial differences in China’s urbanisation are essentially driven by its national policies and the limited amount of liveable space available.
China exhibits three main elevational regions, with natural conditions varying greatly between these regions. The Qinghai–Tibet Plateau in the western region has the highest altitude (on average exceeding 4000 m.a.s.l.); the central region is comprises mainly plateaus and basins (mostly 1000–2000 m.a.s.l.), and the eastern region comprises mostly plains and hills (on average <500 m.a.s.l.) (Li, Guo, and Ma Citation2022). This has resulted in three corresponding climate regions: the eastern monsoon climate region, the northwest arid and semi-arid climate region, and the Qinghai–Tibet high-altitude climate region (Li et al. Citation2010). Three major terrains and three major climate regions determine the macro framework and basic structure of China's urbanisation, resulting in the distribution of three major urban agglomerations (YRD, PRD, and BR) in the monsoon climate area of the eastern plains. The results of NTL urbanisation showed that the city size of these three major urban agglomerations was better than the other two urban agglomerations (MRYR and CC) distributed in the central region. There was no urban agglomeration distribution in the western region, and the CNLI index was the lowest in the Northwest and Southwest regions.
China's urbanisation is led by the government, which promotes, guides, and regulates urbanisation via its laws, regulations and public policies, and other means. For example, the reform and opening-up policy promoted the initial rapid development of the eastern coastal areas (Hu, Chen, and Li Citation2019), resulting in a higher NTL concentration of extremely-high type in the PRD and the YRD compared to other types. The overall CNLI index of the PRD was at the leading level among the five major urban agglomerations, and with the promotion of the YRD regional integration strategy (Qiu and Sun Citation2024), the CNLI index of the YRD surpassed the PRD for the first time in 2013. In 1982, the proposal of ‘Capital Circle’ in the ‘Overall Construction Plan of Beijing’ began the development of the BR (Fan, Lian, and Zhao Citation2022), resulting in the largest increase in NTLA and NTLB during the 37-year period. Policies such as the rise of the central region, the construction of new urbanisation and the coordinated development of the region had promoted the development of the CC and the MRYR, which had led to a high rate increase of NTLA and NTLB, and a decentralised trend of urban distribution (Zhu, Zhu, and Li Citation2021).
5.1.4. Importance of NTL urbanisation research for sustainable development
The acceleration of urbanisation can have serious negative impacts on natural resources and on the social environment, which in turn affecting the speed and quality of urbanisation (Chen et al. Citation2019). Therefore, it is necessary to consider the characteristics of each type of city in China and to make appropriate recommendations for urban development to meet the challenges of environmental sustainability and social equity. Here, the 370 cities in China were classified into four types, Types A, B, C, and D, based on their long time-series trends (only seven typical cities of each type were illustrated in the , and the detailed city classification results was presented in the Supplemental data).
Type A cities exhibited power law growth in NTLA over the 37-year period. Type A cities can be further divided into two types: large and mega cities and Cities in populous provinces. Owing to reasons such as urban resource availability and environmental carrying capacity constraints, most of these cities have reached a saturated level. Such cities should focus on developing the quality of urbanisation and ensuring the coordinated social and environmental development (Fan, Fang, and Zhang Citation2019). There is a need for better sharing of basic public services such as healthcare, education, safety, culture, and sports between migrant workers and vulnerable urban groups, with the aim of achieving equal access to basic public services (Qiao et al. Citation2014). The cities in populous provinces such as Henan and Shandong should focus on optimising its population structure, industrial structure, and urban spatial layout, and accelerate the construction of new urbanisation.
Type B cities are typical deep inland cities with exponential NTLA growth. Many of these cities are located in China’s poverty-stricken border areas, and the volatility of NTLA has increased significantly in recent years. Type B cities require national policies to enhance the vitality of urbanisation to achieve sustained growth. The government should apply external systemic forces, such as government subsidies, to break the path of regional dependence and achieve transformation and diversification of the industrial structure (Bai and Tan Citation2018).
