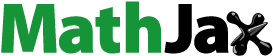
Abstract
The habitual motion path theory states that exhibiting kinematic differences between running and habitual movement tasks may increase the risk of running-related injury. Staying near one’s habitual motion path when running has been shown to reduce compression of the knee joint cartilage, a proxy for decreased soft tissue loading. Previous efforts to quantify one’s habitual motion path and deviation when running have utilised just one habitual movement and one time point during the stance phase of running. The methodology advancement described in this manuscript focused on advancing habitual motion path theory research by (1) increasing the number of movements for quantifying an individual’s habitual motion path; and (2) utilising the entire stance phase when running to quantify deviations as opposed to one time point. We liken the advancements in the method described in this manuscript as with the first method we were using a ruler to assess one’s habitual motion path and deviations, and now we are using calipers; providing a more accurate understanding of an individual’s unique habitual movement and deviation when running. We also discuss future potential research avenues related to understanding running biomechanics and footwear effects that this tool will allow us to explore.
Keywords:
Introduction
The majority of runners will sustain at least one running-related injury per year (Kluitenberg et al., Citation2015; Lun et al., Citation2004). Running injuries can result in long term inactivity as people lose motivation to run following an injury, and typically do not replace running with other physical activity (Davis & Gruber, Citation2020; Lohmander et al., Citation2004). Prevention of running injuries will enable individuals to avoid comorbidities associated with inactivity, including heart disease and obesity. Unfortunately, running-related injury (RRI) rates and location prevalence have had little to no change at the epidemiological level across the past decades (Taunton et al., Citation2002, Kakouris et al., Citation2021; Van der Worp et al., Citation2015).
A variety of extrinsic and intrinsic risk factors have been identified for running injuries. Extrinsic risk factors revolve primarily around training mileage, frequency, and environment (Bovens et al., Citation1989; Hespanhol Junior et al., Citation2013; Paquette et al., Citation2020; Rudzki, Citation1997). Intrinsic risk factors for running injury have been identified as reduced muscular strength, excessive joint movement, and high loading of soft tissues (Boling et al., Citation2009; Kalkhoven et al., Citation2020; Mahieu et al., Citation2006; Paquette et al., Citation2018; Stefanyshyn et al., Citation2006; Warden et al., Citation2021). For decades, the footwear industry focused on ankle pronation as a primary risk factor for injury (Agresta et al., Citation2022; Nigg et al., Citation2015; Nigg & Segesser, Citation1992), with product innovations respectively focused on dual-density midsoles to reduce eversion/pronation of the rearfoot (Cheung et al., Citation2011; Hoffman et al., Citation2015; Willems et al., Citation2021). Pronation, defined as a tri-planar rotation about the ankle joint complex paired with compression of the longitudinal arch, is a natural movement and a necessity for shock absorption (Farris et al., Citation2019; Holowka et al., Citation2022; Ker et al., Citation1987) and economical movement (Stearne et al., Citation2016), yet receives continued attention due to the visual ease of bucketing an individual’s movement pattern to be “hyper,” “over,” “normal,” or “under” pronated despite there being no concrete definition of such movements (Nigg et al., Citation2019) or substantial evidence that a certain amount of pronation is better. While there is prospective evidence that frontal plane ankle kinematics (eversion, eversion rate) are linked to injury (Dudley et al., Citation2017; Kuhman et al., Citation2016; Willwacher et al., Citation2022), there is surmounting evidence that the influence of eversion on injury may not be reflected through classic discrete variables such as peak eversion or eversion range of motion (Nigg et al., Citation2015). Instead, the duration of eversion, which prolongs increased stress/strain of soft tissues along the medial aspect of the foot and ankle (Becker et al., Citation2017, Citation2018; Hannigan & Pollard, Citation2021; Jungmalm et al., Citation2020), or the amount of movement relative to the bodies’ available range of motion may more closely relate to injurious soft tissue stress/strain (Rodrigues et al., Citation2013) than objective discrete kinematics. Ultimately, joint kinematics should be measured and assessed relative to the individual to estimate potential risk for injury as a population or group-wide approach continues to present null findings (Nigg et al., Citation2015).
Product innovations focused on reducing ankle eversion limit the focus on human movement to motion at a single joint in a single plane. Methods for quantifying and understanding the influence of product on human movement as it pertains to potential running injury risk should be more holistic, including movement beyond the ankle joint as the knee is the most commonly injured location in runners (Kakouris et al., Citation2021; Taunton et al., Citation2002; Van der Worp et al., Citation2015); hypothesised to be due to multiple factors such as its role in shock attenuation (Edwards et al., Citation2012), limited range of motion in the frontal and transverse planes compared to the sagittal plane, and its role in mediating footwear-dependent distal load from the foot-ankle complex (Hintermann & Nigg, Citation1998; Meardon et al., Citation2018) and proximal load from the hip (Clark et al., Citation2020; Powers, Citation2010). While movement of the ankle may influence movement of the knee, it is important to study the knee directly because the strength of coupling at the ankle joint is highly variable between individuals (Fischer et al., Citation2018; Hintermann & Nigg, Citation1998).
The preferred movement path was the first proposed paradigm that shifted the focus away from discrete kinematic peaks and averages to optimal movement paths that minimise muscle activity, theoretically reducing joint loading and energetic demand via the body moving through its unique path of least resistance (Nigg, Citation2001; Nigg et al., Citation2015). This paradigm suggests that there is a particular preferred movement path for any given movement task, and that the body will adapt as needed to move through this path of least resistance for the task. According to this paradigm, different movements have different preferred movement paths. Through the lens of footwear, this would mean that there is a different optimal movement path for every footwear condition (Nigg et al., Citation2017). While different footwear constructions will have a path of least resistance that progresses the foot from heel to toe, this path may not minimise soft tissue stress/strain if the shoe is making an individual move in a way that is unfamiliar or different from their habitual movement patterns. Contrary to Nigg’s preferred movement path that is task-specific, the habitual motion path (HMP) suggests shifting the optimum to the person’s unique habitual motion path as defined by their anatomy (Trudeau et al., Citation2019). Cadaver studies have shown that individuals have a ‘minimal resistance movement path’ determined by their unique bone geometries, muscle volumes, tendon stiffness, etc. (Trudeau et al., Citation2019; Wilson et al., Citation1996) that is sensitive to changes in external force (Wilson et al., Citation2000). While the direct link between the HMP theory and all forms of joint loading (joint moments, joint contact forces, etc.) is at this time speculative, staying within one’s HMP when running should theoretically reduce soft tissue stress/strain as the body is moving through its own path of least resistance, not a condition dependent path of least resistance, which in turn should help reduce risk of injury.
