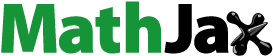
Abstract
Heating and thermal comfort have remarkable share of final energy consumption. Until now, most of the demand for heating applications in buildings is supplied by fossil fuels and electrical technologies. Concerning the exhaustion of fossil fuels in the future and the environmental problems related to their consumption, making use of renewable energy sources can be a practical alternative. On this point, solar energy is an appropriate source to be applied for heating by utilizing different technologies. The function and output of solar heaters depends on numerous factors, and this causes difficulties in the prediction of their performance and modelling. In this scenario, intelligent techniques are helpful and have been used by several scholars in recent years. This paper reviews proposed models for the prediction of the performance of different solar heaters. The literature review reveals that artificial neural Networks represent one of the most used approaches for the performance prediction of solar heaters; however, other intelligent techniques, namely support vector machines, have been used for this purpose too. Moreover, it is found that these methods have the ability to predict with great precision by applying the appropriate approach and architecture. In addition, it can be noted that the function of the models generated based on intelligent techniques are associated with some elements such as the employed function and architecture of the model.
1. Introduction
Building sector decarbonization is significantly crucial to mitigating climate change since this sector is responsible for around 40% of overall energy utilization and 35% of total emission of greenhouse gases in the world (Ahmed et al., Citation2022). Heating has a notable rôle in the amount of energy consumption in the world, especially in the residential sector. Around 50% of building energy demand in 2021 was for water and space heating, which led to the direct emission of 2450 Mt CO2. Despite the growing trend for making use of clean energy technologies for heating, fossil fuels are responsible for more than 60% of heating demand in the world (Goodson et al., Citation2022). Regarding net zero scenario milestones, it is necessary to accelerate decarbonization in heating by 2030 (Goodson et al., Citation2022), which necessitates substituting current technologies with cleaner ones. For this purpose, making use of promising systems with lower emissions such as district heating, heat pumps and renewable-energy-based technologies have been recommended. Renewable-energy sources can be applied for heating by implementing different technologies and tools. For instance, biofuels can be used in heat pumps in order to supply thermal energy to buildings in an efficient and environmentally-friendly way (Blázquez et al., Citation2021). Aside from biofuels, other renewable energies like solar and geothermal are applicable as the heat source for heat pumps (Fang et al., Citation2020; Self et al., Citation2013; Soltani et al., Citation2019). Besides single-source heat pumps, it is possible to integrate heat pumps with more than one renewable energy source, e.g. solar and geothermal (Choi et al., Citation2014). In addition to indirect heat generation using renewable energy sources, it is possible to extract thermal content directly and use it for space or water heating.
Solar heaters are among the clean and renewable energy-based systems applicable for air, space or water-heating (Kashyap et al., Citation2023; Patel et al., Citation2023). Several studies have analysed the function of solar heaters and the elements influencing their output. For instance, Said, Ghodbane, et al. (Citation2021) investigated the performance of a Linear Fresnel solar Reflector (LFR) water heating system. Their findings revealed that the maximum temperature was observed on the tube of receiver and after it hot water had the highest temperature. In addition, they noted that, for the considered system with the ability to heat 0.4 m3 of water per day, the CO2 mitigation was equal to 247.14 kg. Al-Askaree and Al-Muhsen (Citation2023) analysed the function of a Solar Water Heater (SWH) consisting of a serpentine fin core heat exchanger. It was stated that an increase in the water flow speed caused a decrease in efficiency, since the accelerated water period in large quantities was not enough to heat that amount of water. There are some ideas for the enhancement of the performance of SWHs. For instance, Nazari et al. (Citation2022) applied a CuO nanostructure coating to a serpentine tube flat plate SWH. They stated that, by using the coating on the absorber surface, the absorption of solar radiation was increased, which led to an increase in the outlet temperature. This caused an improvement in the exergy efficiency of the collector by 11.2% in comparison with the absorber without a coating. Thangavelu et al. (Citation2021) analysed the effect of the area of a solar collector on the exergetic dimensions of an SWH usable on a domestic scale. They noted that an increase in the collecting area would decrease the exergy efficiency. Furthermore, it was concluded that the exergy destruction cost rate was higher in cases where collectors with larger area were used. Gunasekaran et al. (Citation2021) investigated the influence of the utilization of twisted tape on the function of an evacuated tube collector SWH. They concluded that the insertion of twisted tape having twist ratios of 2 and 3 induced efficiency modification by 6.8% and 4.6%, respectively. Said, Ghodbane, et al. (Citation2021) implemented a 4E (Energy, Exergy, Economic and Environment) analysis of a linear Fresnel reflector usable for hot water production. They noted that the cost of the reflector was US$378.87, which could be recovered after 16 years from first utilization. Moreover, the environmental analysis revealed that the CO2 mitigation potential of the designed system, having a daily hot water production of 4 m3, was 247.14 kg.
As well as SWHs, heaters used for air have been considered and studied in various research, namely Akhbari et al. (Citation2020) analysed the function of a Solar Air Heater (SAH) with a triangular channel and found that the highest thermohydraulic performance was obtainable with an internal peak angle of the channel equal to 60°, for cases between 35° and 60°. Moreover, it was observed that, for a certain thermal efficiency, the designed air heater needed a lower surface area in comparison with a flat plate heater. Jouybari and Lundström (Citation2020) investigated the performance of an SAH by using a thin porous material as a cover for the absorber plate. The highest increment in the thermohydraulic and thermal performance of the system with porous material was more than five times that of the systems without that medium. Hassan et al. (Citation2020) proposed a new architecture for SAH by replacing the flat absorber plate with adjacent tubes and found that, by using the new design, the system mean daily efficiency increases by up to 132.6% in the case of an air mass flow rate of 0.075 kg/s. D. Wang et al. (Citation2020) applied an ‘S’-shaped ribs gap on an SAH and found a notable elevation in the efficiency in comparison with the system with a smooth plate. Afshari et al. (Citation2020) assessed the influence of turbulators modification on the function of an SAH and found that heater maximum efficiency can reach up to 72.41%. Shetty et al. (Citation2020) did a study on the impact of perforation on the output of a cross flow SAH and reported that an increment in the quantity of perforations induced a remarkable increment in the thermohydraulic efficiency, despite a marginal reduction in the thermal efficiency. Some researchers have focused on SAHs with storage units (Baig & Ali, Citation2019). For instance, Sajawal et al. (Citation2019) experimentally analysed a double pass SAH with a finned tube Phase Change Material (PCM). They reported that the utilization of PCM in the system increased the performance and output of the SAH.
Complex system modelling can be performed by applying intelligent methods, namely Support Vector Machines (SVMs) and Artificial Neural Networks (ANNs) (M.M. Rashidi et al., Citation2021). These techniques have been applied in modelling different thermal and renewable energy systems and utilized materials (Razavi et al., Citation2019). For instance, Alotaibi et al. (Citation2020) made use of Group Methods of Data Handling (GMDH) and Multivariate Adaptive Regression Splines (MARSs) for estimating the thermal conductivity of some nanofluids and reported significantly good performance of GMDH in the modelling. In other research (M.H. Ahmadi et al., Citation2019), GMDH was employed for forecasting the thermal resistance and effective thermal conductivity of oscillating heat pipes and it was seen that this method was able to predict the mentioned variables with acceptable precision. Regarding the dependency of solar systems on different elements and parameters, these approaches have been applied for modelling them by different scholars. For example, Sridharan (Citation2020) made use of a generalized regression neural network to forecast the output of a solar dryer. It was discovered that the model was able to predict the performance of the system with an overall accuracy of 96.29%. Tripathy and Kumar (Citation2009) applied an ANN to predict the temperature of food in the process of drying in a mixed mode solar dryer. The results indicated perfect exactness of the predictive model with an R2 of 0.952. Abujazar et al. (Citation2018) employed an ANN for proposing a model for a stepped solar still and generated a model with a Root Mean Square Error (RMSE) of 22.48%. Wang, Kandeal, et al. (Citation2021) applied three methods including ANNs, random forests and multilinear regression for solar still function modelling. Determination coefficients of the mentioned models were 0.9614, 0.9758 and 0.9267, respectively. Sohani et al. (Citation2022) made use of an ANN to model the dynamic performance of an enhanced solar still. The created model was able to forecast daily water production with an error in the range 2.41–5.84%. D. Wang et al. (Citation2020) used an ANN to estimate Photovoltaic (PV) performance by using the data from numerical simulation. The highest relative deviation between the values of the ANN model and the numerical results was around 0.04%.
