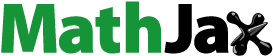
ABSTRACT
As a dominant type of gastric cancer, stomach adenocarcinoma (STAD) is characterized by high morbidity and mortality rates. Anoikis factors participate in tumor metastasis and invasion. This study was designed to identify prognostic risk factors in anoikis-related long non-coding RNAs (lncRNAs) for STAD. First, with STAD expression datasets and anoikis-related gene sets downloaded from public databases, anoikis-related prognostic lncRNA signatures (AC091057.1, ADAMTS9.AS1, AC090825.1, AC084880.3, EMX2OS, HHIP.AS1, AC016583.2, EDIL3.DT, DIRC1, LINC01614, and AC103702.2) were screened by Cox regression to establish a prognostic risk model. Kaplan–Meier and receiver operating characteristic curves were used to evaluate the survival status of patients and verify predictive accuracy of the model. Besides, risk score could be an independent prognostic factor to assess the prognosis of STAD patients. Nomograms of the prognostic model that combined clinical information and risk score could effectively predict survival of STAD patients, as validated by calibration curve. Gene ontology and Kyoto encyclopedia of genes and genomes enrichment analyses were performed for differentially expressed genes (DEGs) in high- and low-risk groups. These DEGs were related to neurotransmitter transmission, signal transmission, and endocytosis. Moreover, we analyzed immune status of different risk groups and found that STAD patients in low-risk group were more sensitive to immunotherapy. A prognostic risk assessment model for STAD using anoikis-related lncRNA genes was constructed here, which was proven to have high predictive accuracy and thus could offer a reference for prognostic evaluation and clinical treatment of STAD patients.
1. Introduction
Ranking fifth in the incidence rate among all cancers, gastric cancer (GC) is a malignancy of the digestive system, with stomach adenocarcinoma (STAD) being the most common subtype [Citation1]. Radical surgery combined with chemotherapy at the early stage is the most efficacious treatment for STAD, although postoperative metastases and recurrence remain a problem for some STAD patients [Citation2]. However, most patients with STAD are diagnosed at a rather advanced stage which is no longer the best time for surgery. Prognosis of patients with advanced STAD is far from desired with a median survival of only 8 months [Citation3]. Conventional therapies yield limited efficacy in patients with advanced GC, and immunotherapy may emerge as another efficient treatment after surgery, chemotherapy, radiotherapy, and target therapy [Citation3]. A prognostic model that enables efficient risk stratification of STAD patients will facilitate the prediction of the overall survival of patients and guide prognostic management.
As a specific form of programmed cell death, anoikis refers to apoptosis triggered by the detachment of normal epithelial cells or solid tumor cells without metastatic nature in situ into the blood [Citation4]. When cells are detached from the extracellular matrix, anoikis prevents tumor cells isolated from the primary tumor from continuing to survive [Citation5]. In this way, the anoikis factor is vital in the development and metastasis of STAD. Analysis of the prognosis of STAD based on the anoikis factor is conducive to the correct assessment of tumor development and metastasis risk. More than 200 nt in length, long non-coding RNAs (lncRNAs) are involved in many human cancers such as GC, lung cancer, liver cancer, pancreatic cancer, and colorectal cancer. Besides, lncRNAs are crucial in various biological processes such as cell proliferation, invasion, apoptosis, and stem cell maintenance [Citation6–10]. Li et al. pointed out that lncRNAs may be potential targets for cancer diagnosis and therapy [Citation10]. There is association between lncRNA expression and anoikis resistance and metastasis in cancer [Citation11–13]. Investigating the effect of anoikis-related lncRNAs on STAD may help doctors better evaluate the prognosis of STAD patients and explore possible targets for disease treatment.
However, to our knowledge, no studies have investigated the effect of anoikis-related lncRNAs on prognosis of STAD. With prognostic markers screened from anoikis-related lncRNAs in STAD, this study built a prognostic model of STAD to predict survival outcomes and draw nomograms with risk score to facilitate prognostic management of STAD patients. Besides, the immune status and response to immunotherapy in high- and low-risk groups were assessed to offer a reference for immune checkpoint blockade therapy and other immunotherapies for STAD.