The method previously used to quantify one’s HMP assessed ankle and knee kinematics during a body-weight shallow squat (Trudeau et al., Citation2019). Frontal and transverse plane kinematics of the ankle and knee were compared between the shallow squat and running movement at the time of peak knee flexion to determine one’s deviation from their HMP when running. From this methodological analysis, the concept of a low and high deviation was created. Low deviation is when a runner is exhibiting similar ankle and knee kinematics during running as they do during their habitual motion during daily activities. On the contrary, a high deviation is when a runner is exhibiting a large difference in ankle and knee joint kinematics while running compared to their habitual motion during daily activities. Deviating away from one’s HMP, whether at the joint-mechanics (Trudeau et al., Citation2019) or spatiotemporal level (Loegering et al., Citation2021) is theorised to cause an increase in soft tissue loading, which may potentially lead to injury. There is biological evidence that increasing one’s deviation away from their HMP increases cartilage volume compression at the knee during a prolonged run (Willwacher et al., Citation2020), demonstrating that deviating away from one’s HMP increases this form of joint loading, as indicated by changes in knee joint cartilage volume measured by MRI scans pre and post run. More specifically, this loading is acting on less-adapted soft tissues as your body is moving through a movement profile that is not habitual when it is deviating away from its HMP.
Quantifying the habitual motion path and how one deviates when running helped to better understand the influence of footwear on individualised joint-level kinematics and create a framework for individualised footwear geometries. For example, two runners may both exhibit ten degrees of peak knee internal rotation when running. With a traditional discrete variable kinematic analysis, these two runners would be deemed the same. However, when compared to their HMP these two runners will more than likely be deviating away from their HMP at different magnitudes. One runner may move through 10 degrees of knee internal rotation regularly throughout their habitual movement while the other runner may only move through two degrees of rotation during their habitual movement. As a result, one would be identified as experiencing high deviation (two degrees of rotation habitually but 10 degrees while running) while the other would be experiencing low deviation (10 degrees of rotation during both habitual movement and when running). Despite having the same peak knee internal rotation angle while running, the runner experiencing high deviation would benefit from a product that guides them closer to their habitual amount of movement (2 degrees) while the runner experiencing low deviation would benefit from a product keeping them at 10 degrees of rotation. This theory may also explain why discrete kinematic metrics on their own, independent of being contextualised to the individual runner, have not been consistently related to injury occurrence. For one individual, a certain amount of motion may be deemed a safe amount, whereas for another individual that amount of motion is too different from how their joints habitually move and may be overloading their soft tissues in un-adapted ways, ultimately resulting in injury.
While quantifying the HMP with a body-weight shallow squat was an exciting first step to better understand the runner at the individual level (Trudeau et al., Citation2019), there were two key limitations. First, it used only one movement, a shallow squat, to establish an individuals’ HMP. Second, deviation from the HMP when running was quantified at a single time point, the occurrence of maximum knee flexion. The use of a single time point at maximum knee flexion may be limiting our ability to understand the individual and footwear effects as the most inter-individual variation and influence of footwear occurs in early or late stance (Day & Baltich, Citation2021; Hannigan & Pollard, Citation2020; Hoitz et al., Citation2021). While this method was pivotal in quantifying human movement at the individual level and unique effects of footwear on joint-level kinematics, we sought to advance this method with the goal of more holistically understanding the runner by (1) adding more habitual movements, and (2) expanding the analysis to the entire stance phase for quantifying the HMP and deviations during running. The purpose of this manuscript is to describe how we addressed these limitations and detail a new methodology to quantify one’s HMP and deviation when running.
Materials and methods
Three-dimensional kinematic data from 185 participants (73 males, 109 females, 3 non-binary; Age: 35 ± 10 years; Height: 170 ± 8 cm; Weight: 66 ± 8 kg) were used for method development and analysis. All participants visited Brooks for product testing and signed an informed consent form prior to data collection. A previously described marker set (Trudeau et al., Citation2019) was used to collect unilateral kinematic data of the rearfoot, shank, and thigh to estimate three-dimensional joint angles of the ankle and knee. The rearfoot was defined and tracked by three markers on the calcaneus arranged in a triangle shape, the shank defined by anatomical markers on the ankle malleoli and femoral condyles and tracked via a rigid cluster affixed close to the segment centre of mass; and the thigh defined by markers on the femoral condyles and greater trochanters and tracked via a rigid cluster affixed close to the segment centre of mass. Joint angles were calculated using a Cardan-Euler X-Y-Z rotation sequence.
Establishing an individuals’ habitual movement
Participants wore sock shoes while performing habitual movements, a 5.5 mm sock liner with a thin layer of rubber glued on the bottom and running socks glued to the top (Trudeau et al., Citation2019). Sock shoes were used due to their minimal intervention on one’s gait but providing enough comfort and protection compared to being barefoot. For a more comprehensive capture of an individual’s habitual movement, participants performed the original shallow squat (Trudeau et al., Citation2019) plus three new baseline motions: lunge, stair descent, and a walk. These motions were selected based on the criteria of (1) sufficient sagittal plane range of motion at the ankle and knee, (2) presence of an eccentric knee flexion phase, (3) feasibility of collection in a lab environment, and (4) these motions are commonly done by individuals day-to-day and can be thought of as highly repeatable. Participants were allowed to practice each motion to ensure comfortability with the movement, and were instructed to perform each motion as described:
Squat: Stand with feet approximately hip-width apart and toes pointed forward like you are about to sit down in a chair, with arms crossed in front of the chest. Perform ten squats to approximately 90° of hip and knee flexion at a frequency that is comfortable, making sure to stand up straight between each repetition.
Lunge: Lunge forward with the right leg to a comfortable depth, trying not to let the knee track forward over the foot, and lunge low enough that the left knee comes near or to the ground. Return to standing and pause after each lunge. Perform six total lunges.
Stair Descent: Starting at the top step of a set of stairs (purchased at a hardware store (height: 20.3 cm, length: 25.4 cm, width: 45.8 cm) with two steps and a custom affixed handrail), descend with the right leg onto the first step, left foot to the second step, ending with the right foot to the bottom. Perform six total stair descent trials.
Walk: Preferred walking speed for each participant was determined by starting at 0.8 m/s and increasing speed by 0.1 m/s until verbal confirmation from the participant that their preferred speed had been reached. Participants walked a minimum of ∼30 s or until they verbally confirmed they felt comfortable, with data collected for 10 s at the end.
Upon completion of habitual movements, participants completed a run in the sock shoes. The run was performed at 3.35 m/s (8 min/mile) for ∼1 min, with data collected during the last 10 s. All unilateral foot-strikes from the retro-reflective markered leg from the 10 s collections were used for analysis.
