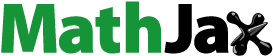
ABSTRACT
Poverty continues to pose significant global challenges. Analyzing poverty distribution is pivotal for identifying spatial and demographic disparities, informing targeted policy interventions, and fostering inclusive and equitable development. The absence of a worldwide pixel-scale time-series poverty dataset has hampered effective policy formulation. To address this gap, we employ the international wealth index (IWI) derived from household survey data to represent poverty levels. Subsequently, a random forest regression model was constructed, with IWI serving as the dependent variable and representative features extracted from nighttime lights, land cover, digital elevation model, and World Bank statistical data serving as independent variables. This yielded a global map of the IWI for low- and middle-income nations at a 10-km resolution spanning 2005 to 2020. The model demonstrated robust performance with an R2 value of 0.74. Over the studied period, areas and populations with IWI ≤ 50 decreased by 8.85% and 16.17%, indicating a steady decrease in global poverty regions. Changes in the IWI at the pixel scale indicate that areas closer to cities have faster growth rates. Furthermore, our poverty estimation models present a novel method for real-time pixel-scale poverty assessments. This study provides valuable insights into the dynamics of poverty, both globally and nationally.
1. Introduction
Poverty is an important factor hindering social progress and economic development. According to the latest World Bank statistics, in 2019, 9% of the world’s population lived in poverty (World Bank Citation2023a). In 2015, the United Nations Sustainable Development Goals (SDGs) listed poverty eradication as one of the top 17 goals, ending poverty in all its forms everywhere (United Nations Citation2023a; Zhou and Liu Citation2022). Therefore, to support poverty alleviation and reduction, it is necessary to monitor and assess the distribution characteristics and trends of poverty effectively.
Traditional poverty measurements rely primarily on survey data from the government and field surveys (Gouveia, Seixas, and Long Citation2018; Jean et al. Citation2016). Because most survey data are based on administrative divisions, the specific characteristics of poverty distribution within these units cannot be observed (Lin et al. Citation2022; Wang, Cheng, and Zhang Citation2012). Additionally, nationally representative household surveys are expensive and time-consuming, and developing and less-developed countries may not be able to afford up-to-date data (Han et al. Citation2021; Henderson, Storeygard, and Weil Citation2012; Sandefur and Glassman Citation2015; Shi et al. Citation2020). These shortcomings can lead to longer and more delayed data update periods, particularly in poor and remote areas (Jean et al. Citation2016; Pokhriyal and Jacques Citation2017; Serajuddin et al. Citation2015). In the absence of survey data, the determination and identification of poverty are difficult.
Earth satellite remote-sensing technology has the advantage of large-scale, real-time dynamic monitoring and can provide a stable data source for poverty identification and assessment. Poverty prediction based on traditional remote sensing data has been widely used at the continental (Perez et al. Citation2017), national (Perez et al. Citation2017; Pokhriyal and Jacques Citation2017), city (Duque et al. Citation2015), and village scales (Barnett et al. Citation2020; Hu et al. Citation2022; Imran, Stein, and Zurita-Milla Citation2014; Ratledge et al. Citation2022). Traditional remote sensing data have also been widely used in poverty research in different fields, such as socioecology (Watmough et al. Citation2019) and Social Assistance (Smythe and Blumenstock Citation2022). In addition, it is worth mentioning that small-area poverty estimation has been an integral component of poverty research. There has been a significant advancement in small-area poverty mapping using remotely sensed variables as supporting information in recent years (De Nicolò, Fabrizi, and Gardini Citation2022; Masaki et al. Citation2022; Newhouse et al. Citation2022; Permatasari, Laksono, and Ubaidillah Citation2023; Ridho, Aisyatul, and Prasetyo Citation2023; Schmid et al. Citation2017). As poverty is a complex phenomenon and traditional remote sensing data are limited in directly capturing human activities, it is feasible to utilize diverse non-traditional remote sensing data to analyze poverty characteristics from multiple perspectives and construct more accurate poverty maps.
Remote sensing of nighttime light (NTL) offers the unique ability to monitor human activity in space. Previous studies have shown a strong positive correlation between NTL and population density (Elvidge et al. Citation2009). NTL can reflect poverty information by capturing the strength of surface light signals (Li et al. Citation2019; Li et al. Citation2019; Noor et al. Citation2008; Shi et al. Citation2020), bridging the gap in poverty estimation using remote sensing data. Elvidge et al. (Citation2009) used NTL data to create a global extreme poverty map at a resolution of 1 km2. Subsequently, NTL data were used as proxies for income and wealth. The related studies can be divided into the following categories: First, NTL and survey data were used to estimate poverty on a small scale, mainly in settlements, counties, and cities (Ni et al. Citation2020; Noor et al. Citation2008; Xu et al. Citation2021; Yu et al. Citation2015). Second, NTL and high-resolution image data, such as Google images and land-use data, were used to obtain national- and continental-scale poverty projections for a short period or a single year using an estimation model (e.g. convolutional neural network (CNN) and Random Forest) (Andreano et al. Citation2020; Jean et al. Citation2016; Lee and Braithwaite Citation2022; Li et al. Citation2020; Ratledge et al. Citation2022; Zhao et al. Citation2019). Finally, poverty was explored on various scales at specific times by integrating NTL, social media, and point-of-interest data (Blumenstock Citation2016; Blumenstock, Cadamuro, and On Citation2015; Shi et al. Citation2020).
Previous studies primarily focused on estimating poverty at various scales. However, a significant number of these studies are limited to single-phase investigations, often overlooking crucial aspects of poverty estimation over extended periods. This results in our inability to understand the spatiotemporal dynamics of poverty promptly and analyze trends in poverty changes. Many studies have used the wealth index to measure poverty (Lee and Braithwaite Citation2022; Ratledge et al. Citation2022; Zhao et al. Citation2019), making it an important method in poverty research. However, the current lack of large-scale global wealth index data limits the use of wealth indices in poverty estimation. To overcome these shortcomings, this study employs the International Wealth Index (IWI), derived from household survey data, to characterize poverty. By leveraging publicly available and regularly updated multi-source data, NTL data, land-use data, digital elevation model (DEM) data, and World Bank statistical data, we developed a poverty estimation model for global low- and middle-income countries using a random forest regression (RFR) model. Subsequently, we generated a time-series spatial distribution map depicting poverty in global low- and middle-income countries for the years 2005–2020. The following section describes the datasets used in this study. Section 3 presents the data preprocessing and poverty estimation methods. The results are presented in Section 4, followed by a discussion in Section 5. Finally, the conclusions are presented in Section 6.