Literature reviews of the utilization of intelligent methods for modelling different solar systems have shown their great performance and high reliability in making predictions. There are some review papers on the usage of these methods for solar systems. For instance, Voyant et al. (Citation2017) provided a review on the usage of machine learning techniques for forecasting solar radiation. In another review study, Mahmood and J.L. Wang (Citation2021) focused on the applications of these techniques to organic solar cells. E. Engel and N. Engel (Citation2022) reviewed the usage of machine learning methods for solar plants. S. Rashidi et al. (Citation2022) provided a review study on the utilization of intelligent techniques for the assessment of solar desalination technologies. Aside from the above-mentioned solar systems, these methods are applicable for different kinds of solar heater. For instance, Li et al. (Citation2017) summarized their recent work and proposed a general framework for the design of solar water heaters by making use of a high-throughput screening method corresponding to machine learning approaches. In spite of the existence of different intelligent techniques for modelling solar heaters, no comprehensive and updated review has been found on this subject. In this regard, this study reviews the scientific publications on the employment of intelligent procedures for modelling the characteristics and performance of solar heaters. The main applications of intelligent methods for modelling solar heaters are the performance prediction of these systems with acceptable exactness and precision by a fast procedure. Moreover, the proposed models can be useful in the process of designing new systems to evaluate their performance and feasibility. In this regard, providing a comprehensive review of previous studies would be useful for scholars, engineers and designers working on solar heaters. In addition, some recommendations are produced for application in upcoming works in this area of science.
2. Intelligent methods
Different kinds of model on the basis of machine learning methods have been proposed for engineering problems. These models, on the basis of the machine learning methods, are attractive for scholars and researchers regarding their advantages, such as remarkable accuracy and fast performance (Ghalandari et al., Citation2021). In this section, some of the most applicable ones in terms of modelling different solar heaters are briefly introduced.
2.1. MultiLayer Perceptron (MLP) artificial neural network
ANNs are among the artificial intelligence concepts inspired by the performance of the human brain in phenomena identification. In MLP ANNs, neurons are located in three or more layers. The 1st, 2nd and 3rd layers are called the input, hidden and output layers. It is possible to use more than one hidden layer in this type of network. A schematic of this network is depicted in Figure . X1 to Xn (i = 1,2, … ,N) are the inputs of the network and n refers to the number of input variables, j refers to the number of neurons, wji are the weights of neurons and refer to the synapse power of the ith neuron to the jth neuron, yj is the network output, which is the modelling result and target of the model, uj is the combination of the output linear layer and is determined using Equation (1) (khosrojerdi et al., Citation2016):
(1)
(1) where
(2)
(2)
(3)
(3)
Figure 1. MLP ANN schematic. Adapted from khosrojerdi et al. (Citation2016).

In the above equations, is the activation function and
refers to the function tendency. There are various activation functions that are usable in these networks. The Tan-sigmoid and Log-sigmoid are among the most used functions in these models, which are as follows (khosrojerdi et al., Citation2016):
(4)
(4)
(5)
(5) In the abovementioned equations,
is the activation function input. In general, a neural network operates similarly to a function that receives as many inputs as the number of input layer neurons and has as many outputs as the number of output layer neurons (khosrojerdi et al., Citation2016).
2.2. Group Method of Data Handling (GMDH)
Another of the ANN-based model approaches is the GMDH, which is applicable for different systems. In GMDH, the output can be traced back to the variables utilized as the inputs. Each neuron in this network receives two inputs and provides an output as a function of them. The layers in the GMDH are composed of these types of neuron. Models based on the GMDH are developed and generated by constructing these kinds of layer until a cut-off state is reached. In Figure , the basic architecture of a neuron in the GMDH is illustrated. The relationship or function between the inputs and output of neurons can be of polynomial type, such as parabolic, cubic or linear, as in Equation (6). Linear functions are very often used in cases having small training datasets such that the result is not affected by polynomials of higher order. In this condition, the neuron output is determined by employing Equation (6) (Rizvi et al., Citation2020):
(6)
(6) In this equation, Y is the output, Cn refer to the coefficients and a and b are the inputs. In order to determine the output, the coefficients are required. In this regard, to obtain the coefficients, Lagrange interpolation is applied. Two neurons’ inputs are applied to generate a matrix equation (Equation 7) for finding the neurons’ coefficients (Rizvi et al., Citation2020):
(7)
(7) The network input layer acts only as the input provider. The subsequent layer, the 1st hidden layer, receives input from the previous layer when activated. This layer has C(N,2) neurons, in which N refers to the number of neurons in the former layer. This network is generated in such a way that a neuron is there for each input combination. Each neuron in this layer utilizes the training data for the determination of transfer functions and tries to forecast the testing data output. The standard error is determined by comparing the forecast data with the actual values. In order to avoid network exponential growth, some of the neurons with high errors are eliminated. Similarly, the subsequent hidden layer utilizes it as the inputs and the procedure continues to reach the acceptable outputs (Rizvi et al., Citation2020).
Figure 2. Schematic of basic neuron architecture (Rizvi et al., Citation2020). © Elsevier. Reproduced by permission of 5497741000403.

2.3. Least Square Support Vector Machine (LSSVM)
Another method applied as intelligent approach in modelling engineering problems and regression is the least square support vector machine. In general, the LSSVM nonlinear function is as shown in Equation (8) (Maleki et al., Citation2021):
(8)
(8) where f refers to the inputs−output relation, w denotes the weight vector and φ is employed to convert the inputs into the characteristic vector (M.A. Ahmadi & Mahmoudi, Citation2016; Ramezanizadeh, Ahmadi, Nazari et al., Citation2019). For topology minimization, a fitting error function should be calculated in the procedure for finding the regression problem output according to Equation (9) (M.A. Ahmadi & Mahmoudi, Citation2016; Ramezanizadeh, Ahmadi, Nazari et al. Citation2019):
(9)
(9) where
is a margin parameter. The limitation equation should be taken into account as follows (Maleki et al., Citation2021):
(10)
(10) where ek is the looseness variable (M.A. Ahmadi & Mahmoudi, Citation2016; Ramezanizadeh et al., Citation2018).
Lagrange multipliers, , are employed to obtain the optimization process solution. These are defined according to Equation (11) as follows:
The optimal state of the regression may be calculated by considering the state parameters and implementation of the partial derivatives in the following equation:
(12)
(12) For linear formulation of these equations, Equation (13) is applied (Maleki et al., Citation2021):
(13)
(13) In the above equation, Y, α and Z are defined as {Y = Y1; … ; Yym}, α = [α1, … ,α1] and Z = φ(X1)TYi, … ,φ(Xm)TYm, respectively. Utilizing the kernel function as K(X, Xk) = φ(X)Tφ(Xk), i = 1,2, … ,m, the LSSVM regression is calculated according to Equation (14) (M.A. Ahmadi & Mahmoudi, Citation2016; Ramezanizadeh, Ahmadi, Nazari et al., Citation2019):
(14)
(14) One of the most conventional kernels in regression error is the radial basis as in Equation (15) (M.A. Ahmadi & Mahmoudi, Citation2016; Ramezanizadeh, Ahmadi, Nazari et al., Citation2019):
(15)
(15) where
is the squared bandwidth. The value of this parameter is calculated by using an optimization approach in which the Mean Squared Error (MSE) is the objective function of the problem.
2.4. Adaptive Neuro Fuzzy Inference System (ANFIS)
A schematic of the Adaptive Neuro Fuzzy Inference System (ANFIS) model in with an output and two inputs is provided in Figure . According to this figure, there are five layers in this model type. In the first layer, the inputs are converted into fuzzy sets on the basis of fuzzy membership functions. Afterwards, the input signals are created in the next layer and the related weight of the membership functions are checked. Subsequently, in the next stage normalized firing strength is determined for the nodes. In the subsequent layer, the 4th layer, the results are converted into defuzzified sets. In the last layer, the outputs of the model are provided. In this layer, the summation of all the input signals, obtained from the previous stage, are defined (Ramezanizadeh, Ahmadi, Nazari et al., Citation2019).
Figure 3. ANFIS schematic for one output and two inputs (Ramezanizadeh, Ahmadi, Ahmadi, et al., Citation2019). ©Elseveier. Reproduced by permission of 5592070599260.

3. Operating principle of solar heaters
In solar heaters, the energy content of a solar beam is utilized in order to increase the temperature of the operating fluid. Depending on the required application, different components can be employed in these technologies. Generally, the main applications of solar heaters, particularly in buildings, are increasing the temperature of air or water. In Figure , a schematic of a simple SAH is provided. In SAHs, an absorber plate is employed to collect solar irradiance and transmit the thermal energy to the air flow through the duct. Moreover, glazing is employed on the glass top surface to boost solar radiation absorption. Furthermore, insulation is used to decrease heat loss from the system (Ghritlahre et al., Citation2021). It is one of the simplest SAH configurations; however, some additional components such as baffles, fins, etc. can be utilized to increase the performance. Moreover, it is possible to enhance the performance further by applying some ideas such as making use of corrugations on the absorber or utilizing porous materials. In addition, more passages can be used in SAHs in order to split air streams to decrease the system top loss (Goel et al., Citation2021). In Figure , classifications of SAHs based on the configuration and employed components are provided.