Deviation calculation
Joint angles of interest for which deviations were calculated were ankle eversion (Eve), knee internal rotation (TIR), and knee ab-adduction (AbAd). This new method omitted calcaneal ab-adduction due to difficulties of accurate measurement with markers placed externally on a shoe (Alcantara et al., Citation2018; Jewell et al., Citation2017). Quantification of one’s deviation from their HMP was completed as described below:
Defining Kernel density plots for each joint angle
Kernel density plots (KDPs) were created from joint angle data from (1) habitual movements and (2) the sock run () using the MATLAB function ‘ksdensity’ with default settings and equally weighted data points. The habitual movement KDP was created using data from the entirety of foot contact during the various movements. Kernel density plots were chosen due to the additional information they provide over a discrete deviation metric, notably the joint angle range of motion, orientation of the joint, and the ability to see where the joint is most commonly oriented throughout stance (the mode). Joint angles from the various movements were combined into one vector for input. For the squat motion the start and end were defined as initiation of knee flexion and maximum knee extension, respectively. Stair descent ankle eversion data was omitted due to the unique forefoot strike pattern of stair descent. The sock run KDP was created using joint angle data from ten stance phases, combined into one vector.
Figure 1. (a) Original method (Trudeau et al., Citation2019) to calculate a deviation as the difference between run and squat joint angle values at maximum knee flexion. (b) Exemplar joint angles from all movements used to create the habitual movement kernel density plot. This process is completed for all three deviations of interest, with knee internal rotation (TIR) plotted here for visualisation. (c) Exemplar comparison of run and habitual movement data for one participant.

Applying vGRF weighting
The sock run KDP was divided into segments defined by the KDP MATLAB function vertices. A weighting factor was applied to each segment, defined as the external vertical ground reaction force (vGRF) normalised to bodyweight at the time of the segment’s average joint angle (). The purpose of the KDP weighting was to place more importance on kinematics that are occurring while the external ground reaction force is higher around midstance, as this is when soft tissue stress/strain of various structures tend to be higher during level ground running (Day & Gillette, Citation2019; Rice et al., Citation2019; Willy et al., Citation2016).
Figure 2. Method for quantifying deviation from one’s habitual motion path when running for an exemplar segment. For all sub-figures, red is running data; blue is habitual movement data. (3a) Quantify average joint angle (θ) from each segment in the run kernel density plot. (3b) Apply weighting to the segment, w, that is the body weight normalised external vGRF at time, t, where θ occurs. (c) Calculate the percent difference, as defined in EquationEquation 3(3)
(3) , between the weighted run area (WRA) and weighted overlap area (WOA), defined in EquationEquations 1
(1)
(1) and Equation2
(2)
(2) , respectively.
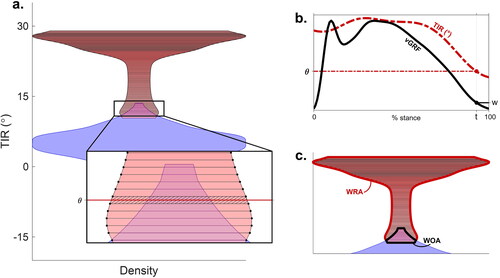
Calculate deviation between habitual movement and sock run
After creation of the habitual movement KDP and the weighted sock run KDP, the amount of overlap between these two plots was quantified using the following equations:
(1)
(1)
(2)
(2)
Where ‘WRA’ is the weighted sock run area, ‘WOA’ is the weighted overlap area, ‘w’ is the external vGRF weighting applied in step 2, and ‘rsa’ and ‘osa’ are the run segment area and overlap segment area, and ‘i’ is each segment, respectively, shown in .
Deviations for each respective joint angle were then calculated using the following equation:
(3)
(3)
This deviation value represents the percentage of the weighted run KDP that does not overlap with the habitual movement KDP. In other words, the percentage of an individual’s joint motion during the run, weighted by loading, that falls outside their habitual movement KDP. shows an example of an individual with a small and large deviation in two respective footwear conditions.
Figure 3. Exemplar plots of (a) one runner exhibiting a high deviation when running in the sock shoe (left) and a low deviation (right) as a result of using a shoe that guided them back to their habitual motion path. (b) three different runners exhibiting a high deviation in the sock shoe but with different habitual motion paths to be guided back to that will theoretically require different footwear constructions to reduce deviation based on their unique movement. The first deviation method (Trudeau et al., Citation2019) did not account for the habitual movement to be guided back to, just the presence of a deviation. For all sub-figures, red is running data; blue is habitual movement data. TIR: knee internal rotation.
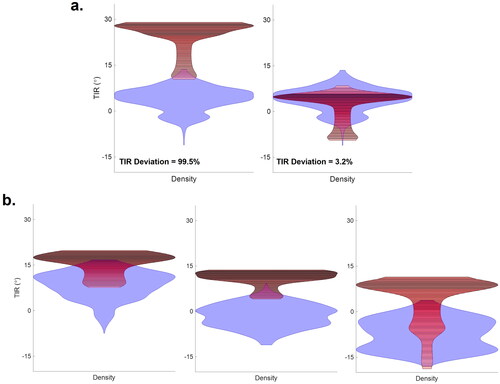
Identification of deviator functional groups
All participants’ joint angle deviations were used to update thresholds defining Low and High deviators. Thresholds were defined by the population median (). Note that a deviation is now represented as a percentage and not a degree magnitude as previously (Trudeau et al., Citation2019), due to using the entire stance phase for quantification.
Results
The biomechanical results from this methodological advancement are focused on updated population thresholds that define an individual as a Low or High deviator. visualises the population distribution for three biomechanical deviations: ankle eversion, knee internal rotation, and knee ab-adduction. To contextualise these percentage deviations into joint angle magnitudes, median deviation thresholds for the study population compared to Trudeau et al., Citation2019, are as follows: 35% ankle eversion corresponds to 4.3 degrees, 32% knee internal rotation corresponds to 3.5 degrees, and 12% knee ab-adduction corresponds to −1 degrees. Note that the participant populations are different between studies due to the requirement for more habitual movements to perform the updated analyses. Additionally, Trudeau et al., Citation2019 represents a deviation quantified at a single timepoint versus a metric to reflect deviation across the entire stance phase, and does not have a ground reaction force weighting factor, as created in this new method.
Discussion
The purpose of this methodological update was to improve the ability to quantify one’s HMP and move beyond using a single time point to quantify one’s deviation from their HMP when running. Including more habitual daily movements into the quantification of one’s HMP provides a more comprehensive dataset to understand an individual’s unique movement at the ankle and knee. Additionally, comparing movement at the ankle and knee during the entire ground contact phase of these habitual movements to the entire ground contact phase of stance during running provides a holistic understanding of one’s deviation from their HMP while running. The results demonstrate that individuals vary on how differently their ankle and knee joints move during running compared to their habitual motion path ( and 4). Reducing deviation has previously been shown to be linked to reduced compression of knee joint cartilage volume over the course of a run (Willwacher et al., Citation2020), a surrogate measure of reduced soft tissue loading. By advancing this testing protocol we can understand more about the runner and their potential risk for tissue overload and injury based on how their body moves on the run relative to their habitual motion.
