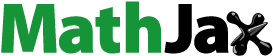
ABSTRACT
Soybean (Glycine max) is the most important plant protein source, and Fall Armyworm (FAW, Spodoptera frugiperda) is considered a major pest. This study aimed to examine the impact of FAW feeding on soybean accessions that vary in their water use efficiency (WUE) traits, by examining FAW growth and life history parameters along with plant growth response to pest damage. Soybean accessions were grown in a greenhouse and exposed to FAW larval feeding for 48 h at three different soybean growth stages: V3, R3, and R6. The growth and development of the FAW and soybeans were monitored. Results showed that faster wilting soybean accessions grow taller and have more leaves than slower wilting accessions, but yield was higher in slower wilting soybean accessions. FAW experienced the highest mortality on mid-stage (R3) soybean plants, but they gained the least mass on early stage (V3) soybean plants. These results can assist in better understanding plant insect-interactions at different life stages in both soybean and FAW with implications for management.
1. Introduction
Soybean [Glycine max (L.) Merr.] is one of the most important agricultural crops in the world, and it has multiple roles in the agricultural industry. Soybeans serve as a major source of protein for humans and livestock alike and account for 90% of U.S. oilseed production [Citation1,Citation2]. In 2022 alone, growers planted 87.2 million acres of soybean, which is equivalent to about $45.7 billion in revenue once harvested [Citation3]. One of the major limiting factors of soybean yield is drought stress, which contributes to 80% of total yield loss when inflicted during its reproductive phase [Citation4–6]. Drought stress also impacts the number and quality of the seeds produced, causing some seeds to have a tough, impermeable seed coating that impedes the seeds’ ability to germinate [Citation7,Citation8]. Additionally, there is a significant reduction in the overall nutritional value of the seeds yielded from soybeans that experience drought stress [Citation9].
Out of all major field crops, soybean is one of the most sensitive to drought, and while a structured watering schedule with the use of irrigation is a seemingly simple solution, less than 15% of farmland in the United States is irrigated [Citation10,Citation11]. Global temperatures are steadily rising with record-breaking summer heat waves, and water has become a finite resource, making Water Use Efficiency (WUE) an obvious point of concern [Citation12]. WUE is determined by the amount of yield produced per unit of water [Citation13]. Annually, 30% of the world’s population faces extreme water scarcity, in 2021 the amount of the world’s population facing extreme water scarcity to 40% [Citation14]. As the competition for water between human and agricultural resources grows fierce, it is extremely important to explore alternative methods of increasing overall water productivity or water use efficiency. In general, soybean plants are drought intolerant, and as global temperatures rise and water becomes scarcer, the potential impact on their yield becomes a major concern. As such, researchers have turned their attention to developing soybean cultivars that maximize yield while also exhibiting traits that are more drought tolerant [Citation15].
Soybeans exhibit a wide variation of drought tolerance and WUE traits [Citation10]. While WUE is a trait that can be screened for, it is extremely expensive to do so on the scale necessary for plant breeders to meet the current demand for soybean production [Citation16]. In order to save time and money, plant breeders look at traits associated with WUE and drought tolerance via Genome Wide Associated Mapping (GWAM) and focus on introducing accessions with these traits instead. Canopy wilting is a highly valuable and accessible trait in soybean screening that can be indicative of both WUE and drought tolerance – it is also one of the first visual cues growers will notice when their plants are under drought stress [Citation12,Citation17,Citation18]. It requires no additional equipment – especially in less developed countries where access to other methods of screening is not feasible due to limitations associated with cost, time, or training [Citation19].
Slow wilting canopies in soybeans are characterized by the conservation of soil moisture through decreased hydraulic conductance and decreased transpiration, thereby increasing WUE, which in turn maintains the appearance of healthy leaves [Citation12,Citation18,Citation20,Citation21]. Simulation models predict that through increased introduction of the slow canopy wilting trait, soybeans can reduce yield loss despite drought stress by more than 80%- increasing its significance when screening drought tolerance in the field [Citation22–24]. Canopy wilting as a marker of plants with higher WUE traits and drought tolerance is an important aspect in gaining a further understanding of the relationship with soybeans, their genetics, and how they interact with the ecosystem.
In addition to the impact on fitness, rising temperatures at local and global scales and an increase in drought frequency can, in turn, increase the severity and frequency of insect pest herbivory and infestations [Citation25–28]. Some insect pests, especially generalists, thrive on drought stressed plants and experience an increase in relative size and population growth [Citation29,Citation30]. Collectively, a combination of drought and herbivory can be highly detrimental to soybeans, resulting in severe yield loss. While WUE traits and their impact on drought tolerance are constantly monitored, there are still unanswered questions regarding the implications of these traits. Namely, how does the shift to soybeans with traits indicative of higher WUE and drought stress impact tolerance to pest damage, and how does it relates to fitness tradeoffs and physical and chemical defenses in plants. Physical defenses include waxes, spines, thorns, and trichomes [Citation31]. Waxes are found on the outermost layer of the cuticle and alter the feeding behavior of insect pests [Citation32]. Spines, thorns, and trichomes are similar in that they deter herbivory[Citation33]. Spines and thorns target larger herbivores – mainly mammals – while trichomes target insect herbivores such as lepidopteran larvae [Citation34,Citation35]). Trichomes are hair-like protrusions that are primarily divided into two groups: glandular and non-glandular [Citation31]. Non-glandular trichomes act as a purely physical defense blocking the insect pests from feeding while incurring damage [Citation36]. Glandular trichomes are a combination of chemical and physical defenses that release toxic substances when stimulated [Citation37]. Trichomes are not only a deterrent against insect herbivory but they can also indicate higher resistance to harsh ultraviolet rays [Citation31].
