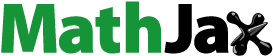
Abstract
Centrifugal pumps are widely used in various fields and have enormous energy-saving potential. In order to predict efficiency quickly and accurately, firstly, by establishing a hydraulic loss model for centrifugal pump impellers, the functional relationship between impeller hydraulic loss and hydraulic efficiency is constructed to establish the objective function. Secondly, calculate the main dimensions of the impeller using the velocity coefficient method and establish the objective function variables of the number of blades Z and outlet placement angle β2. And the velocity coefficient k0. Then, the method of mathematical statistics, namely response surface analysis, is used to solve the relationship between hydraulic efficiency and dependent variables within the range of variables, which plays the role of predicting hydraulic efficiency. Finally, the accuracy of the predictions is verified by numerical simulation. The results show that the hydraulic efficiency of a high specific speed centrifugal pump reaches its maximum at 1000m3/h operating conditions with a blade number of 3, a speed coefficient of 3.9 and an outlet angle of 30°. The study provides a new direction for the hydraulic design of high specific speed centrifugal pumps to achieve more accurate predictions of high specific speed centrifugal pump efficiency.
1. Introduction
According to information, pumps consume nearly 22% of the world's motor energy (Shankar et al., Citation2016). Centrifugal pumps account for more than 75% of the number of pumps and should be used in a wide range of fields, with huge potential for energy savings (Huang et al., Citation2020). High specific speed centrifugal pumps are used in a wide range of applications such as sewage treatment in town buildings, fire-fighting and water supply. Compared to other conventional pumps, high specific speed centrifugal pumps are characterized by a high delivery flow and a relatively low head in terms of performance. The structure is characterized by a large distortion of the impeller blade space and a wider impeller runner. As hydraulic machinery moves towards high speeds and high energy densities, reliability and efficiency have been the dominant criteria (Hergt, Citation1999).
Hence, improving the efficiency of the pump and reducing hydraulic losses has become a hot topic of research on centrifugal pumps today (Capurso et al., Citation2022). In the power industry, researchers have contributed to the reliability and efficiency of power systems through the optimization of centrifugal pumps (Wang et al., Citation2012; Pudjianto et al., Citation2013). In the water industry, Wu et al. (Citation2022) and Lin et al. (Citation2022) improved the energy efficiency of centrifugal pumps through the optimization of blades. Although their proposed design methods can definitely improve energy efficiency, they are all aimed at low specific speed centrifugal pumps. Perissinotto et al. (Citation2021) compared methods for visualizing the flow field within a centrifugal pump, analyzing their advantages and limitations and filling the gaps in the visualization of single-phase flows, gas–liquid and liquid–liquid mixtures. He et al. (Citation2021) visualized the flow characteristics of gas–liquid two-phase flow in a centrifugal pump and pointed out that the gas–liquid two-phase distribution in the impeller has a direct effect on the pressure rise and efficiency of the pump. However, the two academics above do not specifically suggest ways to improve pump efficiency. Wang et al. (Citation2022) proposed a novel approach to improve centrifugal pump performance with regard to the pump head, pump efficiency, and power. This approach guarantees a complicated multi-objective optimization problem and the results show that the centrifugal pump performances were improved. However, for the high flow rates of centrifugal pumps with high specific speed, this method can produce significant deviations. Wang et al. (Citation2017) proposed a method for optimizing the design of a typical multistage centrifugal pump based on an energy loss model and computational fluid dynamics (ELM/CFD), and systematically evaluated the interaction between different types of energy losses. Lin et al. (Citation2021a) and Lin et al. (Citation2021b) based on experiments and numerical simulations to predict the optimum efficiency point and energy loss of turbine centrifugal pumps for optimization. The proposed method can be intuitively used as a reference for the performance optimization and practical operation regulation of turbine centrifugal pump. With the development of information technology, there is a trend towards using machine learning to predict and optimize the efficiency of centrifugal pumps (Zhao et al., Citation2022a; Zhao et al., Citation2022b). However, the methods proposed by these scholars to improve pump efficiency are not applicable to the high specific speed centrifugal pumps in this paper.
