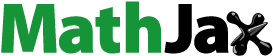
ABSTRACT
Information and Communication Technology (ICT) is advocated to contribute to sustainable transportation by prompting a change in travel patterns. Therefore, using an ICT-enabled city region in India as the study context, the objectives of the study were to examine the influence of ICT on travel patterns in city regions and explore the strategies to enhance ICT usage for positively changed travel patterns. Findings suggest that definite linkages between ICT usage and travel patterns exist. An increase in ICT usage in daily activities of people will enable a significant reduction in trip generation, travel distance and travel time. Strategic interventions including enhancing networking and ICT connectivity, provisioning free Wi-Fi hot spots at important nodal points, and enabling the availability of real-time road transportation-related information can enhance ICT usage leading to a positively changed travel pattern. This is likely to improve traffic movement and reduce congestion contributing to sustainable urban road transportation.
1. Introduction
Sustainable road transportation in cities of developing countries, particularly in India is a serious challenge. Poor road transportation system engenders economic losses and exacerbates environmental degradation (Thynell et al. Citation2010; Tiwari et al. Citation2011). Indian cities are observed to be battling with various road transportation-linked challenges that include road congestion, road safety, delay and travel time increase, etc., which cause sustainability challenges in road transportation. Moreover, such challenges lead to a loss in working labour hours, hampering the economy as well as causing environmental pollution. Several large and medium cities in the country namely Delhi, Mumbai, Hyderabad, Bengaluru, Chennai, Pune, Ahmadabad, Bhubaneswar, etc., suffer from such challenges. For example, in recent years Delhi has been termed one of the most congested and polluted cities in the world. In this regard, travel characteristics and travel patterns in terms of trip generation, distance travel, route choice, use of the type of vehicles, etc., to perform everyday life of people are argued to have a significant impact on the sustainability of the road transportation system (Weiyu and Arul Citation2014; Emuze and Das Citation2015; Hong and Thakuriah Citation2016; Pawlak et al. Citation2020). While the high volume of vehicles − particularly individually driven private vehicles on the roads is causing severe traffic congestion, the use of fossil fuels such as diesel, petrol or compressed natural gases (CNG) is observed to be a major reason for serious environmental pollution. To curb such challenges, the Government of Delhi in 2016 applied an odd and even formula for the use of vehicles − that is, plying vehicles with odd registration numbers on odd-number dates and vehicles with even registration numbers on even-numbered dates on a pilot study. The primary aim of this system was to reduce the use of private vehicular travel and pollution in the city (Hindustan Times Citation2016). Although the results of the study were inconclusive, there was an indication that vehicular congestion can be manageable, and pollution can be reduced if privately (by individuals) driven vehicles are reduced (www.quora.com Citation2016).
Consequently, arguments have emerged that there is a need for change in travel patterns and travel characteristics of people in the cities of India. It is advocated that Information and Communication Technology (ICT) can engender such changes, which consequently can alleviate road transportation-related challenges (Das Citation2014; Das and Emuze Citation2014; Emuze and Das Citation2015). Evidence from European and North American cities, in support of this argument, has shown that the effective use of ICT and smart mobility can influence travel patterns, travel characteristics, economy, and environment positively and consequently make the cities smart and sustainable (Giffinger et al. Citation2007; Yigitcanlar et al. Citation2008; Lombardi Citation2011; Das and Emuze Citation2014). However, some studies suggest a negative association between ICT usage and travel pattern (van Wee et al. Citation2013; Kroesen and Handy Citation2015). For example, ICT use might enhance the generation of trips (Hong and Thakuriah Citation2016). Thus, the impact of ICT on travel patterns is observed to be mixed (van Wee et al. Citation2013; Hong and Thakuriah Citation2016; Delbosc and Mokhtarian Citation2018; G¨ossling Citation2018; Lachapelle and Jean-Germain Citation2019; Yin et al. Citation2021).
Nevertheless, the literature suggests that concerted efforts have been made to meet the mobility challenges in the cities over the years. Intelligent Transportation System (ITS), which is largely founded on ICT remained pivotal in alleviating transportation-related challenges and enhancing efficient transportation over the years (Ng et al. Citation1995; Adler and Blue Citation1998; Hameri and Paatela Citation2005; Thynell et al. Citation2010). As a result, road safety, reduction in congestion and increase in road capacities have been experienced. However, because of the increase in vehicular travel by use of individually driven private vehicles, it is argued that ITS cannot alone solve the problems of road transportation; and may also exacerbate certain costs, particularly related to the environment (Goldman and Gorham Citation2006), thereby aggravating the already existing sustainability challenges of road transportation.
Furthermore, several empirical studies suggest that travellers make their travel decisions and route choices depending upon numerous criteria that include travel cost, travel time and reliability, traffic safety, track comfort, roadway characteristics, utility, information supply, socio-economic and demographic characteristics, and behavioural considerations (Ng et al. Citation1995; Kanninen Citation1996; Adler and Blue Citation1998; Yang and Meng Citation2001; Hameri and Paatela Citation2005; Chen and Ting Citation2007; Lombardi Citation2011; Martínez-Torres et al. Citation2013). However, the most important criterion that influences travel patterns is the minimisation of travel time, which rests on the traffic assignment and in effect on the traffic volume (Chen et al. Citation2001; Yang and Meng Citation2001; Thynell et al. Citation2010; Martínez-Torres et al. Citation2013).
Therefore, it is contended that while ITS assists in the route selection and minimisation of travel time, the use of ICT in socio-economic activities of people would possibly reduce the need for trip generation (as traffic volume is dependent on the need for trip generation) through travel substitution and ridesharing (Das Citation2014; Weiyu and Arul Citation2014; Emuze and Das Citation2015; Cohen-Blankshtain and Rotem-Mindali Citation2016; G¨ossling Citation2018). Moreover, it also can enhance mobility thereby reducing travel time by suggesting a set of choices such as appropriate mode of travel, travel route, estimated travel time required to reach a destination and so on (G¨ossling Citation2018).
However, the literature suggests that the linkages between ICT use and travel patterns have not been explored empirically and explicitly so far specifically in the context of developing countries such as India. Therefore, as a point of departure this study entails exploring whether ICT use by households irrespective of road infrastructural or ITS provisions in a congested city region could influence the travel patterns of people, and if so how and to what extent. Furthermore, since strategies for the provision of ICT in a city are general in nature, it was sought to unravel what specific strategies would enable higher use of ICT, which would bring about change in travel patterns. Since travel pattern is a consequence of the plausible travel behaviour of people, the Theory of Planned Behaviour (TPB) was used as the theoretical premise of the study (Ajzen Citation1991) (c.f: Literature review section).
Therefore, the objectives of this study were
To examine the influence of the use of ICT on the travel patterns of people at the household level in a city region of India that would contribute to sustainable road transportation.
To evaluate ICT-related strategic interventions that would specifically influence the travel patterns of the people of the city region.
In this context of the first objective, by using a case study of an ICT-enabled major city region − Pune in India, this study specifically examined whether ICT use by people in their socio-economic and travel activities assists in a reduction in trip generation, distance travelled and reduction in travel time and if so to what extent. In this context, a hypothesis was framed, which was divided into four sub-hypotheses and tested. The hypothesis was premised on the argument that mobility challenges can be alleviated efficiently and sustainability of road transportation system can be enhanced with the use of advanced technological interventions such as ICT (Ng et al. Citation1995; Adler and Blue Citation1998; Hameri and Paatela Citation2005; Thynell et al. Citation2010). The hypothesis and sub-hypotheses are presented below.
Hypothesis (H): The use of ICT in everyday socio-economic activities of households will reduce vehicular travel that will contribute to sustainable road transportation in terms of reduction in trip generation, reduction in travel distance and reduction in travel time.