Type C cities exhibited log-normal model growth in NTLA over the 37-year period. Type C cities can be further divided into two types: characteristic resource-based cities and third- and fourth-tier cities in Central and Western China. Owing to reasons such as resource depletion and industrial restructuring, resource-based cities tend to decline on the city size. Such cities should further optimise their business environment, promote structural adjustments, improve infrastructure, and enhance their overall competitiveness (Chen et al. Citation2019). The third- and fourth-tier cities in Central and Western China tend to exhibit urban decline, owing to their weak industrial foundation, the lack of sufficient endogenous driving force for development, and the lack of important location-based advantages. Such cities should develop their mountain tourism industries based on their local natural environment, while guiding the formation of a green industrial system and strengthening economic exchange with large neighbouring cities (Wei et al. Citation2020).
Type D cities exhibited linear growth in NTLA over the 37-year period. Type D cities are primarily characterised by features such as light industry, a distinctive culture, ports, tourism, and are distributed primarily in the eastern plains area. Such cities should promote the deep integration of culture and tourism, build a green and low-carbon municipal network, and optimise and enhance urban functions (Gu et al. Citation2021).
By scientifically monitoring and dynamically evaluating the urbanisation level of different regions in China using long-term NTL data, the number, scale, spatial agglomeration pattern, and distribution of cities in each region can be determined. Such findings can provide support for further optimising the spatial pattern of land-use and coordinating regional sustainable development.
5.2. Conclusions
This study examines the evolution of urbanisation and metropolitan area expansion in China using PANDA, the longest time series of NTL data available. Over the 37-year period, China’s urbanisation has increased rapidly, with outward expansion, and the gap in urban scale has decreased. There was more growth in the east and less growth in the west, and growth was faster in large cities and slower in small cities. The areas exhibiting expansion were concentrated primarily in low and medium NTL areas, and the expansion pattern exhibited a transitioned from Low NTL areas to medium NTL areas, and a diffusion from high and extremely-high NTL areas to medium NTL areas. With the exception of East China (including its YRD urban agglomeration) and South China (including its PRD urban agglomeration), where urban development was mainly concentrated in extremely-high NTL areas, all other regions were concentrated in medium NTL areas. The lowest-ranked cities exhibited the greatest stability in type transition of city size.
This study compares the urbanisation characteristics of several major administrative regions and metropolitan areas, identifies regional differences, and provides a theoretical references for the formulation of national economic policies. It reveals trends in urbanisation indicators in China over the 37-year period of reform and opening-up, and provides a quantitative references for achieving the 2030 Agenda for Sustainable Development Goal 11 (SDG11).
Nomenclature
Geolocation information
This paper’s study area is China.
Supplemental Material
Download MS Word (925.4 KB)Acknowledgements
The authors acknowledge all data contributors and platforms that provide data, and express gratitude to anonymous reviewers for constructive comments and improving advice.
Disclosure statement
No potential conflict of interest was reported by the author(s).
Data availability statement
The data that support the findings of this study are available from the corresponding author upon reasonable request.
Additional information
Funding
References
- Bai, Xuemei, Peijun Shi, and Yansui Liu. 2014. “Society: Realizing China’s Urban Dream.” Nature 509 (7499): 158–160. https://doi.org/10.1038/509158a.
- Bai, Ye, and Qinde Tan. 2018. “Study on the Economic Development Path of Inland National Centre Cities–Based on the Perspective of Inland Free Trade Zone.” Industrial & Science Tribune 10: 115–121.
- Bettencourt, Luís M A. 2013. “The Origins of Scaling in Cities.” Science 340 (6139): 1438–1441. https://doi.org/10.1126/science.1235823.
- Bettencourt, Luís M A, José Lobo, Dirk Helbing, Christian Kühnert, and Geoffrey B West. 2007. “Growth, Innovation, Scaling, and the Pace of Life in Cities.” Proceedings of the National Academy of Sciences 104 (17): 7301–7306. https://doi.org/10.1073/pnas.0610172104.
- Burrough, P. A. 2001. “GIS and Geostatistics: Essential Partners for Spatial Analysis.” Environmental and Ecological Statistics 8 (4): 361–377. https://doi.org/10.1023/A:1012734519752.
- Chen, Minghua, Huajun Liu, and Ya’nan Sun. 2016. “Research on the Spatial Differences and Distributional Dynamic Evolution of Financial Development of Five Megalopolises from 2003 to 2013 in China.” Journal of Quantitative & Technological Economics 33 (7): 130–144. https://doi.org/10.13653/j.cnki.jqte.2016.07.009.