There is evidence to suggest that genotypes that express traits associated with drought tolerance have significantly different responses to herbivory compared to genotypes that do not express these traits [Citation38]. When insects fed on soybean genotypes that had slower canopy wilting, they gained significantly less mass than insects that fed on faster wilting genotypes [Citation38]. When choice assays were conducted, insects displayed a preference for fast wilting genotypes compared to slower wilting genotypes [Citation38]. This was largely dependent on the fact that when exposed to drought conditions, some soybean genotypes showed increased tolerance to pest damage, while others showed significantly decreased tolerance [Citation38].
Clearly, there is evidence to suggest that differing WUE traits and drought tolerance across genotypes have differential effects on insect herbivory, but also a significant difference between pests within the same species. While the interaction between slow canopy wilting traits and tolerance to pest damage traits is still relatively unexplored, there is evidence to suggest that there may be an intrinsic link between traits associated with WUE and drought tolerance, namely, canopy wilting and tolerance to pest damage.
To examine this in detail, we used fast and slow wilting soybean accessions and Fall Armyworm (FAW), a generalist lepidopteran herbivore. FAW is a polyphagous pest, originally native to the Americas, that has invaded Africa, Asia, the Middle East, and Australia [Citation3940Citation40. FAW have high fecundity and are highly mobile, they are a pest of over 186 plants, making it a prevalent pest in agriculture [Citation40,Citation41]. Moreover, it has been suggested that spray applications of pesticides against FAW are inefficient due to their high levels of pesticide resistance, as they are resistant to 47 active ingredients in pesticides [Citation42,Citation43. Cultural control of FAW in the form of intercropping with legume species such as Vicia faba (broad bean) and Medicago sativa (alfalfa) has been explored in maize and have been shown to reduce FAW herbivory through deterrence and attraction of natural predators and parasitoids [Citation44,Citation45]. FAW is predominantly a seasonal pest, but with climate change heating winter temperatures, we expect that their activity will be increased as well [Citation41]. Ideally, the impact of FAW herbivory will be softened through biological means, strengthening tolerance to pest damage traits found within plants to reduce the need for harsh chemical applications. Researchers have already identified how to breed for increased WUE traits in plants, but there is a gap in understanding how these traits influence plant–insect interactions.
Specifically, we asked the following questions: 1) Does the soybean growth stage influence FAW larvae development? 2) How does the canopy wilting rate influence FAW growth and development? 3) Are there cascading effects of the soybean growth stage on FAW pupae and/or adults? 3) What are the phenotypical differences between fast-wilting and slow-wilting genotypes?
To answer these questions, we examined plant traits and followed up with FAW feeding behavior as well as FAW growth and development post-feeding. We carried out these experiments on early, mid, and late-stage soybean plants (V3, R3, R6) from a total of 17 genotypes that vary in their wilting rates.
We hypothesized that since fast-wilting genotypes have been found to have better growth traits, they will also allocate more resources toward defenses. Consequently, we predicted that FAW would perform better on slow wilting genotypes. We also hypothesized that regardless of the wilting traits, early vegetative stage plants will have better defenses when compared to other stages of growth, with consequences for FAW.
2. Materials and methods
2.1. Study systems
2.1.1. Insect colony
For all experiments, FAW were purchased as eggs from a commercial vendor (Frontier Agricultural Services, Calexico, CA, USA). Closer to hatching, the color of the eggs changed from green to yellow and then to black. They were hatched at room temperature (21°C–24°C) and then transferred to plastic containers with an artificial diet prior to experimentation. FAW eggs were purchased prior to the soybean plants reaching each desired growth stage (V3, R3, R6) and reared to the second instar for all three infestation events.
2.2. Plant propagation
For all experiments, soybean genotypes were acquired through the GRIN database provided by the USDA. Soybeans were reared in a greenhouse at the University of Arkansas campus under 28°C-30°C temperatures and ~70% humidity conditions on a 16:8 light: dark cycle. Soybeans were fertilized with Osmocote Plus 15–9–12 (ICL Specialty Fertilizers, Summerville, SC, USA) twice a week after true leaves were present and received iron chelate micronutrient (Sprint 330 Chelated Iron 10%, Florham, Park, NJ, USA) every 2 weeks. Seventeen soybean genotypes were planted, with 18 plants per genotype. Not all genotypes germinated, and our analysis is based on 17 genotypes with a total of 177 plants.
2.3. Artificial diet
FAW larvae were reared on a wheat-germ-based artificial diet (Frontier Agricultural Services, Calexico, CA, USA). The diet was composed of 1000 ml water, 8 g agar, and 200 g of General Lepidopteran Diet and prepared as per the specifications of the manufacturer and our previous work [Citation46]. One thousand milliliters of water was placed on a hot plate, and 8 g of agar was added to it and stirred thoroughly. The water was boiled, and 200 g of artificial diet was added. This was mixed thoroughly to avoid clumps and allowed to cool to room temperature, allowing it to solidify before placing it in a refrigerator for storage.
2.4. Experiments
2.4.1. 48-h FAW damage exposure experiment
The growth stages of soybeans observed were vegetative stage 3 (V3), reproductive stage 3 (R3), and reproductive stage 7 (R7). For each stage, FAW larvae were reared on the artificial diet to the 2nd instar for them to reach a weighable mass. Second instar FAW larvae were weighed and then placed in mesh organza bags (Amazon, Seattle, WA, USA) that were then enclosed around a leaf on each plant and removed after 48 h (). The leaves selected were standardized as new, large, and fully developed leaves with no imperfections, mainly located toward the top of the soybean plant.