The four sub-hypotheses are:
HS1: An increase in ICT usage by households will reduce the number of trips by households
HS2: An increase in ICT usage by households will reduce the average distance travelled per trip
HS3: An increase in ICT usage by households will reduce the average distance travelled by households
HS4: An increase in ICT usage by households will reduce the travel time of households
Furthermore, concerning the second objective, a set of strategies was evaluated to observe the crucial strategies that would reinforce the use of ICT in socio-economic and transportation activities, which consequently influence the travel patterns of people positively in a city region.
2. Literature review
2.1. ICT, its impact on road transportation and travel pattern
At the outset, defining and scoping ICT in the specific context of this study was necessary. ICT in general is defined as a diverse set of technological tools and resources which enable the transmission, storing, creation, sharing or exchange of information. In the specific context of this study, the various ICT tools include computers/laptops connected to the internet, smart mobile phones, Tablets, Smartwatches, Smart Mobile Apps, and so on. The various ICT-enabled digital platforms are websites, digital meeting and conferencing platforms such as MS Team, Zoom, smart mobile applications, social media, digital live maps (such as Google Maps), and so on (Germann Molz Citation2012; van den Berg et al. Citation2013), which directly or indirectly are linked to travel behaviour and travel patterns of people through various socio-economic and travel-related activities.
ICT has influenced socio-economic activities across the world. Similarly, it has also impacted various activities related to transportation. For example, ICT by use of the above-mentioned digital instruments can offer travel information systems such as routes, travel time, travel delay, congestion, parking facilities, etc., based on which a traveller can decide on travel ahead. Similarly, ICT can enable one to work from home or perform activities online without physical travel, thereby reducing trip generation and traffic on the roads (Tseng et al. Citation2013; Hu et al. Citation2020). However, it excludes ITS-actuated instruments such as roadside information boards, automated synchronised or sensor-enabled traffic control systems or vehicle-to-vehicles communications.
Initially, transport policymakers have hoped that ICT would offer a substitute for travel, although over time this early optimism has been reduced (De Graaff and Rietveld Citation2007; van Wee et al. Citation2013) because it was argued that people prefer face-to-face interaction and ICT cannot fully compensate for the richness of face-to-face contact (Larsen et al. Citation2007; Aguilera Citation2008). Furthermore, in addition, to travel substitution, ICT for complementarity or induction of travel, modification or neutrality of travel have been argued. In this context, it was found that ICT might generate additional travel (van Wee et al. Citation2013) or assist in modifying the travel pattern and behaviour of people (Salomon Citation2000; Mokhtarian and Salomon Citation2002; Aguiléra et al. Citation2012; Silva et al. Citation2017). Nevertheless, it was construed that with technological advancements’ ICT is likely to impact travel behaviour and consequently travel patterns significantly (Tseng et al. Citation2013; Hu et al. Citation2020). For example, according to Lenz and Nobis (Citation2007), ICT leads to a reorganisation of activities in time and space, which impacts travel behaviour and pattern. In other words, if activities are fragmented over space and time by the use of ICT, then they can be conducted differently. For example, as mentioned previosuly some of the activities can be performed by use of ICT such as e-shopping or Zoom meetings as seen in current times, thereby reducing the need for vehicular travel, whereas some activities would generate additional travel demand.
Previous studies on the relationships between ICT and travel behaviour and travel pattern provided mixed results (Choo et al. Citation2007; Farag et al. Citation2007; Mokhtarian Citation2009; Hong and Thakuriah Citation2016; Delbosc and Mokhtarian Citation2018; Lachapelle and Jean-Germain Citation2019). For example, some studies observed a positive association between ICT use and trip generation (Hong and Thakuriah Citation2016). On the other hand, according to Kroesen and Handy (Citation2015), ICT use frequency is negatively associated with travel behaviour. Also, it can lead to a shift in modal choices because it makes public transit and nonmotorized modes reliable and improves their quality thus more attractive (Bhat and Sardesai Citation2006; G¨ossling Citation2018) and might make car ownership redundant (Delbosc and Currie Citation2014). On the other hand, ICT can make driving more convenient, thus enhancing the car ownership demand (G¨ossling Citation2018; Yin et al. Citation2021). Thus, premised on empirical findings it is not clear whether the use of ICT leads to an increase in travel demand for people or has a small reducing effect on the mobility level of people (van Wee et al. Citation2013). Moreover, the complex interactions between road transportation and ICT usage have not thus resulted in a straightforward linkage between ICT usage and travel patterns, specifically for the reduction of travel.
Therefore, although initial policy expectations were that ICT would greatly contribute to more efficient use of transport infrastructure by spreading travellers across modes, routes, and departure times or in other words by changing the travel behaviour and pattern of people (Commission of the European Communities Citation2001; Federal Transit Administration Citation2003; Department for Transport Citation2004), it was needed to be adjusted (Chorus et al. Citation2006; Chorus et al. Citation2007; Farag and Lyons Citation2010; Pawlak et al. Citation2020). In this context, some scholars observed that since travel behaviour and travel pattern are influenced by people’s social network characteristics, and the propensity to perform social activities (Carrasco and Miller Citation2006), ICT can act as a catalyst in social interaction, thus influencing travel behaviour and travel pattern (Wellman et al. Citation2006; Yin et al. Citation2021).
So, arguments can be made for a better understanding of how ICT influences travel patterns in specific contexts of cities in developing countries. In other words, what is the impact of ICT usage on the reduction of travel in terms of the number of trips, travel distance and travel time needs to be examined, which remains contested so far (Hong and Thakuriah Citation2016; Kroesen and Handy (Citation2015), and thus a knowledge gap. Furthermore, such aspects have not been studied explicitly and the relationships have not been established empirically in the congested cities of developing countries such as India, which necessitated this study.
2.2. Theory of planned behaviour and travel pattern
The Theory of Planned Behaviour (TPB) is used to understand and predict the behaviour of people under certain circumstances (Ambali and Bakar Citation2015). It postulated that behaviours are immediately determined by behavioural intentions and under certain conditions, perceived behavioural control. Behavioural intentions are determined by a combination of three factors which include attitudes towards the behaviour, subjective norms, and perceived behavioural control (Ajzen Citation1991; Kan and Fabrigar Citation2017). This theory offers the advantage of predicting behaviour when people have incomplete voluntary control. In other words, it predicts the behaviour when people are given opportunities and facilities, or compelled by circumstances to behave in a particular way (Ambali and Bakar Citation2015; Shaw Citation2016). TPB has been applied to predict the behaviour of people in transportation activities. For example, it has been used to predict pedestrian behaviour (Shoabjareh, Mamdoohi, ANordfjærn, Citation2021).
According to this theory, people hold certain beliefs that influence behaviour and these beliefs are most often linked to a certain outcome. The travel behaviour and travel pattern of people are generally influenced by a set of factors that include residential selection and urban density, daily travel activity pattern, vehicle ownership, trip and route frequency, route choice and mode choice (Mwale et al. Citation2022). However, the role of ICT has not been explored and emphasised. In this context, two nuances of TPB can be vital for exploring travel patterns. First, TPB can be used to predict the travel pattern of people based on the use of ICT in socioeconomic and travel activities. Second, ICT can enable change in socio-economic and travel activities through planned behaviour, consequently influencing the travel pattern of people. Therefore, in this context, ICT-linked strategic interventions were explored to observe the most influential strategies that would lead to enhanced use of ICT in the socio-economic and travel activities of people. It is advocated that it would lead to planned travel behaviour as proposed by TPB, which consequently would positively influence the travel patterns in the city and contribute to sustainable transportation.
2.3. Methodological approaches for understanding travel pattern
Extant literature suggests that several studies related to travel pattern and travel behaviour have been conducted using a variety of methodological approaches. The important methodological approaches used by the scholars include ordinary least squares (OLS), Poisson regression (PR), geographically weighted regression models (GWRM), multinomial logit (MNL), nested logit (NL), structural equation models (SEM) and qualitative methods (QM) (Mwale et al. Citation2022). Each model has its strengths and limitations. Some of the limitations of these models could be complicated model estimation, challenges in the requirement of adequate sample size, the model may not perform well if data has outliers and so on (Mwale et al. Citation2022). However, since travel pattern is dependent on many unobserved heterogeneous economic, temporal, spatial social and demographic characteristics (Lee et al. Citation2022; Mwale et al. Citation2022), there is also a need to understand the influence of randomness and unobserved heterogeneity. Therefore, a methodological approach integrating unobserved heterogeneity or random effect was deemed pertinent (Singh et al. Citation2020).