Figure 4. Conventional SAH schematic. Adapted from Ghritlahre et al. (Citation2021).

Figure 5. Classifications of SAHs. Adapted from Oztop et al. (Citation2013).

Aside from air, solar heaters are applicable for increasing the temperature of other media such as water, which is another type focused on in this research work. Similar to air, solar beam energy is extracted for increasing the energy content of water. Heating of water can be done directly or indirectly as shown in Figure . In the direct configuration, solar water is circulated in the collector and directly heated up by receiving thermal energy from the collector, while in the indirect type, a heat transfer medium receives heat from the collector and transfers it to water in a heat exchanger. Aside from the heat transfer, other factors affect the classification of SWHs such as the fluid circulation mechanism, active or passive. In active systems, additional components such as pumps are employed for the circulation of water, while in the passive type, circulation is due to buoyancy. Collector systems are other parameters considered in the classification of SWHs. In Figure , the classification of SWHs is represented.
Figure 6. Schematic of (a) direct SWH and (b) indirect SWH. Adapted from Torshizi and Mighani (Citation2017).

Figure 7. Classification of SWH. Adapted from Sarwar et al. (Citation2020).

In both SWHs and SAHs, additional units such as storage components can be used to enhance the output and extend operating hours. Moreover, these systems can be integrated or coupled with other technologies such as auxiliary heaters.
4. Applications of intelligent methods for solar heaters
Solar heaters, as clean technologies, are mainly used in buildings for heating water and space (Liu, Li et al., Citation2015). Similar to other solar systems, there are some complexities in their performance prediction. In addition, different factors are involved in their output that necessitate making use of appropriate methods yielding great exactness of prediction (Calderón-Ramírez et al., Citation2022; Kalogirou et al., Citation1999). Despite the existence of some numerical methods and developed software for modelling these systems, there are some advantages in making use of intelligent methods such as less computational time and cost (Ghritlahre et al., Citation2020a). In this regard, several scholars have applied intelligent methods to generate predictive models for modelling different characteristics of solar heaters.
4.1. Applications of ANNs for modelling solar heaters
Predictive models are applicable for solar heaters and their components with different architectures and configurations. Regarding the dependency of the output of these systems on various elements, these factors must be included in the inputs of the models. One of the most important factors in the output of solar systems is solar radiation. Generally, the output of solar systems increases with solar radiation regarding elevation in the absorbed energy. In addition to solar radiation, there are some other factors depending on the system that influence solar systems output. In this regard, different inputs have been considered in the proposed models for solar heaters. For instance, Bhattacharyya, Sarkar et al. (Citation2021) utilized ANNs to predict the Nusselt number (Nu) and efficiency of helical corrugation with a perforated circular SAH tube using five inputs. The architecture of the models is shown in Figure . In order to propose a model, they utilized experimental data obtained in their previous work (Bhattacharyya et al., Citation2020; Bhattacharyya, Pathak et al., Citation2021). In the experimental study, it was found that the Nu number remarkably enhances with elevation in the Re number, showing the increasing convective heat transfer. In addition, it was shown that the rate of energy transfer increases with decreasing spring and hole ratio. The reason behind this was shown to be swirl/secondary flow and increasing pressure gradient along the radial direction owing to the insertion of spring tape. Moreover, tape with holes induced the development of vortices behind the circular tube that caused elevation in the turbulence and boosted heat transfer (Bhattacharyya et al., Citation2020). In another experimental study (Bhattacharyya, Pathak et al., Citation2021), it was observed that the Nu number increased with the elevation of corrugation angle, while in the case of constant angle of corrugation, the Nu number decreased with the elevation of corrugation pitch ratio. In the case of Nusselt number prediction, the accuracy of training and test sets was 99.90% and 99.96%, respectively, while these values were 99.91% and 99.98% for the mentioned sets, respectively. Souliotis et al. (Citation2009) made use of ANNs to forecast the function of an Integrated Collector Storage (ICS) SWH. The inputs of the created model were ambient temperature, month, speed of wind, total radiation and incidence, and the output was the average temperature of the storage tank. A small deviation was observed between the modelled and the actual average temperature. There are some ideas for modification of solar heaters such as using ribs, fins, baffles, etc. (Saravanakumar et al., Citation2019; Sivakandhan et al., Citation2020; Yadav et al., Citation2022). The performance of solar heaters with these modifications is predictable by intelligent methods. For instance, in a study (Jain et al., Citation2021), experimental data were used for modelling a SAH with a multi-gap V-roughness with staggered elements. The experimental data showed that, at the higher end of Re numbers, the V-geometry with P/e (relative roughness pitch) of 12 provided better thermal efficiency in comparison with the case of P/e’s of 8, 10, 14 and 16, while in cases at the lower end of Re numbers, enhanced thermal performance was noticed for P/e’s of 8 and 10. Three models were based on ANNs by considering relative staggered rib length, relative roughness pitch, the inlet and outlet temperatures of the fluid, and the Reynolds number and average temperature of the plate as the inputs to predict Nusselt number, friction factor and efficiency of a solar heater with staggered elements. R2 values for the training sets of the indicated outputs were 0.999, 0.998 and 0.997, respectively. Chokphoemphun et al. (Citation2020) applied ANNs for forecasting the efficiency, Nusselt number and friction factor of a grooved channel air turbulator heater by considering the type of turbulator, depth ratio, inclination angle and Reynolds number of flow as the inputs. The R2 of the models for Nusselt number, friction factor and efficiency of the system in the optimal case of the models were 0.999,772, 0.998,858 and 0.998,864. In another article (Ghritlahre & Prasad, Citation2018c), an ANN was tested to estimate the heat transfer from the plate of the absorber, which was roughened, to the air flowing via the ducts of the system. The R2 of the created model for the estimation of Nusselt number in their work was 0.995,32 for training sets, demonstrating its satisfactory accuracy. In addition, the forecast date by the ANN model was compared with those calculated by using linear regression and showed a 1.79% higher average of R2. Better performance of intelligent methods compared with linear regression has been observed in other studies. For instance, Shetty et al. (Citation2021) generated two models based on linear regression and ANNs for a solar heater with a circular perforated absorber plate. The R2 of the models based on the regression and ANNs were 0.9751 and 0.9972, respectively. Kalogirou (Citation2000) applied ANNs to estimate the useful energy extracted from an SWH and the temperature rise of water in the storage tank, and the inputs were the average temperatures of cold water and ambient air in addition to diffuse and global solar irradiation at the collector temperature. The R2 of the training sets for the respective outputs were 0.9524 and 0.9644.
Figure 8. Structure of the models used for (a) Nusselt number and (b) efficiency prediction. Adapted from Bhattacharyya, Sarkar et al. (Citation2021).

Aside from the solar heater appropriate for heating a single fluid, i.e. water or air, intelligent methods can be used for systems utilizable for heating more than one fluid. For instance, Yaïci and Entchev (Citation2014) applied an MLP ANN to model a solar thermal system applicable for space heating in addition to domestic hot water. The investigated system was composed of two solar collectors of flat plate type, a storage tank, a propane-fired tank auxiliary energy source, an air handler component and a reservoir of city water. The auxiliary heat source was applied in conditions were the water temperature in the solar storage tank of the solar water was lower than 58°C. The generated model had eight outputs. The performance of the created model was acceptable in predicting the outputs. For instance, stratification temperatures of a preheat water tank were predicted with error less than . These methods could be developed for the prediction of other parameters related to other solar systems usable for both air and water heating systems.
