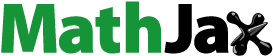
Abstract
Spherical nano-microcarriers generally perform targeted delivery of drugs to blood vessels with lesions. Recently, some studies have shown the importance of the non-spherical nature of nano-microcarriers in their movement toward the target wall inside blood arteries. Non-spherical particles tend to collide and adhere to the target wall due to their high interaction level with the artery wall. However, detailed kinematic studies have yet to be carried out to optimize nano-microcarriers’ shape based on their delivery rate to the target wall inside the artery. In addition, it is important to note that the flow of fluid in vessels can have diverse patterns that may affect the movement of drug carriers having different shapes. It is therefore necessary to study the impact of fluid flow patterns on drug carriers, refine their shapes and sizes to achieve the desired drug delivery rate, and reduce the risk of toxicity in blood vessels that have disorders. In the present study, we tried to provide optimal delivery of different forms of nano-microcarriers to the abdominal aortic aneurysm (AAA) wall, which is a common and dangerous lesion in the artery, based on the rate of particle impact on the inner wall of the AAA and the rate of their exit from the downstream of the aorta artery. The results showed that the non-spherical shape strongly depends on the interaction of the particles on the inner wall of the AAA and their departure rate from the aorta artery. Different shapes with different shape factors have optimal delivery conditions compared to the size of each nano-microcarrier in the drug delivery aimed at the wall.
1. Introduction
Abdominal Aortic Aneurysm (AAA) is located in the lower part of the aorta. If its walls are weakened, it swells and protrudes (Huynh & Miller, Citation2022). Aneurysm enlargement causes its rupture, internal bleeding, and the death of the patient, and annually causes the death of more than 200,000 people worldwide (Huang et al., Citation2022; Wang et al., Citation2023). Aneurysms generally grow slowly and are very complex and irreversible, and their symptoms are difficult to diagnose. Stenoses constitute a significant problem as they cause a decrease in flow and can worsen the reduced blood supply to the affected tissue or organ, which differs based on the blood rheology of individuals. According to Awad et al. (Citation2022), a study was conducted on a flexible tube with a catheter and narrow thrombosis. According to the study, the flow rate decreased because of hydraulic loss, and the resistance was raised due to the power-law index (n) increase in the non-Newtonian model used for blood. As a result, the flow rate decreased even more. Ghalambaz et al. conducted a study (Ghalambaz et al., Citation2021) using carbon nanotubes to enhance temperature characteristics that influence blood flow during peristaltic flow. They examined how increasing clot size affects flow rate and reduces surface shear stress on the clot. Saleem, Akhtar, Alharbi, et al. (Citation2020) thoroughly investigated the impact of slight constriction of a vessel on the wall. Their conclusions revealed that the wall's resistance to fluid likewise increases with an increase in the height of stenosis. Another study (Saleem, Akhtar, Nadeem, et al., Citation2020) examined how the curvature of a path affects two blood-based nano-fluids in peristaltic flow. The study revealed that curved tubes can lead to uneven trapping of boluses. Geometric curvature is another critical parameter in the investigation of stenoses and aneurysms, which has been investigated in the research of Moawad et al. (Citation2022). By examining different geometries in the subclavian artery, they found that the wall shear stress is higher in the curved geometry compared to the straight state, in addition to the rheological characteristics. Also, they claimed that the stress on the stenosis or aneurysm in the convergent type of artery geometry would be much larger than in the divergent one. The treatment of this disease depends on the size of the aneurysm and how dangerous it is. In case of a high risk of aneurysm rupture, specialists use invasive open surgery and non-invasive surgical methods such as endovascular (He et al., Citation2022; Lee et al., Citation2023). One of the other methods in treatment and control is administering the appropriate drug dose, which causes the inflamed vessels to relax and blood pressure to decrease. Suitable and advanced drugs for control are metformin and statins (Li et al., Citation2023). Targeted drug delivery with the help of carriers is a new method that, in addition to the high efficiency due to the appropriate contact of the drug with the inflamed area, can decrease the risks caused by surgery (Shamloo, Ebrahimi, et al., Citation2022).