3. Study context: Pune city region, India
A major ICT-enabled city region in western India, named ‘Pune’ was taken as the case study.
3.1. A brief overview of the location, demographic, and socio-economic profile
The Pune city region is situated between 180 32’ North and 730 51’ East and is located about 170.00 Kilometers (km) southeast of Mumbai city by road. The city region constitutes Pune Municipal Corporation and the adjoining urban areas such as Pimpri – Chinchwad Municipal Corporation in the North-west, Pune Cantonment Board, Kirkee Cantonment Board, Dehuroad Cantonment Board, and Dehu-a census town. It is the seventh-largest city and the eighth-largest metropolitan economy having the highest per capita income in India with the least income disparity. It has a population of about 6 million and is spread over more than 500 Square Kilometers of land area. It constitutes but is not limited to slum dwellers, the industrial workforce, working-class people, knowledge workers and elite industrialists. About 62% of people are under the age of 30 with a median age of 24. The working population in the city is about 34% (Pai et al. Citation2014). The average number of household members ranges between 4.5 in suburban areas and 5.2 for working-class families in industrial areas to an average of 4.64 (http://www.yourarticlelibrary.com). Pune region city is cosmopolitan, and heterogeneous considering the socio-economic strata.
It is well known for its educational facilities and cultural attributes. However, it has become one of the most industrialised cities, particularly in the Western region of the country engaged in glass, sugar, forging, automobile manufacturing, and ICT industries. The availability of adequate basic urban infrastructure facilities including the existence of relatively better transport and communication services, the presence of skilled manpower and its proximity to Mumbai (regarded as the financial capital of India) have in particular helped the establishment of several domestic and multinational ICT companies, attracted huge investment in this sector and emerged as an ICT hub and one of the major ICT enabled cities in the country in recent years.
3.2. ICT profile
ICT has a considerable presence in the city. It includes the use of the internet, web services and smart digital services such as smart mobile phones, computers/laptops Tablets, Smartwatches, Smart Mobile Apps, etc., connected with the internet in the city. The services are provided by several private service providers through broadband, and wireless services with bandwidth ranging from 2.5 Mbps to 100 Mbps (Gadgets 360 Citationn.d.). People can access the internet through broadband connections, wireless connections, and hotspots as well as through their smart cell phones. Usually, the services are provided by different private or corporate service providers and government agencies. It is observed that ICT is influencing the socio-economic and everyday activities of people in the city significantly.
3.3. Road transportation profile
The fast growth of the city is creating pressure on the urban infrastructure, particularly in the transportation sector. It is found that currently there is a large need for daily movement in the city. The purpose of such daily travel includes but is not limited to travelling to workplaces (offices, industries, commercial activities, etc.), educational institutions (travelling to – and picking up and dropping off kids in schools and colleges), entertainment, cultural activities, sporting activities, etc.
To meet the challenges of daily travel people are resorting to individual-driven vehicles such as cars and motorbikes, which have increased the volume of such vehicles and consequent significant generation of trips. For example, the vehicle volume has increased 87 times as against the four times increase in population in the city since the 1960s. Currently, about 53% of households have motorbikes and 13% have cars in the city region. Perhaps the city region has the highest vehicle ownership in the country (450 vehicles per 1000 people) (PMC Citation2006; Pai et al. Citation2014). According to vehicle registration in Pune, 88.00% of the total number of vehicles are private vehicles, out of which 75.00% are motorcycles. The number of drivers estimated from the survey of this study ranges from 0 to 4 with an average of 1.8 drivers per household (in the sample households).
Under the public transportation system, Pune has both bus and local train systems. However, only about 0.80% of vehicles are buses. Pune Mahanagar Parivahan Mahamandala Limited (PMPML) (engaged in metropolitan transportation) operates about 1300 buses per day to facilitate local accessibility. Similarly, local trains (EMU) through its two lines connect the suburban and industrial areas in and around the city offering transportation facilities to about 100,000 people per day. Besides, the Bus Rapid Transit system known as Rainbow BRT was opened in August 2015 ().
Table 1. Profile of road transportation in the study context.
However, it is observed that the transportation system remained a challenge in the city concerning capacity, congestion, and safety. For, example, sometimes congestion becomes so acute during certain parts of the day that it might take as much as 3.0 hours to travel a distance of 5 Kilometers (Rangarajan Citation2010) by road. This results in to increase in travel time and delay and consequently loss of productive labour hours (man-hours), which in effect incur a loss to the Gross Domestic Product (GDP) based on employee contributions. Furthermore, the higher need for travel, vehicle use, increased travel time and travel distance cause higher carbon emissions and pollution (Global Sustainable Systems Research Report, Citation2004). Thus, it is imperative to explore to what extent the use of ICT in daily urban and transportation activities of people in the city can reduce the need for vehicular travel, distance travelled, and travel time or in other words change in travel pattern.
4. Research methods
The study was performed by using a survey research method to collect data and quantitative statistical analyses.
4.1. Data collection
4.1.1. Households survey
A survey research method was used to collect data. For this purpose, a household survey was conducted using a questionnaire. The sample survey was conducted in six important locations in the city to represent its heterogeneity. The household survey was conducted in different wards (administrative divisions) of six representative sub-regions, such as Pune Municipal Corporation in the South-east, Pimpri – Chinchwad Municipal Corporation in the North-west, Pune Cantonment Board, Kirkee Cantonment Board, Dehuroad Cantonment Board, and Dehu-a census town of the city (). The wards for the survey in the six sub-regions were selected based on their geographical locations, demographic characteristics, availability of industrial and other economic activity areas, density, and transportation connectivity to represent the heterogeneity of the city.
Variables relating to ICT accessibility, the intensity of ICT use, and the influence of ICT on transportation and socio-economic activities were included in the survey questionnaires. The variables used for accessibility of ICT include the availability of ICT instruments such as Laptops, Tablets, Computers, Smart Mobile Phones, Smart Mobile Apps, Smartwatches, different ICT services such as availability of the internet, web services and mobile applications used to access the status of ICT in the study area. The intensity of ICT use was measured in terms of the duration of use of ICT (minutes) for socio-economic and travel purposes. The indicators to measure the influence of ICT on travel patterns include the number of trips, the average distance travelled, the reduction in trips and travel distance, and time saved in travel.
A total of 500 pre-tested questionnaires were administered by using a stratified random sampling process of which 459 were returned (with a 92% response rate). The sample size selected was based on a confidence level of 95% at a confidence interval of 5%. The sample size of 459 under these conditions is fairly more than the required sample size of 384. The samples were spread over nine income range categories (varying between <5000 USD (<400000 Indian Rupees (INR)) to >40000 USD (>3200000 INR) with increments of 5000 USD)) in appropriate proportions ranging between 16.12% for the lowest income level and 2.40% for highest income range with an average of 11.11% and median of 11.33%. Further, the sample is reasonably well distributed over the six age groups. The maximum number of the respondents belong to the age group between 36 and 45 followed by 26 and 45. However, the lowest number of respondents belong to the group exceeding 65 years. Furthermore, males represent 64.92% and females represent 35.08% of the total sample size (). The sample size and its distribution over income, age and gender indicate the suitability of the sample.
Table 2. Profile of respondents surveyed.