Research on the hydraulic losses of centrifugal pumps is also abundant and the methods are varied. Nowadays, computational fluid dynamics tools have become more accurate in simulating the flow characteristics in pumps. Through numerical calculations, Shamsuddeen et al. (Citation2021) developed a multistage centrifugal pump for high head and high flow applications and found that substantial losses in head and efficiency were observed at the interface between the first stage volute and the second stage impeller, and an induced diffuser was installed at this location to reduce the circumferential velocity of the outflowing fluid to mitigate the losses. Napreenko and Lamtyugina (Citation2021) present a method for determining the unit hydraulic resistance of A/C systems, showing the advantages of computational fluid dynamics compared to traditional methods using hydraulic resistance coefficients, and give a ‘step-by-step’ example of calculating the unit hydraulic resistance of A/C systems in ANSYS CFX software. Zhou et al. (Citation2019) proposed an innovative method for rigorously describing the variation of pipeline hydraulic losses during multi-batch transport, based on a mixed-time representation that divides the scheduling horizon into two-time window levels, and developed a mixed integer linear programming (MILP) model for multi-product pipeline pump scheduling based on this method. Vasuthevan and Brümmer (Citation2018) carried out a multiphase flow simulation of a screw compressor using the ‘volume of fluid’ method to investigate the mechanism of hydraulic losses. It was found that blade tip velocity had a greater effect on hydraulic losses than on fluid leakage. Many scholars have used mathematical and statistical methods to predict losses and efficiencies of fluid machinery (Li et al., Citation2022). Some scholars have also optimized the hydraulic losses and efficiency of hydraulic turbine (Nishi et al., Citation2020; Šavar et al., Citation2009). These provide a wealth of data and references for the prediction of hydraulic losses and efficiency of centrifugal pumps.
For the current high specific speed centrifugal pump flow characteristics and hydraulic losses in the high flow area of the model is still lack of comprehensive understanding, further research and analysis is of great practical significance. In order to improve pump efficiency, this paper applies a mathematical and statistical method (CCD response surface method) to predict the efficiency of high specific speed centrifugal pumps in the high flow region. The response surface method has become a common optimization method in the fields of medical experiments (Trinh & Kang, Citation2010), chemistry (Norouzi et al., Citation2020; Anwar et al., Citation2018), engines (Yusri et al., Citation2018), and transportation (Li & Yang, Citation2018; Yıldız, Citation2020), which provides a good basis for the application of this paper to centrifugal pumps. Therefore, this paper takes a high specific speed centrifugal pump as the research object, and firstly, based on the hydraulic loss model, uses the loss extremum method to establish the structural parameters of the centrifugal pump impeller as a function of the hydraulic efficiency. The efficiency prediction function relationship for high specific speed centrifugal pumps in the high flow region is then constructed by a mathematical and statistical method (CCD response surface method). Finally, the relationship between pump losses and efficiency is studied for different pump parameters to provide a basis for selecting a high-performance pump under different conditions. This enriches the research direction of high specific speed centrifugal pumps and provides a reference for the safe operation of pumps in different environments and for efficiency prediction, including structural optimization.
2. Theory analysis
2.1. Centrifugal pump impeller hydraulic loss model
Hydraulic losses in centrifugal pumps are mainly generated in the impeller and volute. As the subject of this paper is a high specific speed centrifugal pump with different numbers of blades, the study concentrates on impeller hydraulic losses. The various hydraulic losses in the impeller of a centrifugal pump are as follows.:
Impeller inlet impingement loss:
(1)
(1) where, w1 is the component of relative velocity in the circumferential direction (m/s), k1 is the inlet impingement coefficient, g is the gravity acceleration (m/s2).
Frictional loss in impeller runners:
(2)
(2) where, Z is the number of blades, λ is the along-travel friction coefficient, k2 is the friction coefficient of impeller channel, wa is the average relative velocity (m/s), la is the hydraulic length of the runner (m), Da is the average diameter of the runner (m).
Impeller inlet fluid flow from axial to radial resulting in hydraulic loss:
(3)
(3) where, k3 is the the hydraulic coefficient generated by the axial to radial change of the liquid flow at the impeller inlet, De is the effective diameter of the impeller inlet (m), v is the speed of the impeller inlet without impingement loss (m/s), Qs is the flow rate without impingement loss:
(4)
(4) where, σ is the slip coefficient, ηv is the volumetric efficiency, D2 is the outer diameter of impeller (m), u2 is the impeller outlet velocity (m/s), b2 is the impeller outlet width (m), β2 is the blade outlet angle (°), ψ2 is the extrusion coefficient of impeller outlet, B is the square root of volute throat area(m).
Shrinkage loss in impeller runners:
(5)
(5) where, k4 is the contraction coefficient of impeller channel, w1 is the relative velocity at the impeller inlet (m/s), w2 is the relative velocity at the impeller outlet (m/s).
Impeller outlet hydraulic loss:
(6)
(6) where, k5 is the hydraulic coefficient of impeller outlet, vm2 is the average circumferential velocity at impeller outlet (m/s), vu2 is the circumferential component of impeller outlet velocity(m/s), vs is the average velocity of volute throat(m/s).
2.2. Efficiency function based on impeller hydraulic loss model
The improvement of the efficiency of centrifugal pumps is an important direction and objective of centrifugal pump research. In addition to improving the performance of the pump, it is essential to reduce pump losses if the pump is to have high efficiency. In this paper, the loss extremum method is used to establish the relationship between the structural parameters of the impeller of a centrifugal pump as a function of its hydraulic efficiency for the impeller hydraulic loss model. The parameters of the impeller are selected appropriately to achieve the highest hydraulic efficiency of the centrifugal pump without changing other design parameters. This provides a reference for the efficiency prediction of centrifugal pumps.