The effect of the shapes and dimensions of drug carriers on their performance has been reported in several studies (Cooley et al., Citation2018; Hinde et al., Citation2017; Shachar-Berman et al., Citation2019; Shachar-Berman et al., Citation2020). In recent years researchers have been following the methods of making such nano-microparticles for drug delivery applications, besides tissue engineering, and medical Imaging. Hinde et al. (Citation2017) have shown the effect of the drug-carrier shape on their penetration inside the cells as well as the drug delivery. They have demonstrated that drug-carriers with a higher aspect ratio (such as rod and worm) have more penetration into the cell's nucleus than drug-carriers with spherical shapes (such as vesicle and micelle), which has a better effect on the delivery of cancer drugs such as Doxorubicin. Michaela Cooley et al. (Citation2018) investigated the effect of drug-carrier shape and size on adhesion and margination to the wall of the veins. After performing software calculations for the relevant experiment, they shaped fluorescent particles of polystyrene using thermo-stretching operations in various forms (spherical, oblate, prolate, and rod). Then they coated the receptor and ligand corresponding to the particles and the microfluidic channel (representing the vessel) to compare the parameters mentioned. They found that microparticles with larger sizes and non-spherical shapes compared to spherical and nanoparticles, have higher adhesion and migrate more toward the wall. Their results showed that the highest amount of adhesion is related to oblate or elliptical particles. Ruysschaert (Citation2009) investigated HMSNS-PEG-FA nanoparticles with a core of and synthesized them in capsule, cube, rice, and rhombus forms. Their results proved that this type of nanocarrier has a high specific surface area and shows a higher cellular uptake and a greater rate of Doxorubicin drug release in HeLa cells (especially for rhombus-like shapes (Shachar-Berman et al., Citation2019)). In the numerical research conducted by Shachar-Berman et al. (Citation2019), the amount of dispersion and deposition of spherical and non-spherical particles (ellipses with different aspect ratios) in the limited areas of pulmonary alveoli (acinus) were compared to each other in two normal and deep breathing models. In this report, it was claimed that for nanocarriers (in the range where the equivalent diameter is more than 1–2 micrometers), increasing the aspect ratio (AR) and decreasing the equivalent diameter causes their dispersion to increase (Shachar-Berman et al., Citation2020).
Among all the methods for investigating fluidic parameters, computational fluid dynamics (CFD) has a significant role in cardiovascular studies (Sun et al., Citation2022) and is an exemplary tool for predicting behaviour in situations where technical challenges preclude the use of experimental tests. Due to the intricate turbulent nature (Lan et al., Citation2020) of flow behaviour within stenoses or aneurysms, which include flow swirling and/or velocity increase, it is imperative to utilize precise models for their computational analysis. Various methods are available for the computational analysis of turbulent flows, such as direct numerical solution (DNS), Large Eddy Simulation (LES) (Manchester et al., Citation2022), and widely used Reynolds Averaged Navier-Stokes Equations (RANS) (Vali et al., Citation2019). The choice of method depends on specific requirements and has a significant impact on the accuracy and efficiency of the analysis results. In their study, Zhan et al. (Citation2022) used a model called scale adaptive simulation (SAS) along with the PISO (Pressure Implicit with Splitting of Operators) method to simulate the turbulent flow in AAA. Ma et al. (Citation2022) employed LES and DNS techniques to forecast hemodynamics in a stented aortic coarctation. They found that LBM-based LES was a successful method for simulating aortic flow, verified by experimental 4D-Flow MRI outcomes.
Our previous studies have focused on simulations and modelling of various biological phenomena (Pedram et al., Citation2016; Shamloo et al., Citation2017; Yousefsani et al., Citation2018; Shamloo, Manuchehrfar, et al., Citation2015; Shamloo, Mohammadaliha, et al., Citation2015). Shamloo et al. (Citation2020) measured the amount of microbubbles adhered to the AAA wall under blood flow conditions in a cardiac cycle and the effect of parameters of size and density of MBs as well as the mechanical properties of blood and arterial wall. Ebrahimi et al. (Citation2021) studied the interaction of liposome and solid particles with the wall of the AAA and the adhesion of ligand receptors of nanoparticles to the lumen of the AAA. They showed that by increasing the size of nanoparticles from 400 to 600 nm, the number of nanoparticles adhered to the AAA wall increased by 10.3%. By applying a magnetic field to the microbubbles Shamloo et al. (Citation2022) found that the amount of magnetic microbubbles adhered to the AAA wall increases significantly compared to the condition without the magnetic field. Shamloo et al. (Citation2022) showed in another work, by modelling the ultrasound force in the AAA geometry, the superiority of ultrasound forces over the volume forces and the guidance of microbubbles to the wall of the AAA lumen and the increase of the adhesion of microbubbles ligands to the receptors.