4.2. Applications of other intelligent methods for modelling solar heaters
Aside from MLP ANNs, other intelligent procedures could be used for regression and predictions in different engineering problems and systems (Devi Thangavel et al., Citation2023). These techniques are utilizable for modelling solar heaters or the factors affecting the output of these systems with different configurations and specifications (Ağbulut et al., Citation2021). As an example, H. Esen et al. (Citation2009) applied a Least Squares SVM (LSSVM) to estimate the efficiency of a double-flow SAH. Inputs of the generated model were the temperatures of the air entering and leaving the collector, the temperatures on different points of the plate used as an absorber, T1, T2, T3 and T4 as shown in Figure , and solar radiation. Different kernel functions were used in their model and the predictions were compared with the data determined in the experiments. In conditions using Radial Basis Function (RBF)-kernels, Lin-kernels and Poly-kernels, the R2 values for mass flow rates of 0.03 kg/s and 0.05 kg/s were 0.9997 and 0.9991, 0.9980 and 0.9985, and 0.9990 and 0.9986, respectively. In another work (Liu, Li et al., Citation2015), SVMs, General Regression NNs (GRNNs) and ANNs were employed to forecast the heat loss coefficient and rate of heat collection of water-in-glass evacuated tube type SWHs. The length of tube, number of tubes, distance apart of tube centres, tank heat water mass, area of collector, angle between ground and tube, and final temperature were among the independent variables applied for the modelling. They observed that, for the prediction of the rate of heat collection, the optimal ANN model had the highest accuracy, while for the heat loss coefficient, a GRNN was the best option; however, the training time for SVMs and GRNNs was lower compared with ANNs. Another intelligent method usable for modelling and forecasting is ANFIS, which is based on fuzzy systems (Said, Sundar, et al., Citation2021). This technique could be employed for forecasting the performance of solar heaters or the data influencing the functioning of solar heaters. For example, Rajkumar et al. (Citation2022) made use of ANFIS for the prediction of an SAH with artificial roughness of quatrefoil shape. The applied model showed significantly good performance with an accuracy of 90%. In another study, Mohanty et al. (Citation2017) applied an ANFIS in order to forecast global solar radiation for the economic and energy analysis of solar heaters. For this purpose, the duration of sunshine, humidity and temperature were utilized as inputs, and the clearness index was the output. Six input membership functions including Gaussian, Triangular, bell, two-sided Gaussian, difference sigmoid and trapezoidal were used, while two output membership functions, i.e. linear and constant, were applied. They noted that making use of a Gaussian input function and a linear output function led to better performance, with an MSE of 0.0071.
Figure 9. Schematic of the modelled SAH. Adapted from H. Esen et al. (Citation2009).

4.3. Effects of model characteristics
Characteristics of the models, including the applied function and architecture, are among the parameters that influence the reliability and precision of the predictions. For instance, Ghritlahre and Prasad (Citation2017) utilized an MLP ANN by employing different processing algorithms including conjugate gradient back propagation with Polak–Ribiere Updates (CGP), Scaled Conjugate Gradient back propagation (SCG), One Step Secant back propagation (OSS) and Levenberg–Marquardt back propagation (LM). Their models consisted of four inputs and three outputs as shown in Figure . It was noted that utilization of an LM algorithm led to the creation of the most accurate model with an RMSE of around 3.27, 0.397 and 1.079 for useful heat gain, temperature difference and thermal efficiency, respectively. Similar results have been noted in other studies. For instance, Ghritlahre (Citation2018) tested two algorithms including LM and SCG for estimating the energy and exergy efficiencies of a solar heater with a roughened plate. The inputs of this research were the rate of mass flow, the experiment times, the ambient temperature, the air average temperature, the solar radiation and the temperature of the absorber plate, and the outputs were the energy and exergy efficiencies. It was observed that making use of LM algorithms provided better accuracy than SCG. The R2 of the created model for the exergy and energy efficiencies were 0.957,37 and 0.999,21, respectively. In another work (Ghritlahre & Prasad, Citation2019), several numbers, in the range 10–15, of neurons were applied in the MLP model proposed for the efficiency prediction of solar heaters, both unidirectional and cross flow types. The inputs for generation of the model were time, type of absorber, inlet temperature of fluid, mean temperature of the fluid, mass flow, ambient temperature and solar irradiance. It was observed that making use of 13 neurons causes the generation of a model with the maximum exactness having R2 values of 0.995,24 and 0.997,15 for unidirectional and cross flow types, respectively. The exergetic dimensions of solar heaters have been modelled by these approaches in some other studies. For instance, Saravanakumar et al. (Citation2013) applied ANNs by considering solar intensity, velocity of air and ambient temperature as the inputs, and three outputs including the heater’s first and second law efficiencies and the outlet temperature of collector. The R2’s of the model for all of the mentioned outputs were higher than 0.96, denoting sufficient precision of the estimation.
Figure 10. Model structure for the prediction performance of a solar heater (Ghritlahre & Prasad, Citation2017). © Elsevier. Reproduced by permission of 5497740105102.

The number of inputs and the approach applied can influence a model’s exactness and output precision (Ghritlahre et al., Citation2020a). As an example, Ghritlahre et al. (Citation2020b) made use of Multi-Layer Perceptron (MLP) ANNs and the Group Method of Data Handling (GMDH) to model the thermal efficiency of a solar heater. Among different influential factors, it was found that consideration of the ambient air temperature, the temperature of the inlet air, the mean air temperature, the relative humidity, the plate temperature, the wind velocity, the solar intensity and the mass flow rate in the model led to the highest precision, while adding two other factors, i.e. solar elevation and wind direction, reduced the precision of the model. Furthermore, the closeness of model predictions to the actual quantities demonstrated the better performance of MLP ANNs compared with other approaches. Aidinlou and Nikbakht (Citation2019) tested two types of model based on ANNs for thermohydraulic behaviour modelling of solar heaters. In the first approach, roughened and smooth plates data were forecast separately, while in the second approach, both of them were considered in one stage. For Nusselt number, the minimum values of the MSE of the heaters with smooth and roughened plates were and
, respectively, while this value was
in the case of applying the second method. For the friction factor, the minimum values of MSE under the conditions of consideration for smooth and roughened plates were
and
, respectively, while the MSE was 0.047,68 in the case of using the second approach. In another work (Ghritlahre & Verma, Citation2021), ten different variables, as shown in Figure , were used to estimate a solar heater’s exergy efficiency by making use of three different methods, i.e. MLP ANN, GMDH and MLR. Comparison of the results demonstrated that the best performance among these approaches belonged to MLP ANN. The correlation coefficients (R) of the noted models were 0.999,81, 0.989,77 and 0.976,93, respectively. Ghritlahre and Prasad (Citation2018a) made use of different intelligent methods including MLP, RBF, GRNN and MLR to forecast solar heater thermal performance with a unidirectional flow porous bed. The maximum value of R2 belonged to GRNN, followed by MLP, RBF and MLR, respectively. The higher exactness of ANN-based models could be due to their more complex structure in comparison with the linear regressions. In another study (Ghritlahre & Prasad, Citation2018b), GRNN, MLP and RBF were employed to forecast the exergetic functions of solar heaters with two kinds of absorber plates, i.e. of smooth type and a roughened plate with an arc shaped wire rib. In cases making use of MLPs, the neuron number was varied in range 10–20 and it was found that utilizing 18 neurons led to the maximum precision. For GRNNs, several spread constants were tested and optimal predictions were obtained for cases of a spread constant of 1.5. Finally, in condition of making use of RBF models, a 1–5 spread constant with interval equal to 0.5 was applied. The inputs of the generated models were the intensity of solar radiation, the average temperatures of the plate and fluid, the inlet and ambient air temperatures, the wind speed, the relative humidity and the mass flow rate. Their findings demonstrated the superior performance of RBF models, followed by GRNNs and MLPs.
Figure 11. Schematic of the ANN model for exergy efficiency prediction. Adapted from Ghritlahre and Verma (Citation2021).

In Table , the reviewed studies are summarized.
Table 1. Summary of studies on different types of solar heater.
5. Challenges and limitations
Despite the significant function of intelligent methods in the modelling of solar heaters, there are some challenges and limitations in making use of them. For instance, in order to propose models with high reliability, it is needed to utilize large databases that require experimental work in consideration of different influential variables. Furthermore, the outputs of the models are influenced by the architecture and design of the solar heaters; consequently, it is difficult to generate a model with applicability for different solar heaters. Another challenge is finding all the inputs that can affect the output of the models. For this purpose, considerable knowledge is needed for the developers and generators of the models. Furthermore, it is possible to have some influential qualitative variables that should be converted into relevant quantitative data to be used in the models based on these types of input. Finding the optimal architecture, to have the highest possible precision, is another challenge for some of the machine learning approaches. In order to reach the optimal case, different conditions must be considered, or an appropriate optimization algorithm must be applied.
Overfitting, which prevents the appropriate generalization of models in order to fit observed data on training as well as unobserved data on testing sets, is another challenge that can be faced. There are some approaches to overfitting prevention, such as ‘early-stopping’ by ceasing the training prior to the performance cease the optimize, ‘network-reduction’, which is applied for removing noise in the datasets used for training, ‘data-expansion’, which is introduced for complicated models to tune the hyperparameter sets with a great amount of data, and ‘regularization’, which is applicable by feature-selection and finding more useful and less useful specifications (Decuyper et al., Citation2019).