2. Datasets
In this study, multi-source datasets were used to estimate global poverty in low- and middle-income countries (Appendix Table S1), including household surveys, land use, DEM, NPP-VIIRS-like NTL, and gross national income per capita data. Remote sensing data from multiple sources were projected onto a Mollweide projection. Among these data, the household survey data were used to calculate the IWI, which serves as the dependent variable in the poverty estimation model. The other datasets were used as independent variables.
2.1. Household survey data and IWI
Household survey data were obtained from the Demographic and Health Survey (DHS) Program (https://dhsprogram.com/topics/wealth-index/). This program is a valuable resource for estimating crucial indicators such as household wealth, education, and health (Bruederle and Hodler Citation2018; Jean et al. Citation2016; Weiss et al. Citation2018). To ensure sufficient sample size and alignment with other data time intervals, global poverty was estimated every 5 years instead of annually. Household survey data for different countries were notably concentrated in 2015 (Appendix Table S2). Therefore, using data from this year, we developed a poverty estimation model applicable to 2005, 2010, and 2020.
The DHS household survey data serve as a crucial tool for analyzing within-country and relative economic poverty. However, its initial construction as a country-specific, time-bound relative index (Rutstein and Staveteig Citation2014) limits the direct comparability of WI across countries and over various time periods. In response to this issue, numerous scholars have exerted considerable effort to enhance the comparability of the DHS Wealth Index across various research surveys. Rutstein and Staveteig (Citation2014) introduced the comparable wealth index (CWI) and demonstrated a robust linear correlation between the CWI average and its logarithmic transformation as measured against Gross National Income per capita at Purchasing Power Parity (GNI/P (PPP)). Similarly, Smits and Steendijk (Citation2015) introduced the IWI, establishing a universal set of asset weights across 12 common asset categories. In this study, IWI was selected as the dependent variable because of its broad applicability and comparative utility.
The IWI serves as a comparable indicator of household material well-being and economic status. Its applicability spans all low- and middle-income countries, ensuring comparability across different regions and periods (Smits and Steendijk Citation2015). Data on 12 essential assets are required to calculate a household’s IWI accurately. These include seven consumer durables (ownership of a TV, fridge, phone, bike, car, low-cost utensils, and high-cost utensils), access to two public services (water and electricity), and three housing characteristics (number of sleeping rooms, quality of floor material, and quality of toilet facilities). The IWI scale is additive, and its formula is as follows:
(1)
(1) where
represents the indicator variables and
represents the IWI weights. The IWI ranges from 0 to 100, where 0 represents households with no assets and the lowest-quality housing, and 100 represents households with all assets and the highest-quality housing.
In this study, the IWI was calculated using DHS household survey datasets. Given the IWI allowance for up to three missing indicators, we excluded low- and high-cost appliance utensils that were absent in most countries. In addition, some countries may have additional missing indicators beyond these two. Therefore, for each set of missing variables (s), a specific formula was applied to ensure accurate IWI calculations. The relevant datasets are available on the IWI website (https://globaldatalab.org/iwi/).
2.2. Other multi-source data
The independent variables in the poverty estimation model are obtained from multiple sources (). The first variable was NTL intensity calculated using NPP-VIIRS-like data (Chen et al. Citation2021). The time series of NPP-VIIRS-like NTL data was generated through a new cross-sensor calibration from DMSP-OLS NTL data and the composition of monthly NPP-VIIRS NTL data (Chen et al. Citation2021).
Table 1. The descriptions and values of RFR model parameters.
Second, land-use data were acquired from the European Space Agency (ESA) Climate Change Initiative (CCI) project (http://maps.elie.ucl.ac.be/CCI/viewer/) to extract different land-use types. In this study, land-cover features were integrated into five categories: farmland, unused land, urban land, vegetation, and water. Subsequently, the percentage of each land-use type within each grid was used as an independent variable in the model.
DEM data were extracted from the National Aeronautics and Space Administration (NASA) Earth Observation Data (https://search.earthdata.nasa.gov/) to calculate topographical parameters (e.g. elevation and slope). The mean elevation and slope of each grid were used as independent variables in the model.
Furthermore, to analyze the impact of the national income level classification on poverty estimates, country classifications based on gross national income per capita measured by the purchasing power parity were downloaded from the World Bank’s open database (https://data.worldbank.org.cn/). This study refers to three groups within the world economic category: upper-middle-income, lower-middle-income, and low-income, marking countries 3, 2, and 1, respectively.
Administrative boundaries were obtained from the Global Administrative Area Map (GADM, https://gadm.org/). Population data with a 1 km spatial resolution were collected from the WorldPop Open Population Repository (WOPR, https://www.worldpop.org/). These data were used to extract human settlements.
3. Method
The research framework, as shown in , consists of data preprocessing, model establishment, accuracy verification, and generation of global spatial distribution maps. First, the IWI was calculated based on household survey data, and the characteristic variables were extracted by preprocessing data from multiple sources using a 10 × 10 km grid as the foundational unit. Subsequently, a random forest regression model was constructed using the extracted multi-source variables. Subsequently, the accuracy of the estimated results is verified. Finally, a 10 × 10 km grid was constructed to cover low-income and middle-income countries. The variables within each grid were counted, and the RFR model was used to predict the IWI for all grids. The estimation results were masked using population data, thus retaining only the estimation results for human settlements.
3.1. Data preprocessing
The DHS household survey uses data points to represent the wealth status of a cluster consisting of approximately 30 households. To protect the privacy of the surveyed households, the DHS provided the average latitude and longitude of the household clusters, with a positional displacement applied to each cluster. This allowed for errors of up to 5 km in each direction (with 1% of the rural household clusters permitting errors of up to 10 km). The cluster-level average IWI was obtained by averaging the IWI values of all households within a cluster. The average IWI is then used as the target variable for the poverty estimation model.
Considering the positional error of up to 5 km added to each DHS household survey cluster location, we created a 10 km × 10 km grid centered at each cluster location to ensure that the true location fell within the grid. A 10 km × 10 km grid served as the fundamental unit of analysis, and features were extracted for each grid. Regional statistical tools were used to calculate the mean, maximum, minimum, and area of variables within the grid. The final step was to organize the data from different variables to simplify the model-building process.
