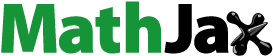
Abstract
This numerical study investigated a single air jet impingement at a constant temperature. Different RANS turbulence models, namely the v2-f model and four Reynolds stress turbulence models (the linear pressure-strain model, the quadratic pressure-strain model, the stress-omega model and the stress-BSL model) were employed to simulate and compare the numerical results with other published measurement data. Here, we show that the v2-f model has better numerical precision in simulating the local Nusselt number and velocity profiles and is in good agreement with a variety of Reynold numbers. These findings can assist scientists or engineers to improve mathematical turbulence modeling and industrial equipment for further development and to enable innovation.
1. Introduction
Jet impingement is important in many industrial applications, such as cooling internal combustion engines, gas turbines, heat treatment processes, defogging of optical surfaces and semiconductor components. At present, many scientists and engineers are striving to make the detailed calculations involved more efficient and accurate. An issue that is likely to cause an impasse in jet impingement studies is the flow at the stagnation point and a combination between laminar-turbulent transitions on the hot surface. The transition from a laminar to a turbulent regime is frequently triggered by instability (Ichimiya, Citation1999). In jet impingement, secondary vortices may occur due to the turbulent flow field along the wall and can be caused by turbulent variations in lateral/radial velocity related to pressure gradient changes, as seen in Figure , which can lead to separation or relainarization zones. Creating a separation or relainarization zone may increase localized heat transfer rates (Cousteix, Citation2003; Dewan et al., Citation2012).
The main reason for the poor rate of heat transfer happens the jet flow characteristic approaching the surface-induced stagnation or laminar deceleration region. The flow builds up a higher static pressure on the surface and loses the flow momentum in the radial direction, substantially impacting local turbulence and heat transfer properties (Zuckerman & Lior, Citation2006).
To better understand the previous scientific findings, this paper is organized as follows: section 1 describes the experimental research, numerical simulation study and exciting applications; section 2 explains the mathematical and numerical modeling; section 3 presents the computational investigation methodology; section 4 presents the computational investigation results; and section 5 provides the discussion of the results. Finally, section 6 discusses the main conclusions and findings.
1.1. Experiment research
Here, we have reviewed the experimental research related to jet impingement, which includes a hypothesis, a variable that the researcher can manipulate, and variables that can be measured. Initially, Kalifa et al. (Citation2016) investigated the dynamics of the turbulent behavior of jets impinging on a flat plate. The experimental investigations of the characteristics on a flat surface can be re depicted and conducted using particle image velocimetry. An impinging jet's behavior clearly shows that the co-flow stream imposed noticeable restrictions on the spreading of the impinging jet and considerably reduced the air jet's entrainment. Bader et al. (Citation2017) experimentally investigated relaminarization in accelerated flat-plate boundary-layer flows using laser Doppler anemometry. To understand the processes in the relaminarization mechanism, the mean value of velocity fluctuation was investigated and showed that strong acceleration leads to a suppression of turbulence production. Chen et al. (Citation2018) evaluated the local flow and average heat transfer characteristics of jet impingement cooling with supercritical pressure fluids using carbon dioxide. The microelectromechanical system (MEMS) method and a low thermal conductivity substrate were integrated with a thermal sensor chip to read and collect the hot surface temperature. The test data showed that the local heat transfer coefficient at the stagnation point improved with increasing heat flux. Singh et al. (Citation2020) determined the effect of the Reynolds number, nozzle-to-plate distance, and loading fraction. The results identified that raising the Reynolds number and loading fraction increased the Nusselt number but increasing the H/D ratio decreased the cooling performance. In addition, they proposed a correlation function based on the experimental data that a proper correlation function might be the best option in jet impingement studies. Issac et al. (Citation2020) applied the impingement of a round jet on a heated flat plate with constant heat flux, which was investigated experimentally and numerically at four different nozzle-to-plate spacings. The numerical results showed that the RNG k-ε turbulence model accurately predicted the outcomes except at stagnation, the k- shear stress transport (SST) turbulence model was in good agreement at various H/D ratios only for low Reynolds numbers, and the v2-f turbulence model predicted a more accurate Nusselt number at the stagnation results. Liu et al. (Citation2020) utilized the volume of fluid with pulse inlet pressure combined with the large eddy simulation (LES) turbulence model to understand the erosion area. They concluded that the frequency of the vortices shedding from the jet nozzle dominated pressure pulsation in the first ring area. Leocadio and Passos (Citation2021) experimentally and computationally investigated heat transport during water jet impingement cooling of a heated steel plate. The heat transfer coefficient (HTC) increased as the surface temperature decreased, and the correlations were used to predict the HTC and the rewetting front radius. This finding with a new correlation should enhance cooling system efficiency in hot strip mills.
1.2. Numerical simulation study
Many real-world engineering problems often encounter a design device with complicated geometry. Jet impingement has limitations in solving complex engineering problems, and the best-in-class turbulence model is still an open question. Large eddy simulation approach shows as a promising technique to simulate turbulent flows which work with many engineering applications (Zahiri & Roohi, Citation2021; Zahiri & Roohi, Citation2019; Lar-Kermani et al., Citation2018). For LES jet impingements, Shademan et al. (Citation2016) used an LES to analyze a turbulent impingement jet with a Reynold number of 28,000 compared with the particle image velocimeter technique. The results suggested that the mean normalized centerline velocity in the free jet, impingement areas, and pressure distribution were consistent between the models and tests. Also, they found that the vortices caused form the Kelvin-Helmholtz instabilities near the nozzle exit were combined, broken down and changed into large-scale structure configuration. Aillaud et al. (Citation2016) focused on a wall-resolved large eddy simulation (LES) of an adiabatic, circular, and submerged impinging jet on a hot flat plate, using a Reynolds number of 23,000 and a nozzle-to-plate distance (H/D) of 2.0. Two conclusions based on the temporal mean Nusselt number were described. First, the LES data provided an excellent level of accuracy compared to existing experimental data. Second, via high-order statistics used to discover the fluid characteristics near the second peak of the Nusselt number, the time variation of pressure distribution revealed the rebound of the main vortices close to the location of the secondary peak and permitted identification of the beginning of unstable separation. Grenson and Deniau (Citation2017) concluded that LES provided the most accurate predictions of the secondary maximum in the Nusselt number of the impinging jet when the hot inlet jet exits have fully developed profiles.