6. Recommendations
In the previous section, work on solar heater modelling by the utilization of intelligent methods has been reviewed. As represented, some work has been done in this field; however, such studies can be developed further in future. There are some points and tips that can be considered for future work. For example, it would be advantageous to generate more comprehensive models employable for several types of solar heater with various configurations and architectures. In this regard, more inputs must be utilized, i.e. the structure of the system, specifications of the fluids, etc. In addition, it is suggested to consider some of the novel intelligent methods that are obtained by coupling optimization algorithms like Particle Swarm Optimization (PSO) (Noushabadi et al., Citation2020) and Genetic Algorithms (GAs) (Alarifi et al., Citation2019b). These optimization algorithms are usable to find the optimal hyperparameters of the intelligent methods to reach the minimum error. In addition, it is suggested to consider solar heaters integrated with storage materials for future modelling studies regarding the benefits of the utilization of storage materials for solar systems (Pachori et al., Citation2022; SunilRaj & Eswaramoorthy, Citation2023). In this regard, the properties of storage materials can be considered as additional input factors. Aside from the storage material, the employment of nanofluids in the collectors of solar heaters can enhance the output (Janardhana et al., Citation2022; Sivasubramanian et al., Citation2022). It could be beneficial to develop some models for solar heaters using nanofluids. Similar to systems integrated with storage materials, the properties of applied nanofluids such as the concentration and specifications of the nanomaterials could be considered as inputs (Maleki et al., Citation2021). Since solar heaters are integrable with other energy technologies such as thermoelectric generators (Chargui et al., Citation2022) and geothermal systems (Qin et al., Citation2021), it could be suggested to generate models by using intelligent methods for these types of system.
Moreover, since the performance of various intelligent techniques depends on different factors, it is useful to consider influential parameters to obtain better models. For instance, in intelligent methods coupled with optimization algorithms, the optimization method can influence the precision of the proposed model. As an example, in a study by Ramezanizadeh, Ahmadi, Ahmadi et al. (Citation2019) on the dynamic viscosity estimation of Al2O3/water nanofluid, different optimization algorithms, namely GAs, PSO, Hybrid GA PSO (HGAPSO) and Imperialist Competitive Algorithms (ICAs) were used in LSSVM methods. Their findings showed that making use of GAs led to the maximum value of the correlation coefficient among the applied algorithms. In another study (Alarifi et al., Citation2019a), the performance of PSO-ANFISs and GA-ANFISs in the modelling of the thermophysical properties of a hybrid nanofluid was compared, and it was pointed out that the utilization of PSO as optimization algorithm can lead to better performance. It seems that the performance of optimization algorithms and their selection based on the highest accuracy of the generated model are dependent on the problem and applied modelling method. In MLP ANNs, different training and transfer functions can be used that can affect the outputs of the model and their closeness to the actual data. For instance, in a study by M.M. Rashidi et al. (Citation2023) on the thermal conductivity of some nanofluids, Tan-sigmoid and radial basis functions were applied in an MLP ANN and it was reported that utilization of radial basis functions can provide a model with higher accuracy for their case study. Similar to the optimization algorithm, selection of the best function can be case-dependent.
7. Conclusion
Intelligent techniques are usable for theperformance forecasting of various kinds of solar heaters. The key conclusions are summarized as follows.
Intelligent procedures are applicable for performance forecasting of both air and water heaters.
Intelligent methods are utilizable for modelling different characteristics of solar heaters including Nusselt number, friction factor, outlet temperature, etc.
Weather data and working conditions are among the main factors included as the inputs of intelligent methods.
In comparison with linear regression, intelligent techniques provide higher prediction exactness.
The precision of the developed models depends on some elements such as model architecture and the functions used.
Aside from the structure and function, the inputs impact the exactness of the models.
Nomenclature
a | = | Input |
b | = | Input |
bj | = | Function tendency |
C | = | Coefficient of polynomial |
ek | = | Looseness parameter |
u | = | Combination of output linear layer |
W | = | Weight of neuron |
Xi | = | ith input |
Y | = | Output |
Greek letters
= | Activation function | |
= | Margin parameter | |
= | Bandwidth |
Abbreviations
ANN | = | Artificial Neural Network |
ANFIS | = | Adaptive Neuro Fuzzy Inference System |
GMDH | = | Group Method of Data Handling |
GRNN | = | General Regression Neural Network |
ICA | = | Imperialist Competitive Algorithm |
LSSVM | = | Least Square Support Vector Machine |
MARS | = | Multivariate Adaptive Regression Spline |
MLP | = | Multilayer Perceptron |
MLR | = | Multiple Linear Regression |
MSE | = | Mean Squared Error |
RBF | = | Radial Basis Function |
SAH | = | Solar Air Heater |
SWH | = | Solar Water Heater |
Disclosure statement
No potential conflict of interest was reported by the authors.
Additional information
Funding
References
- Abujazar, M. S. S., Fatihah, S., Ibrahim, I. A., Kabeel, A. E., & Sharil, S. (2018). Productivity modelling of a developed inclined stepped solar still system based on actual performance and using a cascaded forward neural network model. Journal of Cleaner Production, 170(January), Elsevier: 147–159. https://doi.org/10.1016/J.JCLEPRO.2017.09.092
- Afshari, F., Sözen, A., Khanlari, A., Doğuş Tuncer, A., & Şirin, C. (2020). Effect of turbulator modifications on the thermal performance of cost-effective alternative solar Air heater. Renewable Energy, 158(October), Pergamon: 297–310. https://doi.org/10.1016/J.RENENE.2020.05.148
- Ağbulut, Ü., Gürel, A. E., & Biçen, Y. (2021). Prediction of daily global solar radiation using different machine learning algorithms: Evaluation and comparison. Renewable and Sustainable Energy Reviews, 135(January), Pergamon: 110114. https://doi.org/10.1016/J.RSER.2020.110114
- Ahmadi, M. A., & Mahmoudi, B. (2016). Development of robust model to estimate Gas–Oil interfacial tension using least square support vector machine: Experimental and modeling study. The Journal of Supercritical Fluids, 107(January), Elsevier: 122–128. https://doi.org/10.1016/J.SUPFLU.2015.08.012
- Ahmadi, M. H., Sadeghzadeh, M., Raffiee, A. H., & Chau, K.-W. (2019). Applying GMDH neural network to estimate the thermal resistance and thermal conductivity of pulsating heat pipes. Engineering Applications of Computational Fluid Mechanics, 13(1), 327–336. https://doi.org/10.1080/19942060.2019.1582109
- Ahmed, A., Ge, T., Peng, J., Yan, W. C., Tee, B. T., & You, S. (2022). Assessment of the renewable energy generation towards Net-zero energy buildings: A review. Energy and Buildings, 256(February), Elsevier: 111755. https://doi.org/10.1016/J.ENBUILD.2021.111755
- Aidinlou, H. R., & Nikbakht, A. M. (2019). Intelligent modeling of thermohydraulic behavior in solar Air heaters with artificial neural networks. Neural Computing and Applications, 31(8), Springer: 3279–3293. https://doi.org/10.1007/S00521-017-3269-0
- Akhbari, M., Rahimi, A., & Hatamipour, M. S. (2020). Modeling and experimental study of a triangular channel solar Air heater. Applied Thermal Engineering, 170(April), Pergamon: 114902. https://doi.org/10.1016/J.APPLTHERMALENG.2020.114902
- Alarifi, I. M., Nguyen, H. M., Bakhtiyari, A. N., & Asadi, A. (2019a). Feasibility of ANFIS-PSO and ANFIS-GA models in predicting thermophysical properties of Al2O3-MWCNT/Oil hybrid nanofluid. Materials, 12(21), 3628. https://doi.org/10.3390/ma12213628
- Alarifi, I. M., Nguyen, H. M., Bakhtiyari, A. N., & Asadi, A. (2019b). Feasibility of ANFIS-PSO and ANFIS-GA models in predicting thermophysical properties of Al2O3-MWCNT/Oil hybrid nanofluid. Materials, 12(21), MDPI AG: 3628. https://doi.org/10.3390/ma12213628
- Al-Askaree, E. H., & Al-Muhsen, N. F. O. (2023). Experimental investigation on thermal performance of solar water heater equipped with serpentine Fin core heat exchanger. Cleaner Engineering and Technology, 12(February), Elsevier: 100593. https://doi.org/10.1016/J.CLET.2022.100593
- Alotaibi, S., Amooie, M. A., Ahmadi, M. H., Nabipour, N., & Chau, K. w. (2020). Modeling thermal conductivity of ethylene glycol-based nanofluids using multivariate adaptive regression splines and group method of data handling artificial neural network. Engineering Applications of Computational Fluid Mechanics, 14(1), Taylor & Francis: 379–390. https://doi.org/10.1080/19942060.2020.1715843
- Baig, W., & Ali, H. M. (2019). An experimental investigation of performance of a double pass solar Air heater with foam aluminum thermal storage medium. Case Studies in Thermal Engineering, 14(September), Elsevier: 100440. https://doi.org/10.1016/J.CSITE.2019.100440
- Bhattacharyya, S., Hari Raghavendran, B., & Paul, A. R. (2020). The effect of circular hole spring tape on the turbulent heat transfer and entropy analysis in a heat exchanger tube: An experimental study. Taylor & Francis, 1–20. https://doi.org/10.1080/08916152.2020.1787560.