2. 2. Materials and methods
2.1. Data extraction and differential analysis
From The Cancer Genome Atlas (TCGA) (https://portal.gdc.cancer.gov/), TCGA-STAD dataset (32 normal samples and 375 STAD samples) including RNA-seq expression data, SNV mutation data, and corresponding clinical data was downloaded. Anoikis-related mRNAs were acquired from Genecards (https://www.genecards.org/).
Differential analysis of mRNA expression retrieved from TCGA-STAD was performed using the edgeR package [Citation14] to screen differentially expressed genes (DEGs) (FDR <0.05, logFC > 1). DEGs and anoikis-related mRNAs were intersected to obtain differentially expressed anoikis-related mRNAs.
2.2. Construction of an anoikis-related lncRNA-based STAD risk prognostic model
With clinical information collected from the dataset, samples that survived 30 days were screened and randomly assigned into training and validation sets at a ratio of 7:3. LncRNAs strongly correlated with differentially expressed anoikis-related mRNAs were selected as the study subjects (cor ≥0.5). Univariate Cox regression analysis of selected lncRNAs was carried out using the survival package [Citation15]. The dimensionality of the model was reduced by LASSO analysis using the glmnet package [Citation16] to avoid overfitting. Following that, multivariate Cox regression analysis of the screened lncRNAs was performed with the survival package to construct the final prognostic model.
2.3. Validation of the prognostic model for STAD
The STAD samples were assigned into high- and low-risk groups by the median risk score. Kaplan–Meier survival curves of two groups were drawn using survival and survminer packages (https://rdocumentation.org/packages/survminer/versions/0.4.9). The training set was then analyzed using the time ROC package [Citation17] and the survival package. Receiver operating characteristic (ROC) curves for 1, 3, and 5 years were plotted using area under the curve (AUC) calculated. Finally, the validation set was utilized to assess predictive accuracy and performance of the model.
2.4. Independent prognostic factor analysis
Univariate and multivariate Cox regression analyses that included risk score of STAD samples and clinical information (age, gender, pathological stage, and other characteristics) were performed using the survival package for identifying independent prognostic factors. Forest plots were drawn using the forestplot package. The rms package [Citation18] and survival package were used to draw nomograms based on risk score and clinicopathologic factors (age, gender, T, and N) to further confirm the clinical application of the model. Calibration curves were drawn with the rms package [Citation18] to assess whether the predicted overall survival by nomogram was in accordance with the actual overall survival (1-year, 3-year, and 5-year).
2.5. Gene ontology (GO) and Kyoto encyclopedia of genes and genomes (KEGG) enrichment analyses
The edgeR package [Citation14] was applied for the differential analysis of high- and low-risk groups to screen DEGs (FDR <0.05, logFC > 1). GO and KEGG enrichment analyses of DEGs in the two risk groups were performed separately using the clusterProfiler package [Citation19].
2.6. Analyses of immune infiltrate and immune checkpoint
Tumor microenvironment (TME) of each STAD sample was assessed using the estimate package [Citation20]. Immune score, stromal score, and ESTIMATE score of each sample were calculated, and the Wilcox test was performed. Violin plots were plotted for the two risk groups.
Then, single-sample gene set enrichment analysis (ssGSEA) using GSVA package [Citation21,Citation22] was conducted to compare immune-related cells and functions in the two risk groups, respectively. Correlations between risk score, immune-related cell score, and immune-related function score were calculated to reveal the relation between immune level and risk score.
The expression of PDCD1/CTLA4 in the two groups was analyzed to assess the effectiveness of immunotherapy for patients in different risk groups. Tumor Immune Dysfunction and Exclusion (TIDE) scores were calculated on TIDE (tide.dfci.harvard.edu) to predict anti-tumor immune escape level in STAD patients during immunotherapy.