Similarly, Bader et al. (Citation2017) concluded that the viscous effects were significant for a narrow boundary layer flow over a solid body surface. The wall friction and heat transfer characteristics can affect laminar or turbulent flow conditions, causing a reverse transition or relaminarization from turbulent to laminar. Next, Dey et al. (Citation2018) investigated the flow with the pre-attachment region and found that the velocity profiles follow an exponential function. Huang et al. (Citation2018) studied numerical heat transfer with slot jet impingement by employing the combination turbulence model. The Kato-Launder model, the intermittency transition model, and the crossflow transition model were merged with the primarily original SST turbulence model. When compared to both experimental data and accessible numerical results from previous works, it was discovered that the proposed model performs well regarding various parameters.
In 2020, Pakhomov et al. numerically studied the effect of the pulse form on heat transfer in a pulsed impinging jet using the unsteady RANS model and second-moment closure. The study results found that the heat transfer at the stagnation point varies according to the pulse frequency and the Reynolds number. Singh et al. (Citation2020) investigated the fluid flow properties of a turbulent offset jet impinging on a wavy wall surface. The results provide that pressure near the reattachment point decreases and the magnitude of the decay in the normalized streamwise maximum velocity depends on the wavy surface's amplitude. Similarly, Zhang et al. (2020) investigated ultrafast cooling equipment using the self-excited pulsed nozzle. The results reveal that the self-excited pulsed jet can enhance the perturbations of the boundary layer and significantly increase the turbulence intensity of the plate surface.
1.3. Current and future interesting applications
According to the jet impingement phenomenon, heat transfer intensification methods are vital in many industrial applications (Chen & Chen, Citation2022; Steinberg et al., Citation2022; Ul Haq et al., Citation2022; Xing et al., Citation2022; Zhou et al., Citation2022). Regarding the importance of a wide range of applications, such as aerospace, automotive and semiconductor components, the following sections discuss the applications that require a fidelity standard of accuracy. Gao et al. (Citation2018) investigated the safety impact of gas jets on a jet blast deflector of aircraft engines that significantly interferes with the flight deck environment. This work confirmed that those predicted results from SST k-ω turbulence model was better for compressible viscous gas jet simulations. Zhou et al. (Citation2018) proposed a new jet impingement chip-scale cooling structure to improve silicon wafer manufacturing. This unique design was estimated to provide 70% higher cooling performance for pumping power and more uniform cooling. Radmard et al. (Citation2020) explored the heat transfer from directly manufactured micro pins undergoing jet impingement. Their study showed that the outlet port position significantly affected the hydraulic and thermal resistances. Rane and He (Citation2020) analyzed a two-phase turbine used for power generation in thermal power plants based on a Ranz-Marshall heat transfer correlation and concluded there was good agreement in the BNL nozzle.
However, as late as 2021, the aero-acoustic properties of dual impinging jets remained less understood. Mehta et al. (Citation2021) adopted the shadowgraph technique to investigate the aero-acoustic properties of dual impinging jets. Their findings indicated that changing the nozzle spacing affected the fountain flow strength. The aero-acoustic broadband and discrete amplitude tones were also affected by changing the spacing, with the overall sound pressure level being robust to the gap between nozzles. Erasmus et al. (Citation2021) studied the application of impinging jets in thermal receivers and bayonet tube heat exchangers for concentrating solar power using the transition SST RANS turbulence model and proposed an empirical correlation of the pressure loss factor. Their numerical investigation clearly showed that the dominating total pressure loss occurred when there was rapid jet expansion. Kura et al. (Citation2021) applied a jet impingement on a non-planar surface for a wide range of geometries designed for heat exchangers. The numerical simulations were carried out using an advanced ζ-f turbulence model implemented in OpenFOAM. The results suggested that the geometrical shape influenced the secondary peak in the Nusselt number distribution. Cademartori et al. (Citation2021) employed CFD tools to demonstrate a novel cooling technique through slot jet impingement and compared those of predicted results with an available vertical galvanizing industrial line primarily intended using a round jet shape. From their investigation, slot jet impingement was made more efficient and favorable by increasing the jet wall distance and thus improving the uniformity of transversal temperature distributions. Most recently, Das et al. (Citation2022) applied jet impingement on the absorber plate to enhance the effectiveness of a solar air heater. Their results showed that superior improvement of elongated streamlines and higher turbulent kinetic energy could improve the heat transfer performance.
The above literature review demonstrates that the reverse transition from turbulent to laminar flow has been studied experimentally by many investigators since it not only has a very complex nature but also offers fundamental knowledge into how to control the turbulence mechanism, as well as expand this capability from academic research to practical industrial work. Additionally, it is not easy to understand the flow mechanism in any field with a general turbulence model. Therefore, to increase the understanding of the jet impingement behavior due to the variation of the anisotropic turbulence mechanism, the current study presents all the hidden turbulence equations in the calculation framework and focuses on a new turbulence model that is implemented in commercial software by including separation-induced transition correction such as v2-f, and Reynolds Stress based on -approach and the
-approach. Furthermore, these numerical investigation results are compared with previous experimental results (Baughn et al., Citation1991; Cooper et al., Citation1993).
2. Mathematical and numerical modeling
This section provides turbulence model descriptions considered in the current study. Initially the v2-f model is proposed, which includes the effect of an anisotropy turbulent structure and changes to appropriate velocity scale near walls. Then, the Reynold stress model (RSM) model is presented, which considers the effects of streamline curvature and rapid changes in strain rate tensors. In addition, the RSM in this study is considered using -based and
-based approaches, because both models are still questionable regarding their capability in an impingement jet numerical study. All the equation systems are shown in the next section.
