Abstract
The digital transformation is forcing organizations to change towards more data-driven business models. In this paper, we propose a conceptual framework that explains the role of innovation capabilities as a mediator between big data and business model. Using the design science research method approach, we built the framework based on the existing literature. We then applied the framework to the real-world context with three firms and refined it based on the feedback. This study contributes to big data research by pointing out the role of human and data-driven innovation capabilities in the big data value creation process. The developed framework is practitioner oriented, offering a systematic approach towards the development of big data capabilities.
1. Introduction
Linking the opportunities of big data and the business transformation imperative resulting from digitization leads to a situation where incumbent firms must re-think and innovate in their business models and create new capabilities in order to stay competitive in their business ecosystem. Digital technologies, such as social media, cameras or open web sources, produce vast amounts of data. The number of “things” (mobile devices, sensors, etc.) that are connected to the Internet is rising rapidly. Humans generate more and more digital breadcrumbs; we are actually becoming “walking data generators” (McAfee & Brynjolfsson, Citation2012). The business landscape is becoming turbulent, and changes can happen rapidly. The disruption of many current business models has already begun (Weill & Woerner, Citation2015).
The data generation phenomenon is called datafication. Datafication can be seen as an “information technology-driven sense-making process” (Lycett, Citation2013). As datafication proceeds, more and more data are produced, processed and transferred. These data are often called big data. Although big data is understood in various ways, the importance of utilizing data in modern businesses is undeniable. Several studies show that data-intensive firms are more effective and profitable than their less data-driven rivals (see e.g. Brynjolfsson, Hitt, & Kim, Citation2011; Dehning, Richardson, & Zmud, 2003; McAfee & Brynjolfsson, Citation2012).
Business ecosystems (Iansiti & Levien, Citation2004; Moore, Citation1993) and data-related business models are emerging, providing services like data (data-as-a-service), analytics (analysis-as-a-service) or Internet of Things-related offerings (Chen et al., Citation2011; Leminen et al., Citation2012). Many new startup firms follow the trailblazers like Google or Amazon and formulate their entire business models on top of or around the data. Data are a valuable asset for these kind of firms. Start-ups heavily rely on data in their decision-making processes and product and service innovations.
For most incumbents, however, a data-driven approach is still a new and unexplored area. Technology vendors have introduced frameworks that aim to help organizations in their big data efforts. A typical approach is to offer “how to” examples or success stories where business benefits have been achieved by utilizing a specific technology stack. In addition to practitioners, scholars are increasingly investigating different aspects of big data, such as the value creation mechanisms (Hartmann et al., Citation2016; Wixom, Yen, B., & Relich, 2013) and organizational performance (Akter, Wamba, Gunasekaran, Dubey, & Childe, Citation2016; Ren et al. Citation2017; Wamba, Gunasekaran, Akter, Ren, Dubey, & Childe, et al. Citation2017).
The purpose of this study is to present a practitioner oriented framework that explains the role of human and data-driven innovation capabilities as a mediator between big data and the business model of a firm. Our framework helps in understanding how big data and innovations are shaping business models in the digital transformation. The term mediator is used to reconcile problems and promote development between innovation capabilities, big data and business model. The framework offers ways to organize perspectives on the transformation. This helps practitioners to focus on developing the capabilities and methods that best support the transformation towards data-driven business models.
2. Research method
As big data is an emerging area for both scholars and practitioners, the results of the research should benefit both theoretical and practical viewpoints. Therefore, a natural research discipline for the study is information systems (IS) research. IS research draws from behavioral and design sciences, exploring the combination of technology, organization and people. It should both make theoretical contributions and provide assistance to practitioners in solving current problems (Benbasat & Zmud, Citation1999; Iivari, Citation2003).
Design science is one of the two established paradigms in IS research. Hevner et al. (Citation2004) give a clear goal for design science research in their definition: “Design science creates and evaluates IT artifacts intended to solve identified organizational problems.” Their description of artifacts covers tangible products, constructs, models and methods. Thus, our framework fits into their definition. We followed the design science research method (DSRM) framework (Peffers, Tuunanen, Rothenberger, & Chatterjee, 2007), which provides a nominal process for conducting and evaluating design science research in information systems. shows the DSRM framework as we applied it in our study. Starting by identifying the problem, we followed the framework in our study. This paper represents the last stage, communication. The steps or stages, of the DSRM framework can be identified also by looking at the organization of this paper.
Figure 1. Research method, applied from Peffers et al. (Citation2007).
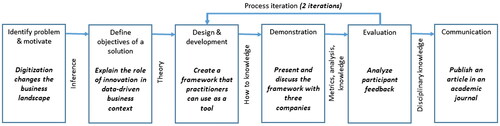
After building the framework, we used three real-world case scenarios for evaluation and to develop the artifact further. First, we tested the framework with two big data intensive firms. Secondly, we analyzed one mature stage big data implementation in order to find out how the framework might have benefited the implementation. We then refined the framework based on the feedback from both iterations. The companies utilize big data and look for opportunities to develop data driven business models. The firms are in different stages of utilizing big data and represent different industries. They focus on potential benefits in cost savings, product development and revenue generation. Thus, the three firms offer different viewpoints to the evaluation of the framework.