4.1.2. The delphi survey
In addition to the households survey, a survey among 19 experts related to ICT and transportation was conducted to identify strategic interventions to improve ICT use and travel characteristics of people in the city. The survey was conducted using the Delphi technique. The experts constituted six professionals from the road transportation field, three ICT professionals, four urban planners, three academicians, and two policy-decision makers. To select the respondents, a list of experts belonging to road transportation (engineers and planners), urban and regional planning, ICT, and policy and decision-making was compiled based on the information gathered from different relevant organisations in which they were engaged and the profiles available online. Criteria such as educational qualification, professional engagement in relevant fields, experience, association with ICT and urban road transportation and current level of engagement were used to select the experts. The experts having a minimum qualification of a Bachelor’s degree, current professional engagement in their respective domains with at least 5 years of experience, and an understanding of urban road transportation challenges and ICT were selected. They were then contacted via personal contacts, telephones and/or emails to invite them to participate in the survey. The experts selected for the survey were finalised based on their willingness and acceptance.
The survey was conducted in two rounds. In the first round, the experts were asked to identify relevant general strategies and ICT-linked strategies that would engender planned behaviour and influence travel pattern. Based on the responses, a set of ICT-linked strategies was compiled. Then the experts were asked to rank the relative influence of ICT-related strategic interventions that would assist in planned behaviour and change in travel pattern. The survey was conducted by using a five-point Likert scale with pointers 1-indicating no influence, 2-less influential, 3-fairly influential, 4-highly influential, and 5-most influential. Nonmaleficence was adhered to while collecting data and care was taken not to harm or endanger any living or nonliving elements.
4.2. Data analyses and model estimation
The data were analysed by both descriptive and inferential statistics and ordinal regression model estimation. Data were cleaned by using the five-stage data cleaning process. Cronbach’s alpha test (Sijtsma Citation2009) of the data collected was conducted to observe the reliability of the data. Furthermore, Kurtosis and Skewness tests were conducted to examine the normality of the data (Cain et al. Citation2017).
4.2.1. Model estimation to examine the impact of ICT
Based on the results of the normality test, a Generalised Linear Model (GLM) estimation for normal distribution and identity link was conducted to observe the interlinkage and relationship among the variables such as ICT use and travel pattern attributes. This modelling approach models how the mean of a continuous response variable y depends on a set of explanatory variables, where i indexes each observation. Income has been considered as the random effect. In this context, the equation with random effect can be formulated as given in EquationEquation 1(1)
(1)
Where yi represents the response variables
µi is the average of the values of the independent variable ICT used for each response variable,
x is the explanatory variable ICT use
€i is the random effect of Income
The various components of the model are:
The random component: The distribution of y has a normal distribution with mean µ and constant variance σ2.
Systematic component: x is the explanatory variable and is linear in the parameters.
Link function: the identity link, .
The models were fitted by using maximum likelihood estimation and validated by use of the Omnibus test, Akaike’s Information Criterion (AIC)Finite Sample Corrected AIC (AICC) and model effect values (significance test values).
The travel pattern attributes such as reduction in the number of trips, reduction in average distance travelled per trip, reduction in average distance travelled per household and reduction in travel time are the response variables and ICT usage is the predictor variable. The analyses were conducted on an aggregate basis by considering all income groups.
4.2.2. Model estimation to evaluate the influence of various strategies
An Ordinal regression model estimation was conducted to explore the relative influence of various strategic measures that would likely enhance ICT use and the consequent change in travel characteristics. The data collected from the experts on strategic options were used for this model estimation.
The ordinal regression model generally predicts the behaviour of the ordinal dependent variable (whose values exist on an arbitrary scale) with a set of independent variables and uses causal analyses, forecasting an effect and trend forecasting. The focus is on the strength of the relationships between two or more variables. It assumes a dependence or causal relationship between one or more independent variables and one dependent variable (Williams and Quiroz Citation2020). The dependent variable is the order response category variable (or response variable) and the independent variables (predictor variables) may be categorical or continuous variables. This model is found suitable when the data collected is on an ordinal scale such as the Likert scale. In this context, the model estimation was cone to examine the strength of the effect of the various strategies for the enhancement of ICT use and the consequent impact on the travel characteristics. In this case, the various strategies were taken as the predictor variables and the influence of these strategies was considered the dependent variable.
While conducting model estimation, the log-linked ordinal regression model was used. The model is represented by EquationEquation 2.(2)
(2)
where Pr is the cumulative probability of the response
being at most
is given by a function
the inverse link function) applied to a linear function of
.
The set of observations is represented by p-length vectors
The logistic function is given by EquationEquation 3
(3)
(3)
where are the set of thresholds with
in which k disjoint segments correspond to k response levels.
The validity and robustness of the model were checked by Goodness of Fit, Nagelkerke r square (Pseudo r square) and test of parallel lines. IBM SPPS V.27 software was used for data analysis.
5. Results: empirical relationships between ICT usage and travel pattern attributes, and Sensitivity analyses
5.1. Level of ICT use and current general travel pattern in the city region
Initially, the data were categorised under 9 income categories at equal intervals ranging from 0–5000 USD to > 40000 USD) per year at a regular interval of 5000 USD before analysing at the aggregate level. Before the data were analysed and used for interpretation, it was initially checked for reliability and normality. The high Cronbach α values between income and ICT usage, income and the number of trips, income and distance travelled, ICT use and the number of trips, ICT use and distance travelled (0.901, 0.904 and 0.848, 0.832, and 0.794 respectively) indicate that the data set was valid and reliable. Similarly, the Kurtosis values were found to range between −7 and + 7 and Skewness values ranged between −2 and + 2 suggesting the normality of the data ().
Table 3. Normality test (Skewness and Kurtosis) results.
show the level of ICT use and general travel pattern of the people of Pune according to their income levels currently. It is revealed that effective ICT usage in households increases with the increase in income. It ranges from 0.75 (45 minutes) hours to 5.4 hours (5 hours 24 minutes) from lowest income to highest income level. This implies that people with higher incomes make higher use of ICT. Similarly, it is indicated that the number of trips per household increases with income. Moreover, on average, the number of trips per household in the city is 7.25 trips. The variation in trip generation among the households is marginal (ranging between 5.45 and 7.92 trips). Although the average distance travelled is 17.95 km per trip per day, it fluctuates considerably between 8.15 and 26.55 km per trip across different income ranges. It indicates that while income influences trip generation, it may not influence the distance travelled per trip. Also, with the use of ICT, the average reduction in trips and distance travelled by households per day is envisaged to be 13.33% and 22.59% respectively. Similarly, with the use of ICT reduction in travel time is expected to be 1.60 hours per household per day on average. In other words, ICT use is likely to reduce the number of trips, average distance travelled and travel time.
Table 4. Income level, ICT usage and travel pattern in Pune.
Table 5. Income level, ICT usage and travel pattern in Pune.
5.2. Relationship between ICT use and travel pattern
presents the model fitting and validation parameters for each GLM (model) estimation. The results show that the model fitting parameters such as AIC and AICC values are low indicating reasonably well-fitting of the model. The Omnibus test and model effect (significance) test results for all parameters are <0.05 indicating the statistical significance and validity of the model. Thus, the models are used for further analyses such as to establish the relationship between ICT usage and various travel pattern of the urban agglomeration and test the hypotheses as well as to develop the models to predict the travel pattern attributes based on ICT use. presents the parameter estimates, intercepts and significance values between the ICT use and different travel pattern attributes.
Table 6. Model fitting and validation parameters of GLM estimation.
It is found that statistically significant relationships exist between ICT usage and the number of trips, ICT usage and distance travelled per trip, ICT usage and average distance travelled by households, ICT and reduction in trips, ICT usage and reduction of distance per trip, ICT usage and reduction in average distance travelled by households, and ICT use and reduction in travel time (for α ≤ 0.05). This implies that with the increase in ICT use or if effective ICT use is done it would lead to a reduction of the number of trips and consequent reduction in travel distance and reduction in travel time by households. The test results also established the hypothesis that the use of ICT in everyday socio-economic activities of households will reduce vehicular travel in terms of reduction in trip generation (Hs1), reduction in the average travel distance by households (Hs2), reduction in average distance travel per trip (Hs3) and reduction in travel time by households (Hs4), () which in turn will contribute to sustainable road transportation.