2.4.1.1. FAW life history parameters measured
The FAW larvae were allowed to feed on the leaves for 48 h and were removed and weighed. After they were weighed, the larvae were placed in petri dishes on an artificial diet as described previously and allowed to feed until pupation. Once they pupated, they were weighed again. After the pupae enclosed to adults, adult mass was also measured.
2.4.1.2. Plant traits measured
After each application of FAW larvae, a damage assessment was conducted on the leaves that FAW fed on. Leaf damage was determined on a scale of 0 to 4, pictured in , of which 0 means no damage and 4 means complete defoliation[Citation47]. In addition to the damage assessment, plant height data and the number of leaves on each plant were recorded to gain insight into how herbivory impacted the growth of soybeans and to compare phenotypic traits of fast-wilting and slow wilting soybean genotypes. Plant height was measured in centimeters from the plant apex to the stem above the soil level. The number of leaves was determined by counting only the fully opened, trifoliate clusters of leaves.
2.5. Trichome density
To assess trichome density across genotypes, we collected four leaves from each plant, ensuring that we were careful to select newer, fully developed leaves located toward the top of the plant. The leaves were hole punched with a 16 mm hole punch and then placed on a microscope slide and covered with a coverslip and analyzed under 10× magnification on a stereo microscope (Zeiss; Oberkochen, Germany). The total area viewed on each leaf was 0.86 mm2. Both the adaxial and abaxial sides of each leaf sample were counted for trichomes as per [Citation36].
2.6. Feeding initiation
To determine if feeding behavior is affected by trichome density as well as wilting speed, we measured the amount of time it took for FAW larvae to initiate feeding on each genotype. Four leaves were taken from each plant, specifically, newer leaves found toward the top of the plant. To ensure that the leaves did not dry out prior to being fed to the larvae, the leaves were collected in batches to be used immediately. In order to transfer them from the greenhouse to the lab, they were stored in falcon tubes filled with water and a damp cotton ball to retain moisture in the stem. A single leaf was placed on a piece of filter paper, abaxial side up. A handheld digital microscope (TAKMLY; Amazon, China) attached to a laptop was used to gain a better view of the larvae while still allowing them a wide range of motion. After starving the larvae (30 minutes for the first instar and 60 minutes for the third instar), they were placed on the filter paper directly next to the leaf. Using a stopwatch, we measured the time (seconds) it took for the larvae to initiate feeding. Leaf side choice was also recorded. If 20 minutes (1,200 s) elapsed without feeding, it was recorded as a refusal to feed. Experimental set up pictured in .
2.7. Pod yield
To determine differences in yield across soybean genotypes without the stress of herbivory, soybean plants were allowed to grow to maturity without environmental stressors such as herbivory or drought. Once the soybean plants reached maturity and had begun filling their pods, the number of pods per plant was counted and totaled.
2.8. Statistical analysis
The 48-h damage exposure treatment considered genotype, wilting speed, and treatment (i.e., damaged or undamaged) in all of the data collected. Each data set was pooled and analyzed using post hoc analysis using Tukey’s tests. When comparing plant height, mass gain, pupal mass, adult mass, and mortality to soybean plant stage, we used ANOVA. ANOVA was also used when comparing any traits across all soybean genotypes. When comparing plant height, mass gain, pupal mass, adult mass, and mortality to wilting speed, we used T tests. Trichome density and time to initiate feeding were also analyzed using T tests.
3. Results
3.1. 48 h damage exposure experiment
3.1.1. FAW life history parameters measured
3.1.1.1. Mass gain
FAW larvae had the least mass gain compared to their original mass when placed on early-stage soybean plants ( = 53.08%, ±10.72). FAW larvae gained 178.21% (±10.66) on mid-stage soybeans and 155.941% (±17.54) on late-stage soybean plants (, p = .001). Across all stages of soybeans, FAW larvae gained more mass on fast wilting genotypes (
= 141.9%, ±11.07) than slow wilting genotypes (
= 100.92% ±11.53) (, p = .0110).
Figure 4. (A) mean mass gained by FAW when exposed to various soybean growth stages for 48 hours. Different letters denote significant differences in mean mass as determined by post hoc analysis using Tukey’s test (p=.001). (B) mean mass gained by FAW after larvae were exposed to either fast or slow wilting genotypes for 48 hours. Different letters denote significant differences in mean mass as determined by post hoc analysis using student t-test (p=.0110).

3.1.1.2. Pupal mass
FAW pupae of larvae that fed on mid stage ( = 0.22 g, ± 0.01) soybean plants were significantly smaller than FAW pupae that fed on early (
= 0.3 g, ± 0.01) or late-stage (
= 0.3 g, ± 0.01) soybean plants (, p = .001). There was no significant difference in mass gain between pupae exposed to either fast (
= 0.26 g, ± 0.01) or slow (
= 0.27 g, ± 0.01) wilting genotypes (, p = .334).
Figure 5. A) mean pupal mass after larvae were exposed to various soybean growth stages for 48 hours. Different letters denote significant differences in mean mass as determined by post hoc analysis using Tukey’s test (p=.001). B) mean pupal mass after larvae were exposed to either fast or slow wilting genotypes for 48 hours. Different letters denote significant differences in mean mass as determined by post hoc analysis using student t-test (p=.334).