The loss extremes method is to achieve the minimum value of the target hydraulic loss through different combinations of several parameter variables under the condition that the design operating point requirements are met. The total hydraulic loss of the impeller can be obtained from the established hydraulic loss model equation as:
(7)
(7) Impeller hydraulic efficiency:
(8)
(8) where, Ht is the theoretical head:
(9)
(9) Based on the centrifugal pump impeller design method – the velocity coefficient method, only the number of blades Z and the outlet angle β2 cannot be expressed by the velocity coefficient, all other parameters can be calculated by the velocity coefficient method. As each speed coefficient of the centrifugal pump can be found by the specific speed ns, this paper only takes the impeller inlet diameter k0 as a function of the variable. With the flow rate, speed and specific speed determined, both the theoretical head and the total impeller hydraulic loss can be expressed as a function of the number of blades Z, the outlet angle β2 and the impeller inlet diameter coefficient k0 as follows:
(10)
(10)
(11)
(11) The hydraulic efficiency can therefore also be expressed as a function of the number of blades Z, the outlet angle β2 and the impeller inlet diameter velocity coefficient k0:
(12)
(12) In consideration of cavitation and efficiency, the high specific speed centrifugal pump k0 is taken as (3.9, 4.5), the number of blades Z is taken as (3,7) and the range of blade exit angle is (20°,30°).
3. Numerical models and experimental verification
3.1. Geometric model and grids
The single-stage high specific speed centrifugal pump studied in this paper consists of the main components such as impeller, volute, rotor and bearings. The pump is of vertical construction, electromechanically integrated, with a downward suction method. The impeller and volute are the main flow-passing components of a centrifugal pump. The impeller is the core component of the pump and the pump head, flow rate, efficiency and cavitation performance including the shape of the characteristic curve are inextricably linked to the parameters of the impeller. The volute is also a very important flow passage component, and its efficiency and matching with the impeller will directly affect the performance of the pump. In order to maintain good hydraulic performance, the profile of the volute should be aligned as closely as possible with the trajectory created by the movement of the water. The design parameters of the centrifugal pump are given in Table .
Table 1. Pump design parameters.
UG is used to model the main flow-passing components of the centrifugal pump separately in 3D. In order to reduce the influence caused by the inlet and outlet sections and to further improve the accuracy of the pump simulation, both the inlet and outlet sections of the pump are extended separately and then assembled. Fluent Meshing is used to generate and optimize the mesh calculations in this paper. The impeller geometry is also relatively complex due to the twisted blades and wide flow paths of the high specific speed centrifugal pump. To address this situation, this paper will use a stronger ortho-hexahedral mesh to divide and optimize the full computational domain. Firstly, local size refinement is performed in the impeller and volute tongue regions, and then a surface mesh with a growth rate of 1.2 is generated based on the Curvature & Proximity function. The final division result is shown in Figure by describing the geometric structure of the computational domain composed of fluid regions without voids and adding three layers of wall boundary layer generation grid. The quality of all grids is above 0.42, which can meet the requirements for numerical calculations.
The actual meshing does not have a significant effect on the results of the calculations up to a certain number of meshes. On the premise that the mesh division meets the calculation requirements, this paper uses the head of a centrifugal pump at a flow rate of 1000 m3/h as an indicator to verify the independence of the mesh and find the right number of meshes to reduce the calculation time. As can be observed in Figure , the pump head no longer varies significantly when the total number of grids is greater than 1.2 million, and the total number of grids is finally determined to be 1.2 million. Of these, the number of grids for each crossflow component is shown in Table .
Table 2. Number of grids per component.
3.2. Boundary condition
The calculation domain of the centrifugal pump in this paper includes the inlet and outlet extensions, the impeller, the volute, in 4 parts. Fluent is used for the numerical simulation, and the wall conditions in the inlet and outlet extensions and the volute area are set to stationary wall. Whereas the impeller as a rotor component is set into a rotational domain, given a rotational speed of 980 r/min, the positive and negative direction of impeller rotation is determined using the right-hand rule. The standard k-ε model is chosen for the turbulence model with the inlet boundary condition set to Total Pressure, the inlet turbulence intensity selected as Medium and the outlet boundary condition set to Mass Flow Rate. In the dynamic-static interface, the coordinate system transformation of the intersection between the inlet pipe and the impeller and between the impeller and the volute is set to Frozen Rotor mode. The solution method adopts SIMPLEC, and all discretization methods choose Second Order Upwind. To improve convergence speed, the sub relaxation factor of pressure is set to 0.8. The average RMS (Rate Monotonic Scheduling) is used as the basis for convergence judgment, with a residual target of 10−4. To further determine whether convergence meets the requirements, the residual of the flow rate at the outlet position is monitored for review. Steady numerical simulation of the internal flow field of the pump under different blade numbers and flow conditions.