- Chen, Mingxing, Chao Ye, Dadao Lu, Yuwen Sui, and Shasha Guo. 2019. “Cognition and Construction of the Theoretical Connotations of New Urbanization with Chinese Characteristics.” Journal of Geographical Sciences 29 (10): 1681–1698. https://doi.org/10.1007/s11442-019-1685-z.
- Chen, Yingbiao, Zihao Zheng, Zhifeng Wu, and Qinglan Qian. 2019. “Review and Prospect of Application of Nighttime Light Remote Sensing Data.” Progress in Geography 38 (2): 205–223. https://doi.org/10.18306/dlkxjz.2019.02.005.
- Cheng, Xin, Hua Shao, Yang Li, Chao Shen, and Peipei Liang. 2019. “Urban Land Intensive Use Evaluation Study Based on Nighttime Light—A Case Study of the Yangtze River Economic Belt.” Sustainability 11 (3): 675. https://doi.org/10.3390/su11030675.
- Connolly, Matthew H. 2018. Statistics and Gis. The Encyclopedia of Archaeological Sciences. https://doi.org/10.1002/9781119188230.saseas0552.
- Ding, Yuan, Jia Hu, Yingbao Yang, Wenyu Ma, Songxiu Jiang, Xin Pan, Yong Zhang, Jingjing Zhu, and Kai Cao. 2022. “Monitoring the Distribution and Variations of City Size Based on Night-Time Light Remote Sensing: A Case Study in the Yangtze River Delta of China.” Remote Sensing 14 (14): 3403. https://doi.org/10.3390/rs14143403.
- Dutt, Ashok K, Allen G Noble, G. Venugopal, and S. Subbiah. 2004. Challenges to Asian Urbanization in the 21st Century. Chennai: Springer Netherlands. https://doi.org/10.1007/1-4020-2531-9.
- Economic and Social Research Institude. 2007. “ArcGIS Desktop Help 9.2.” In Economic a. Redlands, CA, USA: Economic and Social Research Institute. https://webhelp.esri.com/arcgisdesktop/9.2/index.cfm?topicname=natural_breaks_%28jenks%29.
- Elvidge, C. D., K. E. Baugh, E. A Kihn, H. W. Kroehl, and E. R. Davis. 1997. “Mapping City Lights with Nighttime Data from the DMSP Operational Linescan System.” Eng & Remote Sens 63 (6): 727–734. https://doi.org/10.1016/S0924-2716(97)00008-7.
- Fan, Yupeng, Chuanglin Fang, and Qiang Zhang. 2019. “Coupling Coordinated Development Between Social Economy and Ecological Environment in Chinese Provincial Capital Cities-Assessment and Policy Implications.” Journal of Cleaner Production 229: 289–298. https://doi.org/10.1016/j.jclepro.2019.05.027.
- Fan, Jie, Yanan Lian, and Hao Zhao. 2022. “Review of the Research Progress in Beijing-Tianjin-Hebei Region Since 1980.” Acta Geographica Sinica 77 (6): 1299–1319. https://doi.org/10.11821/dlxb202206002.
- Fu, Huyan, Zhenfeng Shao, Peng Fu, and Qimin Cheng. 2017. “The Dynamic Analysis Between Urban Nighttime Economy and Urbanization Using the DMSP/OLS Nighttime Light Data in China from 1992 to 2012.” Remote Sensing 9 (5): 416. https://doi.org/10.3390/rs9050416.
- Gao, Bin, Qingxu Huang, Chunyang He, and Yinyin Dou. 2017. “Similarities and Differences of City-Size Distributions in Three Main Urban Agglomerations of China from 1992 to 2015: A Comparative Study Based on Nighttime Light Data.” Journal of Geographical Sciences 27 (5): 533–545. https://doi.org/10.1007/s11442-017-1391-7.
- Gao, Bin, Qingxu Huang, Chunyang He, and Qun Ma. 2015. “Dynamics of Urbanization Levels in China from 1992 to 2012: Perspective from DMSP/OLS Nighttime Light Data.” Remote Sensing 7 (2): 1721–1735. https://doi.org/10.3390/rs70201721.