3. Results
3.1. Differential analysis, anoikis-related signature gene screening, and lncRNA-based prognostic model construction
After subjecting samples in TCGA-STAD to gene differential expression analysis, a total of 4705 DEGs in STAD that met the threshold (FDR <0.05, logFC > 1) were screened. DEGs and anoikis-related mRNAs (Table S1) were intersected to obtain 185 anoikis-related differentially expressed mRNAs (AnoRGs) ().
Figure 1. Differential analysis and construction of a STAD prognostic model.

A total of 496 lncRNAs that were in relatively high correlation (cor ≥0.5) with AnoRGs were screened. Referring to expression of these 496 lncRNAs and clinical information, samples with survival time >30 days were screened out and randomly divided into a training set (Table S2) and a validation set (Table S3) at a 7:3 ratio. Subsequently, univariate Cox regression analysis screened out 17 candidate lncRNAs dramatically associated with survival (FDR <0.05), among which 11 important feature lncRNAs were eventually selected after the LASSO Cox analysis (). A multivariate Cox regression model was constructed based on feature lncRNAs yielded from LASSO analysis (). Below is the final prognostic risk model for STAD:
3.2. Validation of the anoikis-related lncRNA risk prognostic model
Samples were split into high- and low-risk groups with the median risk score. From risk score distribution diagram () and survival state diagram (), it can be seen that the mortality rate of patients gradually increased with the risk score. The heat map of gene expression () showed a difference between high- and low-risk groups. In general, expression levels of AC084880.3, DIRC1, LINC01614, EMX2OS, AC016583.2, HHIP.AS1, ADAMTS9.AS1, AC090825.1, and EDIL3.DT were higher in high-risk group than in low-risk group, while AC091057.1 and AC103702.2 expression levels were lower in high-risk group. We plotted ROC curves with the training set to evaluate accuracy of the STAD prognostic model. The values of AUC for 1, 3, and 5 years were 0.62, 0.69, and 0.68, respectively (). Survival analysis displayed lower survival rate in high-risk group (p < 0.01) ().
Figure 2. Validation of the predictive performance of the STAD prognostic model based on anoikis-related lncRNAs.

Predictive performance of the model was further validated by ROC and survival curves using the validation set. The AUC values were 0.65, 0.75, and 0.81 at 1, 3, and 5 years, respectively (). Survival curves of the validation set were similar to those of the training set, showing a remarkably lower survival rate in high-risk group (p < 0.01) (). It could be concluded that this established prognostic model had good predictive performance and high accuracy.
3.3. Analysis of independent prognostic factor and clinical significance
To further investigate whether the STAD prognostic model could be an independent prognostic factor, we performed univariate and multivariate Cox regression analyses combining with clinical information. The P values of risk score were less than 0.01, indicating that risk score could be an independent prognostic factor (). To predict survival in STAD patients, risk score was combined with other clinicopathological features to draw nomograms (). The results of calibration curves showed that predicted survival of the prognostic model fitted well with actual survival (). With high accuracy and fitting degree, the risk prognostic model constructed from these 11 signature lncRNAs can effectively assess the prognostic risk of STAD patients.
Figure 3. Analysis of the model as an independent prognostic factor.

3.4. GO and KEGG enrichment analyses
Differential analysis was conducted in two groups to analyze the reasons for survival differences between high- and low-risk groups. After that, enrichment analyses of 1340 DEGs were completed using GO and KEGG to study their differences in biological functions as well as pathways. GO analysis uncovered that these genes were mainly enriched in functions such as muscle system processes, collagen-containing extracellular matrix, receptor–ligand activity, signaling receptor activator activity, and neuronal cell bodies (). KEGG results showed that these genes were mainly enriched in pathways such as PI3K-Akt signaling pathway, neuroactive ligand–receptor interaction, focal adhesions, cAMP signaling pathway, and calcium signaling pathway (). These enrichment results suggested that anoikis-related genes responsible for STAD risk score differences were mainly associated with neurotransmitter transmission, signal transmission, and endocytosis.