Certain limitations were observed in previous studies, as the fact that the fluid flow in vessels and channels can have varying patterns, which can impact the movement of drug carriers with different shapes, was not taken into consideration. Therefore, investigating the effects of fluid flow patterns on drug carriers, optimizing their shapes and sizes based on the desired drug delivery rate, and minimizing the risk of toxicity in blood vessels with disorders are crucial. Thorough understanding of the interactions between drug carriers and fluid flow, as well as the physical properties of the drug carriers themselves, is required for achieving these goals. Advanced models should be developed to overcome these limitations and enable more efficient and safer drug delivery systems. Additionally, exploring the potential of new geometries for drug carriers could further enhance their performance and broaden their applicability in various medical fields.
The present study investigates the effect of the spherical and non-spherical geometry of drug carriers in their different sizes on the targeted delivery of drugs to the inner wall of AAA. Therefore, the AAA geometry of a patient-specific was reconstructed through CT scan images. Then it was modelled to analyse the fluid flow and track the drug-carrying particles with the individual's physiological conditions (pulsatile inlet pressure and velocity). Modelling was done based on particles’ spherical and non-spherical shapes in nano and micro-dimensions, which were analysed for different shape factors. Finally, optimization was evaluated to deliver targeted drug carriers to the AAA based on the number of particles hitting the internal area of the AAA and the number of particles exiting the artery downstream.
2. Materials and methods
2.1. Model geometry
Aneurysm geometry is very effective in blood flow velocity and dynamic movement of nano-microparticles. For this purpose, the special patient geometry model has been used in this research. Firstly, a CT scan image in DCIOM format of a 52-year-old patient’s aneurysm was prepared from Tehran Heart Center. With the help of image processing software, a virtual 3D model of AAA geometry was reconstructed (Biglari et al., Citation2019). Capillaries and extra vessels were removed in CATIA software. AAA geometry has an average thickness of 4 mm. The diameter of the aneurysm is 40 mm, and the limited area of the aneurysm is 377.4 . The lumen areas in branching are equal to 189.7 and 163.8
(Figure (A)).
2.2. Numerical modelling
A transient pressure-based solver was implemented using the SIMPLE scheme solution method to solve nonlinear equations. It uses a relationship between velocity and pressure corrections to enforce mass conservation and generate the solution data by obtaining the pressure field in a time-saving manner. Different methods can be used for the discretization of the convective term. The decision as to which method to select is based on the Reynolds number. If the Reynolds number is higher, it is better to use the upwind scheme. Second-order upwind finite differences combined with a multigrid solver provide an efficient method for solving the steady Navier- Stokes equations in primitive variables with large Reynolds numbers.
For time discretization in numerical simulations, an implicit integration approach was utilized due to the potential lack of realism in solutions for large time frames without careful formulation. Additionally, implicit methods with larger time steps can improve accuracy while reducing computational load. However, implementing these methods may pose challenges due to additional computational steps. Nevertheless, implicit methods are commonly used in practical applications because many problems are classified as stiff. The fully implicit scheme offers the benefit of unconditional stability regardless of the time step size (Mohammadian & Pourmehran, Citation2019; Rashidi et al., Citation2017; Shachar-Berman et al., Citation2020).
Since in this simulation, our complex flow is not aligned with the mesh and the majority of mesh elements are tetrahedral, second order upwind spatial discretization scheme was implemented for momentum, turbulent kinetic energy, turbulent dissipation rate, and energy equations and second order was implemented pressure equation. Least squares cell-based method is also used for gradient equations in the spatial discretization part to yield more accurate results. Time step size was valued and checked by Courant number criteria. Since the maximum velocity in the domain is captured 0.3 (m/s) and the average of velocity is 0.116 (m/s), the characteristic length was considered 3 (mm) and time-step size was set to 0.01 (s) to put the Courant number lower than 1. Using the outcomes of sensitivity analyses performed on the time step, the time step was set 0.001 (s) for unsteady particle tracking (Tsuji et al., Citation1993), and each time step was considered convergent when the residual was less than 1 × 10−5. Consecutively, generated data was saved in each time-step and imported in Tecplot 360 EX 2021 R2 to capture different contours as a post-processing procedure and plotting graphs.