3.2. Model building
The random forest algorithm is an important method in machine learning that has been applied and validated for poverty estimation at different scales (county, city, national, and global) (Niu, Chen, and Yuan Citation2020; Putri, Wijayanto, and Pramana Citation2023; Puttanapong, Prasertsoong, and Peechapat Citation2023; Tian et al. Citation2022; Yao et al. Citation2023; Zhao et al. Citation2019). In this study, the random forest method was used to model and estimate poverty. To verify the superiority of the RFR model, we compared the RFR model with other regression methods, including linear regression (LR), multilayer perceptron (MLP) (Bishop Citation1995; LeCun, Bengio, and Hinton Citation2015), and support vector regression (SVR) (Cortes and Vapnik Citation1995) method. First, the IWI was integrated as the dependent variable, and the features extracted from multiple data sources were used as independent variables (). In this study, 70% of the samples were selected as training samples, and 30% were selected as validation samples. Second, the RFR model parameters were optimized; lists the parameters that were optimized in the RFR model. The parameter values were determined using the grid search method (Lerman Citation1980), a technique for parameter tuning that involves systematic iterations through all possible combinations of candidate parameter values. The best-performing parameters identified through this exhaustive search were selected as final results. We estimated the IWI values of household clusters globally using a 10-fold cross-validation method in which household clusters were randomly divided into 10 equally sized subsets. The model was trained using nine folds as training data, and the trained model was validated using the remaining part of the data. Finally, the optimized parameters were used to train and predict the model.
3.3. Accuracy verification
This study assessed the accuracy of the model using the test-set coefficient of determination (R²), adjusted R² (adj-R²), and out-of-bag score (OOB), as well as numerical analyses of the poverty maps, including the calculation of the root-mean-square error (RMSE) and mean absolute error (MAE). R² is a widely used metric for evaluating the model performance, with values closer to 1 indicating higher accuracy. In contrast, the OOB score is an internal evaluation method specifically used in random forest algorithms. It assesses the performance of the model in real time by calculating its accuracy using samples that are not selected for training, thereby eliminating the need for an additional test set. This approach provides a more reliable indication of the generalization ability of the model. Adj-R ² provides a more accurate reflection of the model's goodness of fit to the observed data, helps prevent overfitting, and enhances the model's generalization ability.
By combining the OOB score, R², and adj-R², a more comprehensive evaluation of model accuracy can be achieved by capturing various aspects of model performance. Higher values of the OOB score, R², and adj-R² indicated better generalization ability and prediction performance of the model.
4. Results
4.1. Model accuracy and feature importance of the RFR model
The selected independent variables were used for RFR modeling after screening and parameter optimization. shows the accuracy comparison of different models; the RFR model achieved the highest model accuracies (R2 = 0.74, adj _R2 = 0.74), along with the smallest errors in MAE and RMSE. Additionally, we calculated the OOB score for the RFR model (0.74), indicating high accuracy and credibility. Therefore, the RFR model was selected as the optimal model for the simulation.
Table 2. Accuracy comparison of different models.
shows all the features used to develop the RFR model, indicating that the NTL data features contributed significantly, accounting for 62% of the model's importance. The SHAP method was used to explain the contribution of individual poverty indicators to the IWI changes. shows all the indicators and their SHAP values. These metrics were ranked from top to bottom along the y-axis according to their contribution to the IWI variation in the RFR model. The colors represent the values of the metric, and the x-axis of the plot shows the distribution of the SHAP values of the fishnet grid cells. The results showed that NTL_MEAN, near_urban, and NTL_MAX had the greatest impact on changes in the IWI. NTL_MEAN and NTL_MAX reflect the intensity of human activity in the region, whereas near_urban represents the distance from the city. This suggests that higher levels of human activity and closer proximity to urban centers correlate with more significant changes in the IWI. This feature distribution is similar to the factor importance analysis of the RFR model, where NTL_MEAN and NTL_MAX also had the most significant influence on IWI. This finding confirms that NTL reflects socioeconomic status to some extent. Other indicators, such as per capita national income, average slope within the grid, and distance to the nearest city, also serve as indicators of household wealth.
4.2. Global IWI map
show the global distribution map of IWI for human settlements in low- and middle-income countries from 2005 to 2020. Regions with a low IWI (IWI ≤ 30) are mainly located in Sub-Saharan Africa, South Asia, the northwestern part of Latin America, and some Caribbean regions. These areas exhibit relatively low levels of economic development and are mostly low-income countries. Conversely, areas with a high IWI (IWI ≥ 60) are mainly found in West Asia, North Africa, and European regions along the Mediterranean coast. Similarly, high IWI results were observed in some cities or regions experiencing rapid economic development, such as Beijing, Shanghai, and the Pearl River Delta in China, New Delhi in India, and Brasilia and São Paulo in Brazil. The middle IWI category (IWI > 30 and < 60) is the most widely distributed wealth index. It is widely found in lower-to-middle-income countries. They are mostly concentrated in South Asia, southeastern Latin America, and Eastern Europe.
Figure 4. Global human settlements IWI distribution map for low- and middle-income countries in 2005.

Figure 5. Global human settlements IWI distribution map for low- and middle-income countries in 2010.

Figure 6. Global human settlements IWI distribution map for low- and middle-income countries in 2015.

Figure 7. Global human settlements IWI distribution map for low- and middle-income countries in 2020.

The global trend in the IWI indicates a significant decrease in low IWI values and an increase in high IWI values. shows the changes in area and population percentages of the IWI from 2005 to 2020. It is evident that the percentage of area and population with IWI ≤ 50 has decreased. Specifically, the area and population percentages decreased from 71.55% and 47.30% in 2005 to 62.70% and 31.13% in 2020. This represents a decrease of 8.85% in area and 16.17% in population. Areas with IWI > 50 exhibited a higher population density, indicating a noticeable trend of population clustering toward higher IWI values. Two notable points should be emphasized. First, Africa remains the main region facing significant poverty challenges, with relatively small changes over time compared with other continents. Second, Asian countries, especially China and India, have seen significant increases in their wealth indices, indicating positive outcomes of their poverty alleviation policies.
The main spatial variation in global poverty is around urban settlements, particularly in middle-income countries such as China, India, and Brazil. Conversely, there is less variation in poverty in rural areas that are far from cities. For instance, the majority of the population in western China is dispersed and far from major cities, resulting in lower IWI growth between 2005 and 2020 compared with the east coast of China, where the population is concentrated and urbanization levels are higher.