3.1.1.3. Adult mass
There was no significant difference between adult masses across early ( = 0.08 g, ±0.01), mid (
= 0.09 g, ±0.02), and late-stage (
= 0.05, ± 0.01) soybean plants (, p = .216). Also, no significant difference was observed in adult masses across FAW that fed on fast (
= 0.07 g, ±0.01) wilting soybeans versus FAW that fed on slow (
= 0.06 g, ± 0.01) wilting soybeans (, p = .563).
Figure 6. A) Mean adult mass after larvae were exposed to various soybean growth stages for 48 h. Let ‘NS’ denote that there are no significant differences in mean mass as determined by post hoc analysis using Tukey’s test (p=.216). B) Mean adult mass after larvae were exposed to either fast or slow wilting genotypes for 48 h. ‘NS’ denote that there are no significant differences in differences in mean mass as determined by post hoc analysis using student t-test (p=.563).

3.1.1.4. Mortality
Across all stages of soybean, there was a significant difference in mortality. FAW larvae experienced the greatest mortality rates in mid-stage (62.79%) soybean plants, followed by early-stage (32.95%) soybean plants, and then late-stage (30.56%) soybean plants (, p = .0001). Increased mortality was observed on faster wilting (50.89%) soybean genotypes than on slower wilting (36.27%) soybean plants (, p = .0360).
Figure 7. A) Mean mortality of FAW larvae across early, mid, and late soybean growth stages. Different letters denote significant differences in mean mortality across soybean growth stages as determined by post hoc analysis using Tukey’s test (p= .0001). B) Mean mortality of FAW across fast wilting genotypes and slow wilting genotypes. Different letters denote significant differences in mean mortality between fast and slow wilting soybean genotypes as determined by post hoc analysis using student t-test (p=.0360).

3.1.2. Plant traits measured
No significant differences were observed between the damage inflicted across early ( = 2.34, ±0.11), mid (
= 2.05, ±0.114), and late (X = 1.95, ±0.165) soybean growth stages (, p = .0781). There was no significant difference between the damage inflicted on fast (
= 2.2, ±0.1) or slow (
= 2.1, ±0.103) wilting soybean genotypes (, p = .4755). Plant height and number of leaves varied significantly across soybean growth stages (). However, faster wilting soybeans were significantly taller and had significantly more leaves than slower wilting soybeans (). Fast wilting plant height was significantly higher (40.13 cm, ±1.4 cm) compared to slower wilting soybean genotypes (29.39 cm, ±0.97 cm) (p = .0001). Fast wilting genotypes had an average of 18.32 (±0.55) leaves, and slower wilting genotypes had about 13.44 (±0.39) leaves (p = .002).
Figure 8. A) Mean damage assessment across early, mid, and late soybean growth stages. Different letters denote significant differences in mean damage inflicted across soybean growth stages as determined by post hoc analysis using Tukey’s test (p=.0781). B) Mean damage assessment of FAW across fast wilting genotypes and slow wilting genotypes. Let ‘NS’ denote that there are no significant differences in mean damage inflicted between fast and slow wilting soybean genotypes as determined by post hoc analysis using student t-test (p=.4755).

Figure 9. Mean plant height across different soybean growth stages (early, mid, late). Different letters denote significant differences in mean plant height as determined by post hoc analysis using Tukey’s test (p<.05). B) Mean plant height across fast wilting and slow wilting soybean genotypes. Different letters denote significant differences in mean plant height as determined by post hoc analysis using student t-test (p=.0001).

Figure 10. A) Mean number of leaves across different soybean growth stages (early, mid, late). Different letters denote significant differences in a mean number of leaves as determined by post hoc analysis using Tukey’s test (p=.0001). B) Mean number of leaves across fast and slow soybean genotypes. Different letters denote significant differences in a mean number of leaves as determined by post hoc analysis using a student t-test (p=.002).

3.1.3. Trichome density
The abaxial surface of soybean leaves had significantly more trichomes ( = 27, ±0.85) than adaxial leaf surfaces (
= 15, ±0.85) across all soybean genotypes (, p = .0001). Faster wilting soybean genotypes have significantly more trichomes (
= 23, ±0.83) than slower wilting soybean genotypes (
= 19, ±1) (, p = .0025).
Figure 11. A) Mean number of trichomes on different soybean leaf sides (adaxial, abaxial). Different letters denote significant differences in a mean number of leaves as determined by post hoc analysis using a student t-test (p=.0001). B) Mean number of trichomes across fast and slow soybean genotypes. Different letters denote significant differences in a mean number of leaves as determined by post hoc analysis using a student t-test (p=.0025).

3.1.4. Feeding initiation
Early instar FAW larvae initiated feeding significantly faster than late instar FAW larvae with a mean initiation time of 76 s (±9.34 s) compared to 153 s (±11.2 s), respectively (, p = .0001). There is no significant difference in the amount of time it took FAW larvae to begin feeding on slow ( = 101 s, ±12.5) and fast (
= 123 s, ±10) wilting soybean genotypes (, p = .1780). No significant difference in refusal to feed across fast (13.48%) or slow (15.32%) wilting soybean genotypes (, p = .5330) was observed.
Figure 12. A) Mean number of seconds it took FAW larvae of different instars to begin feeding on soybean leaves (early, late). Different letters denote significant differences in mean number of leaves as determined by post hoc analysis using a student t-test (p=.0001). B) Mean number of seconds it took FAW larvae to begin feeding on fast and slow soybean genotypes. Let ‘NS’ denote that there are no significant differences in the mean it took FAW larvae to begin feeding using the student t-test (p=.1780).