3.3. Experimental verification
The centrifugal pump external characteristics tests are carried out on a comprehensive test rig with class II accuracy, as shown in Figure . In addition, Table provides the relative error (eT) and measurement range (Mr) of the pressure sensor and electromagnetic flow meter. All experimental results are the set average of 500 samples. The uncertainty of measurement result ΔN is the difference between the true value and the measured value, which is estimated by the square root method as shown in Equation Equation1(2)
(2) 3. The parameter NT is the uncertainty of the sensor, while the parameter ND is the uncertainty of the data acquisition system. The range of input voltage signal is as follows: pressure sensor is 0–15 V, electromagnetic flow meter is 100 V to 240 V. The absolute accuracy of the data acquisition system is 4.28 mV. Random noise and zero order uncertainty are ignored because they are considered very small. The distribution of results is considered as normal distribution, and the coefficient of normal distribution is selected as 1.96 (95% confidence level). The parameter nT represents the number of sensors used to jointly obtain a result. The uncertainty of measurement is also given in Table .
(13)
(13) In this paper, the external characteristics of the centrifugal pump were tested by manufacturing three- and six-blade impellers respectively. Tables and show the results and test values calculated by CFD (Computational Fluid Dynamics) for three and six blades at different flow conditions. It can be observed that when the number of impeller blades is 6, the test head and predicted head under design conditions are 19.40 and 19.60 m respectively, and the relative deviation between the two is 1.03%. At high flow rates (1400m3/h), the relative head deviation is 4.16%. When the number of impeller blades is 3, the test head and predicted head are 15.70 and 15.95 m respectively, with a relative deviation of 1.59%. Similarly, at high flow rate (1400m3/h), the relative head deviation is larger, which is 3.57%. As the flow rate increases, the difference between the experimental and CFD predictions becomes larger for different blade numbers, which may be due to the inevitable cavitation that occurs under high flow conditions. From the table, it can be found that there is a good agreement in the overall trend between the CFD predicted performance curve and the test curve, which indicates that the numerical simulation in this paper has a high reliability within a certain error.
Table 3. Uncertainty analysis of measurement values (FS: full scale).
Table 4. External characteristic data of three-blades.
Table 5. External characteristic data of six-blades.
Table 6. Quadratic regression design factor level coding table.
4. Optimal design by using CCD response surface method
4.1. Determination of test factors and test sites
Response surface methodology (RSM) is a mathematical and statistical method for expressing the relationship between multiple variables and the object of study by constructing an approximate function, and is widely used in various fields, often to study the relationship between a defined target object and multiple other variables. The Design-expert used in this paper provides a visual representation of the relationship between the object of study and a number of other variables through a combination of numerical and morphological methods, from which it is also possible to analyze and find the best response value for the target object under uncertainty among multiple variables. In this paper, the hydraulic efficiency of centrifugal pumps will be predicted on the basis of the functions already constructed.
Common types of response surface methodology designs include: 3k full factor, central combination (composite) design (CCD), box behnken design (BBD). In this paper, the second central combination (composite) design is used in response surface optimization, the setting of the test points is very important. In a three-factor experiment, for example, the three factors chosen, a1, a2 and a3, need to have ranges, and the upper and lower lines of the ranges are called upper and lower levels. The upper, zero and lower levels are normalized to values of 1, 0 and −1. As in Table .
The generic rotating combination design test points are shown in Table below p in the table indicates the number of factors, test points mc, and two test points with absolute value r on each factor axis. p axes will have 2p test points, denoted by mr. m0 is the number of repetitions of the centre point test where each factor is taken to zero level. the generic rotating combination design test points will be arranged as in Table .
Table 7. Setting table of test sites.
Based on the numerical simulation results, a hydraulic loss model is developed as a function of efficiency, which is then combined with central combined (composite) design (CCD)-response surface optimization analysis to perform a regression analysis of the efficiency of high specific speed centrifugal pumps, using this method to find the significant factor affecting the hydraulic efficiency of the pump and to further analyze the effect of this factor on efficiency.
From the function constructed above, it can be observed that the efficiency is a function of the speed parameter k0, the number of blades Z and the blade exit angle β2. In order to meet the design requirements for high specific speed centrifugal pumps, the range of β2 is (20°,30°), the range of k0 is (4.0,4.5) and the range of Z is (3,7). Think of them as x1, x2, x3 and efficiency as y.
The experiments are carried out from the test points designed in the table, where A = x1, B = x2 and C = x3, and the efficiencies obtained from the simulations of the different design parameters, i.e. y, are filled in the corresponding tables. The test points for this paper are shown in Table and the range of parameters is shown in Table below.
Table 8. Test sites.
The objective of the experiment was to find the maximum efficiency, i.e. the maximum value of y in the function. The number of factors involved in the experiment is 3. A generic rotational combination design was used and a table check gave r = 1.682.