2.1. The v2-f turbulence model
The v2-f model is a RANS-based turbulence model which does not require a wall function. It includes additional terms which take account of near-wall anisotropy turbulence and nonlocal pressure-strain in the model. For the standard k – model, the primitive velocity and time scales are dependent on the turbulent kinetic energy,
and
respectively. Since those of previous scales failed to predict wall-bounded flow applications, the v2-f model applies the velocity scale
instead of
for an improved scale near walls, which can match with the boundary condition for the normal component. As a result, the v2-f model has an advantage over the previous models because it can provide proper scaling in representing the damping of turbulent transport close to the wall.
The v2-f model contains four transport equations. Clearly, both the turbulence kinetic energy and the turbulence dissipation equations are employed with those of the k- model but then they use different eddy viscosity assumptions and another turbulence time scale. Also, the
transport equation and the elliptic-relaxation equation are included. The effects of the anisotropic are included and the Helmholtz elliptic equation are solved to define the elliptic relaxation function. For the computational performance improvement of the v2-f model, a variant of this eddy-viscosity model is derived when the change in variables is introduced. This turbulence variable can be regarded as the ratio of the two-time scales: scalar
(isotropic) and lateral
(anisotropic). More information on the v2-f model can be found in ANSYS Inc. (Citation2021)
2.1.1 Transport equation for the v2-f model
The turbulence kinetic energy, turbulence dissipation rate, velocity variance scale, and elliptic relaxation function are derived as follows:
(1)
(1)
(2)
(2)
(3)
(3)
(4)
(4) where
,
, and
. The turbulent length (L) and time (T) scales are expressed as follow:
(5)
(5)
(6)
(6)
(7)
(7)
(8)
(8)
(9)
(9)
(10)
(10)
The variable is solved from the elliptic relaxation equation (Equation4
(4)
(4) ). Here, the model utilizes an elliptic operator to calculate a term comparable to the pressure-strain correlation of the RSM. Ellipticity is described by a modified Helmholtz operator, introducing wall effects via a linear differential equation (ANSYS Inc., Citation2021).
2.2. The reynolds stress model
The Reynolds stress model (RSM) is the most decorative RANS turbulence model. In particular, the model of RSM has higher-level turbulence closures and represents a complete classical turbulence model. The method of closure applied is usually called second-order closure. In the RSM, the stress tensor components of the Reynolds stress are directly determined while the eddy viscosity assumption is avoided. This model relies on the exact Reynolds stress transport equation by couple solving between the transport equations of Reynolds stresses and the dissipation rate; an additional set of seven transport equations are required for complex three-dimensional problems. The advantage of the RSM is that it includes the effects from swirling motion, rotational motion, rapid change in strain rate, and streamline curvature, so that it could be expected to predict jet impingement behavior more accurately. In particular, the -based (linear and quadratic pressure-strain model) and
-based (stress-omega and stress-BSL model) RSMs are used to model the pressure strain and dissipation rate terms, respectively. They are accountable for compromising the accurate prediction of RSM results.
2.2.1 Reynolds stress transport equations
The complete exact transport equations for the transport of the Reynolds stress, , are written as:
(11)
(11) In equation (Equation11
(11)
(11) ),
,
, and
do not require additional modeling. However,
,
, and
need additional modeled equations to describe the assumption to close the equation set.
2.2.2 Modeling the dissipation rate
The scalar dissipation rate computed from the steady transport equation is:
(12)
(12) For the RSM based on the
-equation, the dissipation tensor
is written as:
(13)
(13)
However, where the RSM is coupled based on the or
equation, the dissipation tensor is modeled as:
(14)
(14) where
is computed from modeling the pressure-strain term as described in the next section. For the stress-omega model and stress-
model, the specific dissipation rate is calculated similarly as the standard k-
model and the baseline k-
model, respectively (ANSYS Inc., Citation2021).
2.2.3 Modeling the turbulence kinetic energy
The trace of the Reynolds stress tensor is introduced to model the turbulence kinetic energy (TKE) term as follow:
(15)
(15) For the
-equation based RSMs, a transport equation for the TKE is solved to obtain boundary conditions for the Reynolds stresses using the following model equation:
(16)
(16)
2.2.4 Modeling the pressure-strain term
In this study, we use two -based RSMs (linear and quadratic pressure-strain models) and
-based RSMs (stress-omega and stress-
models), respectively. The elaborate equation system details are as follows:
2.2.4.1. Linear Pressure-Strain Model (RSM-LPS)
The classical approach to modeling the pressure-strain term () uses the following decomposition according to proposals by Gibson and Launder (Citation1978) and Launder (Citation1989):
(17)
(17) The slow pressure-strain term,
, is modeled by:
(18)
(18)
The rapid pressure-strain term, , is modeled by:
(19)
(19) The wall-reflection term,
, represents the redistribution of near wall-normal stresses. It tends to dampen the normal stress perpendicular to the wall while enhancing the stresses parallel to the wall. The calculation model is:
(20)
(20)
In equation (Equation20(20)
(20) ),
is the
unit normal vector in each component,
is the normal distance to the wall, and
.
2.2.4.2. Quadratic Pressure-Strain Model (RSM-QPS)
This model has been revealed to provide advanced performance for a range of basic shear flows, including plane strain, rotating plane shear, and asymmetrical expansion/contraction. It leads to produce a high level of accuracy for complex engineering flows, especially in streamline curvature, as proposed by Speziale et al. (Citation1991) and written as:
(21)
(21) where
represent the Reynolds stress anisotropy tensor and
is the mean rate of rotation tensor defined by:
(22)
(22)
(23)
(23)
2.2.4.3. Stress-Omega Model (RSM-SO)
The stress-omega model is a stress transport model based on the ω-equations and the LRR model. It resembles the κ−ω model due to its excellent predictions for a wide range of turbulent flows requiring wall reflection treatments. The relation of pressure-strain can be written by:
(24)
(24) Therefore,
(25)
(25) where
,
,
,
,
,
, and
are defined by:
(26)
(26)
(27)
(27)
(28)
(28)
(29)
(29)
(30)
(30)
(31)
(31)
(32)
(32)
Where
(33)
(33)
(34)
(34)
2.2.4.4. Stress-BSL Model (RSM-S-BSL)
More specifically, the stress-BSL model is the -based Reynold stress model, as presented by Speziale et al. (Citation1991), which solves the scale equation from the baseline (BSL) k-
model and adopts a blending function for the transport of turbulent dissipation in the same way as the SST k-
turbulence model. It uses the same relation in equation (Equation25
(25)
(25) ) for the pressure-strain correlation in the Reynolds stress equations as the stress-omega model. The coefficient
is equal to
.