3.1.5. Pod yield
Slower wilting soybean genotypes ( = 23, ±0.55) had significantly more pods than faster wilting soybean genotypes (
= 14.5, ±0.46) (, p = .0001).
4. Discussion
In this study, we examined how variation in WUE traits across soybean genotypes impacts FAW growth and development at three different growth stages of soybeans. We found that faster wilting soybean genotypes tend to be taller and have more leaves, which aligned with previous studies [Citation48]. No significant difference was observed in herbivore damage between fast and slow wilting varieties as well as across soybean life stages. This may indicate that while the FAW larvae are damaging the plants equally, there may be effects of fast and slow wilting post-ingestion.
FAW gained more mass, ~40% more when exposed to fast wilting plants compared to slow wilting plants, which also aligned with past literature [Citation38]. FAW mortality was also increased by about 14% on faster wilting plants compared to their slow-wilting counterparts. Since herbivory damage was the same, this increased mass may indicate that although fast wilting plants were more digestible, they may have had an increased toxin load, which proved fatal for FAW. This may be due to the differential production of secondary metabolites between wilting speeds, suggesting that the decrease in susceptibility to abiotic stress allows the plant to divert energy into fortifying chemical deterrents to herbivory. Further investigation is required, as there is great variability across soybean genotypes and increased tolerance to pest damage in slower wilting soybean genotypes has been observed [Citation38].
When exposed to each stage for 48 h, FAW larvae struggled to gain mass on early-stage soybean plants. On early-stage soybean plants, FAW were only able to increase their initial mass by 53%, while on mid-stage and late-stage soybean, FAW increased by a staggering 156% and 178%, respectively. Clearly, early-stage plants in their peak vegetative growth have also invested in higher defenses. However, the pupal mass of FAW that fed on mid-stage soybeans was most impacted and averaged 0.22 g, while early-stage and late-stage averaged 0.3 g. Interestingly, FAW also faced the most mortality on mid-stage soybean plants, with 63% of larvae failing to survive adulthood. We speculate that these effects are also due to the possible partitioning of chemical defenses that may have individual compounds expressed more in early and mid-stages. It has been suggested that there are higher levels of rutin, a type of secondary metabolite, in the early stages of soybean plants, which has been shown to retard the development of FAW at every stage and decrease pupal viability [Citation49,Citation50]. Further investigation is needed, as differential effects are shown in the literature, such as Souza et al. [Citation50] specifically finding that older leaves were more detrimental to FAW and another Lepidopteran pest, velvetbean caterpillar – Anticarsia gemmatalis. Studies by Mai et al. [Citation51] support our findings that earlier-stage soybeans were also detrimental to FAW.
Interestingly, R4 is considered the most vulnerable stage of soybean plant since the plant is beginning to gear up for pod and seed development [Citation52]. It is well documented that the impact of plant stress varies significantly across plant species and even across genotypes within the species [Citation38]. The selected genotypes defended strongly against insect herbivores, and when ingested, as has been shown before, they caused significantly higher mortality [Citation51]. In FAW larvae exposed to early-stage and late-stage, the mortality rate was similar at 33% and 31%, respectively. While differences in adult mass were not significant, it is worth noting that in late-stage soybeans, adult mass hovered at 0.05 g, compared to 0.08 g and 0.09 g in early-stage and mid-stage FAW adults, respectively.
Looking at soybean leaf surface defense mechanisms, there are significantly more trichomes on faster wilting soybean genotypes than slower wilting soybean genotypes by about 17%. Increased trichome density on faster wilting soybean genotypes poses an explanation for increased FAW mortality. Ingestion of trichomes may have pierced the peritrophic membrane in the gut of FAW, causing undigested plant material to mix with hemolymph which may lead to an immune response and eventually death [Citation31]. Research has shown that positive correlations have been found not only between trichome number and stomatal density but also between leaves with wider trichomes having more stomata, which could explain some of our findings with slow and fast wilting soybeans via stomatal conductance [Citation53]. Studies have reported increased trichome density in fast wilting soybean genotypes as a mechanism to overcome drought stress [Citation54]. The abaxial leaf surfaces had significantly more trichomes than the adaxial leaf surfaces by 44%. Although faster wilting soybean genotypes had more trichomes than slower wilting soybean genotypes, there was no significant difference in the number of seconds FAW larvae of early or late instars took to initiate feeding. However, we saw a complete divergence of feeding behavior between early and late FAW instars. Early instar FAW larvae began feeding significantly faster – 50% faster – than late instar FAW larvae. This could indicate that trichomes may be limiting their ability to feed via restricting their movement at later instars [Citation31,Citation36]. No antixenosis effects were observed in the wilting speed between early and late instars of FAW larvae.
Finally, taking a step back and looking at how wilting speed influences yield, there are 37% more pods on slower wilting soybean genotypes than on faster wilting soybean genotypes. Insect herbivory can be reduced above and below ground biomass by 20% and 19%, respectively [Citation38]. A reduction in biomass places a restraint on the forage yield of the crop [Citation55]. The goal of reducing canopy wilting speed in soybean plants is to reduce soybean yield loss under drought conditions. When experiencing ideal growing conditions, there is increased yield from slower wilting soybean genotypes.