4.3. Spatiotemporal variation of IWI in a typical region
To explain the detailed changes in the percentage of area at each IWI level, we selected three types of countries for exploration. China and Brazil are upper-middle-income countries, India and Kenya are lower-middle-income countries, and Uganda and Syria are low-income countries. illustrates the percentage distribution of the area with different IWI levels for each year.
illustrates a decreasing trend in the proportion of low IWI values (IWI ≤ 50 in China and Brazil, IWI ≤ 40 in India, IWI ≤ 30 in Kenya and IWI ≤ 20 in Uganda) from 2005 to 2020, while there is an increasing trend in the proportion of high IWI values (IWI > 50 in China and Brazil, IWI > 40 in India, IWI > 30 in Kenya, and IWI > 20 in Rwanda). Notably, changes in the IWI vary across countries, with low-income countries exhibiting relatively small changes. This phenomenon can be explained by the fact that low-income countries are often at a disadvantage in international trade due to their primary agricultural economies and weak industrial bases (Opoku and Yan Citation2019; World Bank Citation2023b). In addition, the economic development of these countries is influenced by the political environments in which they operate (Bataeva, Chaplaev, and Gachaev Citation2020).
In Syria, different patterns of the IWI were observed compared to those in other countries. From 2005 to 2010, there was a decreasing trend in the percentage of areas with IWI ≤ 40, while the percentage of areas with IWI > 40 showed an increasing trend. However, from 2010 to 2015, there was a notable increase in the percentage of areas with IWI ≤ 40, while the percentage of areas with IWI > 40 showed a decreasing trend. This slow development continued from 2015 to 2020. The outbreak of the Syrian civil war in 2011 is the main reason for this phenomenon. The conflict severely impacted the Syrian economy, resulting in a downward trend in the IWI from 2010 to 2015, followed by slow development from 2015 to 2020 (Kešeljević and Spruk Citation2023; World Bank Citation2023b).
China, one of the world's most populous countries with extensive human settlements, has experienced significant changes in the IWI since 2005. The percentage of IWI values in the range of (30–40] decreased significantly from 10.38% in 2005 to 4.73% in 2020, representing a reduction of 5.65%. Similarly, the percentage of IWI values in the range of 40–50 also decreased significantly, from 29.34% in 2005 to 21.87% in 2020, a reduction of 7.47%. The IWI values in the 60–70 and 70–80 ranges increased significantly from 18.94% and 9.71% in 2005 to 23.89% and 17.24% in 2020, respectively. This represents a significant increase of 4.95% in the 60–70 range and 7.53% in the 70–80 range. The data indicate a rapid decline in areas with a low wealth index and a significant increase in areas and populations with a high wealth index. These trends can be attributed to the successful implementation of China’s poverty alleviation and reduction policies (Liu et al. Citation2020).
Economic development often leads to changes in the locations and sizes of human settlements. The most significant changes in the IWI occurred in cities that serve as primary human settlements. To further illustrate the changes in the IWI over time at the pixel scale, four major urban clusters were selected to represent upper-middle-income, lower-middle-income, low-income, and conflict-affected countries. The selected urban areas were São Paulo and Rio de Janeiro (Brazil), Kolkata (India), Kampala (Uganda), and Aleppo (Syria), as shown in . The wealth indices of cities and urban areas (except Syria) showed an upward trend over time. The main trend was that the IWI in the main urban areas remained stable, while the IWI around the cities showed an upward trend, indicating a significant urban effect. This suggests that areas closer to cities generally have better transportation, access to resources, and employment opportunities than areas farther away. Consequently, these areas have experienced faster economic development and tended to be wealthier. In the case of Syria, which was impacted by the conflict, there was a significant decrease in the IWI range in 2015, with some improvements observed in 2020. This phenomenon is consistent with the changes in the percentage of the IWI area in Syria, as shown in .
The changes in China's IWI from 2005 to 2020 are shown in , highlighting significant shifts, primarily within the interval of (40–80]. During this period, the IWI values shifted from low to high, with notable changes occurring in the (60–70] range. Specifically, 17.82%, 26.42%, and 21.53% of the area within the (60–70] values transitioned to (70–80] during the periods 2005–2010, 2010–2015, and 2015–2020, respectively. Similarly, the IWI values shifted from high to low, with notable changes occurring in the (70–80] range. 20.77%, 30.39%, and 19.50% of the area within the (70–80] values transitioned to (60–70] during the periods 2005–2010, 2010–2015, and 2015–2020, respectively. However, the overall IWI trend in China shifted from low to high from 2005 to 2020.
This situation can be explained by the fact that China has experienced sustained economic growth, and the government has implemented a series of poverty alleviation policies and programs (Liu et al. Citation2020) that have provided the poor with more employment opportunities and sources of income and gradually established a sound social security system. However, rapid economic development has attracted a large number of workers to move to developed regions, leading to a decline in the population of the areas from which they have moved, affecting the local labor market and economic vitality, which may lead to a shrinking of the local market, affecting the survival and development of local enterprises, and consequently leading to a decline in the level of prosperity.
5. Discussion
5.1. Poverty changes in Africa
Africa, particularly sub-Saharan Africa, is one of the largest regions affected by poverty in the world. Many scholars have conducted studies on poverty in this area at different spatial resolutions and scales (Imran, Stein, and Zurita-Milla Citation2014; Jean et al. Citation2016; Smits and Steendijk Citation2015; Lin et al. Citation2022; Yeh et al. Citation2020). According to the World Bank (Citation2023c), approximately 60% of the global population living in extreme poverty lived in sub-Saharan Africa in 2019, based on an international poverty line of $2.15. In fact, since 2011, sub-Saharan Africa has been the region of the world with the largest and growing share of people living in extreme poverty. This phenomenon is confirmed in . In addition, areas with faster IWI growth in sub-Saharan Africa are mainly coastal countries and regions, whereas the interior of the continent has experienced relatively smaller changes. This can be attributed to several factors. First, Sub-Saharan Africa’s remote geographical location from major world economic centers makes economic exchange challenging. Second, the levels of industry and infrastructure limit economic development (Malah Kuete and Asongu Citation2023; Opoku and Yan Citation2019). The region relies heavily on exporting primary products, such as minerals or agricultural products, owing to its low levels of industrialization and poor infrastructure. Finally, social environments are complex. Armed conflicts, diseases, and other factors are also important reasons that hinder economic development (Bataeva, Chaplaev, and Gachaev Citation2020; United Nations Citation2023b).