2.2.5 Modeling turbulent diffusive transport
For the -based RSM,
can be modeled by a scalar turbulent diffusivity equation:
(35)
(35) The turbulent viscosity,
, for the
-based model is computed using:
(36)
(36)
The -equation (stress-omega) and BSL-equation (stress-BSL) calculated from:
(37)
(37) In equation (Equation35
(35)
(35) ), the model constant is
. However, for the
-based approach, the stress-omega model sets
and
, whereas the stress-BSL models use the blending function
from:
(38)
(38)
(39)
(39)
(40)
(40)
(41)
(41) where
represents the positive portion of the cross-diffusion term and
is the distance to the next surface.
3. Computational investigation
The summary of the computational domain is shown in Figure . The configuration includes the circular orifice and the target constant heat flux plate ( = 300
). The jet Reynolds number of 23,000 based on the exit nozzle diameter is ejected out from a nozzle. According to previous experimental literature reviews (Baughn et al., Citation1991; Fenot et al., Citation2005; Hee Lee et al., Citation2002; Attalla, Citation2015), the golden ratio of H/D = 2 is capable of clearly generating the second peak of the Nusselt number, which is the focus parameter for the investigation of the turbulence model in the current paper, where ANSYS Fluent 2020 R1 is used. The inlet velocity profiles are fully developed flow, with no-slip and adiabatic wall boundary conditions applied at the pipe surfaces (Grenson & Deniau, Citation2017).
3.1. Grid independent test
The computational grid in Figure was generated using the ANSYS meshing software with the multizone method. The rest of the computational grid uses a pure structural grid. The hot flat plate surface was created with micrometer first layer cells and 140 circumferences of grid points to obtain
(for boundary layer relaminarization or separation recommended by ANSYS 2020R1, 2020). The grid-independent analysis was carried out over five successively finer grids, as shown in Table . All grids shared the same stretching ratio of 1.10 for the structural grid while loosely refined at far outlet boundaries where to save computational cost, the gradients of the flow variables were expected to be negligible.
Table 1. Computational grid.
The comparison was performed using the Nusselt number on the grid size, with various Nusselt profiles plotted against the ratio, as shown in Figure , compared to previous publication (Baughn et al., Citation1991). According to the findings, the Nusselt profile for Grid-5 was consistent with the experiment. Another important finding in that study was that the grid distribution in the radial direction must be dented sufficiently to obtain the second peak of the Nusselt number prediction. As a result, the current study will investigate all cases using the same grid number.
3.2 Numerical setting and initial conditions
The initialization of the incoming jet into the domain required velocity profiles, the TKE, and the specific dissipation rate at the inflow boundary, solved from a long pipe until fully developed. This condition is matched with those of available experiment. Furthermore, the boundary conditions and numerical settings are described in Table .
Inlet: inlet boundary with fully developed flow characteristics, such as velocity, TKE, and the specific dissipation rate
Top auxiliary inlet: pressure inlet with the inflow direction
Outlet: pressure: outlet boundary with a constant reference pressure
Pipe wall: nonslip adiabatic wall
Heat bottom wall: no-slip wall specified constant heat flux,
= 300 W/m2
Table 2. Numerical setup.
4. Results
This section is divided into three sections. The first part shows the predicted results of the average Nusselt number on a flat plate with a constant heat flux thermal boundary condition. The available experimental data from Baughn et al. (Citation1991) are also plotted for comparison. The second part demonstrates the turbulence quantities versus the axial vertical distance. Finally, the contour plots of the Nusselt number and velocity profiles near the transition wall zones are shown.
4.1. Secondary peak occurrence due to variation in anisotropic turbulence model
To capture the secondary peak phenomenon, a simulation with a Reynolds number of 23,000 is performed and tested with common turbulence models such as v2-f, RSM-LPS, RSM-QPS, RSM-SO, and RSM-S-BSL. These turbulence model variations are applied with the constant heat flux at the bottom plate. The numerical investigation, shown in Figure , describes the distribution of the local Nusselt number magnitude against the ratio. The secondary peak of the Nusselt number magnitude grows in the distribution at
= 2.0 and a value of 110. It can be deduced that all anisotropic models, except for the v2-f turbulence model, fail to predict this second peak. Although the RSM turbulence model is more detailed than those of the general turbulence models (and even though it includes all Reynolds stress transport for all directions), it still cannot capture relaminarization due to a lack of separation-induced transition correction. However, the second peak Nusselt number in RSM-LPS and RSM-QPS can still be observed. For the pressure-strain terms in equations (Equation17
(17)
(17) ) and (Equation21
(21)
(21) ), they are
-based Reynolds stress models with a slow pressure-strain term (also known as the return-to-isotropy), a rapid pressure-strain term, and a wall-reflection term. This relationship may not precisely explain the direct effect on the second peak, but it is expected to calculate the trend in the magnitude of Nusselt number. As shown in equations (Equation21
(21)
(21) )–(Equation23
(23)
(23) ), RSM-QPS has more complex equations than RSM-LPS, most notably the Reynolds stress anisotropy tensor, mean strain rate, and rotation rate. These equations are related to the logarithmic region of a turbulent boundary layer, and we may be able to obtain more accurate results if we improve this calculation algorithm or develop a new unique correlation approach in the boundary layer region. Finally, we still believe that the RSM of these models can help future researchers improve their performance.