When it comes to recommending footwear to runners, in this framework, the shoe that is best is the one that keeps them closer to their HMP. The preferred movement path paradigm speculates that each shoe has its own optimal movement path based on the path of least resistance through the shoe determined by where the shoe will compress, bend, etc. The HMP theory promotes that every person has their own HMP and the shoe that is best for them keeps them closest to that path. For those runners that move differently than their HMP while running, footwear with support technology can provide the extra guidance needed to bring them closer to their HMP (Trudeau et al., Citation2019; Willwacher et al., Citation2020).
In addition to quantifying one’s HMP deviation, this new method allows for quantification of the amount of motion an individual has during habitual daily life tasks and while running (range of motion of the KDPs), and a more robust understanding of their joints’ general positioning during movement (mode of the KDPs), beyond discrete values such as peak angles. This information allows us to understand the running movement at a more comprehensive level knowing how each runner tends to move. This deeper layer of understanding runners as unique individuals and how they change when running will allow us to more granularly understand the different types of runners that a product should be created for. showcases three runners exhibiting high deviation but needing to be guided back to different habitual motion patterns. Theoretically, runners of different movement patterns would have different footwear that works best for them (Hoerzer et al., Citation2015), as observed with runners exhibiting high and low deviation being in proper footwear (Willwacher et al., Citation2020). This is why footwear brand product lines are created with footwear of varying build characteristics (hardness, flexibility, heel-toe drops, geometry, etc.) that together determine how a shoe compresses and flexes in a unique way to move with or guide motion of the foot and leg. It is important to note that while footwear is generally classified into categories, i.e. neutral or support, this does not mean that all shoes within these classifications will function exactly the same (). Specific footwear build characteristics will work uniquely better for different runners based on their HMP as well as injury history. This new method is an important step in working towards identifying population functional groups based on individual movement patterns, and how one deviates when running, to help guide runners to the shoe that is best for them as unique individuals.
Figure 5. Exemplar individual classified as exhibiting a low deviation when running (left, sock condition), and running in two different footwear of neutral classification. Shoe B (right) is pushing the runner away from their habitual motion path to exhibit a high deviation, despite being classified as neutral like shoe A (Middle). This suggests that wearing the wrong shoe can theoretically increase risk of injury, as a shoe can increase one’s habitual motion path deviation.
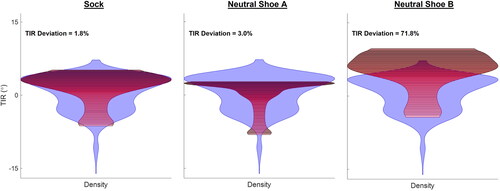
Continued advancements in footwear creation
One exciting aspect about the novel method presented in this paper is the new ability to holistically understand how one’s body is behaving relative to its non-running (habitual) state throughout stance phase. We can now understand the dynamic range of motion of an individuals’ joints in addition to their deviation. There may be implications for joint motion being an important factor for footwear prescription in addition to how much one deviates away from their HMP, notably within the amount of one’s biologically allowed range of motion (Rodrigues et al., Citation2013). Further work is needed to understand functional groups of runners based on their amount of joint motion relative to the amount of biologically allowed motion in addition to how much they deviate, and what footwear works best for them.
Utilising the full stance phase to quantify deviations creates the potential to understand how one deviates during various sub-phases of stance, e.g., braking vs. propulsion or kinematic gait events based upon foot angle progression. Further work is needed to study these more granular phases of stance, and we anticipate this will allow for a better understanding of human motion, fatigue effects, clinical gait conditions, and more thorough footwear design recommendations specifically focused on the heel, midfoot, or forefoot depending on how one deviates at different sub-phases of stance. Notably, as footwear has become taller (thicker stack height), these shoes have been associated with more ankle eversion in the second half of stance (Hannigan & Pollard, Citation2020; Sinclair et al., Citation2021), a prospectively identified aetiological injury mechanism (Becker et al., Citation2017; Citation2018; Hannigan & Pollard, Citation2021; Jungmalm et al., Citation2020). It is of importance to have advanced analysis tools to study the influence of footwear on a runner’s biomechanics to help best protect them during the entire stance phase. In regard to working towards personalised footwear, studying deviations during certain sub-phases such as early stance may be more important as this is where the most inter-individual variation in biomechanics and footwear effects occurs (Day & Baltich, Citation2021; Hoitz et al., Citation2021).
Limitations and future opportunities
Measuring frontal and transverse plane rotations is subject to error due to skin motion artefact (Leardini et al., Citation2005). When performing footwear assessments, researchers should be aware of the coupling and differences in range of motion between frontal and transverse plane rotations of the foot versus shoe (Alcantara et al., Citation2018; Jewell et al., Citation2017). Despite the relationship between transverse plane calcaneal rotation and tibial rotation (Fischer et al., Citation2018), we recommend not examining calcaneal rotation during shod running due to needing to cut holes in the heel counter which compromises fit and comfort of the shoe.
This new method utilises the external vGRF as a weighting factor for the run KDPs which are used to quantify HMP deviation. The translation of using the external vGRF weighting from level-ground or treadmill collections to real world running requires further investigation as external vGRF is not well correlated to internal loading [of the tibia] during graded running (Baggaley et al., Citation2022; Matijevich et al., Citation2019; Rice et al., Citation2023). As this new method advances the ability to holistically study the runner kinematically, a logical next step is to contextualise joint kinetics to the individual.
One aspect that a deviation does not encompass is the difference in rate at which movement occurs. While external vGRF loading rate, and subsequent strain rate of bone, is not conducive of stress fracture risk (Edwards, Citation2018; Loundagin et al., Citation2018); certain other soft tissue injuries may mechanistically be due to higher strain rates, such as iliotibial band syndrome (Hamill et al., Citation2008). While ensuring staying close to one’s HMP while running can help decrease some measures of joint loading (Willwacher et al., Citation2020), there is still a potential elevated risk due to running itself and the subsequent higher strain rates of tissues that may cause injury.