We examined the impact of the soybean growth stage on FAW growth and development and analyzed phenotypical differences between soybean genotypes through the lens of fast wilting and slow wilting traits. There is significant variability between genotypes, even within their respective wilting speed categories. While there is vast research analyzing the impacts of different eradication methods on FAW at all stages, the consequences of herbivory by FAW on soybean are still relatively unexplored. There is evidence to indicate that soybean releases different secondary metabolites that influence pest damage; however, it is unclear how that response varies between soybean genotypes and especially between genotypes with different wilting speeds [Citation56]. There is also the variation between soybean genotypes and how more significant expansion of Genome-Wide Association Studies (GWAS) is needed to further quantify the diverse traits seen between genotypes. As previously mentioned, WUE traits and drought tolerance traits have multiple genetic markers and, therefore, have varying impacts on plant fitness [Citation48]. This indicates that secondary metabolite biosynthesis may be triggered by abiotic stress that differs significantly not only between plant species but also within plant genotypes. An area that requires further exploration is how these traits culminate into trophic interactions in agricultural ecosystems, such as insect herbivory. Various studies have examined how drought stress impacts insect herbivory, and there is a wide variety of responses ranging from positive to negative [Citation30,Citation57,Citation58]. Determining whether drought stress causes an increase in secondary metabolite production (e.g., plant defense compounds) in plants depends mainly on the type of plant and the type of insect pest inflicting damage, and there is evidence to suggest that even the lineage of the plant can also cause a differential response to insect damage [Citation38,Citation59]. Coupling WUE traits with herbivory to examine whether trophic cascades are affected is not only an interesting line of research but can also lead to developing lines with better stress responses.
There is a significant need for the characterization of these traits across soybean genotypes to gain a further understanding of how these traits influence plant–insect interactions. FAW is a prolific polyphagous pest that is plaguing multitudes of crops. It is a voracious feeder and generalist that evades various pest treatments and places a significant financial burden on growers [Citation60]. To minimize the damage FAW inflicts on crops, we must gain a better understanding of its interactions with its host plants to identify insect resistant traits found in them and examine how those influence WUE traits. Growers and plant breeders have been in an arms race with insect pests like FAW and their increased resistance. Now, climate change has added rising temperatures and drought to the equation. To produce soybean accessions that maximize yield while deterring insect pests, we must better understand how these traits tie together through further characterization of these traits and how they impact plant defenses.
Author contributions
Conceptualization, J.A. (Jessica Ayala) & R.K. (Rupesh Kariyat); methodology, J.A (Jessica Ayala), E.M (Evelyn Madrigal) and R.K. (Rupesh Kariyat); validation, formal analysis, J.A. (Jessica Ayala) & R.K. (Rupesh Kariyat); investigation, J.A (Jessica Ayala), A.V. (Alejandro Vasquez), E.M (Evelyn Madrigal) & D.B. (Devi Balakrishnan); resources, R.K. (Rupesh Kariyat) and J.G. (Justin George); data curation, J.A. (Jessica Ayala), E.M (Evelyn Madrigal) & R.K. (Rupesh Kariyat); writing – original draft preparation, J.A. (Jessica Ayala); writing – review and editing, R.K. (Rupesh Kariyat), A.V. (Alejandro Vasquez), D.B. (Devi Balakrishnan), J.G. (Justin George); supervision, R.K. (Rupesh Kariyat); project administration, R.K. (Rupesh Kariyat); funding acquisition, R.K. (Rupesh Kariyat) & J.G. (Justin George). All authors have read and agreed to the published version of the manuscript.
Acknowledgments
The authors acknowledge Brandon Wodka at the Rosen Green House facility at the University of Arkansas.
Disclosure statement
No potential conflict of interest was reported by the author(s).
Data availability statement
All data will be made available on request.
Additional information
Funding
References
- Food and Agriculture Organization of the United Nations. FAO-UN. Fao.org. Food and Agriculture Organization of the United Nations; 2024. https://www.fao.org/land-water/databases-and-software/crop-information/soybean/en/
- Fried HG, Narayanan S, Fallen B, et al. Evaluation of soybean [Glycine max (L.) Merr.] genotypes for yield, water use efficiency, and root traits. PLoS One. 2019;14(2):e0212700. doi: 10.1371/journal.pone.0212700
- American Soybean Association. Annual soy stats results. American Soybean Association; 2022. https://soygrowers.com/education-resources/publications/soy-stats/
- Bray EA. Responses to abiotic stresses. Biochem Molecul Biol Plants; 2000. p. 1158–13.
- Heatherly LG. Drought stress and irrigation effects on germination of harvested soybean seed. Crop sci. 1993;33(4):777–781. doi: 10.2135/cropsci1993.0011183X003300040029x
- Purcell LC, Specht JE. Physiological traits for ameliorating drought stress. Soybeans: Improvement, Prod Uses. 2004;16:569–620.
- Dornbos DL Jr, Mullen RE. Influence of stress during soybean seed fill on seed weight, germination, and seedling growth rate. Can J Plant Sci. 1991;71(2):373–383. doi: 10.4141/cjps91-052
- Ku YS, Au-Yeung WK, Yung YL, et al. Drought stress and tolerance in soybean. A Comprehensive Survey Int Soybean Res—Genetic, Physiol, Agron Nitrog Relationships. 2013:209–237.
- Bellaloui N, Gillen AM, Mengistu A, et al. Responses of nitrogen metabolism and seed nutrition to drought stress in soybean genotypes differing in slow-wilting phenotype. Front Plant Sci. 2013;4:498. doi: 10.3389/fpls.2013.00498
- Maleki A, Naderi A, Naseri R, et al. Physiological performance of soybean cultivars under drought stress. Bullet Environ, Pharmacol Life Sci. 2013;2(6):38–44.
- Wallander S, Hrozencik A, Aillery M. Some irrigation organizations rely on formal drought plans. Amber Waves: The Economics Of Food, Farming, Natural Resources, And Rural America; 2022.