The v2-f model is like the standard k-ε model; however, it includes near-wall turbulence anisotropy and nonlocal pressure-strain effects. It is a general low-Reynolds number turbulence model valid up to solid walls and thus does not require wall functions. Although the model was designed to simulate attached or mildly separated boundary layers, it also accurately simulates separation-dominated flows. The v2-f model is distinguished by its use of the velocity scale rather than TKE to calculate eddy viscosity, defined as the velocity fluctuation normal to the streamlines by the variable , and is the solution to the elliptic relaxation equation. In this case, the v2-f model uses an elliptical operator to compute a term like the RSM’s pressure strain correlation. Ellipticity is defined by a modified Helmholtz operator that introduces wall effects via a linear differential equation. From the above explanation, it is advantageous to discover that the near-wall relation has more chance for state-of-the-art improvement to separation and relaminarization behavior.
4.2. Velocity profiles
The axial velocity component profiles of the impinging jet (onto a constant hot plate H/D = 2.0, Re = 23,000) are shown in Figure and compared to experimental data from Cooper (1993). The results show that the effect of near-wall velocity was consistent with all turbulence models in the range of 0.5 1.0. Furthermore, the error increases significantly as the
ratio increases. Interestingly, Figure 6(c) at
= 1.5 depicts the velocity profiles at the local Nusselt number minimum in the negative first peak that all the turbulence models are not capable of predicting, especially RSM. When we look more closely at the RSM turbulence model, it is discovered that the
-based models could predict a slight trend of local Nusselt second peak trends faster than experimental data. Comparing the resulting velocity to the experiment, the RSM
-based (RSM-LPS, RSM-QPS) model had consistently less error than the
-based (RSM-SO, RSM-S-BSL) approach. As a result, these findings clearly show that the prediction of the second peak may be related to the
-transport equation; it is highly recommended that to improve the accuracy of the RSM inner sublayer, we need some mathematical modifications, such as an inner new
-transport equation or a new correlation of the internal sublayer (Panda, Citation2019). In addition, the stress-omega model, which is
-based stress-transport model related to the
-transport equations and does not require any wall reflection treatments. The stress-
model eliminates the free-stream sensitivity that is examined by the baseline (BSL) model. The near-wall model equation was integrated into both models. As a result, it may be difficult to predict the occurrence of the Nusselt second peak behavior. In contrast, in Figure 6(e–f), the velocity profiles negatively deviate more from the RSM, and v2-f is overpredicted while moving away from the jet core region. We suspect that the energy dissipation of turbulence is relatively high. However, considering the increased
in vertical distance, v2-f still predicted axial velocity well compared to the experiment. It can be concluded that v2-f and all RSM turbulence models are not practical for predicting inside near-wall boundary velocity, although v2-f has improved near-wall turbulence anisotropy and nonlocal pressure-strain effects. Nevertheless, the RSM has also modified the wall function but is still not as accurate as we expected. This finding is like the numerical results of Zuckerman et al. (2006)
Figure 6. Comparisons of simulated and measured mean velocity with H/D = 2 and Re = 23,000: (a) r/D = 0.5, (b) r/D = 1.0, (c) r/D = 1.5, (d) r/D = 2.0, (e) r/D = 2.5, and (f) r/D = 3.0.

When = 2.0 is indicated as the second peak of the local Nusselt number described in Figure 6(d), we discovered that the v2-f model accurately predicts velocity profiles in all regimes. However, all RSM turbulence models fail to predict the entire range. In the detailed analyses, the
-based approach can detect the second local Nusselt number faster than experimental data, but it has more error in the velocity profiles than the
-based approach. We can conclude that the faster second peak causes increasingly faster turbulent energy dissipation, resulting in low momentum in the entire region. The v2-f model uses a velocity variance scale and an elliptic relaxation function to detect the relaminarization behavior in the region of separation-induced relaminarization.
4.3. Turbulence intensity and turbulence kinetic energy profiles
Since the flow of the jet impingement is anisotropic, in Figure 7(a–d), the mentioned parameters are shown for turbulence intensity (TI) and turbulence kinetic energy (TKE) for the cases = 2.0 and
= 1.5 and 2.0. Physically, the TI or unresolved unsteadiness is calculated from the mean square fluctuation components divided by the mean absolute velocity, while TKE is characterized by measured root-mean-square velocity fluctuations or can be calculated based on the closure method in the RANS equations. Generally, TKE can be produced by fluid shear, friction, or integral scale and then transferred down the turbulence energy cascade and dissipated by viscous forces. This production, transport, and dissipation process can be expressed as separation-induced relaminarization (Ting, Citation2016). Therefore, if we find that the Nusselt number and velocity profiles of v2-f can produce the best results compared to experimental data, we can assume that in this study, v2-f is the best turbulence model even before comparison with the RSM; hence, the results in this section will focus on v2-f computational data to represent the baseline of accuracy (Issac et al. (Citation2020); Zuckerman et al (2006)).
Figure 7. Turbulence intensity and turbulence kinetic energy distributions before and after the second peak: (a) TI at r/D = 1.5, (b) TI at r/D = 2.0, (c) TKE at r/D = 1.5, and (d) TKE at r/D = 2.0.

Considering all RSM turbulence models in terms of TI and TKE estimation the RSM-LPS and RSM-QPS values are nearly identical across all regions, RSM-S-BSL values are in the same order if , and RSM-SO is a significant blunder across all regimes. Despite these challenges, when we move from the jet core to the second peak region (
= 2.0), we suspect a very complex mechanism due to relaminarization behavior is occurring. However, we believe that the Nusselt number and average velocity profile prediction results from v2-f model provides the best mapping with an experiment in the relaminarization region. Hence, we hypothesize that TI and TKE must be good enough to be a key for this study in terms of analyses for another turbulence model. When considered close to the wall, the
-based method is superior to the
-based method. As a result, we can infer that the RSM
-based approach is not suitable for separation-induced relaminarization processes; if it is used in the future, more modification will be needed to improve the near-wall region. However, the TI is often referred to as the turbulence level, which is correlated with the TKE. Here, we can show that the improvement may modify the TKE equation, TI equation, or a related equation (Khalaji et al., Citation2016; Huang et al., Citation2018; Zhang et al., Citation2019).