4.2. Analysis of variance and response regression model
Variance is a statistic that indicates the degree of dispersion of a random variable and the expected value. For statistical purposes and judgement of the data, an analysis of variance (ANOVA) is conducted on the efficiency of the target variables following the process of ANOVA to obtain ANOVA Table . According to the data in the table, y is significantly related to the model in the established regression equation and the misfit term is not significant, which indicates that the established functional model is mathematically and statistically significant. The response regression model can be obtained by removing p < 0.05 items according to the statistical analysis results:
(13)
(13) The degree of influence (significance) of the three factors, blade exit placement angle, speed coefficient and number of blades, on the efficiency y can be visualized by the regression diagram in Figure , where the slope of the factor has a positive relationship with the response value y. As can be observed in the graph, the three factors have the following significant effects on the efficiency y in descending order, number of blades (C), exit angle (A) and speed coefficient (B). As the equation of the function y with respect to x1, x2, x3 has three variables, it is not possible to plot the relevant graph on the plane. In order to obtain a graph of the relationship between the three variables on the plane it is necessary to determine one variable so that the relationship between the response values and the variables in the graph can be seen more visually.
Table 9. Values range of x1, x2, x3.
Table 10. Design of parameter coding table.
Table 11. Analysis of variance table.
4.3. Response surface diagram analysis
The two-factor interaction plots of hydraulic efficiency versus outlet angle, velocity coefficient and number of blades can be derived from Design expert, respectively (Figures ). The efficiency contour plot is shown on the left and the 3D plot on the right.
The contour plot on the left is the projection of the 3D response surface on the right. As shown in the figure, when the number of blades (5 blades in the figure) is certain, in the range of impeller exit angle (20°,30°) and speed coefficient (3.9,4.5), the speed coefficient is constant and the efficiency increases with the increase of exit angle. When the exit angle is constant, the velocity coefficient increases and the efficiency decreases with increasing velocity coefficient. Among them, the decrease of efficiency is larger when the speed coefficient is in (3.9,4.2). When the efficiency is 88%, the magnitude of contour change is small. This indicates that the relationship between outlet angle and efficiency is not significant, and provides guidance for us to mainly consider velocity coefficient and secondly consider blade outlet angle when optimizing efficiency. The minimum value of efficiency in the contour is 88% and the maximum value of efficiency is 89%.
As shown in Figure , when the speed factor is 4.2 and the exit angle is constant, the efficiency increases with decreasing number of blades. But at this speed coefficient, when the number of blades is constant, the efficiency increases with the increase of the exit angle. Therefore, the minimum value of efficiency appears in the region where the number of blades is larger and the exit angle is smaller. The maximum value of efficiency appears in the area of smaller number of blades and larger exit angle. Specifically, the minimum value of the efficiency contour appears in the area where the number of blades is (6, 7) and the exit angle is (20°, 24°), and the value is 87.6%. The maximum value appears in the area where the number of blades is (3, 4) and the exit angle is (28°, 30°), with a value of 88.6%. This indicates that under a certain velocity coefficient, excessive blades and a small outlet angle can seriously reduce the efficiency of high specific speed centrifugal pumps. Therefore, we need to try to avoid this situation when designing pumps (Table ).
It can be observed from Figure that when the exit angle is 30° and the speed coefficient ranges from (3.9, 4.5), the efficiency decreases with increasing speed coefficient when the number of blades is certain, especially the speed coefficient is most significant in the range of (3.9, 4.2). And when the speed coefficient is constant, the overall efficiency decreases with the increase of the number of blades. In the contour plots, the contour lines tend to be straight indicating a significant relationship between the corresponding number of blades and efficiency. This indicates that when the blade outlet angle is constant, both high blade number and large velocity coefficient will reduce the efficiency of the centrifugal pump, but the influence of velocity coefficient is more significant. Therefore, the determination of velocity coefficient in the design parameters of the centrifugal pump cannot be ignored. The minimum value of the efficiency contour in the graph is 87.5% and the maximum value is 89%.
4.4. Determine the optimal scheme
From the ANOVA table (Table ), it is clear that the interaction of exit angle and impeller inlet diameter velocity coefficient has a significant effect on efficiency. Therefore, the effect of exit angle and impeller inlet diameter velocity coefficient on efficiency was analyzed separately in this paper for different number of blades.
The three curves above Figure represent the efficiency distribution when the impeller inlet diameter velocity coefficient is 3.9. And the remaining curve represents the efficiency distribution when the velocity coefficient is 4.5. It can be seen that the hydraulic efficiency decreases as the number of blades increases. When the number of blades is 3 and 7, the maximum and minimum values of efficiency (response value) appear under the interaction of outlet angle and velocity coefficient, respectively. Because the number of blades with higher efficiency is generally chosen in practical applications, the data of seven blades are not referred to. And it can be clearly seen from Figure that when the exit angle is at (28°, 30°), the highest efficiency point appears. When the speed coefficient is 3.9, the blade exit angle is 30°, the number of blades is 3, the hydraulic efficiency reaches the maximum value, the value is 89.5%. Although all three factors in this article have ranges, it can verify the feasibility of our efficiency prediction method and provide a basis for us to expand the parameter range in the future.