Other research methods, like action research (Baskerville, Citation1999; Lewin, Citation1947) or action design research (Sein et al., Citation2011) provide applicable methods for dealing with organizational change as well. However, we found that the design science DSRM framework would meet the needs of our research. We were developing an artifact (the framework) and therefore preferred design science to action research. As our goal was to develop a generic framework in a novel area, we preferred rapid iterations with moderate user input instead of their long-term commitment. In addition, practitioners favor approaches that present initial concepts or assumptions in order to provoke new ideas, instead of a clean sheet of paper as a starting point. Experimenting is a common technique for iterative development. The development, demonstration and evaluation stages of DSRM form a cycle that fits well to rapid experimenting without overloading the participating firms.
3. Building the framework
Linking the opportunities of big data (as a consequence of datafication) and the business transformation imperative (resulting from the digital transformation) leads to a situation where incumbent firms must re-think (innovate) their business models and create new capabilities in order to stay competitive in their business ecosystem. The objective of the developed framework is twofold:
Advance big data research by addressing the role of innovation capabilities in data-driven business context.
Assist practitioners in their big data initiatives by offering a framework that helps them in developing required capabilities.
3.1. Theoretical background
The framework treats innovation capabilities as a mediating factor between the business model and big data. Therefore, the nature of the framework is multi-disciplinary. The elements are related to strategic management and information systems research.
The value chain and five forces model, e.g. (Porter, Citation1991; Porter & Millar, Citation1985), are commonly used strategic management frameworks. The former is an internal view of the business, whereas the latter provides an outside-in industry view. Porter’s models explains the source of competitive advantage by two factors: low cost or differentiation. Another established strategic management framework is the resource-based view (Wernerfelt, Citation1984). It combines the internal and external views of previous models. The resource-based view aligns well with data-oriented business environments, where digital ecosystems, e.g. (Weill & Woerner, Citation2015), are increasingly used to add value.
IS research draws from behavioral and design sciences, exploring the combination of technology (including data), organization and people. This research should both make theoretical contributions and provide assistance to practitioners in solving current problems (Benbasat & Zmud, Citation1999; Iivari, Citation2003). One of the strengths of information systems research stems from the combination of behavioral and design sciences; technology, data and behavior are inseparable in an information system.
Digitalization implies business changes that are technology driven. Thus, strategic management and IS research provide a broader view to the topic. Moreover, change requires innovation. Start-ups and incumbents search for new, technology and data driven innovations. We discuss how data- and human-driven innovations act as a mediator between (big) data and business model later in this paper. In this section, we briefly present and define the building blocks from the above described disciplines that our framework relies on, namely the concepts of business model, capabilities, innovation and big data.
3.1.1. Business model
The concept of business model has been vividly discussed in the strategic management discipline. It relates to how an organization arranges its functions in order to create value. The term business model is often used interchangeably with strategy (Burkhart et al., Citation2011). Many definitions exist for both terms (e.g. Amit & Zott, Citation2001; Casadesus-Masanell & Ricart, Citation2010; Teece, Citation2010; Timmers, Citation1998). However, there seems to be consensus that these concepts differ from each other: strategy is more focused on competition and product-market matters, whereas the business model describes how the strategy is implemented (Zott, Amit, & Massa, Citation2011).
Osterwalder and Pigneur (Citation2010) define the business model as follows: “A business model describes the rationale of how an organization creates, delivers, and captures value.” They also present a business canvas framework that serves as a tool when analyzing how an enterprise creates value. Furr and Dyer (Citation2014) present a simplified business canvas framework. It includes three main elements: solution (which is further divided for value proposition and pricing strategy), cost structure (activities and resources) and customer acquisition (relationships and channels).
3.1.2. Capabilities
Capabilities are related to resources and the resource-based view (RBV) of a firm (Wernerfelt, Citation1984). In the resource-based view of a firm, resources and capabilities are the key concepts that explain a firm’s competitive advantage. Teece (Citation2007) introduced the concept of dynamic capabilities, defining them as “the firm's ability to integrate, build and reconfigure internal and external competences to address rapidly changing environments,” which complements the RBV by explaining how firms renew their competencies in order to adapt to the changing business environment.
Big data is a disruptive new technology. Incumbent firms typically rely on the idea that their current capabilities are relevant with regard to new technologies, but often this is just based on assumptions (Sainio, Citation2005). Accordingly, incumbents often fail to take advantage of disruptive technologies, even when they are well aware of them (Christensen, Citation2013). These are the main caveats for incumbents and therefore a deeper understanding of the datafication phenomenon and correct recognition of the required capabilities are crucial steps when a firm assesses the effects of big data.