4.4 Velocity and nusselt contour
The predicted velocity field with H/D = 2.0 is depicted in Figure . Once the grid is independent and fine enough with , the velocity vector described in Figure 8(a) shows parabolic profiles in the inner layer of the boundary layer. Next, for the local Nusselt number contour of v2-f, as depicted in Figure 8(b), given the details at the center of jet impingement with the so-called stagnation point, it increases the local Nusselt number and decreases monotonically at r/D = 2.0, which produces a simulated result in the second peak. These results are consistent with the mechanism described in other research (Zhang et al., Citation2019; Ekkad & Singh, Citation2021), giving us confidence that all our settings are correct and that we have obtained numerical fidelity results. The region of highest TKE immediately adjacent to the wall corresponds to the part of the secondary peak in the Nusselt number; so, this peak can be attributed to the development of turbulent flow in the wall jet (Zuckerman et al., 2006). Figure 8(c) shows a region where the deceleration of the flow and decay of the wall-jet boundary layer caused a reduction in turbulence and a decrease in the Nusselt number. The other anisotropic turbulence models could not predict the secondary peak because they produced different (and likely incorrect) velocity fields, directly influencing the thermal energy transfer calculations. The four-equation v2-f model resolves the complete boundary layer and includes an elliptic relaxation function, which tracks the damping effects of walls on the velocity variance scale. This finding emphasizes that if we need greater accuracy regarding the Nusselt number, we should not only improve the elaborate anisotropy transport equation model but also the near-wall modeling must be considered, such as the
-based approach and the
-based approach.
4.5. The effect of the Reynolds Number
For comparison, the results shown in Figure focus only on the v2-f turbulence model to investigate the effect of the Reynolds number. The formation of the secondary peak of the Nusselt number can be captured by the v2-f turbulence model for all Reynolds numbers in this study. The location of the secondary peak removes downward in the radial direction as the Reynolds number increases. Based on these findings, we hypothesize that the secondary peak is caused by the spreading wall jet’s transition from a laminar to a turbulent boundary layer flow. Furthermore, as the Reynolds number increases, the effect of the toroidal flow phenomenon, which forms in the shear region around the circumference of the jet, striking the impinging plate appears to be enhanced (Issac et al., Citation2020).
5. Conclusions
Numerical study of jet impingement using a variety of relevant anisotropic turbulence models were investigated and compared those of results with available experimental data. Surprisingly, better agreement with experimental data was obtained with the turbulence models based on some near-wall turbulence anisotropy and nonlocal pressure-strain effects. The advantage of the v2-f model is using the velocity scale instead of the turbulence kinetic energy to evaluate the eddy viscosity. The anisotropic wall effects are modeled through the elliptic relaxation function by solving an additional elliptic equation of the Helmholtz type. Consequently, the secondary peak in the Nusselt number dependency on a radius could be predicted with reasonable accuracy compared to the second-order closure Reynolds stress turbulence models. The secondary peak location was found at r/D = 2.05 with the experiment and produced similar results to other study. At H/D = 2.0, the golden ratio that clearly shows the secondary peak of the Nusselt number decreases in the radial direction between the stagnation point; at a radial distance of approximately r/D = 2.05, a second peak is observed. Initially, the first peak of Nusselt number prediction is at r/D = 0.5 due to the change in the radial flow velocity as it travels radially downstream from the stagnation point. When the jet deflects after hitting the surface, it accelerates rapidly but at a greater distance, as the spreading of the jet causes its velocity to diminish. Finally, we found that the transport equation based on v2-f produces better results for all flow characteristics compared to the RSM in all approaches. The critical point for turbulence modeling improvement is identified by focusing on the near-wall transport theorem. However, this modification or improvement is challenging and may require considerably more relevant referenced tests.
Disclosure statement
No potential conflict of interest was reported by the author(s).
References
- Aillaud, P., Duchaine, F., Gicquel, L. Y. M., & Didorally, S. (2016). Secondary peak in the Nusselt number distribution of impinging jet flows: A phenomenological analysis. Physics of Fluids, 28(9), https://doi.org/10.1063/1.4963687
- ANSYS Inc. (2021). ANSYS Fluent V2F Turbulence model manual. 15317(January).
- Attalla, M. (2015). Stagnation region heat transfer for circular jets impinging on a flat plate. Experimental Heat Transfer, 28(2), 139–155. https://doi.org/10.1080/08916152.2013.829134
- Bader, P., Pieringer, P., & Sanz, W. (2017). On the capability of transition models to predict relaminarization. 12th European Conference on Turbomachinery Fluid Dynamics and Thermodynamics, ETC 2017. https://doi.org/10.29008/etc2017-328
- Bader, P., & Sanz, W. (2017). On boundary layer relaminarization in an highly accelerated high pressure turbine stator flow. Proceedings of the ASME Turbo Expo, 2B-2017, V02BT41A009. https://doi.org/10.1115/GT2017-63296.