Figure 8. Graph of interaction between exit angle and velocity coefficient. (a) Number of blades Z = 3. (b) Number of blades Z = 4. (c) Number of blades Z = 5. (d) Number of blades Z = 6 and (e) Number of blades Z = 7.

Figure reflects that the predicted value of the response surface method is in good agreement with its response value. The response value is the value calculated by the hydraulic loss model from the test point data, while the predicted value is calculated by the regression model constructed by the functional equation. Because in practical applications, the number of blades and outlet angle are generally chosen as integers, and the three factors studied in this paper have a range and all have a significant effect on the efficiency, so it is also possible to choose different values of the factors through the constructed model. The final results of the model predictions are visualized in the software Design-expert, which predicts its hydraulic efficiency.
4.5. Verification by using CFD
In order to further verify the reliability of the hydraulic efficiency model predictions, impeller models with different blades with a blade exit angle of 30° and an impeller inlet diameter velocity coefficient of 3.9 were built respectively, based on the previously established hydraulic models, without changing the volute design parameters. Numerical calculations are carried out by dividing the mesh and setting the boundary conditions. The results of the treatment were calculated by Fluent at a flow working condition of 1000m3/h flow working condition. The number of blades and the hydraulic efficiency are obtained in Figure below.Based on the FLUENT processing results, the hydraulic efficiency of the three-blade model pump is maximum when the velocity coefficient is 3.9 and the blade outlet angle is 30°. This result is consistent with the model prediction. This indicates that the model establishment has a certain guiding effect on the prediction of the hydraulic efficiency of the high specific speed centrifugal pump. At the same time, the numerical calculation of the model and the analysis of the response surface also provide a certain basis for the design of the parameters of the high ratio speed centrifugal pump. Because the hydraulic efficiency directly affects the efficiency of the centrifugal pump, when the hydraulic efficiency is higher, the pump efficiency will also be higher, so the prediction of the hydraulic efficiency of the centrifugal pump is equivalent to the prediction of the efficiency of the centrifugal pump. Therefore, the prediction of centrifugal pump performance has a certain feasibility and accuracy.
To highlight the effectiveness of our optimization, as shown in Figure , taking 6 blades as an example, it can be seen that there is no significant change in the impeller area before and after optimization, and the distribution is uniform. In contrast, the range of turbulent kinetic energy variation inside the volute is relatively large. Before optimization, the turbulent kinetic energy value is relatively large, with most areas inside the volute being 1.5m2/s2, with the larger value appearing on the outer side of the volute reaching 4.0m2/s2. After optimization, the turbulence intensity of the volute becomes uniform, indicating that the flow in this part is not complex and the energy loss is small. For the total pressure distribution, it is evident that in the impeller area, the impeller distribution is uniform and there is no significant change in size. The distribution of total pressure in the volute is uneven. Before optimization, relative high pressure appeared near the volute near the impeller, with most of the high-pressure values reaching 300kPa. In comparison, after optimization, there was no significant change in the impeller, the low-pressure range of the impeller flow channel decreased, and the total pressure value inside the volute decreased. So overall, our method has a significant optimization effect on the internal flow characteristics of centrifugal pumps.
5. Conclusion
In this paper, the range of variables is determined according to the demand of high specific speed centrifugal pumps, and the method of mathematical statistics (response surface analysis) is applied to solve the equation of hydraulic efficiency and dependent variable in the range of variables, which serves to predict the hydraulic efficiency. And verify its accuracy by numerical simulation. Through the relationship between hydraulic efficiency and efficiency, the efficiency and other performance of centrifugal pumps are further predicted. The idea of extreme value method of hydraulic loss also provides some reference direction for the hydraulic design of impeller. The main conclusions are as follows.
Although there is relative error between the numerical simulation and the test results of the high specific speed centrifugal pump, the change trend is basically the same. Practice has proved that the experimental design of this study has certain credibility, so the optimization results can be used for the design of high specific speed centrifugal pump in the future.
From response surface analysis, it can be concluded that the blade inlet diameter velocity coefficient has the greatest impact on hydraulic efficiency among the three factors of blade outlet angle, blade number, and velocity coefficient. Blade exit angle (x1), speed coefficient (x2), number of blades (x3) and target variable efficiency are subjected to ANOVA. The results show that y is significantly related to the model in the established regression equation and the misfit term is not significant. This indicates that the established functional model has mathematical and statistical significance. Based on the results of the statistical analysis to remove the p < 0.05 term, the centrifugal pump hydraulic efficiency response regression model was established:
Based on the regression diagram it can be visualized that the blade inlet diameter velocity coefficient has the greatest effect on the hydraulic efficiency, followed by the two-factor interaction diagram where the interaction between the outlet angle and the impeller inlet diameter velocity coefficient is found to have a significant effect on the efficiency. Hence, a separate analysis of the effect of outlet angle and impeller inlet diameter velocity coefficient on efficiency at different numbers of blades shows that the overall hydraulic efficiency decreases with increasing number of blades.