- Bhattacharyya, S., Pathak, M., Sharifpur, M., Chamoli, S., & Ewim, D. R. E. (2021). Heat transfer and exergy analysis of solar Air heater tube with helical corrugation and perforated circular disc inserts. Journal of Thermal Analysis and Calorimetry, 145(3), Springer Science and Business Media B.V.: 1019–1034. https://doi.org/10.1007/S10973-020-10215-X/METRICS
- Bhattacharyya, S., Sarkar, D., Roy, R., Chakraborty, S., Goel, V., & Almatrafi, E. (2021). Application of New artificial neural network to predict heat transfer and thermal performance of a solar Air-heater tube. Sustainability, 13(13), Multidisciplinary Digital Publishing Institute: 7477. https://doi.org/10.3390/SU13137477
- Blázquez, C. S., Borge-Diez, D., Nieto, I. M., Martín, A. F., & González-Aguilera, D. (2021). Multi-Parametric evaluation of electrical, biogas and natural Gas geothermal source heat pumps. Renewable Energy, 163(January), Pergamon: 1682–1691. https://doi.org/10.1016/J.RENENE.2020.10.080
- Calderón-Ramírez, M., Gomez-Náfate, J. A., Ríos-Fuentes, B., Rico-Martínez, R., Martínez-Nolazco, J. J., & Botello-Álvarez, J. E. (2022). Analysis of the thermal efficiency of flat plate solar Air heaters considering environmental conditions using artificial neural networks. Revista Mexicana de Ingeniería Química, 21(3), 1–3. https://doi.org/10.24275/RMIQ/SIM2833
- Chargui, R., Bechir, N., & Tashtoush, B. (2022). A novel hybrid solar water heater system integrated with thermoelectric generators: Experimental and numerical analysis. Journal of Cleaner Production, 368(September), Elsevier: 133119. https://doi.org/10.1016/J.JCLEPRO.2022.133119
- Choi, J., Kang, B., & Cho, H. (2014). Performance comparison between R22 and R744 solar-geothermal hybrid heat pumps according to heat source conditions. Renewable Energy, 71(November), Pergamon: 414–424. https://doi.org/10.1016/J.RENENE.2014.05.057
- Chokphoemphun, S., Hongkong, S., Thongdaeng, S., & Chokphoemphun, S. (2020). Experimental study and neural networks prediction on thermal performance assessment of grooved channel Air heater. International Journal of Heat and Mass Transfer, 163(December), Pergamon: 120397. https://doi.org/10.1016/J.IJHEATMASSTRANSFER.2020.120397
- Decuyper, M., Stockhoff, M., Vandenberghe, S., & Ying, X. (2019). An overview of overfitting and Its solutions. Journal of Physics: Conference Series, 1168(2), IOP Publishing: 022022. https://doi.org/10.1088/1742-6596/1168/2/022022
- Devi Thangavel, K., Seerengasamy, U., Palaniappan, S., & Sekar, R. (2023). Prediction of factors for controlling of green house farming with fuzzy based multiclass support vector machine. Alexandria Engineering Journal, 62(January), Elsevier: 279–289. https://doi.org/10.1016/J.AEJ.2022.07.016
- Engel, E., & Engel, N. (2022). A review on machine learning applications for solar plants. Sensors, 22(23), Multidisciplinary Digital Publishing Institute: 9060. https://doi.org/10.3390/S22239060
- Esen, H., Ozgen, F., Esen, M., & Sengur, A. (2009). Modelling of a New solar Air heater through least-squares support vector machines. Expert Systems with Applications, 36(7), Pergamon: 10673–10682. https://doi.org/10.1016/J.ESWA.2009.02.045
- Fang, G., Chan, M. y., Yan, H., Chen, W., Deng, S., & Liu, X. (2020). An experimental study on the operating performances of a novel Bed-based Air source heat pump (B-ASHP) system. Energy and Buildings, 223(September), Elsevier: 110191. https://doi.org/10.1016/J.ENBUILD.2020.110191
- Ghalandari, M., Shahrestani, M. I., Maleki, A., Shadloo, M. S., & Assad, M. E. H. (2021). Applications of intelligent methods in various types of heat exchangers: A review. Journal of Thermal Analysis and Calorimetry, 145(4), Springer Science and Business Media B.V.: 1837–1848. https://doi.org/10.1007/S10973-020-10425-3/METRICS
- Ghritlahre, H. K. (2018). Development of feed-forward back-propagation neural model to predict the energy and exergy analysis of solar Air heater. Trends in Renewable Energy, 4(2), Future Energy Service and Publishing: 213–235. https://doi.org/10.17737/TRE.2018.4.2.0078
- Ghritlahre, H. K., Chandrakar, P., & Ahmad, A. (2020a). Solar Air heater performance prediction using artificial neural network technique with relevant input variables. Archives of Thermodynamics, 41(3), Polska Akademia Nauk: 255–282. https://doi.org/10.24425/ATHER.2020.134579
- Ghritlahre, H. K., Chandrakar, P., & Ahmad, A. (2020b). Application of ANN model to predict the performance of solar Air heater using relevant input parameters. Sustainable Energy Technologies and Assessments, 40(August), Elsevier: 100764. https://doi.org/10.1016/J.SETA.2020.100764
- Ghritlahre, H. K., Chandrakar, P., & Ahmad, A. (2021). A comprehensive review on performance prediction of solar air heaters using artificial neural network. Annals of Data Science, 8(3), 405–449. Springer Science and Business Media Deutschland GmbH. https://doi.org/10.1007/s40745-019-00236-1.
- Ghritlahre, H. K., & Prasad, R. K. (2017). Prediction of thermal performance of unidirectional flow porous Bed solar Air heater with optimal training function using artificial neural network. Energy Procedia, 109(March), Elsevier: 369–376. https://doi.org/10.1016/J.EGYPRO.2017.03.033
- Ghritlahre, H. K., & Prasad, R. K. (2018a). Investigation of thermal performance of unidirectional flow porous Bed solar Air heater using MLP, GRNN, and RBF models of ANN technique. Thermal Science and Engineering Progress, 6(June), Elsevier: 226–235. https://doi.org/10.1016/J.TSEP.2018.04.006
- Ghritlahre, H. K., & Prasad, R. K. (2018b). Exergetic performance prediction of solar Air heater using MLP, GRNN and RBF models of artificial neural network technique. Journal of Environmental Management, 223(October), Academic Press: 566–575. https://doi.org/10.1016/J.JENVMAN.2018.06.033
- Ghritlahre, H. K., & Prasad, R. K. (2018c). Prediction of heat transfer of Two different types of roughened solar Air heater using artificial neural network technique. Thermal Science and Engineering Progress, 8(December), Elsevier: 145–153. https://doi.org/10.1016/J.TSEP.2018.08.014
- Ghritlahre, H. K., & Prasad, R. K. (2019). Modelling of back propagation neural network to predict the thermal performance of porous Bed solar Air heater. Archives of Thermodynamics, 40(4), Polish Academy of Sciences: 103–128. https://doi.org/10.24425/ATHER.2019.131430
- Ghritlahre, H. K., & Verma, M. (2021). Accurate prediction of exergetic efficiency of solar Air heaters using various predicting methods. Journal of Cleaner Production, 288(March), Elsevier: 125115. https://doi.org/10.1016/J.JCLEPRO.2020.125115
- Goel, V., Hans, V. S., Singh, S., Kumar, R., Pathak, S. K., Singla, M., Bhattacharyya, S., Almatrafi, E., Gill, R. S., & Saini, R. P. (2021). A comprehensive study on the progressive development and applications of solar Air heaters. Solar Energy, 229(November), Elsevier Ltd: 112–147. https://doi.org/10.1016/j.solener.2021.07.040
- Goodson, T., Briens, F., Camarasa, C., Delmastro, C., Staas, L., & Petrichenko, K. (2022). Heating. https://www.iea.org/reports/heating.