3.5. Assessment of immune infiltration levels in different risk groups
We first scored stromal cell fraction and immune cell fraction of samples and performed the differential analysis of immune scores in high- and low-risk groups. It was found that stromal score, immune score, and ESTIMATE score were higher in high-risk group (p < 0.001) (). Results from the ssGSEA approach showed that macrophages, neutrophils, T helper cells, T follicular helper cells, and regulatory T cells (Treg) were significantly higher in high-risk group (p < 0.001) (). Differential analysis of immune function showed that CCR, inflammation-promoting, T cell co-inhibition, para-inflammation, and Type II IFN response were significantly higher in high-risk group than in low-risk group, which may correlate with tumor progression (p < 0.001) (). Based on the above-mentioned observations, prognostic risk score should be associated with the immune microenvironment.
Figure 5. Assessment of immune infiltration in the high- and low-risk groups.

3.6. Expression of immune checkpoint molecule and TIDE score results
Although antibodies against immune regulators CTLA4 and PD-L1/PD-1 are proven to be successful in a variety of cancers, only a subset of people present durable responses [Citation23]. In this study, we further analyzed the response to immunotherapy in high- and low-risk groups by assessing expression of PDCD1 and CTLA4. The results found significantly higher expression of PDCD1 and CTLA4 in high-risk group (p < 0.01) ().
Figure 6. Expression of immune checkpoint molecule and TIDE scores for high- and low-risk groups.

According to the TIDE database, the TIDE scores of samples from different risk groups (Table S4) were calculated to predict the effect of immune checkpoint inhibition therapy. With lower TIDE scores in low-risk group and higher TIDE scores in high-risk group, patients in high-risk group were prone to immune escape (p < 0.001) ().
4. Discussion
Despite thorough research and substantial improvement in diagnosis and treatment of STAD, the morbidity and mortality rates remain high [Citation24]. Several studies have demonstrated that anoikis exerts critical function in tumor invasion and metastasis [Citation4]. Multiple mechanisms and TME contribute to anoikis resistance in cancer cells to counteract apoptosis mechanisms and maintain pro-survival signals to counteract allergic reactions after detachment from stromal surfaces. All these events are considered promising targets for antimetastatic pharmacological therapy. Therefore, the impact of anoikis-related lncRNAs on prognosis of STAD became the focus of this study.
Based on 11 feature lncRNAs related to the prognosis of STAD, namely, AC091057.1, ADAMTS9.AS1, AC090825.1, AC084880.3, EMX2OS, HHIP.AS1, AC016583.2, EDIL3.DT, DIRC1, LINC01614, and AC103702.2, screened from AnoRGs-related lncRNAs in this study, the prognosis model was constructed. Immune-related AC091057.1 has been used to construct the lung adenocarcinoma risk signature [Citation25]. ADAMTS9.AS1 regulates colorectal cell migration and proliferation in vivo and in vitro and inhibits Wnt/β-catenin signaling pathway from participating in its mediated gene transcriptional regulation [Citation26]. EMX2OS, an enhancer RNA from enhancer regions, bears some relation with poor survival outcomes in STAD [Citation27]. The aberrant expression of HHIP-AS1, which is actively transcribed from a bidirectional promoter shared with the Sonic Hedgehog (SHH) regulator HHIP, is a hallmark of the SHH signaling pathway driving tumors [Citation28]. Increased expression of DIRC1 in gastric cancer tissue may influence cancer cell proliferation, invasion, and migration through the AKT/mTOR signaling [Citation29]. Since AC090825.1, AC084880.3, AC016583.2, EDIL3.DT, and AC103702.2 have been rarely reported in cancer studies, the molecular mechanism by which these lncRNAs are involved in cancer is unknown. In summary, most of these lncRNAs directly or indirectly affect tumor development and progression by regulating cell proliferation and migration or acting on signaling pathways.