Through response surface analysis and verification by CFD numerical simulation, the high specific speed centrifugal pump studied in this paper can reach its maximum hydraulic efficiency at 1000m3/h operating conditions with a blade number of 3, a velocity coefficient of 3.9 and an outlet angle of 30°. This research provides a new direction for the hydraulic design of high specific speed centrifugal pumps. Therefore, the prediction of the hydraulic efficiency of high specific speed centrifugal pumps based on the response surface method has a certain feasibility and accuracy.
Due to the direct impact of hydraulic efficiency on the efficiency of centrifugal pumps, when the hydraulic efficiency is high, the pump efficiency will also be higher. Therefore, predicting the hydraulic efficiency of centrifugal pumps is equivalent to predicting the efficiency of centrifugal pumps. The calculation and establishment of hydraulic efficiency models and response surface analysis in this article provide a certain basis for the parameter design of high specific speed centrifugal pumps, and enrich the research direction of high specific speed centrifugal pumps.
Disclosure statement
No potential conflict of interest was reported by the author(s).
Additional information
Funding
References
- Anwar, M., Rasul, M. G., Ashwath, N., & Rahman, M. M. (2018). Optimisation of second-generation biodiesel production from Australian native stone fruit oil using response surface method. Energies, 11(10), 2566. https://doi.org/10.3390/en11102566
- Capurso, T., Bergamini, L., & Torresi, M. (2022). A new generation of centrifugal pumps for high conversion efficiency. Energy Conversion and Management, 256, 115341. https://doi.org/10.1016/j.enconman.2022.115341
- He, D., Zhao, L., Chang, Z., Zhang, Z., Guo, P., & Bai, B. (2021). On the performance of a centrifugal pump under bubble inflow: Effect of gas-liquid distribution in the impeller. Journal of Petroleum Science and Engineering, 203, 108587. https://doi.org/10.1016/j.petrol.2021.108587
- Hergt, P. H. (1999). Pump research and development: past, present, and future. Journal of Fluids Engineering, https://doi.org/10.1115/1.2822198
- Huang, R., Zhang, Z., Zhang, W., Mou, J., Zhou, P., & Wang, Y. (2020). Energy performance prediction of the centrifugal pumps by using a hybrid neural network. Energy, 213, 119005. https://doi.org/10.1016/j.energy.2020.119005
- Li, H., Han, Y., Shi, W., Tiganik, T., & Zhou, L. (2022). Automatic optimization of centrifugal pump based on adaptive single-objective algorithm and computational fluid dynamics. Engineering Applications of Computational Fluid Mechanics, 16(1), 2222–2242. https://doi.org/10.1080/19942060.2022.2143901
- Li, T. Z., & Yang, X. L. (2018). Probabilistic stability analysis of subway tunnels combining multiple failure mechanisms and response surface method. International Journal of Geomechanics, 18(12), 04018167. https://doi.org/10.1061/(ASCE)GM.1943-5622.0001315
- Lin, T., Li, X., Zhu, Z., Xie, J., Li, Y., & Yang, H. (2021a). Application of enstrophy dissipation to analyze energy loss in a centrifugal pump as turbine. Renewable Energy, 163, 41–55. https://doi.org/10.1016/j.renene.2020.08.109
- Lin, T., Zhu, Z., Li, X., Li, J., & Lin, Y. (2021b). Theoretical, experimental, and numerical methods to predict the best efficiency point of centrifugal pump as turbine. Renewable Energy, 168, 31–44. https://doi.org/10.1016/j.renene.2020.12.040
- Lin, Y., Li, X., Zhu, Z., Wang, X., Lin, T., & Cao, H. (2022). An energy consumption improvement method for centrifugal pump based on bionic optimization of blade trailing edge. Energy, 246, 123323. https://doi.org/10.1016/j.energy.2022.123323
- Napreenko, K. S., & Lamtyugina, A. V. (2021). Hydraulic losses optimization methods used in air conditioning system valve design for different closure angles. In IOP conference series: Materials science and engineering (Vol. 1027, No. 1, p. 0012022). IOP Publishing. https://doi.org/10.1088/1757-899X/1027/1/012022
- Nishi, Y., Suzuo, R., Sukemori, D., & Inagaki, T. (2020). Loss analysis of gravitation vortex type water turbine and influence of flow rate on the turbine’s performance. Renewable Energy, 155, 1103–1117. https://doi.org/10.1016/j.renene.2020.03.186
- Norouzi, M., Fazeli, A., & Tavakoli, O. (2020). Phenol contaminated water treatment by photocatalytic degradation on electrospun Ag/TiO2 nanofibers: Optimization by the response surface method. Journal of Water Process Engineering, 37, 101489. https://doi.org/10.1016/j.jwpe.2020.101489