- Gao, Han, Jiahong Liu, Chao Mei, Wang Hao, and Chuang Liu. 2021. “Calculating the Water Dissipation of Buildings in Urban Areas Based on Global Nighttime Light Data.” Mapping Intimacies 15 (11): e0242663. https://doi.org/10.21203/rs.3.rs-428698/v1.
- Gong, Peng, Xuecao Li, Jie Wang, Yuqi Bai, Bin Chen, Tengyun Hu, Xiaoping Liu, et al. 2020. “Annual Maps of Global Artificial Impervious Area (GAIA) Between 1985 and 2018.” Remote Sensing of Environment 236: 111510. https://doi.org/10.1016/j.rse.2019.111510.
- Gu, Jiang, Xin Chen, Xinru Guo, and Suyan Zhang. 2021. “The Logical Framework and Strategic Path for Perfecting the Modern Cultural Industry System During the 14th Five-Year Plan Period.” Journal of Management World 37 (3): 9–18. https://doi.org/10.19744/j.cnki.11-1235/f.2021.0032.
- He, Chunyang, Zhifeng Liu, Siyuan Gou, Qiaofeng Zhang, Jinshui Zhang, and Linlin Xu. 2019. “Detecting Global Urban Expansion Over the Last Three Decades Using a Fully Convolutional Network.” Environmental Research Letters 14 (3): 34008. https://doi.org/10.1088/1748-9326/aaf936.
- He, Chunyang, Wenlu Lu, Min Xu, and Zhifeng Liu. 2022. “Dataset of Urban Built-up Area in China (1992-2020) V1.0.” National Tibetan Plateau Data Centre. https://doi.org/10.11888/HumanNat.tpdc.272851.
- Henderson, M., E. T. Yeh, P. Gong, C. Elvidge, and K. Baugh. 2003. “Validation of Urban Boundaries Derived from Global Night-Time Satellite Imagery.” International Journal of Remote Sensing 24 (3): 595–609. https://doi.org/10.1080/01431160304982.
- Hu, Wei, Xiaodong Chen, and Chuansong Li. 2019. “The Evolution of the Spatial Pattern of China’s Industrial Economic Development Since the Reform and Opening-Up.” Jiangsu Social Sciences 2: 43–53. https://doi.org/10.13858/j.cnki.cn32-1312/c.2019.02.006.
- Huang, Qingxu, Chunyang He, Bin Gao, Yang Yang, Zhifeng Liu, Yuanyuan Zhao, and Yue Dou. 2015. “Detecting the 20 Year City-Size Dynamics in China with a Rank Clock Approach and DMSP/OLS Nighttime Data.” Landscape and Urban Planning 137: 138–148. https://doi.org/10.1016/j.landurbplan.2015.01.004.
- Huang, Qingxu, Han Zhang, Jasper van Vliet, Qiang Ren, Raymond Yu Wang, Shiqiang Du, Zhifeng Liu, and Chunyang He. 2021. “Patterns and Distributions of Urban Expansion in Global Watersheds.” Earth’s Future 9 (8): e2021EF002062. https://doi.org/10.1029/2021EF002062.
- Jenks, G. F. 1967. “The Data Model Concept in Statistical Mapping.” International Yearbook of Cartography 7 (1): 186–190.
- Jiang, Shengnan, Guoen Wei, Zhenke Zhang, Yue Wang, Minghui Xu, Qing Wang, Priyanko Das, and Binglin Liu. 2020. “Detecting the Dynamics of Urban Growth in Africa Using DMSP/OLS Nighttime Light Data.” Land 10 (13): 1–19. https://doi.org/10.3390/land10010013.
- Jiang, Zhidian, Weixin Zhai, Xiangfeng Meng, and Ying Long. 2020. “Identifying Shrinking Cities with NPP-VIIRS Nightlight Data in China.” Journal of Urban Planning and Development 146 (4): 4020034. https://doi.org/10.1061/(ASCE)UP.1943-5444.0000598.
- Kamarajugedda, Shankar Acharya, Pradeep V Mandapaka, and Edmond Y M Lo. 2017. “Assessing Urban Growth Dynamics of Major Southeast Asian Cities Using Night-Time Light Data.” International Journal of Remote Sensing 38 (21): 6073–6093. https://doi.org/10.1080/01431161.2017.1346846.