While this update was a key advancement in our kinematic understanding of the runner, there are more areas to focus future advancements in further understanding the runner and their interaction with footwear. Kinematically, it is important to understand a runner’s joint coupling behaviour, as this will influence how distal changes at the foot affect the knee (Fischer et al., Citation2018; Hintermann & Nigg, Citation1998). Understanding groups of runners (i.e., aerial vs. terrestrial) (Gindre et al., Citation2015; van Oeveren et al., Citation2021) is also of importance as differences in contact time, leg-stiffness, and other parameters may influence injury risk or how one interacts with footwear. Further research into the speed-dependency of these interactions is also warranted as speed affects cadence (Burns et al., Citation2019), joint-level mechanics (Day & Hahn, Citation2019; Orendurff et al., Citation2018), and whole-leg mechanics (Kulmala et al., Citation2018). Lastly, we also look towards a north star of subject-independent analyses to understand how to minimise soft-tissue loading and reduce injury risk. Tissue loading models with subject-specific parameters (Knaus & Blemker, Citation2021, Firminger et al., Citation2021) that can also account for the effects of modern materials such as increased Achilles stretch with soft foams (Esposito et al., Citation2022) to model stress/strain is a north star for helping protect runners from injury, as small changes in stress/strain carry elevated risk of tissue failure over numerous loading cycles (Edwards, Citation2018). Lastly, using statistical shape modelling of the lower extremity bones (Cerveri et al., Citation2020; Krähenbühl et al., Citation2020; Lenz et al., Citation2021; Schuster et al., Citation2024) to understand an individual’s habitual motion more accurately is of interest.
Conclusion/application
This methodological evolution increases our ability to understand the runner as a unique individual by quantifying their habitual motion at the ankle and knee during daily movements. Utilising several habitual daily movements and quantifying joint motion throughout the entire ground contact phase provides a more holistic understanding of one’s habitual motion path. Comparing ankle and knee kinematics during running to one’s habitual motion of the joints allows us to quantify one’s deviation on the run. For individuals that exhibit a large deviation from their HMP while running, supportive footwear technology can provide the extra guidance needed to return to their HMP. Quantifying the ability of footwear to help keep a runner close to their HMP (neutral products) or assist them back to their HMP (support products) is the primary method for how footwear is evaluated at Brooks. While a prospective study showing that minimising HMP deviations reduces injury risk has not yet been completed, we believe minimising one’s HMP deviation is one piece of the large puzzle in how to reduce one’s risk of running-related injury (Mai et al., Citation2022) and recommend that others implement the methodology described in this paper.
Looking forward there are many avenues of continued exploration to further understand runners and their interaction with products. Including but not limited to more in-depth investigation into types of deviators (ankle vs. knee, expanding beyond the bucket of high and low deviators); one’s amount of habitual motion and motion on the run, how different runner types uniquely interact with footwear, and running-speed dependency of footwear effects. Further, we look forward to the development of more robust analysis tools to study the runner and footwear effects.
Lastly, it is to be noted that this manuscript has solely focused on the biomechanics of understanding runners and the influence of footwear. The other key problem solved for in footwear testing is the experience that a runner has in a shoe. Different runners prioritise different aspects of a running shoe based upon personal preference (e.g., feel the ground or float above the ground on a thick stack of cushion) or the purpose of their run (Agresta et al., Citation2022). When creating footwear for runners, the experience of the shoe and the optimal biomechanical support both need to be taken into consideration, as different footwear construction techniques are used to create an experience (ex. soft and resilient foam or high bending stiffness), which may be more optimal for certain habitual motion paths over others.
Disclosure statement
No potential conflict of interest was reported by the author(s).
References
- Agresta, C., Giacomazzi, C., Harrast, M., & Zendler, J. (2022). Running injury paradigms and their influence on footwear design features and runner assessment methods: A focused review to advance evidence-based practice for running medicine clinicians. Frontiers in Sports and Active Living, 4, 815675. https://doi.org/10.3389/fspor.2022.815675
- Alcantara, R. S., Trudeau, M. B., & Rohr, E. S. (2018). Calcaneus range of motion underestimated by markers on running shoe heel. Gait & Posture, 63, 68–72. https://doi.org/10.1016/j.gaitpost.2018.04.035
- Baggaley, M., Derrick, T. R., Vernillo, G., Millet, G. Y., & Edwards, W. B. (2022). Internal tibial forces and moments during graded running. Journal of Biomechanical Engineering, 144(7), 011009. https://doi.org/10.1115/1.4053423
- Becker, J., James, S., Wayner, R., Osternig, L., & Chou, L. (2017). Biomechanical factors associated with achilles tendinopathy and medial tibial stress syndrome in runners. The American Journal of Sports Medicine, 45(11), 2614–2621. https://doi.org/10.1177/0363546517708193
- Becker, J., Nakajima, M., & Wu, W. F. W. (2018). Factors contributing to medial tibial stress syndrome in runners: A prospective study. Medicine and Science in Sports and Exercise, 50(10), 2092–2100. https://doi.org/10.1249/MSS.0000000000001674
- Boling, M., Padua, D., Marshall, S., Guskiewicz, K., Pyne, S., & Beutler, A. (2009). A prospective investigation of biomechanical risk factors for patellofemoral pain syndrome. The joint undertaking to monitor and prevent ACL injury. The American Journal of Sports Medicine, 37(11), 2108–2116. https://doi.org/10.1177/0363546509337934
- Bovens, A., Janssen, G., Vermeer, H., Hoeberigs, J., Janssen, M., & Verstappen, F. (1989). Occurrence of running injuries in adults following a supervised training program. International Journal of Sports Medicine, 10 Suppl 3(S3), S186–S190. https://doi.org/10.1055/s-2007-1024970