- Perissinotto, R. M., Verde, W. M., Biazussi, J. L., Bulgarelli, N. A. V., Fonseca, W. D. P., de Castro, M. S., de Moraes Franklin, E., & Bannwart, A. C. (2021). Flow visualization in centrifugal pumps: A review of methods and experimental studies. Journal of Petroleum Science and Engineering, 203, 108582. https://doi.org/10.1016/j.petrol.2021.108582
- Pudjianto, D., Djapic, P., Aunedi, M., Gan, C. K., Strbac, G., Huang, S., & Infield, D. (2013). Smart control for minimizing distribution network reinforcement cost due to electrification. Energy Policy, 52, 76–84. https://doi.org/10.1016/j.enpol.2012.05.021
- Šavar, M., Kozmar, H., & Sutlović, I. (2009). Improving centrifugal pump efficiency by impeller trimming. Desalination, 249(2), 654–659. https://doi.org/10.1016/j.desal.2008.11.018
- Shamsuddeen, M. M., Ma, S. B., Kim, S., Yoon, J. H., Lee, K. H., Jung, C., & Kim, J. H. (2021). Effect of an inducer-type guide vane on hydraulic losses at the inter-stage flow passage of a multistage centrifugal pump. Processes, 9(3), 526. https://doi.org/10.3390/pr9030526
- Shankar, V. K. A., Umashankar, S., Paramasivam, S., & Hanigovszki, N. (2016). A comprehensive review on energy efficiency enhancement initiatives in centrifugal pumping system. Applied Energy, 181, 495–513. https://doi.org/10.1016/j.apenergy.2016.08.070
- Trinh, T. K., & Kang, L. S. (2010). Application of response surface method as an experimental design to optimize coagulation tests. Environmental Engineering Research, 15(2), 63–70. https://doi.org/10.4491/eer.2010.15.2.063
- Vasuthevan, H., & Brümmer, A. (2018). Multiphase-flow simulation of a rotating rectangular profile within a cylinder in terms of hydraulic loss mechanisms. In IOP conference series: Materials science and engineering (Vol. 425, No. 1, p. 0012002). IOP Publishing. https://doi.org/10.1088/1757-899X/425/1/012002
- Wang, C., Shi, W., Wang, X., Jiang, X., Yang, Y., Li, W., & Zhou, L. (2017). Optimal design of multistage centrifugal pump based on the combined energy loss model and computational fluid dynamics. Applied Energy, 187, 10–26. https://doi.org/10.1016/j.apenergy.2016.11.046
- Wang, C. N., Yang, F. C., Nguyen, V. T. T., & Vo, N. T. (2022). CFD analysis and optimum design for a centrifugal pump using an effectively artificial intelligent algorithm. Micromachines, 13(8), 1208. https://doi.org/10.3390/mi13081208
- Wang, D., Parkinson, S., Miao, W., Jia, H., Crawford, C., & Djilali, N. (2012). Online voltage security assessment considering comfort-constrained demand response control of distributed heat pump systems. Applied Energy, 96, 104–114. https://doi.org/10.1016/j.apenergy.2011.12.005
- Wu, C., Pu, K., Li, C., Wu, P., Huang, B., & Wu, D. (2022). Blade redesign based on secondary flow suppression to improve energy efficiency of a centrifugal pump. Energy, 246, 123394. https://doi.org/10.1016/j.energy.2022.123394
- Yıldız, B. S. (2020). Optimal design of automobile structures using moth-flame optimization algorithm and response surface methodology. Materials Testing, 62(4), 371–377. https://doi.org/10.3139/120.111494
- Yusri, I. M., Majeed, A. A., Mamat, R., Ghazali, M. F., Awad, O. I., & Azmi, W. H. (2018). A review on the application of response surface method and artificial neural network in engine performance and exhaust emissions characteristics in alternative fuel. Renewable and Sustainable Energy Reviews, 90, 665–686. https://doi.org/10.1016/j.rser.2018.03.095
- Zhao, J., Pei, J., Yuan, J., & Wang, W. (2022a). Energy-saving oriented optimization design of the impeller and volute of a multi-stage double-suction centrifugal pump using artificial neural network. Engineering Applications of Computational Fluid Mechanics, 16(1), 1974–2001. https://doi.org/10.1080/19942060.2022.2127913
- Zhao, X., Zhang, D., Zhang, R., & Xu, B. (2022b). A comparative study of Gaussian process regression with other three machine learning approaches in the performance prediction of centrifugal pump. Proceedings of the Institution of Mechanical Engineers, Part C: Journal of Mechanical Engineering Science, 236(8), 3938–3949. https://doi.org/10.1177/09544062211050542
- Zhou, X., Zhang, H., Qiu, R., Liang, Y., Wu, G., Xiang, C., & Yan, X. (2019). A hybrid time MILP model for the pump scheduling of multi-product pipelines based on the rigorous description of the pipeline hydraulic loss changes. Computers & Chemical Engineering, 121, 174–199. https://doi.org/10.1016/j.compchemeng.2018.10.001