- Gunasekaran, N., Manoj Kumar, P., Raja, S., Sharavanan, S., Avinas, K., Aakash Kannan, P., & Gokul, S. (2021). Investigation on ETC solar water heater using twisted tape inserts. Materials Today: Proceedings, 47(January), Elsevier: 5011–5016. https://doi.org/10.1016/J.MATPR.2021.04.586
- Hassan, H., Abo-Elfadl, S., & El-Dosoky, M. F. (2020). An experimental investigation of the performance of New design of solar Air heater (tubular). Renewable Energy, 151(May), Pergamon: 1055–1066. https://doi.org/10.1016/J.RENENE.2019.11.112
- Jain, P. K., Lanjewar, A., Jain, R., & Rana, K. B. (2021). Performance analysis of multi-Gap V-roughness with staggered elements of solar Air heater based on artificial neural network and experimental investigations. Environmental Science and Pollution Research, 28(25), Springer: 32905–32920. https://doi.org/10.1007/S11356-021-12875-0
- Janardhana, K., Sivakumar, A., Gladson, G. J. N., Ramesh, C., Syed Musthafa, A., & Gopinathan, R. (2022). Study on the performance of a flat plate solar water heater using a hybrid nanofluid. Materials Today: Proceedings, 69, 1145–1149. August. Elsevier. https://doi.org/10.1016/J.MATPR.2022.08.181.
- Jouybari, N. F., & Staffan Lundström, T. (2020). Performance improvement of a solar Air heater by covering the absorber plate with a thin porous material. Energy, 190(January), Pergamon: 116437. https://doi.org/10.1016/J.ENERGY.2019.116437
- Kalogirou, S. A. (2000). Performance prediction of a solar water heater using artificial neural networks. World Renewable Energy Congress VI, January. Pergamon, 2163–2166. https://doi.org/10.1016/B978-008043865-8/50465-7.
- Kalogirou, S. A., Panteliou, S., & Dentsoras, A. (1999). Artificial neural networks used for the performance prediction of a thermosiphon solar water heater. Renewable Energy, 18(1), Pergamon: 87–99. https://doi.org/10.1016/S0960-1481(98)00787-3
- Kashyap, T., Thakur, R., Sethi, M., Tripathi, R. K., Thakur, A., Kumar, S., Kumar, P., Goel, B., & Chand, S. (2023). The influence of reflector on the thermal performance of single and double pass solar Air heater: A comprehensive review. Materials Today: Proceedings, 72, 1260–1269. September. Elsevier. https://doi.org/10.1016/J.MATPR.2022.09.297.
- khosrojerdi, S., Vakili, M., Yahyaei, M., & Kalhor, K. (2016). Thermal conductivity modeling of graphene nanoplatelets/deionized water nanofluid by MLP neural network and theoretical modeling using experimental results. International Communications in Heat and Mass Transfer, 74, Elsevier B.V.: 11–17. https://doi.org/10.1016/j.icheatmasstransfer.2016.03.010
- Li, H., Liu, Z., Liu, K., & Zhang, Z. (2017). Predictive power of machine learning for optimizing solar water heater performance: The potential application of high-throughput screening. International Journal of Photoenergy, 2017, Hindawi Limited, 1–10. https://doi.org/10.1155/2017/4194251
- Liu, Z., Li, H., Zhang, X., Jin, G., & Cheng, K. (2015). Novel method for measuring the heat collection rate and heat loss coefficient of water-in-glass evacuated tube solar water heaters based on artificial neural networks and support vector machine. Energies, 8(8), Multidisciplinary Digital Publishing Institute: 8814–8834. https://doi.org/10.3390/EN8088814
- Mahmood, A., & Wang, J. L. (2021). Machine learning for high performance organic solar cells: Current scenario and future prospects. Energy & Environmental Science, 14(1), Royal Society of Chemistry: 90–105. https://doi.org/10.1039/D0EE02838J
- Maleki, A., Haghighi, A., & Mahariq, I. (2021). Machine learning-based approaches for modeling thermophysical properties of hybrid nanofluids: A comprehensive review. Journal of Molecular Liquids, Elsevier B.V. 322, 114843. https://doi.org/10.1016/j.molliq.2020.114843.
- Mohanty, S., Rout, A., Patra, P. K., & Sahoo, S. S. (2017). ANFIS based solar radiation data forecasting for energy & economic study of solar water heaters in eastern India. International Journal of Control Theory and Applications, 10(April), 179–190.
- Nazari, M., Jafarmadar, S., & Khalilarya, S. (2022). Exergy and thermoeconomic analyses of serpentine tube flat-plate solar water heaters coated with CuO nanostructures. Case Studies in Thermal Engineering, 35(July), Elsevier: 102072. https://doi.org/10.1016/J.CSITE.2022.102072
- Noushabadi, A. S., Dashti, A., Raji, M., Zarei, A., & Mohammadi, A. H. (2020). Estimation of cetane numbers of biodiesel and diesel oils using regression and PSO-ANFIS models. Renewable Energy, 158(October), Pergamon: 465–473. https://doi.org/10.1016/J.RENENE.2020.04.146
- Oztop, H. F., Bayrak, F., & Hepbasli, A. (2013). Energetic and exergetic aspects of solar Air heating (solar collector) systems. Renewable and Sustainable Energy Reviews, 21(May), Pergamon: 59–83. https://doi.org/10.1016/J.RSER.2012.12.019
- Pachori, H., Choudhary, T., & Sheorey, T. (2022). Significance of thermal energy storage material in solar Air heaters. Materials Today: Proceedings, 56(January), Elsevier: 126–134. https://doi.org/10.1016/J.MATPR.2021.12.516
- Patel, R., Kulkarni, K. G., Havaldar, S. N., & Deshmukh, V. N. (2023). A review on the factors that decrease the performance of a solar water heater. Materials Today: Proceedings, 72, 3068–3074. September. Elsevier. https://doi.org/10.1016/J.MATPR.2022.09.064.
- Qin, D., Liu, J., & Zhang, G. (2021). A novel solar-geothermal system integrated with earth–to–Air heat exchanger and solar Air heater with phase change material—numerical modelling, experimental calibration and parametrical analysis. Journal of Building Engineering, 35(March), Elsevier: 101971. https://doi.org/10.1016/J.JOBE.2020.101971
- Rajkumar, P., Raja, M., Stephen Sagayaraj, A., Melvin Jones, J., Suseendhar, P., & Karthiga, M. (2022). Performance enhancement & prediction of solar Air heater with quatrefoil shaped artificial roughness using adaptive neuro fuzzy inference system. November. Institute of Electrical and Electronics Engineers (IEEE), 1579–1583. https://doi.org/10.1109/ICECAA55415.2022.9936587
- Ramezanizadeh, M., Ahmadi, M. A., Ahmadi, M. H., & Alhuyi Nazari, M. (2019). Rigorous smart model for predicting dynamic viscosity of Al2O3/water nanofluid. Journal of Thermal Analysis and Calorimetry, 137(1), 307–316. https://doi.org/10.1007/s10973-018-7916-1
- Ramezanizadeh, M., Ahmadi, M. H., Nazari, M. A., Sadeghzadeh, M., & Chen, L. (2019). A review on the utilized machine learning approaches for modeling the dynamic viscosity of nanofluids. Renewable and Sustainable Energy Reviews, 114(October), Pergamon: 109345. https://doi.org/10.1016/J.RSER.2019.109345
- Ramezanizadeh, M., Nazari, M. A., Ahmadi, M. H., & Açıkkalp, E. (2018). Application of nanofluids in thermosyphons: A review. Journal of Molecular Liquids, 272(December), Elsevier: 395–402. https://doi.org/10.1016/J.MOLLIQ.2018.09.101
- Rashidi, M. M., Alhuyi Nazari, M., Mahariq, I., & Ali, N. (2023). Modeling and sensitivity analysis of thermal conductivity of ethylene glycol-water based nanofluids with alumina nanoparticles. Experimental Techniques, 2022, March. Springer: 83–90. https://doi.org/10.1007/S40799-022-00567-4
- Rashidi, M. M., Nazari, M. A., Mahariq, I., Assad, M. E. H., Ali, M. E., Almuzaiqer, R., Nuhait, A., & Murshid, N. (2021). Thermophysical properties of hybrid nanofluids and the proposed models: An updated comprehensive study. Nanomaterials, 11(11), Multidisciplinary Digital Publishing Institute: 3084. https://doi.org/10.3390/NANO11113084
- Rashidi, S., Karimi, N., & Yan, W. M. (2022). Applications of machine learning techniques in performance evaluation of solar desalination systems – A concise review. Engineering Analysis with Boundary Elements, 144(November), Elsevier: 399–408. https://doi.org/10.1016/J.ENGANABOUND.2022.08.031