- Chamarthi SK, Kaler AS, Abdel-Haleem H, et al. Identification and confirmation of loci associated with canopy wilting in soybean using genome-wide association mapping. Front Plant Sci. 2021;12:698116. doi: 10.3389/fpls.2021.698116
- Hatfield JL, Dold C. Water-use efficiency: advances and challenges in a changing climate. Front Plant Sci. 2019;10:429990. doi: 10.3389/fpls.2019.00103
- Van Vliet MT, Jones ER, Flörke M, et al. Global water scarcity including surface water quality and expansions of clean water technologies. Environ Res Lett. 2021;16(2):024020. doi: 10.1088/1748-9326/abbfc3
- Sinclair TR, Purcell LC, Sneller CH. Crop transformation and the challenge to increase yield potential. Trends Plant Sci. 2004;9(2):70–75. doi: 10.1016/j.tplants.2003.12.008
- Chen J, Chang SX, Anyia AO. The physiology and stability of leaf carbon isotope discrimination as a measure of water‐use efficiency in barley on the Canadian prairies. J Agron Crop Sci. 2011;197(1):1–11. doi: 10.1111/j.1439-037X.2010.00440.x
- Fletcher AL, Sinclair TR, Allen LH Jr. Transpiration responses to vapor pressure deficit in well watered ‘slow-wilting’and commercial soybean. Environ Exp Bot. 2007;61(2):145–151. doi: 10.1016/j.envexpbot.2007.05.004
- King CA, Purcell LC, Brye KR. Differential wilting among soybean genotypes in response to water deficit. Crop sci. 2009;49(1):290–298. doi: 10.2135/cropsci2008.04.0219
- Kunert K, Vorster BJ. In search for drought-tolerant soybean: is the slow-wilting phenotype more than just a curiosity? J Exp Bot. 2020;71(2):457–460. doi: 10.1093/jxb/erz235
- Devi MJ, Sinclair TR. Fixation drought tolerance of the slow‐wilting soybean PI 471938. Crop sci. 2013;53(5):2072–2078. doi: 10.2135/cropsci2013.02.0095
- Ries LL, Purcell LC, Carter TE Jr, et al. Physiological traits contributing to differential canopy wilting in soybean under drought. Crop sci. 2012;52(1):272–281. doi: 10.2135/cropsci2011.05.0278
- Charlson DV, Bhatnagar S, King CA, et al. Polygenic inheritance of canopy wilting in soybean [glycine max (L.) merr.]. Theor Appl Genet. 2009;119(4):587–594. doi: 10.1007/s00122-009-1068-4
- Sinclair TR, Messina CD, Beatty A, et al. Assessment across the United States of the benefits of altered soybean drought traits. Agronomy J. 2010;102(2):475–482. doi: 10.2134/agronj2009.0195
- Ye H, Song L, Schapaugh WT, et al. The importance of slow canopy wilting in drought tolerance in soybean. J Exp Bot. 2020;71(2):642–652. doi: 10.1093/jxb/erz150
- Hamann E, Blevins C, Franks SJ, et al. Climate change alters plant–herbivore interactions. New Phytol. 2021;229(4):1894–1910. doi: 10.1111/nph.17036
- Kuczyk J, Müller C, Fischer K. Plant-mediated indirect effects of climate change on an insect herbivore. Basic Appl Ecol. 2021;53:100–113. doi: 10.1016/j.baae.2021.03.009
- Rouault G, Candau JN, Lieutier F, et al. Effects of drought and heat on forest insect populations in relation to the 2003 drought in Western Europe. Annals Forest Sci. 2006;63(6):613–624. doi: 10.1051/forest:2006044
- Roy BA, Güsewell S, Harte J. Response of plant pathogens and herbivores to a warming experiment. Ecol. 2004;85(9):2570–2581. doi: 10.1890/03-0182
- Staley JT, Mortimer SR, Masters GJ, et al. Drought stress differentially affects leaf‐mining species. Ecol Entomol. 2006;31(5):460–469. doi: 10.1111/j.1365-2311.2006.00808.x
- Gely C, Laurance SG, Stork NE. How do herbivorous insects respond to drought stress in trees? Biol Rev. 2020;95(2):434–448.
- Kaur J, Kariyat R. Role of trichomes in plant stress biology. Evolut Ecol Plant-Herbivore Interact; 2020. p. 15–35.
- Balakrishnan D, Bateman N, Kariyat RR. Rice physical defenses and their role against insect herbivores. Planta. 2024;259(5):110. doi: 10.1007/s00425-024-04381-7
- Kariyat RR, Hardison SB, De Moraes CM, et al. Plant spines deter herbivory by restricting caterpillar movement. Biol Lett. 2017;13(5):20170176.
- Gélin U, Charles-Dominique T, Davies TJ, et al. The evolutionary history of spines – a Cenozoic arms race with mammals. bioRxiv. 2023:2023–02.