Targeted immune checkpoint therapy is a novel treatment for GC, although the immune response is absent in some patients [Citation30,Citation31]. We assessed immune infiltration levels among STAD patients who did respond to immunotherapy. Immunosuppressive Treg cells can induce immune escape in TME, thereby promoting tumor metastasis and progression [Citation32]. Significant differences in inflammation-promoting and part inflammation functions suggest that inflammation may greatly contribute to malignant tumor development and progression [Citation33]. Enhanced enrichment of T cell co-inhibition function observed in high-risk group may inhibit T cell activation. Subsequently, we further investigated the response to immunotherapy in high- and low-risk groups. The difference in expression of PDCD1 and CTLA4 suggested that STAD tumor cells in high-risk group were more likely to evade attack by the host immune system, which was also confirmed by the TIDE score. Taken together, it can be seen that the prognostic risk of STAD constructed by anoikis-related factors can distinguish the immune level of patients and provide a reference for STAD patients considering immunotherapy in clinical practice.
Although the STAD prognostic model is constructed by standard procedures and had its performance proved by convincing evidence, unfortunately, there still exist some limitations. Firstly, the molecular function of some prognostic risk markers in STAD is unknown. Secondly, this study used only retrospective data to construct and validate the STAD prognostic risk model. Clinical observation should be included to further verify predictive ability of the prognostic model and evaluate its value in clinic.
Author contribution
Conceptualization: Lina Lu, Guofa Jiang
Data curation: Min Yu
Formal Analysis: Wei Huang
Acquisition: Wei Huang
Investigation: Hui Chen
Methodology: Hui Chen
Project administration: Guofa Jiang
Resources: Guofa Jiang
Software: Guofa Jiang
Supervision: Lina Lu
Validation: Gangxiu Li
Visualization: Gangxiu Li
Writing – original draft: Lina Lu, Min Yu
Writing – review & editing: All authors
Declaration of Conflicting Interests
The authors report no conflict of interest.
Ethics approval and consent to participate
Not applicable.
Supplemental Material
Download Zip (2.3 MB)Disclosure statement
No potential conflict of interest was reported by the author(s).
Data availability statement
The datasets generated and analyzed during the current study are not publicly available but are available from the corresponding author on reasonable request.
Supplementary material
Supplemental data for this article can be accessed online at https://doi.org/10.1080/19932820.2023.2220153.
Additional information
Funding
References
- Sung H, Ferlay J, Siegel RL, et al. Global cancer statistics 2020: globocan estimates of Incidence and mortality worldwide for 36 cancers in 185 countries. CA Cancer J Clin. 2021;71:209–9.
- Zhou R, Peng N, Li W. Constructing a novel gene signature derived from oxidative stress specific subtypes for predicting survival in stomach adenocarcinoma. Front Immunol. 2022;13:964919.
- Li K, Zhang A, Li X, et al. Advances in clinical immunotherapy for gastric cancer. Biochim Biophys Acta Rev Cancer. 2021;1876(2):188615.
- Sun Z, Zhao Y, Wei Y, et al. Identification and validation of an anoikis-associated gene signature to predict clinical character, stemness, IDH mutation, and immune filtration in glioblastoma. Front Immunol. 2022;13:939523.
- Majidpoor J, Mortezaee K. Steps in metastasis: an updated review. Med Oncol. 2021;38:3.
- Xing C, Sun SG, Yue ZQ, et al. Role of lncRNA LUCAT1 in cancer. Biomed Pharmacother. 2021;134:111158.
- Loewer S, Cabili MN, Guttman M, et al. Large intergenic non-coding RNA-RoR modulates reprogramming of human induced pluripotent stem cells. Nat Genet. 2010;42:1113–1117.
- Gupta RA, et al. Long non-coding RNA HOTAIR reprograms chromatin state to promote cancer metastasis. Nature. 2010;464:1071–1076.
- Venkatraman A, et al. Maternal imprinting at the H19–Igf2 locus maintains adult haematopoietic stem cell quiescence. Nature. 2013;500(7462):345–349. DOI:10.1038/nature12303
- Li J, Meng H, Bai Y, et al. Regulation of lncRNA and its role in cancer metastasis. Oncol Res. 2016;23:205–217.
- Dai W, Tian C, Jin S. Effect of lncRNA ANRIL silencing on anoikis and cell cycle in human glioma via microRNA-203a. Onco Targets Ther. 2018;11:5103–5109.