Table 7. Relationship between income level, ICT usage and travel pattern.
5.3. Empirical relationship between ICT usage and different travel pattern attributes, sensitivity analyses and simulated scenarios
Empirical relationships between ICT usage and the four important travel pattern variables such as reduction in the number of trips per day (y1), reduction in travel distance per trip (km) (y2), reduction in travel distance by households per day in km (y3) and reduction in travel time by households per day in hours (y4) were established by using Generalised linear (identity linked) modelling approach. In this case, the travel pattern variables (y1, y2, y3, and y4) were taken as the response variables and ICT usage (x) was used as the predictor variable. Further, reductions in various travel pattern attributes were considered for developing the model and scenario analyses because it was needed to examine how and to what extent changes in ICT usage would engender variations in these travel pattern attributes instead of their absolute values such as total trips, total distance travelled and total travel time, which might be influenced by many other exogenous socioeconomic, vehicular and environmental variables in addition to ICT usage. The acceptable model fitting parameters (, and p-values ≤0.05 indicate the validity of the relations. The empirical relationships are presented by EquationEquation 4(4)
(4) -7.
5.3.1. ICT usage and reduction in trips by households
The relationship between ICT usage and the mean reduction in trips by households is presented in EquationEquation 4(4)
(4)
Where y1= Reduction in trips per household; x = mean ICT usage in hours
5.3.2. ICT usage and reduction in average travel distance per trip by households
EquationEquation 5(5)
(5) presents the relationship between ICT usage and the mean reduction in average travel distance per trip by households.
Where y2= Reduction average travel distance per trip by households.; x = mean ICT usage in hours
5.3.3. ICT usage and reduction in travel distance by households per day
The relationship between ICT usage and the reduction in average travel distance by households per day is given by EquationEquation 6(6)
(6)
Where y3= Reduction in average travel distance by households per day; x = mean ICT usage in hours
5.3.4. ICT usage and reduction in travel time by households per day
EquationEquation 7(7)
(7) provides the relationship between ICT usage and the reduction in travel time per household per day.
Where y4= Reduction in travel time per household per day; x = mean ICT usage in hours
5.3.5. Sensitivity analyses and simulated scenario results
Sensitivity analyses were conducted by using the above four EquationEquation 3(3)
(3) -EquationEquation 7
(7)
(7) to examine how and to what extent ICT usage would engender a reduction in different travel pattern attributes. For this purpose, 20 scenarios in addition to the current scenario (Business as Usual (BS)) were created by varying the ICT usage (varying ICT usage from its current state starting from −50% up to 150% at an interval of 10%). These scenarios were chosen considering a realistic variation in the ICT use by households (obtained from the survey) in the foreseeable future.
presents the variations in the four travel pattern attributes based on the change in ICT usage. presents the range of such variations. It was found that with a reduction in ICT usage between 10% and 50% from the BS, the average number of trips per household will reduce trips to 0.64 and 0.27 trips respectively from the base case scenario of a reduction of 1.01 trips. Similarly, if the ICT usage is increased between 10% and 150% from the current state, a higher reduction in trips between 1.10 and 2.13 trips per household will be experienced (). Overall, with a variation of −50% and + 150% of ICT usage from the BS, the reduction of trips would range between −63.8% and 191.2% of the trips respectively (). Similarly, under the same scenarios of the reduction of ICT use between 50% and 10% from the BS, the travel distance per trip will increase between 2.25 km and 3.30 km. On the other hand, with an increase in ICT usage from 10% to 150% compared to the BS, the travel distance per trip will reduce by 3.81 km to 7.45 km respectively (). However, the overall reduction in travel distance per trip will range between −29.30% and 109.13% over the BS scenario with a variation of ICT use between −50% and 150% respectively (). Furthermore, it was also found that the average travel distance per household will reduce by 23.65 km and 14.68 km respectively under the reduction of ICT use between 10% and 50% compared to 30.78 km under BS. However, if ICT use is increased by 10% to 150%, a reduction in travel distance by households ranging between 32.44 km and 59.10 km will be experienced (). In other words, the overall reduction might range between −43.09% and 129.17% over the BS (). Similarly, the analyses of travel time show that compared to the travel time reduction of 1.42 hours under the BS, a reduction of ICT usage by 10% and 50% will engender a travel time reduction of 1.26 hours and 0.8 hours respectively. However, when ICT use is increased by 10% to 150% from the BS, the reduction of travel time by households will range between 1.49 hours and 3.05 hours showing a significant reduction in travel time (). This reduction will range between −40.67% and +121.30% over the BS scenarios with a variation of ICT usage from −50% to 150% (). Thus, it demonstrates that ICT usage has a significant influence on various travel pattern attributes. In other words, significant ICT usage will lead to a significant reduction in the number of trips, travel distance per trip, travel distance by household and travel time per household.
5.4. Strategic interventions for enhanced ICT use and influence on travel pattern
Four strategic interventions were evaluated by ordinal regression model estimation to examine how ICT usage can be enhanced in socio-economic activities and transportation, which would influence the travel pattern of people in the urban agglomeration. The strategies were identified through discussions with the experts. While making model estimation, the four identified strategies were compared with the current scenario with the availability of limited information by ICT usage and conventional means. However, before the model estimation, the validity and robustness of the model were checked by use of Model fitting information, Goodness of fit, Nagelkerke (Pseudo R square), and Test of parallel lines. presents the model validation parameters and the results indicate that the model is valid and can be used for model estimation.
Table 8. Model validation parameters of ordinal regression model estimation.
The model estimation results are presented in . According to the model estimation results three strategies (1) enhancing networking and ICT connectivity in the urban agglomeration, (2) provision of free Wi-Fi hot-spots at important nodal points and activity centres, and (3) enabling online availability of real-time road transportation and travel-related information, are statistically significant, thus could able to enhance ICT use in socio-economic activities and influence travel patterns. Furthermore, the provision of free Wi-Fi hot spots at important nodal points and activity centres would be the most influential strategy that would positively influence travel patterns. Similarly, the strategies of enhancing networking and ICT connectivity in the urban agglomeration and enabling online availability of real-time road transportation and travel-related information would influence the travel pattern significantly. However, the strategy of enabling real-time road transportation and travel-related information by ITS at nodal points and on roadsides is statistically insignificant, thus could not be conclusively ascertained that it will impact travel patterns positively.
Table 9. Strategic interventions for enhanced ICT usage and influence on travel pattern.
6. Discussion and conclusions
The challenges of sustainable road transportation, specifically in cities in developing countries such as India warranted investigations to find plausible policy interventions to attain sustainability. Changes in travel patterns of people are envisaged to be essential elements that would assist in meeting the challenge. In this regard, ICT usage has been argued to be pivotal to engendering change (Lenz and Nobis Citation2007; Tseng et al. Citation2013). ICT has been increasingly influencing every aspect of city life in recent years in India. It has a significant presence in the cities which can leverage the socio-economic functions of people. It is also being increasingly used in travel activities of people. For example, certain travel information such as route choice and traffic conditions is available through the use of ICT are fully available and some advanced travel information such as real-time travel information, parking lots availability, ticketing, bookings, etc., are partially available in different contexts. Since the augmentation of road infrastructure is not able to solve the road transportation challenges in Indian cities, an argument has been proffered that effective use of ICT in day-to-day socio-economic and travel activities would able to reduce road transportation challenges in cities of India and enable sustainable transportation. However, before how ICT could contribute to sustainable transportation, it was essential to examine how ICT could influence the travel pattern of people which could reduce road transportation-related challenges, specifically in congested cities. Therefore, this study explored the influence of ICT on the travel pattern of people in a city in India by using the Pune city region as a case study. Also, strategic options that would influence travel characteristics positively were explored.