2.2.1. Blood property
Blood flow modelling in AAA geometry was done numerically in a computational software. In this simulation, the blood fluid is considered non-Newtonian and incompressible. Although blood is considered a Newtonian fluid with specific density and viscosity in a wide range of flows, and this assumption has been widely accepted in many AAA models, we consider the blood as a non-Newtonian fluid in some circumstances. Turbulence effects, turbulence transition, and pulsatile blood velocity with negative values, which cause small vortex structures have enormous effects on the physiologically important flow quantities, the mathematical formulation, and solution methodology of the arterial blood flow (Zhan et al., Citation2022). Furthermore, since blood is a suspension of cells, it behaves like a non-Newtonian fluid when passing through narrow blood vessels and at low shear rates in abnormal flow states, such as in the presence of stenosis, aneurysm, and thrombosis (Sankar & Hemalatha, Citation2007).
Previous studies (Ebrahimi, Vatani, et al., Citation2021; Forouzandehmehr & Shamloo, Citation2018; Kwon, Citation2008) on blood rheology show that dynamic viscosity is a function of blood shear rate, and there is a non-linear relationship between blood shear rate and blood shear stress. Due to the critical issues explained here, the current study utilizes the Carreau–Yasuda equation to model blood viscosity, considering the non-linear geometry and the fluctuations in shear rate caused by pulsating physiological velocity waveforms as follows (Kwon, Citation2008).
(1)
(1) where
and
are the viscosity of fluid with infinite shear and the viscosity of fluid without shear, respectively.
displays the time constant,
is the local shear rate, and
is the power-law index. These values are extracted from previous articles
= 0.00345 [pa.s],
= 0.161 [pa.s],
= 39.418 [s], and
= 0.479 (Kwon, Citation2008) (Table ).
Table 1. Some mechanical and rheological properties in the simulation.
2.2.2. Turbulence flow
The geometry of AAA and the effects caused by the curvature of the artery walls causes internal flow, adverse pressure gradients, and separation. Therefore, the hydrodynamic movement of fluid in real models is in the form of turbulence flow. In order to model turbulent flows and the effects of velocity fluctuations in the blood, shear stress transport turbulent modelling was used. The combined shear stress transport model satisfies the continuity and momentum equations by considering the high shear stress of the fluid in the vicinity of walls, low shears in the free stream, and their mutual effects on drug delivery and reaching the carriers to the inflamed area. The following equations show the conservation of mass and momentum in three directions (Burmasheva & Prosviryakov, Citation2022).
(2a)
(2a)
(2b)
(2b) In the (Equation 2b),
is the blood density, and
is the fluid velocity which is a function of heart rate. The thermodynamic pressure, the volume forces, and the shear stress tensor are
,
, and
, respectively (Burmasheva & Prosviryakov, Citation2022). The shear stress tensor is dependent on fluid movement and is a function of strain rate (
) and Kronecker delta (
). The Equation 3a until Equation 3b are defined as follows (Lovato et al., Citation2022). Note that
is Lame’s coefficient.
(3a)
(3a)
(3b)
(3b)
(3c)
(3c)
The Equation (4) governing the turbulence kinetic energy () and specific dissipation rate (
) in k-omega turbulent modelling are:
(4a)
(4a)
(4b)
(4b)
(4c)
(4c)
The turbulence-kinetic-energy Equation (4a) contains no special compressibility terms involving pressure work, diffusion, or dilatation. Although a dilatation–dissipation modification to the k equation improves compressible mixing-layer predictions, the same modification has a detrimental effect on shock-separated-flow predictions. Hence, it is omitted from the equation for general applications. Note that the turbulent-diffusion terms in Equation (4a) and Equation (4b) (i.e. the terms proportional to
and
) are proportional to
rather than to the eddy viscosity. This means that the only terms in these equations that are implicitly affected by the stress limiter are the production terms (via the Reynolds-stress tensor).
2.2.3. Closure coefficients
The various closure coefficients appearing in the k-omega model are ,
,
and
.
(5a)
(5a)
(5b)
(5b)
(5c)
(5c)
(5d)
(5d)
(5f)
(5f)
Note that is the rotation tensor and
is mean vorticity.
is the strain-rate tensor.
is Galilean-invariant Favre-averaged strain-rate tensor (Wilcox, Citation2008).
The geometry walls were assumed to be rigid, and no-slip boundary condition was considered for the walls (Table ). Moreover, the boundary conditions at the vessel's entrance and outlets were applied in the form of physical zone conditions by applying the pulsatile velocity inlet and pressure outlet boundary conditions compatible with profiles of three cardiac cycles (Figure (B)).
Table 2. Boundary conditions.