- Burns, G. T., Zendler, J. M., & Zernicke, R. F. (2019). Step frequency patterns of elite ultramarathon runners during a 100-km road race. Journal of Applied Physiology (Bethesda, Md.: 1985), 126(2), 462–468. https://doi.org/10.1152/japplphysiol.00374.2018
- Cerveri, P., Belfatto, A., & Manzotti, A. (2020). Predicting knee joint instability using a tibio-femoral statistical shape model. Frontiers in Bioengineering and Biotechnology, 8, 253. https://doi.org/10.3389/fbioe.2020.00253
- Cheung, R. T. H., Chung, R. C. K., & Ng, G. Y. F. (2011). Efficacies of different external controls for excessive foot pronation: a meta-analysis. British Journal of Sports Medicine, 45(9), 743–751. https://doi.org/10.1136/bjsm.2010.079780
- Clark, K. P., Meng, C. R., & Stearne, C. J. (2020). ‘Whip from the hip’: Thigh angular motion, ground contact mechanics, and running speed. Biology Open, 9(10), bio053546. https://doi.org/10.1242/bio.053546
- Davis, J., & Gruber, A. (2020). Injured runners do not replace lost running time with other physical activity. Medicine and Science in Sports and Exercise, 52(5), 1163–1168. https://doi.org/10.1249/MSS.0000000000002227
- Day, E., & Baltich, J. (2021). Planar ground reaction force timing ratios for different footwear groups. Footwear Science, 13(sup1), S11–S12. https://doi.org/10.1080/19424280.2021.1916611
- Day, E., & Gillette, J. (2019). Acute effects of wedge orthoses and sex on iliotibial band strain during overground running in nonfatiguing conditions. The Journal of Orthopaedic and Sports Physical Therapy, 49(10), 743–750. https://doi.org/10.2519/jospt.2019.8837
- Day, E., & Hahn, M. (2019). Dynamic angular stiffness about the metatarsophalangeal joint increases with running speed. Human Movement Science, 67, 102501. https://doi.org/10.1016/j.humov.2019.102501
- Dudley, R. I., Pamukoff, D. N., Lynn, S. K., Kersey, R. D., & Noffal, G. J. (2017). A prospective comparison of lower extremity kinematics and kinetics between injured and non-injured collegiate cross country runners. Human Movement Science, 52, 197–202. https://doi.org/10.1016/j.humov.2017.02.007
- Edwards, W. B. (2018). Modeling overuse injuries in sport as a mechanical fatigue phenomenon. Exercise and Sport Sciences Reviews, 46(4), 224–231. https://doi.org/10.1249/JES.0000000000000163
- Edwards, W. B., Derrick, T. R., & Hamill, J. (2012). Musculoskeletal attenuation of impact shock in response to knee angle manipulation. Journal of Applied Biomechanics, 28(5), 502–510. https://doi.org/10.1123/jab.28.5.502
- Esposito, M., Wannop, J. W., & Stefanyshyn, D. J. (2022). Effects of midsole cushioning stiffness on Achilles tendon stretch during running. Scientific Reports, 12(1), 4193. https://doi.org/10.1038/s41598-022-07719-x
- Farris, D. J., Kelly, L. A., Cresswell, A. G., & Lichtwark, G. A. (2019). The functional importance of human foot muscles for bipedal locomotion. Proceedings of the National Academy of Sciences of the United States of America, 116(5), 1645–1650. https://doi.org/10.1073/pnas.1812820116
- Firminger, C. R., Haider, I. T., Bruce, O. L., Wannop, J. W., Stefanyshyn, D. J., & Edwards, W. B. (2021). Are subject-specific models necessary to predict patellar tendon fatigue life? A finite element modelling study. Computer Methods in Biomechanics and Biomedical Engineering, 25(7), 729–739. https://doi.org/10.1080/10255842.2021.1975683
- Fischer, K. M., Willwacher, S., Arndt, A., & Brüggemann, G.-P. (2018). Calcaneal adduction and eversion are coupled to talus and tibial rotation. Journal of Anatomy, 233(1), 64–72. https://doi.org/10.1111/joa.12813
- Gindre, C., Lussiana, T., Hebert-Losier, K., & Mourot, L. (2015). Aerial and terrestrial patterns: A novel approach to analyzing human running. International Journal of Sports Medicine, 37(1), 25–29. https://doi.org/10.1055/s-0035-1555931
- Hamill, J., Miller, R., Noehren, B., & Davis, I. (2008). A prospective study of iliotibial band strain in runners. Clinical Biomechanics (Bristol, Avon), 23(8), 1018–1025. https://doi.org/10.1016/j.clinbiomech.2008.04.017
- Hannigan, J. J., & Pollard, C. D. (2020). Differences in running biomechanics between a maximal, traditional, and minimal running shoe. Journal of Science and Medicine in Sport, 23(1), 15–19. https://doi.org/10.1016/j.jsams.2019.08.008
- Hannigan, J. J., & Pollard, C. D. (2021). Biomechanical analysis of two runners who developed leg injuries during a six-week transition to maximal running shoes: A case series. Journal of Sports Sciences, 39(20), 2305–2311. https://doi.org/10.1080/02640414.2021.1930683
- Hespanhol Junior, L. C., Pena Costa, L. O., & Lopes, A. D. (2013). Previous injuries and some training characteristics predict running-related injuries in recreational runners: a prospective cohort study. Journal of Physiotherapy, 59(4), 263–269. https://doi.org/10.1016/S1836-9553(13)70203-0
- Hintermann, B., & Nigg, B. M. (1998). Pronation in runners: Implications for injuries. Sports Medicine (Auckland, N.Z.), 26(3), 169–176. https://doi.org/10.2165/00007256-199826030-00003
- Hoerzer, S., von Tscharner, V., Jacob, C., & Nigg, B. M. (2015). Defining functional groups based on running kinematics using Self-Organizing Maps and Support Vector Machines. Journal of Biomechanics, 48(10), 2072–2079. https://doi.org/10.1016/j.jbiomech.2015.03.017
- Hoffman, S. E., Peltz, C. D., Haladik, J. A., Divine, G., Nurse, M. A., & Bey, M. J. (2015). Dynamic in-vivo assessment of navicular drop while running in barefoot, minimalist, and motion control footwear conditions. Gait & Posture, 41(3), 825–829. https://doi.org/10.1016/j.gaitpost.2015.02.017
- Hoitz, F., von Tscharner, V., Baltich, J., & Nigg, B. M. (2021). Individuality decoded by running patterns: Movement characteristics that determine the uniqueness of human running. PloS One, 16(4), e0249657. https://doi.org/10.1371/journal.pone.0249657
- Holowka, N. B., Gillinov, S. M., Virot, E., & Lieberman, D. E. (2022). Effects of footwear cushioning on leg and longitudinal arch stiffness during running. Journal of Biomechanics, 133, 110869. https://doi.org/10.1016/j.jbiomech.2021.110869
- Jewell, C., Trudeau, M. B., Rohr, E., Brueggemann, P., Willwacher, S., Fischer, K. M., & Hamill, J. (2017). Calcaneal movement measured by skin versus shoe-mounted markers. Footwear Science, 9(sup1), S72–S73. https://doi.org/10.1080/19424280.2017.1314345
- Jungmalm, J., Nielsen, R. O., Desai, P., Karlsson, J., Hein, T., & Grau, S. (2020). Associations between biomechanical and clinical/anthropometrical factors and running-related injuries among recreational runners: a 52-week prospective cohort study. Injury Epidemiology, 7(1), 10. https://doi.org/10.1186/s40621-020-00237-2