3.1.3. Innovation
In order to innovate effectively and develop an innovative organization culture, the firm needs to understand the nature of innovation. Innovation and change are tightly related: to innovate effectively aims at changing something. Many different perspectives towards the concept of innovation have been taken in several disciplines. Thus, there are different definitions of the term (e.g. those suggested by Ettlie & Reza, Citation1992; Schumpeter, Citation1942; West & Anderson, Citation1996). After a review of existing definitions, Baregheh, Rowley, & Sambrook, (Citation2009) provided a synthesized definition that views innovation as a dynamic capability: “Innovation is the multi-stage process whereby organizations transform ideas into new/improved products, service or processes, in order to advance, compete and differentiate themselves successfully in their marketplace.”
3.1.4. Big data
Laney (2001) described three essential dimensions of big data: “volume, velocity and variety” (the 3 V definition). Volume refers to ever-increasing amounts of data. Velocity indicates the need to capture and analyze high-speed data in (near) real-time or else the value may be lost. Variety relates to different types of data, be it structured or non-structured, such as social media posts or a video. In recent years, scholars and especially practitioners have developed numerous big data definitions. Ylijoki and Porras (Citation2016b) present a detailed discussion about big data definitions and the history of the term, concluding that the abovementioned dimensions are not only used in most of the definitions, but also form a logically coherent set. For the purposes of this research, the 3 V definition is adequate.
3.2. Innovation capabilities as mediator framework
The value proposition of big data is that companies can gain benefits by making use of it. Indeed, new, data-driven business models are emerging, like the data-as-a-service or analytics-as-a-service approaches discussed by Chen et al., Citation2011). To give another example, van’t Spijker (Citation2014) lists five value generation models for data-driven business: 1) selling data directly, 2) innovating products through data, 3) swapping commodity offerings into value-added services, 4) creating interaction in the value chain and 5) creating a network of value based on data exchange. One example of data monetization is presented by Najjar and Kettinger (Citation2013), who have investigated the process, benefits and drawbacks of the data monetization process in a large drug retailing firm. Internet of Things-related business models are evolving (Bucherer & Uckelmann, Citation2011; Chan, Citation2015; Leminen et al., Citation2012; Westerlund, Leminen, & Rajahonka, Citation2014). These examples clearly indicate that big data affects business models.
We adopt the business canvas model by Furr and Dyer (Citation2014) into our framework, shown in , as it covers essential elements from the innovation point of view. The business canvas model provides a (sufficiently) detailed starting point to understand the potential impact of big data in business context. Organizations can develop different scenarios using the canvas as a tool. It helps in concentrating on different viewpoints, such as streamlining core activities or developing new pricing strategies with data and analytics. On the other hand, it helps in seeing the big picture, as it covers all core functions of an organization. Moreover, we use the 3 V definition of big data (Laney, Citation2001), supplemented with a categorization element for each of the dimensions, in order to ease the use in practice.
The data deluge and changing business models are challenging incumbents to develop new capabilities. Approaches using resource-based theory have been popular among big data researchers recently, (Braganza, Brooks, Nepelski, Ali, & Moro, Citation2017; Gupta & George, Citation2016; Mikalef et al., Citation2017). We make no exception to the approach. However, we wish to add to the conversation the role of innovation capabilities in shaping the business model, as shown in . In the big data context, we distinguish between “data- and human-driven” innovations.
The “human-driven approach” to innovation states that innovations stem from people (Harmaakorpi & Melkas, Citation2012). New ideas – the seeds of innovations – often emerge sub-consciously, when our brain associates things. Dyer, Gregersen, and Christensen (Citation2008) claim that by training certain skills systematically, anyone can accelerate associative thinking. The four discovery skills are: observing (especially looking for surprises), networking with people from different backgrounds, experimenting a lot and questioning the status quo. Innovative people spend more time exercising these skills than others. New ideas emerge from self-transcending knowledge (Scharmer, Citation2001) as a result of associations. Self-transcending knowledge is future-oriented knowledge. It could be considered to be tacit knowledge prior to its embodiment. A good example of a person utilizing this approach was Steve Jobs.
Enabling human-driven innovation requires that individuals may use part of their time in seemingly unproductive discovery-skills development. This is important for developing self-transcending knowledge. Another requirement is that the organization’s culture and management practices must support risk-taking and allow mistakes. Dyer, Gregersen, and Christensen (Citation2011) present four principles that the most innovative organizations apply: 1) innovation is everybody’s job, 2) both incremental and disruptive innovations must be considered, 3) innovations are developed in small teams and 4) managed risks must be taken. These are by no means trivial requirements: e.g. Sandberg and Aarikka-Stenroos (Citation2014) identify a restrictive mindset, lack of discovery competences and unsupportive organizational structures as the three main internal barriers to radical innovations in incumbent firms.