- Razavi, R., Sabaghmoghadam, A., Bemani, A., Baghban, A., Chau, K. w., & Salwana, E. (2019). Application of ANFIS and LSSVM strategies for estimating thermal conductivity enhancement of metal and metal oxide based nanofluids. Engineering Applications of Computational Fluid Mechanics, 13(1), Taylor & Francis: 560–578. https://doi.org/10.1080/19942060.2019.1620130
- Rizvi, Z. H., Husain, S. M. B., Haider, H., & Wuttke, F. (2020). Effective thermal conductivity of sands estimated by group method of data handling (GMDH). Materials Today: Proceedings, 26(January), Elsevier: 2103–2107. https://doi.org/10.1016/J.MATPR.2020.02.454
- Said, Z., Ghodbane, M., Tiwari, A. K., Ali, H. M., Boumeddane, B., & Ali, Z. M. (2021). 4E (energy, exergy, economic, and environment) examination of a small LFR solar water heater: An experimental and numerical study. Case Studies in Thermal Engineering, 27(October), Elsevier: 101277. https://doi.org/10.1016/J.CSITE.2021.101277
- Said, Z., Syam Sundar, L., Rezk, H., Nassef, A. M., Ali, H. M., & Sheikholeslami, M. (2021). Optimizing density, dynamic viscosity, thermal conductivity and specific heat of a hybrid nanofluid obtained experimentally via ANFIS-based model and modern optimization. Journal of Molecular Liquids, 321(January), Elsevier B.V.: 114287. https://doi.org/10.1016/j.molliq.2020.114287
- Sajawal, M., Ur Rehman, T., Ali, H. M., Sajjad, U., Raza, A., & Bhatti, M. S. (2019). Experimental thermal performance analysis of finned tube-phase change material based double pass solar Air heater. Case Studies in Thermal Engineering, 15(November), Elsevier: 100543. https://doi.org/10.1016/J.CSITE.2019.100543
- Saravanakumar, P. T., Mayilsamy, K., & Boopathi Sabareesh, V. (2013). ANN modeling of forced convection solar Air heater. International Review on Modelling and Simulations, 6(6), Praise Worthy Prize S.r.l: 1955–1960. https://doi.org/10.1109/ICCTET.2013.6675911
- Saravanakumar, P. T., Somasundaram, D., & Matheswaran, M. M. (2019). Thermal and thermo-hydraulic analysis of Arc shaped Rib roughened solar Air heater integrated with fins and baffles. Solar Energy, 180(March), Pergamon: 360–371. https://doi.org/10.1016/J.SOLENER.2019.01.036
- Sarwar, J., Khan, M. R., Rehan, M., Asim, M., & Kazim, A. H. (2020). Performance analysis of a flat plate collector to achieve a fixed outlet temperature under semi-arid climatic conditions. Solar Energy, 207(September), Elsevier Ltd: 503–516. https://doi.org/10.1016/j.solener.2020.06.088
- Self, S. J., Reddy, B. V., & Rosen, M. A. (2013). Geothermal heat pump systems: Status review and comparison with other heating options. Applied Energy, 101(January), Elsevier: 341–348. https://doi.org/10.1016/J.APENERGY.2012.01.048
- Shetty, S. P., Nayak, S., Kumar, S., & Vasudeva Karanth, K. (2021). Thermo-Hydraulic performance prediction of a solar Air heater with circular perforated absorber plate using artificial neural network. Thermal Science and Engineering Progress, 23(June), Elsevier: 100886. https://doi.org/10.1016/J.TSEP.2021.100886
- Shetty, S. P., Paineni, A., Kande, M., Madhwesh, N., Yagnesh Sharma, N., & Vasudeva Karanth, K. (2020). Experimental investigations on a cross flow solar Air heater having perforated circular absorber plate for thermal performance augmentation. Solar Energy, 197(February), Pergamon: 254–265. https://doi.org/10.1016/J.SOLENER.2020.01.005
- Sivakandhan, C., Arjunan, T. V., & Matheswaran, M. M. (2020). Thermohydraulic performance enhancement of a New hybrid duct solar Air heater with inclined Rib roughness. Renewable Energy, 147(March), Pergamon: 2345–2357. https://doi.org/10.1016/J.RENENE.2019.10.007
- Sivasubramanian, R., Kumaresan, G., Santhosh, N., Reshvanth, K. S., Syed Riyasudeen, S., & Shannon Medone, R. (2022). Performance analysis of multipass tubes solar water heater using nanofluids. Materials Today: Proceedings, 66(January), Elsevier: 1051–1055. https://doi.org/10.1016/J.MATPR.2022.04.838
- Sohani, A., Hoseinzadeh, S., Samiezadeh, S., & Verhaert, I. (2022). Machine learning prediction approach for dynamic performance modeling of an enhanced solar still desalination system. Journal of Thermal Analysis and Calorimetry, 2021(April), Springer: 1–12. https://doi.org/10.1007/S10973-021-10744-Z
- Soltani, M., Kashkooli, F. M., Dehghani-Sanij, A. R., Kazemi, A. R., Bordbar, N., Farshchi, M. J., Elmi, M., Gharali, K., & Dusseault, M. B. (2019). A comprehensive study of geothermal heating and cooling systems. Sustainable Cities and Society, 44(January), Elsevier: 793–818. https://doi.org/10.1016/J.SCS.2018.09.036
- Souliotis, M., Kalogirou, S., & Tripanagnostopoulos, Y. (2009). Modelling of an ICS solar water heater using artificial neural networks and TRNSYS. Renewable Energy, 34(5), Pergamon: 1333–1339. https://doi.org/10.1016/J.RENENE.2008.09.007
- Sridharan, M. (2020). Application of generalized regression neural network in predicting the performance of natural convection solar dryer. Journal of Solar Energy Engineering, Transactions of the ASME, 142(3). American Society of Mechanical Engineers (ASME). https://doi.org/10.1115/1.4045384/1066328.
- SunilRaj, B. A., & Eswaramoorthy, M. (2023). Performance study on forced convection mode V-trough solar air heater with thermal storage: An experimental approach. Materials Today: Proceedings, September. Elsevier. 72, 1664–1672. https://doi.org/10.1016/J.MATPR.2022.09.450.
- Thangavelu, S. K., Khoo, R. J., & Piraiarasi, C. (2021). Exergy and exergoeconomic analysis of domestic scale solar water heater by the effect of solar collector area. Materials Today: Proceedings, 47(January), Elsevier: 5004–5010. https://doi.org/10.1016/J.MATPR.2021.04.584
- Torshizi, M. V., & Mighani, A. H. (2017). The application of solar energy in agricultural systems. Renewable Energy and Sustainable Development, 3(2), Arab Academy for Science, Technology, and Maritime Transport (AASTMT): 234–240. https://doi.org/10.21622/resd.2017.03.2.234
- Tripathy, P. P., & Kumar, S. (2009). Neural network approach for food temperature prediction during solar drying. International Journal of Thermal Sciences, 48(7), Elsevier Masson: 1452–1459. https://doi.org/10.1016/J.IJTHERMALSCI.2008.11.014
- Voyant, C., Notton, G., Kalogirou, S., Nivet, M. L., Paoli, C., Motte, F., & Fouilloy, A. (2017). Machine learning methods for solar radiation forecasting: A review. Renewable Energy, 105(May), Pergamon: 569–582. https://doi.org/10.1016/J.RENENE.2016.12.095
- Wang, D., Liu, J., Liu, Y., Wang, Y., Li, B., & Liu, J. (2020). Evaluation of the performance of an improved solar air heater with “S” shaped ribs with gap. Solar Energy, 195(January), Pergamon: 89–101. https://doi.org/10.1016/J.SOLENER.2019.11.034
- Wang, Y., Kandeal, A. W., Swidan, A., Sharshir, S. W., Abdelaziz, G. B., Halim, M. A., Kabeel, A. E., & Yang, N. (2021). Prediction of tubular solar still performance by machine learning integrated with Bayesian optimization algorithm. Applied Thermal Engineering, 184(February), Pergamon: 116233. https://doi.org/10.1016/J.APPLTHERMALENG.2020.116233
- Yadav, A. S., Gupta, S., Agrawal, A., Saxena, R., Agrawal, N., & Nashine, S. (2022). Performance enhancement of solar Air heater by attaching artificial Rib roughness on the absorber plate. Materials Today: Proceedings, 63(January), Elsevier: 706–717. https://doi.org/10.1016/J.MATPR.2022.05.064
- Yaïci, W., & Entchev, E. (2014). Performance prediction of a solar thermal energy system using artificial neural networks. Applied Thermal Engineering, 73(1), Pergamon: 1348–1359. https://doi.org/10.1016/J.APPLTHERMALENG.2014.07.040