5.2. Advantages of global poverty estimation models
Compared with previous studies, this study demonstrated several improvements. First, the accuracy of the model used in this study is acceptable and robust. The global model accuracy, as measured by the R2 and OOB accuracies, was 0.74. These results were consistently observed during the validation of model accuracy for the long time series. Based on a summary of the existing literature, small-scale poverty measurement studies tend to exhibit higher model accuracy than their large-scale counterparts (Hu et al. Citation2022; Lee and Braithwaite Citation2022; Li et al. Citation2020; Shao and Li Citation2023; Yin, Qiu, and Zhang Citation2020; Yu et al. Citation2015; Zhao et al. Citation2019). This is primarily because small-scale studies use region-specific variables to improve the overall accuracy of models. The accuracy of our study aligns with these findings and is comparable to that of other large-scale poverty studies (Chi et al. Citation2022; Elvidge et al. Citation2009; Jean et al. Citation2016; Lee and Braithwaite Citation2022; Yeh et al. Citation2020). However, most previous studies were single-phase studies, with few long-term phase studies. Furthermore, in the data input section, this study reduced the amount of input data while maintaining accuracy, resulting in decreased dependence on the input data compared to previous methods (Zhao et al. Citation2019).
5.3. Limitations and future work
This study has some limitations. First, household survey data published by the DHS were initially divided into two categories: urban and rural. However, in this study, we did not distinguish between urban and rural samples, and instead analyzed them collectively. This approach was adopted to ensure that both types of samples were used for model construction and poverty estimation to provide a more comprehensive representation of the overall poverty situation within a country.
Another challenge is that the spatial resolution of this study was 10 km × 10 km. Although this resolution is suitable for analyzing large-scale time-series changes, it has limitations in accurately reflecting detailed poverty information within cities and small areas. The emergence of novel data sources such as call detail records (CDRs), smartphone metadata, and Wi-Fi connectivity has expanded the scope of precise poverty estimation. Moreover, the widespread adoption of deep learning models offers novel approaches to enhance the timeliness and accuracy of poverty estimates (Ziulu et al. Citation2022).
Additionally, ignoring the population structure and the impact of survey weights attached to units in the survey data may lead to unexpected errors in poverty estimation. In small-area poverty studies, indicators from survey data are usually assigned different weights to better reflect poverty levels. Furthermore, population structure (e.g. age and sex) and household size are recognized as being strictly connected to poverty. However, these factors were not adequately accounted for in the model used in this study when making large-scale poverty estimates utilizing survey data, which may have led to biased estimation results. WorldPop provides estimates of the population size stratified by sex and age, which can be used to correct future biases (WorldPop Citation2023).
6. Conclusion
In this study, we utilized an RFR model based on multi-source data, including household survey data, land-use data, NPP-VIIRS-like data, DEM, and World Bank statistical data, to generate a time-series spatial distribution map of the IWI for global low- and middle-income countries at a 10 km resolution spanning from 2005 to 2020. The IWI serves as an indicator of poverty. The results indicate that the global model exhibits higher accuracy and out-of-bag (OOB) scores for both at 0.74 for R² and OOB, suggesting the development of a highly accurate wealth index estimation method. Based on the established model, we analyzed the changing trends in the IWI of typical countries at different scales. Overall, there is a global decrease in the proportion of areas and populations with an IWI ≤ 50, with China and India showing the largest declines. In addition, changes in the IWI at the pixel scale indicate that the IWI growth rate is higher in areas closer to cities and that armed conflicts have a significant impact on changes in the IWI.
This global low- and middle-income country time-series wealth index product can contribute to achieving SDGs and conducting global poverty research at various scales, including the distribution of impoverished populations, estimation of poverty headcounts, and strategic evaluation of poverty eradication efforts.
Author contributions
Yangguang Li, Bin Wu, Congxiao Wang, Zuoqi Chen, Bailang Yu conceived and designed the study. Yangguang Li and Shaoyang Liu contributed to the conception of the study, and performed the data analysis. Yangguang Li, wrote the paper. Bin Wu, Congxiao Wang, Zuoqi Chen, Bailang Yu, and Shaoyang Liu reviewed and edited the manuscript. All authors read and approved the manuscript.
Disclosure statement
No potential conflict of interest was reported by the author(s).
Data availability statement
The time series (2005–2020) of international wealth index data in the Mollweide Projection with a spatial resolution of 10 km can be freely accessed at https://doi.org/10.7910/DVN/KEV9WN
(Li et al. 2023), which is stored as a zip file (∼2 MB) for each year. By uncompressing the zip file, the annual IWI data are provided in GeoTIFF format (∼28 MB). These data can be processed using open-source software such as QGIS.Additional information
Funding
References
- Andreano, Maria Simona, Roberto Benedetti, Federica Piersimoni, and Giovanni Savio. 2020. “Mapping Poverty of Latin American and Caribbean Countries from Heaven Through Night-Light Satellite Images.” Social Indicators Research 156 (2–3): 533–562. https://doi.org/10.1007/s11205-020-02267-1.
- Barnett, Matthew J., Douglas Jackson-Smith, Joanna Endter-Wada, and Melissa Haeffner. 2020. “A Multilevel Analysis of the Drivers of Household Water Consumption in a Semi-Arid Region.” Science of the Total Environment 712: 136489. https://doi.org/10.1016/j.scitotenv.2019.136489.
- Bataeva, Patimat, Hussein Chaplaev, and Ahmed Gachaev. 2020. “The Impact of Local Armed Conflicts on the Economic Performance of Countries in 1990–2019.” Economic Annals-XXI 182 (3–4): 41–48. https://doi.org/10.21003/ea.V182-05.
- Bishop, Christopher M. 1995. Neural Networks for Pattern Recognition. New York: Oxford university press.
- Blumenstock, Joshua, Gabriel Cadamuro, and Robert On. 2015. “Predicting Poverty and Wealth from Mobile Phone Metadata.” Science 350 (6264): 1073–1076. https://doi.org/10.1126/science.aac4420.
- Bruederle, Anna, and Roland Hodler. 2018. “Nighttime Lights as a Proxy for Human Development at the Local Level.” PLoS One 13 (9): e0202231. https://doi.org/10.1371/journal.pone.0202231.