Another way to view innovations relies on data and automation. A novel approach, “innovation automation,” is data-driven. Data-driven innovation suggests that the innovation processes could and should, be automated (Shaughnessy, Citation2015). This approach puts technology and (big) data at the core of the innovation processes. More and more data becomes available, technological and analytical capabilities are increasing and data processing costs are decreasing. Utilizing automation and vast volumes of different data will produce a more holistic view, leading to more data-driven decisions and more agile innovation processes. For example, big data might be used for early recognition of trends, combined with automatized simulations of possible scenarios in order to fertilize people’s thinking and, in turn, new human innovations. An example of innovation automation approach is Netflix, a company offering on-demand movies and television series.
Data-driven innovation, especially when combined with rapid experiments, can significantly speed up the innovation process, as the Netflix (Amatriain, Citation2013) example shows. Manyika et al. (Citation2011) claim e.g. that manufacturing firms can reduce their product development costs by 20–30% as well as achieve up to 50% faster time-to-market cycle by utilizing big data. One case study of utilizing data to drive innovations is documented by Jetzek, Avital, and Bjorn-Andersen(2014). However, for most incumbents, innovating with big data is in its infancy.
Innovation capabilities are at the core of the transformation of a firm. Therefore, understanding the nature of innovation is a prerequisite of a fertile innovation process. However, the kind of activities that efficient innovation processes require are in contrast with the current procedures of most incumbent firms. Incumbents organize themselves to be effective. They concentrate on minimizing all kinds of waste (like allocating time to seemingly unproductive activities such as developing discovery skills) and avoiding errors. This indicates that focusing on the organizational aspects of innovation capabilities is an important factor for a successful business transformation.
4. Evaluating the framework
The purpose of demonstration and evaluation phases was to assess the applicability of the framework in practical situations in business. Another objective was to gather feedback in order to develop the framework further.
4.1. Demonstration
The following sections describe the demonstration phase. In addition to presenting our approach and the companies, we provide a synthesis of the findings.
4.1.1. Demonstration process
First, we presented the framework to two big data intensive firms. One operates in several countries, offering cloud-based human resource (HR) solutions, and the other is a startup company offering SaaS-based integrations. Both firms act as “data hubs” and deal with large amounts of data. This gives them opportunities to develop even smarter data-related services or products, like predictive HR signals or benchmarking analyses. Using the proof-of-concept approach, we demonstrated the usage of the framework through semi-structured interviews. The people we interviewed were members of the executive boards of their firms. Our aim was to find out the usability of the framework in firms that are in the quite early stages of exploiting big data. In the second iteration, we performed a “postmortem” examination of a real-world big data case. Reflecting the case against the framework, we explored how the framework might have helped the company to understand the impact of big data.
4.1.2. Demonstration contexts
The arrangements for the demonstrations of the framework were as follows: we prepared materials for the interviews beforehand. The material consisted of an overview presentation of the framework and a brief questionnaire to assess the current situation. As orientation material, we sent a set of preliminary questions to the interviewees a couple of days before the interviews. The duration of each interview was around two hours. We recorded the interviews and took notes. At the end, we asked for feedback, both verbal and written. Afterwards, we analyzed our notes and outlined them in memos. Any unclear points were checked in the recordings. The interviewees reviewed the memos.
The first iteration was to introduce the framework to the HR solutions provider and the integration services provider. Two interviewers participated in both interviews. Sympa, the HR solutions provider, is an established firm in the HR business. Their key offering is Sympa HR, a cloud-based tool for managing employment relationships, from recruitment to termination of employment. The firm is an established company that already has a large number of customers in several countries. However, they are seeking rapid growth. Their management has discussed big data, although they have not yet formulated a clear big data strategy.
The other firm, Flashnode, is a Finnish startup offering services for interconnecting and synchronizing data. The firm’s business model relies on highly standardized integrations, which challenges the traditional tailored interface development approach. A key objective of automated integrations is to reduce the manual work done by client companies. They have an aggressive growth strategy. Their position as a data hub offers various opportunities to utilize big data. The company accumulates huge amounts of data in terms of transaction volumes.
The second iteration was the postmortem examination of an existing big data initiative. A bus company operating 430 buses in urban and suburban areas wanted to improve their cost efficiency. A potential target for this purpose was to reduce fuel costs, as these costs represent the company’s second largest expense. Meeting the business goal would require a transformation process consisting of training and motivating of the drivers, and a technical solution to collect detailed fuel consumption data. We applied the same procedures as in the first iteration, with the exception that only one interviewer was present. During the analysis of the case, we interviewed the owner of the big data initiative (the chief technical officer, CTO) and inspected the software vendor’s project documentation. The bus firm treated the initiative as a business development program
4.1.3. Demonstration findings
Here are the key findings of research and interviews of three different firms. The HR solution provider firm sees several areas where big data can add value to their business, such as integrating external data, utilizing metadata that their platform generates, and creating a “Sympa tribe” ecosystem for their customers. Moreover, they increasingly utilize sensor data, such as access control logs and working time recordings.