- Kakouris, N., Yener, N., & Fong, D. (2021). A systematic review of running-related musculoskeletal injuries in runners. Journal of Sport and Health Science, 10(5), 513–522. https://doi.org/10.1016/j.jshs.2021.04.001
- Kalkhoven, J. T., Watsford, M. L., & Impellizzeri, F. M. (2020). A conceptual model and detailed framework for stress-related, strain-related, and overuse athletic injury. Journal of Science and Medicine in Sport, 23(8), 726–734. https://doi.org/10.1016/j.jsams.2020.02.002
- Ker, R. F., Bennett, M. B., Bibby, S. R., Kester, R. C., & Alexander, R. M. (1987). The spring in the arch of the human foot. Nature, 325(7000), 147–149. https://doi.org/10.1038/325147a0
- Kluitenberg, B., van Middelkoop, M., Diercks, R., & van der Worp, H. (2015). What are the differences in injury proportions between different populations of runners? A systematic review and meta-analysis. Sports Medicine (Auckland, N.Z.), 45(8), 1143–1161. https://doi.org/10.1007/s40279-015-0331-x
- Knaus, K. R., & Blemker, S. S. (2021). 3D Models reveal the influence of achilles subtendon twist on strain and energy storage. Frontiers in Bioengineering and Biotechnology, 9, 539135. https://doi.org/10.3389/fbioe.2021.539135
- Krähenbühl, N., Lenz, A. L., Lisonbee, R. J., Peterson, A. C., Atkins, P. R., Hintermann, B., Saltzman, C. L., Anderson, A. E., & Barg, A. (2020). Morphologic analysis of the subtalar joint using statistical shape modeling. Journal of Orthopaedic Research: Official Publication of the Orthopaedic Research Society, 38(12), 2625–2633. https://doi.org/10.1002/jor.24831
- Kuhman, D. J., Paquette, M. R., Peel, S. A., & Melcher, D. A. (2016). Comparison of ankle kinematics and ground reaction forces between prospectively injured and uninjured collegiate cross country runners. Human Movement Science, 47, 9–15. https://doi.org/10.1016/j.humov.2016.01.013
- Kulmala, J.-P., Kosonen, J., Nurminen, J., & Avela, J. (2018). Running in highly cushioned shoes increases leg stiffness and amplifies impact loading. Scientific Reports, 8(1), 17496. https://doi.org/10.1038/s41598-018-35980-6
- Leardini, A., Chiari, L., Della Croce, U., & Cappozzo, A. (2005). Human movement analysis using stereophotogrammetry: Part 3. Soft tissue artifact assessment and compensation. Gait & Posture, 21(2), 212–225. https://doi.org/10.1016/j.gaitpost.2004.05.002
- Lenz, A. L., Krähenbühl, N., Peterson, A. C., Lisonbee, R. J., Hintermann, B., Saltzman, C. L., Barg, A., & Anderson, A. E. (2021). Statistical shape modeling of the talocrural joint using a hybrid multi-articulation joint approach. Scientific Reports, 11(1), 7314. https://doi.org/10.1038/s41598-021-86567-7
- Loegering, I. F., Rasske, K., Berthiaume, C., & Trudeau, M. B. (2021). A novel method for estimating an individual’s habitual cadence across a range of running speeds from GPS running watch data. Footwear Science, 13(sup1), S61–S62. https://doi.org/10.1080/19424280.2021.1917682
- Lohmander, L., Ostenberg, A., Englund, M., & Roos, H. (2004). High prevalence of knee osteoarthritis, pain and functional limitations in female soccer players twelve years after anterior cruciate ligament injury. Arthritis and Rheumatism, 50(10), 3145–3152. https://doi.org/10.1002/art.20589
- Loundagin, L. L., Schmidt, T. A., & Edwards, W. B. (2018). Mechanical fatigue of bovine cortical bone using ground reaction force waveforms in running. Journal of Biomechanical Engineering, 140(3), 0310031–0310035. https://doi.org/10.1115/1.4038288
- Lun, V., Meeuwisse, W., Stergiou, P., & Stefanyshyn, D. (2004). Relation between running injury and static lower limb alignment in recreational runners. British Journal of Sports Medicine, 38(5), 576–580. https://doi.org/10.1136/bjsm.2003.005488
- Mahieu, N., Witvrouw, E., Stevens, V., Van Tiggelen, D., & Roget, T. (2006). Intrinsic risk factors for the development of achilles tendon overuse injury: A prospective study. The American Journal of Sports Medicine, 34(2), 226–235. https://doi.org/10.1177/0363546505279918
- Mai, P., Robertz, L., Robbin, J., Thelen, M., Kurz, M., Trudeau, M. B., Weir, G., Hamill, J., & Willwacher, S. (2022). An analytical framework to understand individual running-related injury risk response patterns to footwear. Proceedings of the Institution of Mechanical Engineers, Part P: Journal of Sports Engineering and Technology, 1–13. https://doi.org/10.1177/17543371221100044
- Matijevich, E. S., Branscombe, L. M., Scott, L. R., & Zelik, K. E. (2019). Ground reaction force metrics are not strongly correlated with tibial bone load when running across speeds and slopes: Implications for science, sport and wearable tech. PloS One, 14(1), e0210000. https://doi.org/10.1371/journal.pone.0210000
- Meardon, S. A., Willson, J. D., Kernozek, T. W., Duerst, A. H., & Derrick, T. R. (2018). Shoe cushioning affects lower extremity joint contact forces during running. Footwear Science, 10(2), 109–117. https://doi.org/10.1080/19424280.2018.1501771
- Nigg, B. M. (2001). The role of impact forces and foot pronation: A new paradigm. Clinical Journal of Sport Medicine: Official Journal of the Canadian Academy of Sport Medicine, 11(1), 2–9. https://doi.org/10.1097/00042752-200101000-00002
- Nigg, B. M., Baltich, J., Hoerzer, S., & Enders, H. (2015). Running shoes and running injuries: mythbusting and a proposal for two new paradigms: ‘Preferred movement path’ and ‘comfort filter. British Journal of Sports Medicine, 49(20), 1290–1294. https://doi.org/10.1136/bjsports-2015-095054
- Nigg, B. M., Behling, A.-V., & Hamill, J. (2019). Foot pronation. Footwear Science, 11(3), 131–134. https://doi.org/10.1080/19424280.2019.1673489
- Nigg, B. M., & Segesser, B. (1992). Biomechanical and orthopedic concepts in sport shoe construction. Medicine & Science in Sports & Exercise, 24(5), 595–602. https://doi.org/10.1249/00005768-199205000-00014
- Nigg, B. M., Vienneau, J., Smith, A. C., Trudeau, M. B., Mohr, M., & Nigg, S. R. (2017). The preferred movement path paradigm: Influence of running shoes on joint movement. Medicine and Science in Sports and Exercise, 49(8), 1641–1648. https://doi.org/10.1249/MSS.0000000000001260
- Orendurff, M. S., Kobayashi, T., Tulchin-Francis, K., Tullock, A. M. H., Villarosa, C., Chan, C., Kraus, E., & Strike, S. (2018). A little bit faster: Lower extremity joint kinematics and kinetics as recreational runners achieve faster speeds. Journal of Biomechanics, 82, 404–175. https://doi.org/10.1016/j.jbiomech.2018.10.034
- Paquette, M. R., & Miller, R. H. (2018). Reconciling new with old injury paradigms and the need to dig deeper – comment on Nigg et al. Current Issues in Sport Science, 3, 105.