- Kaur I, Kariyat RR. Eating barbed wire: direct and indirect defensive roles of non‐glandular trichomes. Plant Cell Environ. 2020;43(9):2015–2018. doi: 10.1111/pce.13828
- Watts S, Kariyat R. Picking sides: feeding on the abaxial leaf surface is costly for caterpillars. Planta. 2021;253(4):1–6. doi: 10.1007/s00425-021-03592-6
- Watts S, Kariyat R, Buckley T. Morphological characterization of trichomes shows enormous variation in shape, density and dimensions across the leaves of 14 Solanum species. AoB Plants. 2021;13(6):lab071. doi: 10.1093/aobpla/plab071
- Grinnan R, Carter TE, Johnson MT. Effects of drought, temperature, herbivory, and genotype on plant–insect interactions in soybean (glycine max). Arthropod Plant Interact. 2013;7(2):201–215. doi: 10.1007/s11829-012-9234-z
- Hruska AJ. Fall armyworm (Spodoptera frugiperda) management by smallholders. CABI Rev. 2019;2019:1–11. doi: 10.1079/PAVSNNR201914043
- Kenis M, Benelli G, Biondi A, et al. Invasiveness, biology, ecology, and management of the fall armyworm. Spodoptera frugiperda; 2022.
- Yan XR, Wang ZY, Feng SQ, et al. Impact of temperature change on the fall armyworm, Spodoptera frugiperda under global climate change. Insects. 2022;13(11):981. doi: 10.3390/insects13110981
- Purdue University. Fall armyworms. Purdue University - College of Agriculture; 2023. https://ag.purdue.edu/department/entm/extension/field-crops-ipm/corn/fall-armyworms.html
- Mota-Sanchez D, Wise JC. Arthropod pesticide resistance database (APRD). Michigan State University 2021. 2024. https://www.pesticideresistance.org
- Udayakumar A, Shivalingaswamy TM, Bakthavatsalam N. Legume-based intercropping for the management of fall armyworm, Spodoptera frugiperda L in maize. J Plant Dis Protect. 2021;128(3):775–779. doi: 10.1007/s41348-020-00401-2
- Wu K, Jiang C, Zhou S, et al. Optimizing arrangement and density in maize and alfalfa intercropping and the reduced incidence of the invasive fall armyworm (Spodoptera frugiperda) in southern China. Field Crops Res. 2022;287:108637. doi: 10.1016/j.fcr.2022.108637
- Singh S, Kariyat RR. Exposure to polyphenol-rich purple corn pericarp extract restricts fall armyworm (Spodoptera frugiperda) growth. Plant Signal Behav. 2020;15(9):1784545. doi: 10.1080/15592324.2020.1784545
- Chavana J, Singh S, Vazquez A, et al. Local adaptation to continuous mowing makes the noxious weed Solanum elaeagnifolium a superweed candidate by improving fitness and defense traits. Sci Rep. 2021;11(1):6634.
- Chamarthi SK, Kaler AS, Abdel‐Haleem H, et al. Identification of genomic regions associated with the plasticity of carbon 13 ratio in soybean. Plant Genome. 2023;16(1):e20284. doi: 10.1002/tpg2.20284
- Silva TRFB, Almeida ACDS, Moura TDL, et al. Effect of the flavonoid rutin on the biology of Spodoptera frugiperda (Lepidoptera: Noctuidae). Acta Scientiarum Agron. 2016;38:165–170. doi: 10.4025/actasciagron.v38i2.27956
- Souza BH, Costa EN, Ribeiro ZA, et al. Soybean leaf age and plant stage influence expression of resistance to velvetbean caterpillar and fall armyworm. Chemoecol. 2021;31(6):377–390. doi: 10.1007/s00049-021-00360-6
- Mai VC, Tran NT, Nguyen DS. The involvement of peroxidases in soybean seedlings’ defense against infestation of cowpea aphid. Arthropod Plant Interact. 2016;10(4):283–292. doi: 10.1007/s11829-016-9424-1
- Neyla T, Moriles-Miller J, Gustafson K, et al. (PDF) Soybean Growth Stages. Research Gate; 2019. https://www.researchgate.net/publication/331683847_Soybean_Growth_Stages
- Amaliah N, Zubaidah S, Kuswantoro H. Trichomes and stomata diversity in soybean (Glycine max L. Merill) lines. In: IOP Conference Series: Earth and Environmental Science; Malang, Indonesia; (Vol. 276). IOP Publishing; 2019. p. 012025
- Romero B, Dillon FM, Zavala JA. Different soybean cultivars respond differentially to damage in a herbivore-specific manner and decreas herbivore performance. Arthropod Plant Interact. 2020;14(1):89–99. doi: 10.1007/s11829-019-09730-y
- Abebe AT, Adewale S, Chigeza G, et al. Diallel analysis of soybean (glycine max L.) for biomass yield and root characteristics under low phosphorus soil conditions in Western Ethiopia. PLoS One. 2023;18(2):e0281075. doi: 10.1371/journal.pone.0281075
- Mattson WJ, Haack RA. The role of drought in outbreaks of plant-eating insects. BioScience. 1987;37(2):110–118. doi: 10.2307/1310365
- Carnicer J, Stefanescu C, Vives‐Ingla M, et al. Phenotypic biomarkers of climatic impacts on declining insect populations: a key role for decadal drought, thermal buffering and amplification effects and host plant dynamics. J Animal Ecol. 2019;88(3):376–391. doi: 10.1111/1365-2656.12933
- Chen JJ, Sun Y, Kopp K, et al. Effects of water availability on leaf trichome density and plant growth and development of Shepherdia× utahensis. Front Plant Sci. 2022;13:855858. doi: 10.3389/fpls.2022.855858
- Herbivory Variability Network*†, Robinson ML, Hahn PG, et al. Plant size, latitude, and phylogeny explain within-population variability in herbivory. Sci. 2023;382(6671):679–683. doi: 10.1126/science.adh8830
- Naganna R, Jethva DM, Bhut JB, et al. Present status of new invasive pest fall armyworm, Spodoptera frugiperda in India: a review. J Entomol Zool Stud. 2020;8(2):150–156.