The empirical models (EquationEquation 4(4)
(4) -EquationEquation 7
(7)
(7) suggest that definite linkages between ICT usage and travel pattern attributes such as reduction in trip generation, reduction in distance travel per trip, reduction in average distance travel by households, and reduction in travel time of households exist. Also, the hypothesis that ICT usage in everyday socio-economic and travel activities of households will reduce vehicular travel in terms of reduction in trip generation (Hs1), reduction in travel distance per trip (Hs2), reduction in travel distance by households (Hs3)and reduction in travel time (Hs4)were established. The findings from the sensitive analyses show that a variation of ICT usage between −50% and +150% from the BS, will reduce the trips between −63.8% and 191.2%. Similarly, the reduction in travel distance per trip per household will range between −29.30% and 109.13%. Moreover, the reduction in average travel distance per household per day will likely be between −43.09% and 129.17%. Similarly, travel time per household will be reduced by −40.67% and 121.30% over the BS scenarios. This implies that higher use of ICT to perform socio-economic and travel activities can reduce the number of trips, save on travel time and reduce travel distance significantly. Moreover, on the other hand, a decrease in ICT usage might have an adverse impact on the travel pattern. However, ICT use will have more positive impacts than envisaged by policymakers initially (De Graaff and Rietveld Citation2007). These findings confirmed some of the previous findings that an increase in ICT usage will lead to a change in travel patterns, particularly in terms of travel substitution (positive association between ICT usage (Cohen-Blankshtain and Rotem-Mindali Citation2016; Gössling 2018) and reduction in travel pattern attributes such as trip generation, distance travel and travel time. In other words, it can bring changes in attitude, subjective norms and perceived behavioural control involuntarily as postulated by TPB (Ajzen Citation1991; Ambali and Bakar Citation2015; Kan and Fabrigar Citation2017; Hu et al. Citation2020), which are likely to bring changes in travel behaviour and consequently change travel pattern. However, the findings of the study differ from the propositions of a negative association between ICT and travel patterns made by several scholars (van Wee et al. Citation2013; Kroesen and Handy Citation2015; Hong and Thakuriah Citation2016), which could be because of contextual issues that are largely different from the context of ICT-enabled but congested cities of India.
Furthermore, there is also a need to understand how the attitude and perceived behaviour towards travel patterns may be involuntarily changed. In this context, interventions premised upon TPB would likely play a critical role. Since ICT is an enabler, it was also needed to examine how ICT use can be substantially enhanced so that it can become significantly influential to bring in changes in the attitude and behaviour of people, specifically concerning travel patterns. In other words, it is argued that appropriate ICT-related strategic interventions will engender planned behaviour among people concerning their daily socio-economic and travel activities (Ambali and Bakar Citation2015; Hu et al. Citation2020). In this context, the evaluation of different strategies suggested three strategic interventions such as enhancing networking and ICT connectivity in the urban agglomeration, providing free Wi-Fi hot-spots at important nodal points and activity centres, and enabling online availability of real-time road transportation and travel-related information would likely influence the socio-economic and travel activities of people. This would likely enforce people to plan their activities, leading to a change in their travel behaviour and travel pattern as proposed by the TPB. In other words, in alignment with the arguments of different scholars (Lenz and Nobis Citation2007; Ambali and Bakar Citation2015; Das Citation2016; Hu et al. Citation2020), strategies to enhance ICT usage will engender planned behaviour among people towards socio-economic and travel activities that would impact the travel pattern in the city region significantly.
However, the study has certain limitations. It is based on primary data and a limited sample survey of an ICT-enabled city region. The study was also conducted on an aggregate basis because of the nature of the data collected. Besides, there are other underlying confounding factors such as lifestyle, activity patterns, choice of people, location, affordability, quality of ICT infrastructure, etc., which could imply unobserved heterogeneity challenges through random effects that may also influence the use of ICT and travel patterns of people. Also, TPB has not been used to predict the travel pattern of people based on the use of ICT. Rather, appropriate ICT-linked strategic interventions for enhanced ICT usage in socio-economic and travel activities of people, which might provoke planned travel behaviour bringing positive change in travel patterns were explored. Therefore further research is required to generalise the findings. The further scope of this study includes the extension of the study contexts and the inclusion of unobserved heterogeneity underpinned by social, spatial, and other confounding factors. Furthermore, how TBP can be used to predict travel behaviour and pattern based on ICT usage needs to be explored. Moreover, there is also a need for empirically establishing ICT-enabled travel patterns and the sustainability of road transportation, which could be another scope of future research.
The findings of the study have both practical and theoretical implications. Practically, the study showed that effective ICT usage can assist in a significant reduction in trip generation and travel distance, and save travel time, which consequently can contribute to sustainable road transportation in Indian cities. In other words, enhancing networking and ICT connectivity in the city region, provisioning of free Wi-Fi hot spots at important nodal points and activity centres, the enabling online availability of real-time road transportation and travel-related information, which can be done by reinforcing the already existing ICT infrastructure and services, will make people use ICT in socio-economic and travel activities. Consequently, there will reduction in unwarranted travel requirements unless it is reasonably essential. Besides, the use of ICT in travel needs will assist in optimal planning and management of trips and consequent reduction of travel distance and delay. Therefore, policymakers, practitioners and city authorities or governance structures should make efforts through strategic interventions to enhance ICT infrastructure and services and encourage the people to use these for their socio-economic and travel activities. This would likely bring a positive change in travel patterns, which could contribute to sustainable road transportation in the cities. However, these findings could be pertinent to cities in India and similar developing countries. Theoretically, it explicitly corroborated and reinforced the previous propositions that ICT usage is positively associated with travel patterns, specifically in the cities of developing countries.
The novelty and contribution of the study include the explicit establishment of a positive association between ICT use and travel pattern attributes of people specifically in the ICT-enabled congested cities of India premised upon TPB. It also offers empirical relationships to predict the travel pattern attributes (reduction in trips, travel distance and travel time) based on the ICT use. Furthermore, the study proposed strategic interventions that could assist enhanced ICT use specifically linked to travel of people, which were not observed exclusively so far in the extant literature.
Disclosure statement
No potential conflict of interest was reported by the authors.
Additional information
Notes on contributors
Dillip Kumar Das
Professor Dillip Kumar Das, Ph.D. in Urban and Regional Planning, blends expertise in Civil Engineering and City Planning. Currently dedicated to academia at the University of KwaZulu Natal, South Africa, his journey spans universities in India, Ethiopia and South Africa. Preceding his academic role from 2001, he has significant experience consultancies and government sectors in India. As a member of the Sustainable Road Transportation Group at UKZN, a registered Professional Engineer, and A-rated researcher recognised by SANRAL, his research spans across sustainable urban development, transportation planning, Applied Systems Analysis, system dynamics modeling, Multi-Criteria Decision-Making Modeling, etc. Professor Das has significantly contributed to several research and industry-based projects, co-authored two books as the lead author and published numerous peer-reviewed research articles. His commitment also extends to editorial roles in multiple esteemed journals, enhancing the scholarly landscape and fostering academic community growth.
Sanjaykumar G Sonar
Dr. Sanjaykumar G. Sonar, an alumnus of the prestigious College of Engineering, Pune (COEP), holds a Bachelor's degree in Civil Engineering (1999) and a Master's in Town and Country Planning (2003). His academic journey continued at the Indian Institute of Technology (IIT), Roorkee, where he earned his Ph.D. in Urban Planning in 2007. Commencing his career at the Delhi Development Authority, Dr. Sonar later contributed significantly to the Mumbai Metropolitan Region Development Authority. Returning to COEP Technological University, he now imparts his expertise as a faculty member in the Department of Planning. Dr. Sonar, a prolific author, has published research papers and co-authored a notable book on Regional Planning. Engaged in various professional bodies, he remains an active participant in their endeavors.