- Seitz AK, et al. Profiling of long non-coding RNAs identifies LINC00958 and LINC01296 as candidate oncogenes in bladder cancer. Sci Rep. 2017;7:395.
- Adeshakin FO, et al. Mechanisms for modulating anoikis resistance in cancer and the relevance of metabolic reprogramming. Front Oncol. 2021;11:626577.
- Robinson MD, McCarthy DJ, Smyth GK. edgeR: a bioconductor package for differential expression analysis of digital gene expression data. Bioinformatics. 2010;26:139–140.
- Sengupta RBD Modeling survival data: extending the cox model by terry m. Therneau; Patricia MG. Modeling Survival Data: Extending the Cox Model by Terry M. Sankhya Ser A. 2003;65:843–844. Therneau.
- Friedman J, Hastie T, Tibshirani R. Regularization paths for generalized Linear Models via Coordinate Descent. J Stat Softw. 2010;33(1):1–22.
- Blanche P, Dartigues JF, Jacqmin-Gadda H. Estimating and comparing time-dependent areas under receiver operating characteristic curves for censored event times with competing risks. Stat Med. 2013;32(30):5381–5397.
- Huang C, Liu Z, Xiao L, et al. Clinical significance of serum ca125, ca19-9, ca72-4, and fibrinogen-to-lymphocyte ratio in gastric cancer with peritoneal dissemination. Front Oncol. 2019;9:1159.
- Yu G, Wang LG, Han Y, et al. clusterProfiler: an R package for comparing biological themes among gene clusters. OMICS. 2012;16(5):284–287.
- Yoshihara K, et al. Inferring tumour purity and stromal and immune cell admixture from expression data. Nat Commun. 2013;4:2612.
- Hanzelmann S, Castelo R, Guinney J. GSVA: gene set variation analysis for microarray and RNA-seq data. BMC Bioinf. 2013;14:7.
- Buchbinder EI, Desai A. ***CTLA-4 and PD-1 pathways: similarities, differences, and implications of their inhibition. Am J Clin Oncol. 2016;39(1):98–106.
- Chen DS, Mellman I. Elements of cancer immunity and the cancer–immune set point. Nature. 2017;541(7637):321–330.
- Wang N, Liu D. Identification and validation a necroptosis related prognostic signature and associated regulatory axis in stomach adenocarcinoma. Onco Targets Ther. 2021;14:5373–5383.
- Jin D, Song Y, Chen Y, et al. Identification of a seven-lncRNA immune risk signature and construction of a predictive nomogram for lung adenocarcinoma. Biomed Res Int. 2020;2020:1–17.
- Li N, et al. Long non-coding RNA ADAMTS9-AS1 suppresses colorectal cancer by inhibiting the wnt/beta-catenin signalling pathway and is a potential diagnostic biomarker. J Cell Mol Med. 2020;24:11318–11329.
- Liu GX, Tan YZ, He GC, et al. EMX2OS plays a prognosis-associated enhancer RNA role in gastric cancer. Medicine (Baltimore). 2021;100:e27535.
- Bartl J, et al. The HHIP-AS1 lncRNA promotes tumorigenicity through stabilization of dynein complex 1 in human SHH-driven tumors. Nat Commun. 2022;13:4061.
- Li Z, Yang AJ, Wei FM, et al. Significant association of DIRC1 overexpression with tumor progression and poor prognosis in gastric cancer. Eur Rev Med Pharmacol Sci. 2018;22:8682–8689.
- Zhao Q, et al. Immunotherapy for gastric cancer: dilemmas and prospect. Brief Funct Genomics. 2019;18:107–112.
- Zeng C, et al. Identification and validation of a novel cellular senescence-related lncRNA prognostic signature for predicting immunotherapy response in stomach adenocarcinoma. Front Genet. 2022;13:935056.
- Chen S, Gu J, Zhang Q, et al. Development of biomarker signatures associated with anoikis to predict prognosis in endometrial carcinoma patients. J Oncol. 2021;2021:3375297.
- Diakos CI, Charles KA, McMillan DC, et al. Cancer-related inflammation and treatment effectiveness. Lancet Oncol. 2014;15(11):e493–503.