- Chen, Zuoqi, Bailang Yu, Chengshu Yang, Yuyu Zhou, Shenjun Yao, Xingjian Qian, Bin CongxiaoWang, and JianpingWu. Wu. 2021. “An Extended Time Series (2000–2018) of Global NPP-VIIRS-Like Nighttime Light Data from a Cross-Sensor Calibration.” Earth System Science Data 13 (3): 889–906. https://doi.org/10.5194/essd-13-889-2021.
- Chi, Guanghua, Han Fang, Sourav Chatterjee, and Joshua E Blumenstock. 2022. “Microestimates of Wealth for all low-and Middle-Income Countries.” Proceedings of the National Academy of Sciences 119 (3): e2113658119. https://doi.org/10.1073/pnas.2113658119.
- Cortes, Corinna, and Vladimir Vapnik. 1995. “Support-Vector Networks.” Machine Learning 20: 273–297. https://doi.org/10.1007/Bf00994018.
- De Nicolò, Silvia, Enrico Fabrizi, and Aldo Gardini. 2022. “Extended Beta Models for Poverty Mapping. An Application Integrating Survey and Remote Sensing Data in Bangladesh.” 10.6092/unibo/amsacta/7074. https://amsacta.unibo.it/id/eprint/7074.
- Duque, Juan C, Jorge E Patino, Luis A Ruiz, and Josep E Pardo-Pascual. 2015. “Measuring Intra-Urban Poverty Using Land Cover and Texture Metrics Derived from Remote Sensing Data.” Landscape and Urban Planning 135: 11–21. https://doi.org/10.1016/j.landurbplan.2014.11.009.
- Elvidge, Christopher D., Paul C. Sutton, Tilottama Ghosh, Benjamin T. Tuttle, Kimberly E. Baugh, Budhendra Bhaduri, and Edward Bright. 2009. “A Global Poverty map Derived from Satellite Data.” Computers & Geosciences 35 (8): 1652–1660. https://doi.org/10.1016/j.cageo.2009.01.009.
- Gouveia, João Pedro, Júlia Seixas, and Gavin Long. 2018. “Mining Households’ Energy Data to Disclose Fuel Poverty: Lessons for Southern Europe.” Journal of Cleaner Production 178: 534–550. https://doi.org/10.1016/j.jclepro.2018.01.021.
- Han, Peng, Qing Zhang, Yanyun Zhao, and Frank Yonghong Li. 2021. “High-resolution Remote Sensing Data Can Predict Household Poverty in Pastoral Areas, Inner Mongolia, China.” Geography and Sustainability 2 (4): 254–263. https://doi.org/10.1016/j.geosus.2021.10.002.
- Henderson, J. Vernon, Adam Storeygard, and David N. Weil. 2012. “Measuring Economic Growth from Outer Space.” American economic review 102 (2): 994–1028. https://doi.org/10.1257/aer.102.2.994.
- Hu, Shan, Yong Ge, Mengxiao Liu, Zhoupeng Ren, and Xining Zhang. 2022. “Village-level Poverty Identification Using Machine Learning, High-Resolution Images, and Geospatial Data.” International Journal of Applied Earth Observation and Geoinformation 107: 102694. https://doi.org/10.1016/j.jag.2022.102694.
- Imran, Muhammad, Alfred Stein, and Raúl Zurita-Milla. 2014. “Investigating Rural Poverty and Marginality in Burkina Faso Using Remote Sensing-Based Products.” International Journal of Applied Earth Observation and Geoinformation 26: 322–334. https://doi.org/10.1016/j.jag.2013.08.012.
- Jean, Neal, Marshall Burke, Michael Xie, W. Matthew Davis, David B. Lobell, and Stefano Ermon. 2016. “Combining Satellite Imagery and Machine Learning to Predict Poverty.” Science 353 (6301): 790–794. https://doi.org/10.1126/science.aaf7894.
- Joshua Evan, Blumenstock 2016. “Fighting Poverty with Data.” Science 353 (6301): 753–754. https://doi.org/10.1126/science.aah5217.
- Kešeljević, Aleksandar, and Rok Spruk. 2023. “Estimating the Effects of Syrian Civil War.” Empirical Economics:1–33. https://doi.org/10.1007/s00181-023-02470-2.
- LeCun, Yann, Yoshua Bengio, and Geoffrey Hinton. 2015. “Deep Learning.” Nature 521 (7553): 436–444. https://doi.org/10.1038/nature14539.
- Lee, Kamwoo, and Jeanine Braithwaite. 2022. “High-resolution Poverty Maps in Sub-Saharan Africa.” World Development 159: 106028. https://doi.org/10.1016/j.worlddev.2022.106028.
- Lerman, P. M. 1980. “Fitting Segmented Regression Models by Grid Search.” Journal of the Royal Statistical Society Series C: Applied Statistics 29 (1): 77–84. https://doi.org/10.2307/2346413.
- Li, Guie, Zhongliang Cai, Xiaojian Liu, Ji Liu, and Shiliang Su. 2019. “A Comparison of Machine Learning Approaches for Identifying High-Poverty Counties: Robust Features of DMSP/OLS Night-Time Light Imagery.” International Journal of Remote Sensing 40 (15): 5716–5736. https://doi.org/10.1080/01431161.2019.1580820.
- Li, Guie, Liyun Chang, Xiaojian Liu, Shiliang Su, Zhongliang Cai, Xinran Huang, and Bozhao Li. 2019. “Monitoring the Spatiotemporal Dynamics of Poor Counties in China: Implications for Global Sustainable Development Goals.” Journal of Cleaner Production 227: 392–404. https://doi.org/10.1016/j.jclepro.2019.04.135.
- Li, Chengsong, Wunian Yang, Qiaolin Tang, Xiaolu Tang, Junjie Lei, Mingyan Wu, and Shuyue Qiu. 2020. “Detection of Multidimensional Poverty Using Luojia 1-01 Nighttime Light Imagery.” Journal of the Indian Society of Remote Sensing 48 (7): 963–977. https://doi.org/10.1007/s12524-020-01126-3.
- Lin, Yi, Tinghui Zhang, Xuanqi Liu, Jie Yu, Jonathan Li, and Kyle Gao. 2022. “Dynamic Monitoring and Modeling of the Growth-Poverty-Inequality Trilemma in the Nile River Basin with Consistent Night-Time Data (2000–2020).” International Journal of Applied Earth Observation and Geoinformation 112: 102903. https://doi.org/10.1016/j.jag.2022.102903.