- Kundak, Seda, and Vedia Dkmeci. 2018. “A Rank-Size Rule Analysis of The City System at The Country and Province Level in Turkey.” ICONARP International Journal of Architecture and Planning 6 (1): 77–98. https://doi.org/10.15320/ICONARP.2018.39.
- Levin, Noam, and Yishai Duke. 2012. “High Spatial Resolution Night-Time Light Images for Demographic and Socio-Economic Studies.” Remote Sensing of Environment 119: 1–10. https://doi.org/10.1016/j.rse.2011.12.005.
- Li, M. Q., Q. S. Ge, Z. X. Hao, J. Y. Zheng, and S. F. He. 2010. “Characteristics of Cold–Warm Variation in the Hetao Region and Its Surrounding Areas in China During the Past 5000 Years.” Climate of the Past 6 (4): 475–481. https://doi.org/10.5194/cp-6-475-2010.
- Li, Tingting, Zengzhang Guo, and Chao Ma. 2022. “Spatiotemporal Changes of Piedmont Phenology in the Transitional Zone Between the Second and Third Steps, China.” Geographical Research 41 (11): 3000–3020. https://doi.org/10.11821/dlyj020211206.
- Li, Lanbing, and Binglian Liu. 2020. “Prospect for Major Issues of China’s Regional Economic Development During the 14th Five-Year Plan Period.” Journal of Management World 36 (5): 17. https://doi.org/10.19744/j.cnki.11-1235/f.2020.0068.
- Li, Wenbin, Yongfeng Lyu, Shaoming Wang, Xiaoyan Zhou, and Jianming Ying. 2021. “Trends in Molecular Testing of Lung Cancer in Mainland China Over the Decade 2010 to 2019.” JTO Clinical and Research Reports 2 (4): 100163. https://doi.org/10.1016/j.jtocrr.2021.100163.
- Liu, Zhifeng, Chunyang He, Qiaofeng Zhang, Qingxu Huang, and Yang Yang. 2012. “Extracting the Dynamics of Urban Expansion in China Using DMSP-OLS Nighttime Light Data from 1992 to 2008.” Landscape and Urban Planning 106 (1): 62–72. https://doi.org/10.1016/j.landurbplan.2012.02.013.
- Luo, Qing, and Xiaojian Li. 2019. “The Spatial Differentiation and Influencing Factors of Urban Centres in China Based on VIIRS Night Light.” Geographical Research 38 (1): 155–166. https://doi.org/10.11821/dlyj020180467.
- Ma, Jinji, Jinyu Guo, Safura Ahmad, Zhengqiang Li, and Jin Hong. 2020. “Constructing a New Inter-Calibration Method for DMSP-OLS and NPP-VIIRS Nighttime Light.” Remote Sensing 12 (6): 937. https://doi.org/10.3390/rs12060937.
- Ma, Mingguo, Qin Lang, Hong Yang, Kaifang Shi, and Wei Ge. 2020. “Identification of Polycentric Cities in China Based on NPP-VIIRS Nighttime Light Data.” Remote Sensing 12 (19): 3248. https://doi.org/10.3390/rs12193248.
- Ma, Ting, Chenghu Zhou, Tao Pei, Susan Haynie, and Junfu Fan. 2012. “Quantitative Estimation of Urbanization Dynamics Using Time Series of DMSP/OLS Nighttime Light Data: A Comparative Case Study from China’s Cities.” Remote Sensing of Environment 124: 99–107. https://doi.org/10.1016/j.rse.2012.04.018.
- Meisner, T. 2020. “Urbanization and the Ecology of the Urban Environment: Risks and Prospects for Sustainable Development.” Humanities of the South of Russia 9 (3): 190–201. https://doi.org/10.18522/2227-8656.2020.3.14.
- Milesi, C., C. D. Elvidge, R. R. Nemani, and S. W. Running. 2003. “Assessing the Impact of Urban Land Development on Net Primary Productivity in the Southeastern United States.” Remote Sensing of Environment 86 (3): 401–410. https://doi.org/10.1016/S0034-4257(03)00081-6.