In the second case the provider of system integration the data is internally mostly applied to product development prioritization. Based on the big data, internal analyzes have also been carried out to compare customer-specific data volumes and other business customers trends. In this case, customer data is enriched with other external sources. Based on the above, the purpose is to develop a reporting service that provides benchmarking data to customers. For example, how does our business compare to industry in general in terms of transactions of subscription volumes.
In the case of the bus company they set the business goal as follows: to educate and motivate the drivers to change their driving habits, which will in turn lead to reduction in fuel consumption. They appointed the CTO to lead the program. They consulted potential software and hardware vendors and selected the technologies. The implementation started with a pilot project, which integrated the technologies using data from ten buses. After an assessment of the pilot, a full-scale implementation project followed. During the following months, the project team implemented a production-ready system and solved several data quality-related issues. At the same time, the firm created a change management plan for the release and rollout. After two years of use, the system has proved to be a success. It has met the original business goal. The system and its usage have costs, but the net result is a significant cost reduction due to permanently lower fuel consumption. Moreover, the firm has recorded remarkable improvement with regard to the traveling experience in customer satisfaction surveys. The passengers have noticed that the drivers drive smoothly. A third achieved benefit is, of course, the reduction of carbon-di-oxide emissions.
4.2. Evaluation
In this section we first analyze our findings from the demonstration. Next, we offer building blocks for practitioners by describing an exemplary process and discussion on how to apply the framework in practical situations.
4.2.1. Evaluation of the demonstration
The first iteration of the demonstration phase confirmed that the framework provides a mental model for evaluating the effects of big data in the business context. The interviewees found the framework useful for this purpose. The HR solution provider emphasized that the framework helps to create a systematic approach that will save time. Managing a growing business is hectic; they could not tie key resources to long consulting projects. The integration services provider stated that the framework offers guidance and provides understanding of big data adoption. They saw big data as a strategic issue. Applying the framework would help identify the required changes, as the business model aspect of the framework emphasizes the strategic importance of big data. Another key aspect of the framework is focusing on the importance of data, as “you can always build or buy algorithms, but you cannot generate data from the void.”
In both firms, we noted that the framework helped keep the discussion focused. It helped in keeping concentrated on one topic at a time. Moreover, the discussion regarding new ideas was lively. Although this may be related to personal characteristics, the framework facilitated the ideation. Both firms considered the framework useful in their feedback. After the first iteration, we reflected the discussions in our framework. From the theory point of view, no new aspects were recognized. By looking at the practical side, the interviewees appreciated our orientation material, as it helped them to perceive the framework.
In the second iteration, we analyzed the bus firm’s big data initiative. The initiative started when the firm noticed that the current technology enabled them to collect detailed data from the buses. This idea led ultimately to the big data initiative. The management of the company considered that the big data initiative would strengthen their competitive situation due to increased operational efficiency.
The firm set a clear objective for the program, although they did not define an explicit value for it. They also set a member of the executive board (the CTO) to lead the program. They focused on meeting the business goal, i.e. reducing fuel consumption. The firm also recognized real-time monitoring needs on the horizon. However, as the fuel consumption analysis did not require real-time processing, they decided to postpone the implementation to later phases. The project team, consisting of members of the firm and a software vendor, identified the required datasets and drew up a cost-effective architecture without paying attention to additional features. In the pilot phase, neither the firm nor the software vendor considered real-time needs. The project members may have discussed possible future scenarios in their coffee breaks, but the project did not consider those scenarios officially. This ultimately led to a situation where further development to meet the real-time needs is difficult.
Our framework would have helped the bus firm to understand the situation better at the beginning of the program. The owner of the big data initiative shared this view and saw that the framework would have been helpful. A better understanding of the data might have helped them to earlier identify certain data-related issues that reflected on the attitudes of middle management. Moreover, the framework would have helped to develop capabilities to tackle the data issues. Another capability-related matter was the shortage of analytical capabilities, which has been a hindrance to making use of the gathered data. In addition, using the framework in the early phases would probably have stressed the future needs. This, in turn, would probably have led to a different architecture or technology selection in the project phase.
The two companies that had less experience with big data paid only a little attention to developing capabilities, whereas the postmortem indicated that more attention should have been paid to capability development during the project. Another observation, although an expected one, was that the usage of the framework benefits from facilitation. Preparing supporting material for the interviews and group work adds a middle layer between the framework and the daily operations. This helps the participants connect the framework concretely with their own context. Using a few questions or brief examples that concretely link to the firm’s current situation would concretize the subject and help link big data to the firm’s business context even better than a generic approach.