- Liu, Mingyue, Xiaolong Feng, Sangui Wang, and Huanguang Qiu. 2020. “China’s Poverty Alleviation Over the Last 40 Years: Successes and Challenges.” Australian Journal of Agricultural and Resource Economics 64 (1): 209–228. https://doi.org/10.1111/1467-8489.12353.
- Malah Kuete, Yselle F., and Simplice A. Asongu. 2023. “Infrastructure Development as a Prerequisite for Structural Change in Africa.” Journal of the Knowledge Economy 14 (2): 1386–1412. https://doi.org/10.1007/s13132-022-00989-w.
- Masaki, Takaaki, David Newhouse, Ani Rudra Silwal, Adane Bedada, and Ryan Engstrom. 2022. “Small Area Estimation of non-Monetary Poverty with Geospatial Data.” Statistical Journal of the IAOS 38 (3): 1035–1051. https://doi.org/10.3233/SJI-210902.
- Newhouse, David Locke, Joshua D. Merfeld, Anusha Ramakrishnan, Tom Swartz, and Partha Lahiri. 2022. “Small Area Estimation of Monetary Poverty in Mexico Using Satellite Imagery and Machine Learning.” Available at SSRN 4235976. https://doi.org/10.2139/ssrn.4235976.
- Ni, Ye, Xutao Li, Yunming Ye, Yan Li, Chunshan Li, and Dianhui Chu. 2020. “An Investigation on Deep Learning Approaches to Combining Nighttime and Daytime Satellite Imagery for Poverty Prediction.” IEEE Geoscience and Remote Sensing Letters, 1–5. https://doi.org/10.1109/LGRS.2020.3006019.
- Niu, Tong, Yimin Chen, and Yuan Yuan. 2020. “Measuring Urban Poverty Using Multi-Source Data and a Random Forest Algorithm: A Case Study in Guangzhou.” Sustainable Cities and Society 54: 102014. https://doi.org/10.1016/j.scs.2020.102014.
- Noor, Abdisalan M., Victor A. Alegana, Peter W. Gething, Andrew J. Tatem, and Robert W. Snow. 2008. “Using Remotely Sensed Night-Time Light as a Proxy for Poverty in Africa.” Population Health Metrics 6 (1): 1–13. https://doi.org/10.1186/1478-7954-6-5.
- Opoku, Eric Evans Osei, and Isabel Kit-Ming Yan. 2019. “Industrialization as Driver of Sustainable Economic Growth in Africa.” The Journal of International Trade & Economic Development 28 (1): 30–56. https://doi.org/10.1080/09638199.2018.1483416.
- Perez, Anthony, Christopher Yeh, George Azzari, Marshall Burke, David Lobell, and Stefano Ermon. 2017. “Poverty prediction with public landsat 7 satellite imagery and machine learning.” arXiv preprint arXiv:1711.03654. https://doi.org/10.48550/arXiv.1711.03654.
- Permatasari, Novia, Bagaskoro Cahyo Laksono, and Azka Ubaidillah. 2023. “Small Area Estimation of Poverty using Remote Sensing Data (Case Study: Expenditure Per Capita Estimation of Very Poor Households in West Java, Indonesia).” Proc. Of ISI World Statistics Congress (WSC), International Statistical Institute, Ottawa, Canada. https://www.isi-next.org/media/abstracts/ottawa-2023_ca3836ef5000fd509ab002bf356175c1.pdf.
- Pokhriyal, Neeti, and Damien Christophe Jacques. 2017. “Combining Disparate Data Sources for Improved Poverty Prediction and Mapping.” Proceedings of the National Academy of Sciences 114 (46): E9783–E9792. https://doi.org/10.1073/pnas.1700319114.
- Putri, Salwa Rizqina, Arie Wahyu Wijayanto, and Setia Pramana. 2023. “Multi-Source Satellite Imagery and Point of Interest Data for Poverty Mapping in East Java, Indonesia: Machine Learning and Deep Learning Approaches.” Remote Sensing Applications: Society and Environment 29: 100889. https://doi.org/10.1016/j.rsase.2022.100889.
- Puttanapong, Nattapong, Nutchapon Prasertsoong, and Wichaya Peechapat. 2023. “Predicting Provincial Gross Domestic Product using Satellite Data and Machine Learning Methods: A Case Study of Thailand.” Asian Development Review 40 (02): 39–85. https://doi.org/10.1142/S0116110523400024.
- Ratledge, Nathan, Gabe Cadamuro, Brandon de la Cuesta, Matthieu Stigler, and Marshall Burke. 2022. “Using Machine Learning to Assess the Livelihood Impact of Electricity Access.” Nature 611 (7936): 491–495. https://doi.org/10.1038/s41586-022-05322-8.
- Ridho, Helen Cantika, Laura Aisyatul, and Rindang Bangun Prasetyo. 2023. “Small Area Estimation of Multidimensional Poverty in East Java Province using Satellite Imagery.” Proceedings of The International Conference on Data Science and Official Statistics, https://doi.org/10.34123/icdsos.v2023i1.417.
- Rutstein, Shea Oscar, and Sarah Staveteig. 2014. Making the Demographic and Health Surveys Wealth Index Comparable. Vol. 9. Rockville, MD: ICF International.
- Sandefur, Justin, and Amanda Glassman. 2015. “The Political Economy of Bad Data: Evidence from African Survey and Administrative Statistics.” The Journal of Development Studies 51 (2): 116–132. https://doi.org/10.1080/00220388.2014.968138.
- Schmid, Timo, Fabian Bruckschen, Nicola Salvati, and Till Zbiranski. 2017. “Constructing Sociodemographic Indicators for National Statistical Institutes by Using Mobile Phone Data: Estimating Literacy Rates in Senegal.” Journal of the Royal Statistical Society Series A: Statistics in Society 180 (4): 1163–1190. https://doi.org/10.1111/rssa.12305.
- Serajuddin, Umar, Hiroki Uematsu, Christina Wieser, Nobuo Yoshida, and Andrew Dabalen. 2015. “Data Deprivation: Another Deprivation to End.” World Bank Policy Research Working Paper (7252). https://ssrn.com/abstract=2600334.