Mohamed Mostafa Hassan Mostafa
Professor Mohamed Mostafa Hassan Mostafa, a distinguished Civil Engineer, earned his PhD in Pavement Engineering from the University of Liverpool, UK (2009) and an MSc in Transportation Engineering (2003). Currently, he serves as the Academic Leader of the ACL Cluster at the University of KwaZulu-Natal, overseeing Agriculture Engineering, Civil Engineering, and Land Surveying. Additionally, he holds the esteemed position of Chair and Director for the KZNDoT Chair in Sustainable Transportation. With a rich academic history spanning Egypt, the UK, and South Africa, Professor Mostafa specializes in sustainable roads, focusing on pavement research encompassing asphalt, smart transportation, and geotechnical pavement applications. Recognized as a researcher category A by SANRAL, he contributes extensively to professional bodies and has published over 80 articles. Notably, he founded and chairs the Sustainable Transportation Research Group (STRg) at UKZN.
References
- Adler J, Blue V. 1998. Toward the design of intelligent traveller information system. Transp ResPart C. 6(3):157–172. doi: 10.1016/S0968-090X(98)00012-6.
- Aguilera A. 2008. Business travel and mobile workers. Transp Res Part a. Pol And Prac. 42(8):1109–1116. doi: 10.1016/j.tra.2008.03.005.
- Aguiléra A, Guillot C, Rallet A. 2012. Mobile ICTs and physical mobility: review and research agenda. Transp Res Part a. Pol And Prac. 46(4):664–672. doi: 10.1016/j.tra.2012.01.005.
- Ajzen I. 1991. The Theory of planned behavior. Organiz Behav And Hum Decis Proc. 50(2):179–211. doi: 10.1016/0749-5978(91)90020-T.
- Ambali A, Bakar A. 2015. The Theory of planned behaviour and its relation to ICT adoption. IGI Global. doi: 10.4018/978-1-4666-6579-8.ch007.
- Bhat CR, Sardesai R. 2006. The impact of stop-making and travel time reliability on commute mode choice. Transp Res Part B: Methodo. 40(9):709–730. doi: 10.1016/j.trb.2005.09.008.
- Cain MK, Zhang Z, Yuan KH. 2017. Univariate and multivariate skewness and kurtosis for measuring nonnormality: prevalence, influence and estimation. Behav Res. 49(5):1716–1735. doi: 10.3758/s13428-016-0814-1.
- Carrasco J, Miller EJ. 2006. Exploring the propensity to perform social activities: a social networks approach. Trans. 33(5):463–480. doi: 10.1007/s11116-006-8074-z.
- Chen C, Ting C. 2007. Combining Lagrangian heuristic and ant colony system to solve the single source capacitate facility location problem. Transp Res Part E. 44(6):1099–1122. doi: 10.1016/j.tre.2007.09.001.
- Chen T, Chang H, Tzeng G. 2001. Using a weight assessing model to identify route choice criteria and information effects. Transp Res Part A. 35(3):197–224. doi: 10.1016/S0965-8564(99)00055-5.
- Choo S, Lee T, Mokhtarian PL. 2007. Do transportation and communications tend to be substitutes, complements, or neither? Transp Res Rec: J Of The Transp Boa. 2010(1):121–132. doi: 10.3141/2010-14.
- Chorus CG, Molin EJE, van Wee GP. 2006. Travel information as an instrument to change car-drivers travel choices: a literature review. Euro J Of Transp And Infra Res. 6(4):335–364.
- Chorus CG, Arentze TA, Timmermans HJP. 2007. Information impact on quality of multimodal travel choices: conceptualizations and empirical analyses. Transp. 34(6):625–645. doi: 10.1007/s11116-007-9120-1.
- Cohen-Blankshtain G, Rotem-Mindali O. 2016. Key research themes on ICT and sustainable urban mobility. Int J Of Sust Transp. 10(1):9–17. doi: 10.1080/15568318.2013.820994.
- Commission of the European Communities. 2001. European transport policy for 2020: time to decide. Luxembourg: Commission of the European Communities.
- Das D. 2014. Evaluation of control parameters for smart mobility in the context of a South African city-A case of Bloemfontein city. Proc Of Plan Africa Conf. 138–151.
- Das D, Emuze F. 2014. Smart city perspectives of Bloemfontein, South Africa. Jof Const Proj Manag And Innov. 4(2):930–950.
- Das D. 2016. Engendering image of creative cities by use of information and communication Technology in developing countries. Urb Plann. 1(3):1–12. doi: 10.17645/up.v1i3.686.
- De Graaff T, Rietveld P. 2007. Substitution between working at home and out-of-home: the role of ICT and commuting cost. Transp Rese Part A. 41(2):142–160. doi: 10.1016/j.tra.2006.02.005.
- Delbosc A, Currie G. 2014. Changing demographics and young adult driver license decline in Melbourne, Australia (1994–2009). Transp. 41(3):529–542. doi: 10.1007/s11116-013-9496-z.
- Delbosc A, Mokhtarian P. 2018. Face to Facebook: the relationship between social media and social travel. Transp Pol. 68:20–27. doi: 10.1016/j.tranpol.2018.04.005.
- Department for Transport. 2004. The future of transport: a network for 2030. London: Department for Transport.
- Emuze F, Das D. 2015. Regenerative ideas for urban roads in South Africa. Proc Of The Instof Civ Engin: Mun Engin168. 168(4):209–219. doi: 10.1680/muen.14.00041.
- Farag S, Lyons G. 2010. Explaining public transport information use when a car is available: attitude theory empirically investigated. Transp. 37(6):897–913. doi: 10.1007/s11116-010-9265-1.
- Farag S, Schwanen T, Dijst M, Faber J. 2007. Shopping online and/or in-store? a structural equation model of the relationships between e-shopping and in-store shopping. Transp Rese Part A Pol And Prac. 41(2):125–141. doi: 10.1016/j.tra.2006.02.003.
- Federal Transit Administration. 2003. Customer preferences for transit ATIS. Research report nr. FTA-OH-26-7015-2003.1. Washington, DC: Federal Transit Administration.
- Gadgets 360. n.d. India’s fastest broadband internet service providers. [Accessed on 15 September 2017’ http://gadgets.ndtv.com/internet/features/indias-fastest-broadband-internet-service-providers-810697.
- Germann Molz J. 2012. Travel connections: tourism, technology and togetherness in a mobile world. Florence, KY, USA: Routledge.
- Giffinger R, Fertne C, Kramar H, Kalasek R, Pichler-Milanović N, Meijers E. 2007. Smart cities –ranking of European Medium-Sized cities. Centre of regional science. Vienna University of Technology, Vienna, Austria, Final Project Report, pp. 1–25.
- Global Sustainable Systems Research Report. 2004. Pune vehicle activity study, University of California at Riverside, pp. 1–3.
- Goldman T, Gorham R. 2006. Sustainable urban transport: four innovative directions. Tech In Soc. 28(1–2):261–273. doi: 10.1016/j.techsoc.2005.10.007.
- G¨ossling S. 2018. ICT and transport behaviour: a conceptual review. Int J Of Sust Transp. 12(3):153–164. doi: 10.1080/15568318.2017.1338318.
- Hameri A, Paatela A. 2005. Supply network dynamics as a source of new business. Int J Of Prod Eco. 98(1):41–55. doi: 10.1016/j.ijpe.2004.09.006.
- Hindustan Times. 2016. Delhi’s odd-even plan: all you need to know about the formula. https://www.hindustantimes.com/delhi/delhi-s-odd-even-plan-genius-or-a-recipe-for-nightmare/story-0rFNFUTEHncNHpWZh2jtTI.html
- Hong J, Thakuriah P. 2016. Relationship between motorized travel and time spent online for nonwork purposes: an examination of location impact. Int J Of Sust Transp. 10(7):617–626. http://www.yourarticlelibrary.com/essay/family-types-on-the-basis-of-size-of-household-in-india/41330/.
- Hu X, Zhu X, Chiu Y, Tang Q. 2020. Will information and incentive affect traveller’s day-to-day departure time decisions?—an empirical study of the decision-making evolution process. Int J Of Sust Transp. 14(6):403–412. doi: 10.1080/15568318.2019.1570402.