- Baughn, J. W., Hechanova, A. E., & Yan, X. (1991). An experimental study of entrainment effects on the heat transfer from a flat surface to a heated circular impinging jet. Journal of Heat Transfer, 113(4), 1023–1025. https://doi.org/10.1115/1.2911197
- Cademartori, S., Cravero, C., Marini, M., & Marsano, D. (2021). CFD simulation of the slot jet impingement heat transfer process and application to a temperature control system for galvanizing line of metal band. Applied Sciences, 11(3), 1149–1123. https://doi.org/10.3390/app11031149
- Chen, K., Xu, R., & Jiang, P. (2018). Experimental study of jet impingement cooling with carbon dioxide at supercritical pressures on micro structured surfaces. The Journal of Supercritical Fluids, 139, 45–52. https://doi.org/10.1016/j.supflu.2018.05.002
- Chen, Y. J., & Chen, Z. S. (2022). A prediction model of wall shear stress for ultra-high-pressure water-jet nozzle based on hybrid BP neural network. Engineering Applications of Computational Fluid Mechanics, 16(1), 1902–1920. https://doi.org/10.1080/19942060.2022.2123404
- Cooper, D., Jackson, D. C., Launder, B. E., & Liao, G. X. (1993). Impinging jet studies for turbulence model assessment—I. Flow-field experiments. International Journal of Heat and Mass Transfer, 36(10), 2675–2684. https://doi.org/10.1016/S0017-9310(05)80204-2
- Cousteix, J. (2003). Aircraft aerodynamic boundary layers. In Encyclopedia of physical science and technology (pp. 301–317). Elsevier. https://doi.org/10.1016/b0-12-227410-5/00906-6
- Das, S., Biswas, A., & Das, B. (2022). Numerical analysis of a solar Air heater With Jet impingement—comparison of performance between Jet designs. Journal of Solar Energy Engineering, 144(1), https://doi.org/10.1115/1.4051478
- Dewan, A., Dutta, R., & Srinivasan, B. (2012). Recent trends in computation of turbulent jet impingement heat transfer. Heat Transfer Engineering, 33(4–5), 447–460. https://doi.org/10.1080/01457632.2012.614154
- Dey, S., Ravi Kishore, G., Castro-Orgaz, O., & Ali, S. Z. (2018). Reynolds stress in submerged turbulent plane offset jets: Mathematical model. Journal of Engineering Mechanics, 144(6), 06018001. https://doi.org/10.1061/(ASCE)EM.1943-7889.0001456
- Ekkad, S. V., & Singh, P. (2021). A modern review on jet impingement heat transfer methods. Journal of Heat Transfer, 143(6), https://doi.org/10.1115/1.4049496
- Erasmus, D. J., Lubkoll, M., & von Backström, T. W. (2021). Jet impingement heat transfer within a hemisphere. Heat and Mass Transfer, 57(6), 931–948. https://doi.org/10.1007/s00231-020-02977-9
- Fenot, M., Vullierme, J.-J., & Dorignac, E. (2005). Local heat transfer due to several configurations of circular air jets impinging on a flat plate with and without semi-confinement. International Journal of Thermal Sciences, 44(7), 665–675. https://doi.org/10.1016/j.ijthermalsci.2004.12.002
- Gao, F. D., Wang, D. X., Wang, H. D., & Jia, M. M. (2018). Numerical analysis and verification of the gas jet from aircraft engines impacting a jet blast deflector. Chinese Journal of Mechanical Engineering, 31(1), https://doi.org/10.1186/s10033-018-0285-7
- Gibson, M. M., & Launder, B. E. (1978). Ground effects on pressure fluctuations in the atmospheric boundary layer. Journal of Fluid Mechanics, 86(3), 491–511. https://doi.org/10.1017/S0022112078001251
- Grenson, P., & Deniau, H. (2017). Large-Eddy simulation of an impinging heated jet for a small nozzle-to-plate distance and high reynolds number. International Journal of Heat and Fluid Flow, 68, 348–363. https://doi.org/10.1016/j.ijheatfluidflow.2017.09.014
- Hee Lee, D., Youl Won, S., Taek Kim, Y., & Suk Chung, Y. (2002). Turbulent heat transfer from a flat surface to a swirling round impinging jet. International Journal of Heat and Mass Transfer, 45(1), 223–227. https://doi.org/10.1016/S0017-9310(01)00135-1
- Huang, H., Sun, T., Zhang, G., Sun, L., & Zong, Z. (2018). Modeling and computation of turbulent slot jet impingement heat transfer using RANS method with special emphasis on the developed SST turbulence model. International Journal of Heat and Mass Transfer, 126, 589–602. https://doi.org/10.1016/j.ijheatmasstransfer.2018.05.121
- Ichimiya, M. (1999). The effect of a single roughness element on a flat plate boundary layer transition. In Engineering turbulence modelling and experiments 4 (pp. 597–606). Elsevier. https://doi.org/10.1016/B978-008043328-8/50057-6
- Issac, J., Singh, D., & Kango, S. (2020). Experimental and numerical investigation of heat transfer characteristics of jet impingement on a flat plate. Heat and Mass Transfer, 56(2), 531–546. https://doi.org/10.1007/s00231-019-02724-9
- Kalifa, R. B., Habli, S., Saïd, N. M., Bournot, H., & Palec, G. L. (2016). The effect of coflows on a turbulent jet impacting on a plate. Applied Mathematical Modelling, 40(11–12), 5942–5963. https://doi.org/10.1016/j.apm.2016.01.037
- Khalaji, E., Nazari, M. R., & Seifi, Z. (2016). 2D numerical simulation of impinging jet to the flat surface by k−ω−−f turbulence model. Heat and Mass Transfer, 52(1), 127–140. https://doi.org/10.1007/s00231-015-1688-y
- Kura, T., Fornalik-Wajs, E., Wajs, J., & Kenjeres, S. (2021). Curved surface minijet impingement phenomena analysed with ζ-f turbulence model. Energies, 14(7), https://doi.org/10.3390/en14071846
- Lar-Kermani, E., Roohi, E., & Porte-Agel, F. (2018). Evaluating the modulated gradient model in large eddy simulation of channel flow with OpenFOAM. Journal of Turbulence, 19(7), 600–620. https://doi.org/10.1080/14685248.2018.1483078
- Launder, B. E. (1989). Second-moment closure and its use in modelling turbulent industrial flows. International Journal for Numerical Methods in Fluids, 9(8), 963–985. https://doi.org/10.1002/fld.1650090806
- Leocadio, H., & Passos, J. C. (2021). Experimental investigation of heat transfer characteristics during water jet impingement cooling of a high-temperature steel surface. Ironmaking & Steelmaking, 48(7), 819–832. https://doi.org/10.1080/03019233.2021.1872467
- Liu, B., Pan, Y., & Ma, F. (2020). Pulse pressure loading and erosion pattern of cavitating jet. Engineering Applications of Computational Fluid Mechanics, 14(1), 136–150. https://doi.org/10.1080/19942060.2019.1695675
- Mehta, Y., Natarajan, K., Gustavsson, J., & Kumar, R. (2021). An experimental investigation into the effect of nozzle spacing in supersonic twin jets. AIAA Scitech 2021 Forum, 1–19. https://doi.org/10.2514/6.2021-1293.