- Montgomery, Mark R. 2008. “The Urban Transformation of the Developing World.” Science 319 (5864): 761–764. https://doi.org/10.1126/science.1153012.
- Pan, Jinghu, and Yanxing Hu. 2018. “Spatial Identification of Multi-Dimensional Poverty in Rural China: A Perspective of Nighttime-Light Remote Sensing Data.” Journal of the Indian Society of Remote Sensing 46 (7): 1093–1111. https://doi.org/10.1007/s12524-018-0772-4.
- Pu, Yingxia, Ronghua Ma, Xiaodong Ma, and Chaolin Gu. 2009. “Spatio-Temporal Dynamics of City-Size Distribution in Yangtze River Delta.” Geographical Research 28 (1): 161–172. https://doi.org/10.11821/yj2009010018.
- Qiao, Qiao, Guiguang Hou, Ning Sun, and Jianxin Xia. 2014. “Construction and Empirical Study on Evaluation Index System for the Equalization of Environmental Basic Public Service.” Environmental Science & Technology 37 (12): 241–246.
- Qiu, Siyuan, and Wei Sun. 2024. “Measuring Integrative Level and Analysis of Radiative Capacity in China’s Urban Agglomerations.” Geographical Research 43 (2): 303–321. https://doi.org/10.11821/dlyj020230597.
- Sakamoto, Hiroshi, and Nazrul Islam. 2008. “Convergence Across Chinese Provinces: An Analysis Using Markov Transition Matrix.” China Economic Review 19 (1): 66–79. https://doi.org/10.1016/j.chieco.2006.07.002.
- Shaban, Abdul, Karima Kourtit, and Peter Nijkamp. 2020. “India’s Urban System: Sustainability and Imbalanced Growth of Cities.” Sustainability 12 (7): 2941. https://doi.org/10.3390/su12072941.
- Shen, Jie. 2020. “The Impact of Intercity Connection on Population Distribution in Urban Agglomerations: Based on Interlocking Model and Nighttime Light Data.” Population & Economics 3: 14–31. https://doi.org/10.3969/j.issn.1000-4149.2020.00.019.
- Shen, Jie. 2021. “The Measurement of Urban Concentration in China and the Study of Its Spatial Differentiation Characteristics : Based on DMSP-OLS Nighttime Light Data.” Economic Geography 41 (5): 46–56. https://doi.org/10.15957/j.cnki.jjdl.2021.05.006.
- Sutton, P. 1997. “Modeling Population Density with Night-Time Satellite Imagery and GIS.” Computers Environment & Urban Systems 21 (3–4): 227–244. https://doi.org/10.1007/s12524-021-01443-1.
- Wei, Shimei, Jinghu Pan, Yongnian Zhang, and Dahong Zhang. 2019. “Based on Spatial Evolution of Zhongyuan Urban Agglomeration Based on DMSP-OLS Nighttime Light Data.” Human Geography 34 (6): 12. https://doi.org/10.13959/j.issn.1003-2398.2019.06.009.
- Wei, Deng, Shao Yao Zhang, Hao Zhang, Li Peng, and Ying Liu. 2020. “Transitional Geospace from the Perspective of Human-Nature Coupling: Concept, Connotations, Attributes, and the Research Framework.” Geographical Research 39 (4): 761–771. https://doi.org/10.11821/dlyj020200189.
- Xu, Huimin, and Shougeng Hu. 2021. “Chinese City Size Evolution Under Perspective of Nighttime Light Remote Sensing.” Geomatics and Information Science of Wuhan University 46 (1): 40–49. https://doi.org/10.13203/j.whugis20190330.
- Xu, Weipan, Xun Li, and Haohui Chen. 2018. “A Comparative Research on the Rank-Size Distribution of Cities in China and the United States Based on Urban Nighttime Light Data.” Progress in Geography 37 (3): 385–396. https://doi.org/10.18306/dlkxjz.2018.03.009.
- Xu, Pengfei, Muying Lin, and Pingbin Jin. 2021. “Spatio-Temporal Dynamics of Urbanization in China Using DMSP/OLS Nighttime Light Data from 1992–2013.” Chinese Geographical Science 31 (1): 70–80. https://doi.org/10.1007/s11769-020-1169-1.