The demonstration phase confirmed our initial assumption that simplicity is a virtue when providing building blocks to practitioners. This assumption in mind we carefully considered in the first place the balance between theoretical completeness and practical viewpoints in the presentation of the framework. E.g., the simplified business canvas model (Furr & Dyer, Citation2014) we chose, covers essential components from innovation point of view. However, it should be noted that extension to full business model canvas (Osterwalder & Pigneur, Citation2010) can easily be done (by scholars or practitioners) whenever required.
4.2.2. Suggestions for making use of the framework
Companies can choose different practical approaches to the framework according their situation and objectives. Increasing the role of data in current business model, e.g. by automating delivery processes to decrease transaction costs requires a different approach than developing a new, data-driven model. Based on the evaluation phase of our framework, we synthesized an exemplary usage scenario as follows.
Assess current situation and big data impact: This can be done by looking at the business model components (see ) one by one and creating scenarios of potential big data effects. Effects can be either risks or opportunities; some of the effects are such that they cannot be influenced, some of them are within the reach of the company.
Define business objectives. Clear goals and ownership are best practices for any business development initiative. Especially in the case of new things solid leadership is important to achieve the set goals. This phase might be iterative, innovating back and forth between the business model and big data. What internal or external data could affect our business? What data do we need in order to best run our business? Novel ideas or long-term, strategic goals often require experimenting for verification.
Evaluate/ideate big data value potential. Many innovations are human-driven, but increasingly, data are a source of innovations. Humans ideate things that require gathering and combining new and existing data in novel ways. Accordingly, experimenting with data may reveal insights that spark ideas. Human-driven and data-driven innovations are not mutually exclusive. Instead, they can, and should, support each other, effectively creating an innovation loop. Our framework provides support for these activities by explaining the theoretical background and enablers for an effective innovation process.
Identify required datasets and capabilities. Identifying data that is beneficial to the business is a two-way operation. As one may intuitively think when looking at our framework (), human-driven innovation comes down to a question: “What data do we need to fulfill this business need?” On the other hand, data-driven innovations ask: “Do we have data or can we access data that is beneficial to our business?” Once the datasets are identified, the modified 3V model shown in can be used to classify and categorize the data at a more detailed level. This, in turn, reveals possible gaps in ICT capabilities.
As another building block, and from the IT point of view, the 3 V model of big data (Laney, Citation2001) is a good starting point. Adding categorization elements to the dimensions (see ) and classifying any available or required data source (internal or external) accordingly helps to identify potential development needs of the current IT platform. For example, a business need may need to harvest and analyze social media data. The framework helps to translate the business need into an IT-related requirement: Do we have the hardware and software that is required to gather and process (relatively) low volumes of unstructured data in near real-time? It should, however, be noted that there are technical and data-related challenges (Benabdellah et al., Citation2016; Ylijoki & Porras, Citation2016a). Technical challenges are hardware- and software -related, such as managing vast volumes of data with a Hadoop cluster, or using a not only SQL (NoSQL) database to store unstructured data. Analytical capabilities are human-centric, like building a predictive analytics model or interpreting a business need into an algorithm. A firm can develop technical and analytical capabilities in-house, or it can leverage service providers.
5. Conclusion
The paradigm shift towards more data-intensive business landscape is inevitable. Companies must consider the combination of big data, innovations and potential value against the required transformation when they plan their big data initiatives. For incumbents, the transformation may be more revolutionary than evolutionary, which implies a complicated process. Our practitioner oriented framework helps in understanding the role of innovations and (big) data in the digital transformation. Understanding these factors forms the basis for informed management decisions regarding business transformation and the capabilities the transformation requires.
In this paper, we have presented a multi-disciplinary framework that contributes to research by pinpointing the role of human and data-driven innovation capabilities as a mediator between big data and the business model. The framework combines elements from strategic management, innovations research and resource-based theory. It helps to understand the role of human and data-driven innovations, and innovation capabilities in an organizational context. Thus, the framework acts as a mental model that offers a way to organize perspectives on the digital transformation, especially from the practitioner’s point of view. Understanding the theoretical background of the innovation process in the big data context will help practitioners to focus on developing the capabilities and methods that best support the transformation towards data driven business models.
References
- Akter, S., Wamba, S. F., Gunasekaran, A., Dubey, R., and Childe, S. J. (2016). How to improve firm performance using big data analytics capability and business strategy alignment? International Journal of Production Economics, 182, 113–131.
- Amatriain, X. (2013). Beyond data: from user information to business value through personalized recommendations and consumer science. Paper presented at Proceedings of the 22nd ACM International Conference on Information & Knowledge Management, San Francisco, California, USA, October 27, November 01, 2013 (pp. 2201–2208).
- Amit, R., and Zott, C. (2001). Value creation in e-business. Strategic Management Journal, 22(6-7), 493–520.