- Kan MPH, Fabrigar LR. 2017. Theory of planned behavior. In: Zeigler-Hill V Shackelford T, editors. Encyclopedia of personality and individual differences. Cham: Springer. doi:10.1007/978-3-319-28099-8_1191-1.
- Kanninen B. 1996. Intelligent transportation systems: an economic and environmental policy assessment. Transp Res Part A. Pol And Prac. 30(1):1–10. doi: 10.1016/0965-8564(95)00014-3.
- Kroesen M, Handy SL. 2015. Is the rise of the e-society responsible for the decline in car use by young adults? Results from the Netherlands. Transp Res Rec: J Of The Transp Boa. 2496(1):28–35. doi: 10.3141/2496-04.
- Lachapelle U, Jean-Germain F. 2019. Personal use of the internet and travel: evidence from the Canadian general social survey’s 2010 time use module. Trav Behav And Soc. 14:81–91. doi: 10.1016/j.tbs.2018.10.002.
- Larsen J, Urry J, Axhausen K. 2007. Networks and tourism. Mobile and social life. Ann Of Tour Res. 34(1):244–262. doi: 10.1016/j.annals.2006.08.002.
- Lee I, Cho SH, Kim K, Kho SY, Kim DK, Meloni S. 2022. Travel pattern-based bus trip origin-destination estimation using smart card data. PloS One. 17(6):e0270346. doi: 10.1371/journal.pone.0270346.
- Lenz B, Nobis C. 2007. The changing allocation of activities in space and time by the use of ICT– ‘fragmentation’ as a new concept and empirical results. Transp Res Part A Pol And Prac. 41(2):190–204. doi: 10.1016/j.tra.2006.03.004.
- Lombardi P. 2011. Managing the green IT agenda. Intel Build Int. 3(1):41–45. doi: 10.3763/inbi.2010.0008.
- Martínez-Torres MR, Díaz-Fernández MC, Tora SL, Barrero FJ. 2013. Identification of new added value services on intelligent transportation systems. Behav & Inform Techn. 32(3):307–320. doi: 10.1080/0144929X.2010.529942.
- Mwale M, Luke R, Pisa N. 2022. Factors that affect travel behaviour in developing cities: a methodological review. Transp Res Interdis Persp. 16:100683. doi: 10.1016/j.trip.2022.100683.
- Mokhtarian P. 2009. If telecommunication is such a good substitute for travel, why does congestion continue to get worse? Transp Let. 1(1):1–17. doi: 10.3328/TL.2009.01.01.1-17.
- Mokhtarian PL, Salomon I. 2002. Emerging travel patterns: do telecommunications make a difference?, in “perpetual motion: travel behavior Research opportunities and application challenges”. In: H. S. Mahamassani, editor. Amsterdam: Pergamon, Elsevier; p. 592.
- Ng L, Barfield W, Mannering F. 1995. A survey-based methodology to determine information requirements for advanced traveller information systems. Transp Res Part C. 3(2):113–127. doi: 10.1016/0968-090X(95)00001-Y.
- Pai M, Gadgil R, Mahendra A, Vernekar S, Rebecca H, Chanchani R 2014. Motorized two Wheelers in Indian cities, a case study of the city of Pune. Working Paper. EMBARQ, India Mumbai, Available online at: http://www.embarqindia.org.
- Pawlak J, Circella G, Mahmassani HS, Mokhtarian PL. 2020. Information and communication Technologies (ICT), activity decisions, and travel choices: 20 years into the Second Millennium and where do we go next? Standing Committee on effects of information and communication Technologies (ICT) on travel choices (ADB20). Washington, D.C: Transportation Research Board.
- PMC. 2006. Pune city Development plan 2006-2012 under JnNURM, Pune: Pune Municipal Corporation.
- Rangarajan A 2010. BRTS- Bus Rapid Transit system in Pune: modelling, simulation and feasibility analysis. In Proceedings of the 2010 International Conference on Industrial Engineering and Operations Management, 9 – 10 January 2010. Dhaka, Bangladesh.
- Salomon I. 2000. Can telecommunications help solve transportation problems?, in Handbook of transport modelling. Hensher, D A, Button, K, editors. Amsterdam: Pergamon, Elsevier; p. 790.
- Shaw KL. 2016. Patient education, motivation, compliance, and adherence to physical activity, exercise, and rehabilitation. In: Magee JD, Zachazewski JE, Quillen WS, Manske RC, editors. Pathology and intervention in musculoskeletal rehabilitation, Philadelphia: W.B. Saunders; pp. 1–24.
- Shoabjareh AH, Mamdoohi AR, Nordfjærn T. 2021. Analysis of pedestrians’ behaviour: a segmentation approach based on latent variables. Accident Anal Prev. 157:106160. doi: 10.1016/j.aap.2021.106160.
- Sijtsma K. 2009. On the use, the misuse, and the very limited usefulness of Cronbach’s alpha. Psychomet. 74(1):107–120. doi: 10.1007/s11336-008-9101-0.
- Silva JDA, de One J, Gasparovic S. 2017. The relation between travel behaviour, ICT usage and social networks, the design of a web-based survey. Transp Res Proc. 24:515–522. doi: 10.1016/j.trpro.2017.05.482.
- Singh R, Graham D, Anderson R. 2020. Quantifying the effects of passenger-level heterogeneity on transit journey times. Data-Centric Eng. 1:E15. doi: 10.1017/dce.2020.15.
- Thynell M, Mohan D, Tiwari G. 2010. Sustainable transport and the modernisation of urban transport in Delhi and Stockholm. Cities. 27(6):421–429. doi: 10.1016/j.cities.2010.04.002.
- Tiwari R, Cervero R, Schipper L. 2011. Driving CO2 reduction by integrating transport and urban design strategies. Cities. 28(5):394–405. doi: 10.1016/j.cities.2011.05.005.
- Tseng YY, Knockaert J, Verhoef ET. 2013. A revealed preference study of behavioural impacts of real-time traffic information. Transp Res Part C: Emer Techn. 30:196–209. doi: 10.1016/j.trc.2011.11.006.
- van den Berg P, Arentze T, Timmermans H. 2013. A path analysis of social networks, telecommunication and social activity-travel patterns. Transp Res Part C: Emer Techn. 26:256–268. doi: 10.1016/j.trc.2012.10.002.
- van Wee B, Geurs K, Chorus C. 2013. Information, communication, travel behaviour and accessibility. J Of Transp And Lan Use. 6(3):1–16. doi: 10.5198/jtlu.v6i3.282.
- Weiyu Z, Arul C. 2014. Internet studies and development discourses: the cases of China and India. Infor Techn For Dev. 20(4):324–338. doi: 10.1080/02681102.2013.796546.
- Wellman B, Hoga B, Berg K, Boase J, Carrasco JA, Côté R, Kayahara J, Kennedy YLM, Tran P. 2006. Connected lives: the project. In: Purcell P, editor. Networked neighbourhoods. London: Springer; pp. 161–216.
- Williams RA, Quiroz C. 2020. Ordinal Regression Models. In: Atkinson P, Delamont S, Cernat A, Sakshaug JW Williams RA, editors. Advances in methods and practices in psychological science. SAGE Research Methods Foundations. doi: 10.4135/9781526421036885901.
- www.quora.com. 2016. How successful was the Delhi’s odd-even plan?
- Yang H, Meng Q. 2001. Modeling user adoption of advanced traveler information systems: dynamic evolution and stationary equilibrium. Transp Res Part A Policy Pract. 35(10):895–912. doi: 10.1016/S0965-8564(00)00030-6.
- Yin C, Wang X, Shao C, Le Pira M. 2021. Do the effects of ICT use on trip generation vary across travel modes? Evidence from Beijing. J Of Adv Transp. 2021:1–11. doi: 10.1155/2021/6699674.
- Yigitcanlar T, O’Connor K, Westerman C. 2008. The making of knowledge cities: Melbourne’s knowledge-based urban development experience. Cities. 25(2):63–72. doi: 10.1016/j.cities.2008.01.001.