- Shao, Zixuan, and Xi Li. 2023. “Multi-scale Estimation of Poverty Rate Using Night-Time Light Imagery.” International Journal of Applied Earth Observation and Geoinformation 121: 103375. https://doi.org/10.1016/j.jag.2023.103375.
- Shi, Kaifang, Zhijian Chang, Zuoqi Chen, Jianping Wu, and Bailang Yu. 2020. “Identifying and Evaluating Poverty Using Multisource Remote Sensing and Point of Interest (POI) Data: A Case Study of Chongqing, China.” Journal of Cleaner Production 255. https://doi.org/10.1016/j.jclepro.2020.120245.
- Smits, Jeroen, and Roel Steendijk. 2015. “The International Wealth Index (IWI).” Social Indicators Research 122 (1): 65–85. https://doi.org/10.1007/s11205-014-0683-x.
- Smythe, Isabella S., and Joshua E. Blumenstock. 2022. “Geographic Microtargeting of Social Assistance with High-Resolution Poverty Maps.” Proceedings of the National Academy of Sciences 119 (32): e2120025119. https://doi.org/10.1073/pnas.2120025119.
- Tian, Fuyou, Bingfang Wu, Hongwei Zeng, Gary R. Watmough, Miao Zhang, and Yurui Li. 2022. “Detecting the Linkage Between Arable Land use and Poverty Using Machine Learning Methods at Global Perspective.” Geography and Sustainability 3 (1): 7–20. https://doi.org/10.1016/j.geosus.2022.01.001.
- United Nations. 2023a. “United Nations Sustainable Development Goals(SDG).” Accessed August 28, 2023a. https://www.un.org/sustainabledevelopment/sustainable-development-goals/.
- United Nations. 2023b. “Economic Development in Africa Report 2021.” August 28, 2023b. https://unctad.org/publication/economic-development-africa-report-2021.
- Wang, Wen, Hui Cheng, and Li Zhang. 2012. “Poverty Assessment using DMSP/OLS Night-Time Light Satellite Imagery at a Provincial Scale in China.” Advances in Space Research 49 (8): 1253–1264. https://doi.org/10.1016/j.asr.2012.01.025.
- Watmough, Gary R., Charlotte L. J. Marcinko, Clare Sullivan, Kevin Tschirhart, Patrick K. Mutuo, Cheryl A. Palm, and Jens-Christian Svenning. 2019. “Socioecologically Informed use of Remote Sensing Data to Predict Rural Household Poverty.” Proceedings of the National Academy of Sciences 116 (4): 1213–1218. https://doi.org/10.1073/pnas.1812969116.
- Weiss, D. J., A. Nelson, H. S. Gibson, W. Temperley, S. Peedell, A. Lieber, M. Hancher, et al. 2018. “A Global Map of Travel Time to Cities to Assess Inequalities in Accessibility in 2015.” Nature 553 (7688): 333–336. https://doi.org/10.1038/nature25181.
- World Bank. 2023a. “World Bank Open Data.” Accessed August 28, 2023a. https://data.worldbank.org.cn/indicator/SI.POV.DDAY.
- World Bank. 2023b. “World Bank Open Data.” Accessed August 28, 2023b. https://data.worldbank.org.cn/.
- World Bank. 2023c. “Poverty and Inequality Platform.” Accessed August 28, 2023c. https://pip.worldbank.org.
- Worldpop. 2023. “Age and Sex Structres.” Accessed March 12, 2023 https://hub.worldpop.org/geodata/listing?id=65.
- Xu, Jianbin, Jie Song, Baochao Li, Dan Liu, and Xiaoshu Cao. 2021. “Combining Night Time Lights in Prediction of Poverty Incidence at the County Level.” Applied Geography 135: 102552. https://doi.org/10.1016/j.apgeog.2021.102552.
- Yao, Yao, Jianfeng Zhou, Zhenhui Sun, Qingfeng Guan, Zhiqiang Guo, Yin Xu, Jinbao Zhang, Ye Hong, Yuyang Cai, and Ruoyu Wang. 2023. “Estimating China’s Poverty Reduction Efficiency by Integrating Multi-Source Geospatial Data and Deep Learning Techniques.” Geo-spatial Information Science, 1–17. https://doi.org/10.1080/10095020.2023.2165975.
- Yeh, Christopher, Anthony Perez, Anne Driscoll, George Azzari, Zhongyi Tang, David Lobell, Stefano Ermon, and Marshall Burke. 2020. “Using Publicly Available Satellite Imagery and Deep Learning to Understand Economic Well-Being in Africa.” Nature Communications 11 (1): 2583. https://doi.org/10.1038/s41467-020-16185-w.
- Yin, Jian, Yuanhong Qiu, and Bin Zhang. 2020. “Identification of Poverty Areas by Remote Sensing and Machine Learning: A Case Study in Guizhou, Southwest China.” ISPRS International Journal of Geo-Information 10 (1), https://doi.org/10.3390/ijgi10010011.
- Yu, Bailang, Kaifang Shi, Yingjie Hu, Chang Huang, Zuoqi Chen, and Jianping Wu. 2015. “Poverty Evaluation Using NPP-VIIRS Nighttime Light Composite Data at the County Level in China.” IEEE Journal of Selected Topics in Applied Earth Observations and Remote Sensing 8 (3): 1217–1229. https://doi.org/10.1109/jstars.2015.2399416.
- Zhao, Xizhi, Bailang Yu, Yan Liu, Zuoqi Chen, Qiaoxuan Li, Congxiao Wang, and Jianping Wu. 2019. “Estimation of Poverty Using Random Forest Regression with Multi-Source Data: A Case Study in Bangladesh.” Remote Sensing 11 (4): 375–393. https://doi.org/10.3390/rs11040375.
- Zhou, Yang, and Yansui Liu. 2022. “The Geography of Poverty: Review and Research Prospects.” Journal of Rural Studies 93: 408–416. https://doi.org/10.1016/j.jrurstud.2019.01.008.
- Ziulu, Virginia, Jessica Meckler Gonzalo, Hernández Licona, and Jozef Vaessen. 2022. “Poverty Mapping: Innovative Approaches to Creating Poverty Maps with New Data Sources.” In IEG Methods and Evaluation Capacity Development Working Paper Series. Independent Evaluation Group. Washington, DC: World Bank. https://ieg.worldbankgroup.org/evaluations/poverty-mapping-innovative-approaches-creating-poverty-maps-new-data-sources.