- Baregheh, A., Rowley, J., and Sambrook, S. (2009). Towards a multidisciplinary definition of innovation. Management Decision, 47(8), 1323–1339.
- Baskerville, R. L. (1999). Investigating information systems with action research. Communications of the Association for Information Systems: 2(19). DOI: https://doi.org/10.17705/1CAIS.00219
- Benabdellah, A. B., Asmaa, B., Imane Z, and El Moukhtar. (2016). Big data for supply chain management: opportunities and challenges. Paper presented at IEEE/ACS 13th International Conference of Computer Systems and Applications (AICCSA) 29 Nov.–2 Dec. 2016, Agadir, Morocco.
- Benbasat, I., and Zmud, R. W. (1999). Empirical research in information systems: The practice of relevance. MIS Quarterly, 23(1), 3–16.
- Braganza, A., Brooks, L., Nepelski, D., Ali, M., and Moro, R. (2017). Resource management in big data initiatives: Processes and dynamic capabilities. Journal of Business Research, 70, 328–337.
- Brynjolfsson, E., Hitt, L. M., and Kim, H. H. (2011). Strength in Numbers: How does data-driven decision-making affect firm performance?” (2011). ICIS 2011 Proceedings. 13. https://aisel.aisnet.org/icis2011/proceedings/economicvalueis/13
- Bucherer, E., and Uckelmann, D. (2011). Business Models for the Internet of Things. In: Uckelmann D., Harrison M., Michahelles F. (eds) Architecting the Internet of Things. Berlin, Heidelberg: Springer.
- Burkhart, T. K., Julian, W., and Dirk, L. P. (2011). Analyzing the business model concept—A comprehensive classification of literature. Paper presented at Thirty Second International Conference on Information Systems, December 2–5, Shanghai China 2011.
- Casadesus-Masanell, R., and Ricart, J. E. (2010). From strategy to business models and onto tactics. Long Range Planning, 43(2-3), 195–215.
- Chan, H. C. (2015). Internet of Things business models. Journal of Service Science and Management, 8(4), 552–568.
- Chen, Y. K., Jeffrey, C. M., and Abrams, C. (2011). Analytics ecosystem transformation: a force for business model innovation. Paper presented at SRII Global Conference (SRII), 2011 Annual 29 March; 2 April 2011, San Jose, CA, USA (pp.11–20).
- Christensen, C. (2013). The innovator’s dilemma: When new technologies cause great firms to fai. Boston, Massachusetts: Harvard Business Review Press, 5th edition.
- Dehning, B., Richardson, V. J., and Zmud, R. W. (2003). The value relevance of announcements of transformational information technology investments. MIS Quarterly, 27(4), 637–656.
- Dyer, J., Gregersen, H., and Christensen, C. (2008). Entrepreneur behaviors, opportunity recognition, and the origins of innovative ventures. Strategic Entrepreneurship Journal, 2(4), 317–338.
- Dyer, J., Gregersen, H., and Christensen, C. (2011). The Innovator’s DNA. Boston, Massachusetts: Harvard Business Review Press.
- Ettlie, J. E., and Reza, E. M. (1992). Organizational integration and process innovation. Academy of Management Journal, 35(4), 795–827.
- Furr, N., and Dyer, J. (2014). The innovator’s method. Boston, Massachusetts: Harvard Business Review Press.
- Gupta, M., and George, J. F. (2016). Toward the development of a big data analytics capability. Information & Management, 53(8), 1049–1064.
- Harmaakorpi, V., and Melkas, H. (2012). Practice-based innovation. insights, applications and policy implications. Berlin, Germany: Springer.
- Hartmann, P., Zaki, M. E., Feldmann, N., and Neely, A. D. (2016). Capturing value from big data–A taxonomy of data-driven business models used by start-up firms. International Journal of Operations & Production Management, 36(10), 1382–1406.
- Hevner, A. R., et al. (2004). Design science in information systems research. MIS Quarterly, 28(1), 75–105.
- Iansiti, M., and Levien, R. (2004). Strategy as ecology. Harvard Business Review, 82(3), 68–81.
- Iivari, J. (2003). The IS core-VII: towards information systems as a science of meta-artifacts. Communications of the Association for Information Systems, 12(1), 568–581.
- Jetzek, T., Avital, M., and Bjorn-Andersen, N. (2014). Data-driven innovation through open government data. Journal of Theoretical and Applied Electronic Commerce Research, 9(2), 100–120.
- Laney, D. (2001). 3D data management: controlling data volume, velocity and variety. Meta Group Research Note, 6, 70–73.
- Leminen, S., et al. (2012). Towards IoT ecosystems and business models. In, Internet of Things, smart spaces, and next generation networking. A. Sergey, B. Sergey, K. Yevgeni, (Eds.), St. Petersburg, Russia: Springer, pp. 15–26.