- Pakhomov, M. A., & Terekhov, V. I. (2020). RANS simulation of the effect of pulse form on fluid flow and convective heat transfer in an intermittent round jet impingement. Energies, 13(15), https://doi.org/10.3390/en13154025
- Panda, J. P. (2019). A review of pressure strain correlation modeling for reynolds stress models. Proceedings of the Institution of Mechanical Engineers, Part C: Journal of Mechanical Engineering Science, 234(8), 1528–1544. https://doi.org/10.1177/0954406219893397
- Radmard, V., Hadad, Y., Azizi, A., Rangarajan, S., Hoang, C. H., Arvin, C., Sikka, K., Schiffres, S. N., & Sammakia, B. (2020). Direct Micro-Pin Jet Impingement Cooling for High Heat Flux Applications. 36th Annual Semiconductor Thermal Measurement, Modeling and Management Symposium, SEMI-THERM 2020 - Proceedings, 91–96. https://doi.org/10.23919/SEMI-THERM50369.2020.9142864.
- Rane, S., & He, L. (2020). CFD analysis of flashing flow in two-phase geothermal turbine design. Journal of Computational Design and Engineering, 7(2), 238–250. https://doi.org/10.1093/JCDE/QWAA020
- Shademan, M., Balachandar, R., Roussinova, V., & Barron, R. (2016). Round impinging jets with relatively large stand-off distance. Physics of Fluids, 28(7), https://doi.org/10.1063/1.4955167
- Singh, T. P., Kumar, A., & Satapathy, A. K. (2020). Fluid flow analysis of a turbulent offset jet impinging on a wavy wall surface. Proceedings of the Institution of Mechanical Engineers, Part C: Journal of Mechanical Engineering Science, 234(2), 544–563. https://doi.org/10.1177/0954406219880209
- Speziale, C. G., Sarkar, S., & Gatski, T. B. (1991). Modelling the pressure–strain correlation of turbulence: An invariant dynamical systems approach. Journal of Fluid Mechanics, 227, 245–272. https://doi.org/10.1017/S0022112091000101
- Steinberg, C., Liu, M., & Hung, D. L. S. (2022). A combined experimental–numerical study towards the elucidation of spray–wall interaction on step geometries. Engineering Applications of Computational Fluid Mechanics, 16(1), 1866–1882. https://doi.org/10.1080/19942060.2022.2098828
- Ting, D. D. S.-K. (2016). Turbulence Scales. Basics of Engineering Turbulence, 69–98. https://doi.org/10.1016/B978-0-12-803970-0.00004-0
- Ul Haq, U., Ahmed, A., Mustansar, Z., Shaukat, A., Cukovic, S., Nadeem, F., Talay, S., Iqbal Khan, M. J., & Margetts, L. (2022). Computational modeling and simulation of stenosis of the cerebral aqueduct due to brain tumor. Engineering Applications of Computational Fluid Mechanics, 16(1), 1018–1030. https://doi.org/10.1080/19942060.2022.2056511
- Xing, C., Le, G., & Deng, H. (2022). Numerical study on jet noise suppression with water injection during one-nozzle launch vehicle lift-off. Engineering Applications of Computational Fluid Mechanics, 16(1), 1173–1194. https://doi.org/10.1080/19942060.2022.2072953
- Zahiri, A. P., & Roohi, E. (2019). Anisotropic minimum-dissipation (AMD) subgrid-scale model implemented in OpenFOAM: Verification and assessment in single-phase and multi-phase flows. Computers & Fluids, 180(C), 190–205. https://doi.org/10.1016/j.compfluid.2018.12.011
- Zahiri, A. P., & Roohi, E. (2021). Assessment of anisotropic minimum-dissipation (AMD) subgrid-scale model: Gently-curved backward-facing step flow. International Journal of Modern Physics C, 32(05), 2150068. https://doi.org/10.1142/S0129183121500686
- Zhang, F., & Wang, S. (2020). Numerical analysis for jet impingement and heat transfer Law of self-excited pulsed nozzle. ISIJ International, 60(11), 2485–2492. https://doi.org/10.2355/isijinternational.ISIJINT-2020-001
- Zhang, G., Huang, H., Sun, T., Li, N., Zhou, B., & Sun, Z. (2019). Analysis of the performance of a new developed shear stress transport model in a turbulent impinging jet flow. Physics of Fluids, 31(11), 115110. https://doi.org/10.1063/1.5118675
- Zhou, F., Jung, K. W., Fukuoka, Y., & Dede, E. M. (2018). Chip-scale cooling of power semiconductor devices: Fabrication of Jet impingement design. Proceedings of the International Symposium on Power Semiconductor Devices and ICs, 2018-May, 516–519. https://doi.org/10.1109/ISPSD.2018.8393716
- Zhou, Z., Bao, Y., Sun, P., & Li, Y. (2022). Cooling of rocket plume using aqueous jets during launching. Engineering Applications of Computational Fluid Mechanics, 16(1), 20–35. https://doi.org/10.1080/19942060.2021.2004926
- Zuckerman, N., & Lior, N. (2006). Jet impingement heat transfer: Physics, correlations, and numerical modeling. Advances in Heat Transfer, 39(C), 565–631. https://doi.org/10.1016/S0065-2717(06)39006-5