- Xu, Jiejia, Shuying Zang, and Xiaodong Na. 2020. “Evolution of Urban Spatial Patterns in the Three Provinces of Northeast China Based on the Nighttime Light Data.” Journal of Glaciology and Geocryology 42 (2): 693–703. https://doi.org/10.7522/j.issn.1000-0240.2019.1041.
- Yang, Jie, and Xin Huang. 2021. “The 30 m Annual Land Cover Dataset and Its Dynamics in China from 1990 to 2019.” Earth System Science Data 13 (8): 3907–3925. https://doi.org/10.5281/zenodo.4417810.
- Yang, Yang, Jianguo Wu, Ying Wang, Qingxu Huang, and Chunyang He. 2021. “Quantifying Spatiotemporal Patterns of Shrinking Cities in Urbanizing China: A Novel Approach Based on Time-Series Nighttime Light Data.” Cities 118: 103346. https://doi.org/10.1016/j.cities.2021.103346.
- You, Haolin, Jun Yang, Bing Xue, Xiangming Xiao, Jianhong Xia, Cui Jin, and Xueming Li. 2021. “Spatial Evolution of Population Change in Northeast China During 1992–2018.” Science of The Total Environment 776: 146023. https://doi.org/10.1016/j.scitotenv.2021.146023.
- Yu, Bailang, Congxiao Wang, Wenkang Gong, Zuoqi Chen, Kaifang Shi, Bin Wu, Yuchen Hong, Qiaoxuan Li, and Jianping Wu. 2021. “Nighttime Light Remote Sensing and Urban Studies: Data, Methods, Applications, and Prospects.” National Remote Sensing Bulletin 25 (1): 342–364. https://doi.org/10.11834/jrs.20211018.
- Yuan, Xiaotian, Li Jia, Massimo Menenti, and Min Jiang. 2022. “Consistent Nighttime Light Time Series in 1992–2020 in Northern Africa by Combining DMSP-OLS and NPP-VIIRS Data.” Big Earth Data 6 (4): 603–632. https://doi.org/10.1080/20964471.2022.2031542.
- Zhang, Lixian, Zhehao Ren, Bin Chen, Peng Gong, Haohuan Fu, and Bing Xu. 2021. “A Prolonged Artificial Nighttime-Light Dataset of China (1984-2020).” National Tibetan Plateau / Third Pole Environment Data Center. https://doi.org/10.11888/Socioeco.tpdc.271202.
- Zheng, Qiming, Karen C Seto, Yuyu Zhou, Shixue You, and Qihao Weng. 2023. “Nighttime Light Remote Sensing for Urban Applications: Progress, Challenges, and Prospects.” ISPRS Journal of Photogrammetry and Remote Sensing 202: 125–141. https://doi.org/10.1016/j.isprsjprs.2023.05.028.
- Zheng, Zihao, Zhifeng Wu, Yingbiao Chen, Zhiwei Yang, and Francesco Marinello. 2020. “Exploration of Eco-Environment and Urbanization Changes in Coastal Zones: A Case Study in China Over the Past 20 Years.” Ecological Indicators 119: 106847. https://doi.org/10.1016/j.ecolind.2020.106847.
- Zheng, Zihao, Zhifeng Wu, Yingbiao Chen, Zhiwei Yang, and Francesco Marinello. 2021. “Analyzing the Ecological Environment and Urbanization Characteristics of the Yangtze River Delta Urban Agglomeration Based on Google Earth Engine.” Acta Ecologica Sinica 41 (2): 717–729. https://doi.org/10.5846/stxb202003250687.
- Zhou, Decheng, Shuqing Zhao, Shuguang Liu, Liangxia Zhang, and Chao Zhu. 2014. “Surface Urban Heat Island in China’s 32 Major Cities: Spatial Patterns and Drivers.” Remote Sensing of Environment 152: 51–61. https://doi.org/10.1016/j.rse.2014.05.017.
- Zhu, Zheng, Xiang Zhu, and Shuangshuang Li. 2021. “Evolution Process and Characteristics of Spatial Structure of Urban Agglomeration in the Middle Reaches of the Yangtze River.” Acta Geographica Sinica 76 (4): 799–817. https://doi.org/10.11821/dlxb202104003.