- Lewin, K. (1947). Frontiers in group dynamics II. Channels of group life; social planning and action research. Human Relations, 1(2), 143–153.
- Lycett, M. (2013). Datafication’: making sense of (big) data in a complex world. European Journal of Information Systems, 22(4), 381–386.
- Manyika, J., et al. (2011). Big data: The next frontier for innovation, competition, and productivity. In J. Manyika and M. Chui, (Eds.), McKinsey Global Institute. Available at: http://www.mckinsey.com/insights/business_technology/big_data_the_next_frontier_for_innovation.
- McAfee, A., and Brynjolfsson, E. (2012). Big data: The management revolution. Harvard Business Review, 90(10), 61–67.
- Mikalef, P., et al. (2017). Big data analytics capabilities: A systematic literature review and research agenda. Information Systems and e-Business Management, 16(3), 1–32.
- Moore, J. F. (1993). Predators and prey: A new ecology of competition. Harvard Business Review, 71(3), 75–83.
- Najjar, M. S., and Kettinger, W. J. (2013). Data monetization: Lessons from a retailer’s journey. MIS Quarterly Executive, 12(4), 213–225.
- Osterwalder, A., and Pigneur, Y. (2010). Business model generation: A handbook for visionaries, game changers, and challengers, Hoboken, NJ: John Wiley & Sons.
- Peffers, K., Tuunanen, T., Rothenberger, M. A., and Chatterjee, S. (2007). A design science research methodology for information systems research. Journal of Management Information Systems, 24(3), 45–77.
- Porter, M. E. (1991). Towards a dynamic theory of strategy. Strategic Management Journal, 12(S2), 95–117.
- Porter, M. E., and Millar, V. E. (1985). How information gives you competitive advantage. Harvard Business Review, 64(4): 149–160.
- Ren, S. J.-F., et al. (2017). Modelling quality dynamics, business value and firm performance in a big data analytics environment. International Journal of Production Research, 55(17), 5011–5026.
- Sainio, L.-M. (2005). The effects of potentially disruptive technology on business model - a case study of new technologies in ICT industry. Lappeenranta, Finland: Lappeenranta University of Technology.
- Sandberg, B., and Aarikka-Stenroos, L. (2014). What makes it so difficult? A systematic review on barriers to radical innovation. Industrial Marketing Management, 43(8), 1293–1305.
- Scharmer, C. O. (2001). Self-transcending knowledge: Sensing and organizing around emerging opportunities. Journal of Knowledge Management, 5(2), 137–151.
- Schumpeter, J. A. (1942). Socialism, capitalism and democracy. United States: Harper and Brothers.
- Sein, M., et al. (2011). Action design research. MIS Quarterly, 35(1), 37–56.
- Shaughnessy, H. (2015). Shift: A user’s guide to the new economy, Boise, Idaho: Tru Publishing.
- Teece, D. J. (2010). Business models, business strategy and innovation. Long Range Planning, 43(2-3), 172–194.
- Teece, D. J. (2007). Explicating dynamic capabilities: The nature and microfoundations of (sustainable) enterprise performance. Strategic Management Journal, 28(13), 1319–1350.
- Timmers, P. (1998). Business models for electronic markets. Electronic Markets, 8(2), 3–8.
- Van’t Spijker, A. (2014). The new oil: Using innovative business models to turn data into profit. Basking Ridge, NJ: Technics Publications.
- Wamba, S. F., Gunasekaran, A., Akter, S., Ren, S. J-F., Dubey, R., and Childe, S. J. (2017). Big data analytics and firm performance: Effects of dynamic capabilities. Journal of Business Research, 70, 356–365.
- Weill, P., ans Woerner, S. L. (2015). Thriving in an increasingly digital ecosystem. MIT Sloan Management Review, 56(4), 27–34.
- Wernerfelt, B. (1984). A resource-based view of the firm. Strategic Management Journal, 5(2), 171–180.
- West, M. A., and Anderson, N. R. (1996). Innovation in top management teams. Journal of Applied Psychology, 81(6), 680–693.
- Westerlund, M., Leminen, S., and Rajahonka, M. (2014). Designing business models for the Internet of Things. Technology Innovation Management Review, 4(7), 5–13.
- Wixom, B. H., Yen, B., and Relich, M. (2013). Maximizing value from business analytics. MIS Quarterly Executive, 12(2), 111–123.
- Ylijoki, O., and Porras, J. (2016a). Conceptualizing big data: Analysis of case studies. Intelligent Systems in Accounting, Finance and Management, 23(4), 295–310.
- Ylijoki, O., and Porras, J. (2016b). Perspectives to definition of big data: A mapping study and discussion. Journal of Innovation Management, 4(1), 69–91.
- Zott, C., Amit, R., and Massa, L. (2011). The business model: Recent developments and future research. Journal of Management, 37(4), 1019–1042.