- Paquette, M. R., Napier, C., Willy, R. W., & Stellingwerff, T. (2020). Moving beyond weekly “distance”: Optimizing quantification of training load in runners. journal of Orthopaedic & Sports Physical Therapy, 50(10), 564–569. https://doi.org/10.2519/jospt.2020.9533
- Powers, C. (2010). The influence of abnormal hip mechanics on knee injury. A biomechanical perspective. The Journal of Orthopaedic and Sports Physical Therapy, 40(2), 42–51. https://doi.org/10.2519/jospt.2010.3337
- Rice, H., Kurz, M., Mai, P., Robertz, L., Bill, K., Derrick, T. R., & Willwacher, S. (2023). Speed and surface steepness affect internal tibial loading during running. Journal of Sport and Health Science, 13(1), 118–124. https://doi.org/10.1016/j.jshs.2023.03.004
- Rice, H., Weir, G., Trudeau, M., Meardon, S., Derrick, T., & Hamill, J. (2019). Estimating tibial stress throughout the duration of a treadmill run. Medicine and Science in Sports and Exercise, 51(11), 2257–2264. https://doi.org/10.1249/MSS.0000000000002039
- Rodrigues, P., TenBroek, T., & Hamill, J. (2013). Runners with anterior knee pain use a greater percentage of their available pronation range of motion. Journal of Applied Biomechanics, 29(2), 141–146. https://doi.org/10.1123/jab.29.2.141
- Rudzki, S. (1997). Injuries in Australian Army recruits. Part I: Decreased incidence and severity of injury seen with reduced running distance. Military Medicine, 162(7), 472–476. https://doi.org/10.1093/milmed/162.7.472
- Schuster, R., Cresswell, A., & Kelly, L. (2024). Human foot form and function: Variable and versatile, yet sufficiently related to predict function from form. Proceedings of the Royal Society B, 291.
- Sinclair, J., Brooks, D., Taylor, P. J., & Liles, N. B. (2021). Effects of running in minimal, maximal and traditional running shoes: A musculoskeletal simulation exploration using statistical parametric mapping and Bayesian analyses. Footwear Science, 13(2), 143–156. https://doi.org/10.1080/19424280.2021.1892834
- Stearne, S. M., McDonald, K. A., Alderson, J. A., North, I., Oxnard, C. E., & Rubenson, J. (2016). The foot’s arch and the energetics of human locomotion. Scientific Reports, 6(1), 19403. https://doi.org/10.1038/srep19403
- Stefanyshyn, D., Stergiou, P., Lun, V., Meeuwisse, W., & Worobets, J. (2006). Knee angular impulse as a predictor of patellofemoral pain in runners. The American Journal of Sports Medicine, 34(11), 1844–1851. https://doi.org/10.1177/0363546506288753
- Taunton, J. E., Ryan, M. B., Clement, D. B., Mckenzie, D. C., Lloyd-Smith, D. R., & Zumbo, B. D. (2002). A retrospective case-control analysis of 2002 running injuries. British Journal of Sports Medicine, 36(2), 95–101. https://doi.org/10.1136/bjsm.36.2.95
- Trudeau, M. B., Willwacher, S., Weir, G., Rohr, E., Ertel, C., Bruggemann, G.-P., & Hamill, J. (2019). A novel method for estimating an individual’s deviation from their habitual motion path when running. Footwear Science, 11(3), 135–145. https://doi.org/10.1080/19424280.2019.1615004
- Van der Worp, M. P., ten Haaf, D. S. M., van Cingel, R., de Wijer, A., Nijhuis-van der Sanden, M. W. G., & Staal, J. B. (2015). Injuries in runners; A systematic review on risk factors and sex differences. PloS One, 10(2), e0114937. https://doi.org/10.1371/journal.pone.0114937
- van Oeveren, B. T., de Ruiter, C. J., Beek, P. J., & van Dieën, J. H. (2021). The biomechanics of running and running styles: A synthesis. Sports Biomechanics, 23(4), 516–554. https://doi.org/10.1080/14763141.2021.1873411
- Warden, S. J., Edwards, W. B., & Willy, R. W. (2021). Preventing bone stress injuries in runners with optimal workload. Current Osteoporosis Reports, 19(3), 298–307. https://doi.org/10.1007/s11914-021-00666-y
- Willems, T. M., Ley, C., Goetghebeur, E., Theisen, D., & Malisoux, L. (2021). Motion-control shoes reduce the risk of pronation-related pathologies in recreational runners: A secondary analysis of a randomized controlled trial. The Journal of Orthopaedic and Sports Physical Therapy, 51(3), 135–143. https://doi.org/10.2519/jospt.2021.9710
- Willwacher, S., Mählich, D., Trudeau, M. B., Hamill, J., Weir, G., Brüggemann, G.-P., & Bratke, G. (2020). The habitual motion path theory: Evidence from cartilage volume reductions in the knee joint after 75 minutes of running. Scientific Reports, 10(1), 1363. https://doi.org/10.1038/s41598-020-58352-5
- Willwacher, S., Kurz, M., Robbin, J., Thelen, M., Hamill, J., Kelly, L., & Mai, P. (2022). Running-related biomechanical risk factors for overuse injuries in distance runners: A systematic review considering injury specificity and the potentials for future research. Sports Medicine (Auckland, N.Z.), 52(8), 1863–1877. https://doi.org/10.1007/s40279-022-01666-3
- Willy, R., Halsey, L., Hayek, A., Johnson, H., & Willson, J. (2016). Patellofemoral joint and achilles tendon loads during overground and treadmill running. The Journal of Orthopaedic and Sports Physical Therapy, 46(8), 664–672. https://doi.org/10.2519/jospt.2016.6494
- Wilson, D., Feikes, J., Zavatsky, A., Bayona, F., & O’Connor, J. (1996). The one degree-of-freedom nature of the human knee joint – basis for a kinematic model. In J. A. Hoffer, A. Chapman, & J. J. Eng (Eds.), Proceedings of the ninth biennial conference of the Canadian society for biomechanics (pp. 194–195). Conference Organizing Committee.
- Wilson, D. R., Feikes, J. D., Zavatsky, A. B., & O’Connor, J. J. (2000). The components of passive knee movement are coupled to flexion angle. Journal of Biomechanics, 33(4), 465–473. https://doi.org/10.1016/s0021-9290(99)00206-7