2.3. Forces acting on the drug carriers
Generally, two types of volume and surface forces are applied to drug carrier particles floating in the bloodstream. Volumetric force, gravity acting on unit mass (), and since the patient lies horizontally on the bed to perform the treatment process, this force exerts on the AAA geometry perpendicular to the blood flow, which is calculated by finite volume analysis. The surface forces acting on particles include Brownian force (
), particle-particle interaction force (
), lift force (
), and drag force (
) (Yin et al., Citation2003). As a result of the pressure gradient perpendicular to the blood flow direction, the lift force is created. The drag force is applied to the particles against the direction of the flow velocity and depends on the shape and surface of the particle.
Discrete Phase Modelling (DPM) was used to simulate the blood fluid from the Eulerian point of view and to track the drug-carrying particles in the continuous phase framework from the Lagrangian point of view and the interaction of the two phases with each other. So, the exchange of mass and momentum is possible with the balance of internal forces. According to Newton’s second law, the movement path of particles can be predicted (Zastawny et al., Citation2012).
(6)
(6) In the Equation (6), the velocity and density of a particle are
and
, respectively. In the solving process of hydrodynamic movement, the blood fluid is diluted, and the particles have a concentration with a volume fraction of less than 1%. All numerical models for discrete phase modelling have discrepancies between real conditions and simulations to such different extents. The sensitivity of these forces exerted on the particles must be analysed accurately to capture their effects on the exact particle movements and path lines, and a comparison with experimental data will boost the accuracy of the simulated results. For example, the Brownian and Saffman's lift forces should be considered only for nanoparticles (Mohammadian & Pourmehran, Citation2019). The virtual mass is disregarded in this study since the fluid density and particle density are almost the same, but the pressure gradient force was considered for the particles’ motion in the fluid flow, where pulsatile pressure variations can significantly affect the particle behaviour. The Magnus force, the lift generated by particle rotation exerted from fluid, is also considered since the particles in blood flow rotate in some vortices and rotational flows in AAA, but reversely the effect of the Magnus lift force on the fluid from particles is not considered in DPM-coupled simulations. For another discrepancy between reality and the numerical study, we can mention that according to the files capturing the location, number, and time that particles collided with the artery's wall, some particles are trapped in some spots and detected by the software several times in a very short period. However, in real conditions, the first contact between the particle and the wall suffices for the drug delivery and release of their drug content. Even though the AAA wall's mechanical properties make it flexible in real conditions to behave as a viscoelastic material so that energy transmitted is both stored and dissipated due to elastic and viscous components of arterial walls, we set the AAA wall as a rigid body with no-slip condition and the displacement and the variation of the internal and external radius in each pulse did not affect the drug delivery performance or particles motion which is widely accepted in AAA and aortic system research (Kang et al., Citation2021; Khanafer et al., Citation2007).
2.4. Turbulent dispersion effect
The movement of particles could be determined using the average flow without considering the impact of turbulent flow, on the other side the dispersion of small particles is greatly affected by turbulence in the flow (Yan et al., Citation2020). In this study we used a stochastic approach called the discrete random walk model (DRWM) that was studied by previous researchers (Dufresne et al., Citation2009; Vosswinkel et al., Citation2012) to consider the turbulent dispersion effect and instantaneous turbulent velocity fluctuations on particle trajectories. We occupied the CL constant, which represents the integral time scale, and found that the particle trajectory was significantly affected by turbulence if the CL value was large. Each eddy is characterized by a Gaussian distributed random velocity fluctuation u’, v’ and w’. Velocity fluctuations are expressed in Equation (7), where ζ is a normal distributed random number while k is the turbulent kinetic energy.
(7)
(7) In the given model, the particle represents a group of physical particles (parcel) with similar properties. However, the model's total number of injected particles may not be the same as the real-world conditions. A summary of solution methods and models is given in Table .
Table 3. Solution methods and models.
2.5. Particle tracking for fluid flow
During blood circulation, the impact of nano-microparticles with the aneurysm area is very important for drug delivery and drug contents release. In this simulation, to investigate the effect of the shape and size of drug-carrying nano-microparticles on their adhesion to the aneurysm area, nano-microparticles with different values of shape factors 1, 0.8, 0.7, 0.5, and 0.3 were investigated. Particles are randomly released in the blood at time intervals of 0.6, 1.6, and 2.6 s according to the blood velocity profile (Forouzandehmehr & Shamloo, Citation2018). The particle density was considered 1000 . The motion of nano-microparticles in the artery is influenced by different forces and rotational velocities that can result in surface tension on the particles. However, as encounters low levels of force and rotation, this impact is considered insignificant and has been excluded from our examination (Ghodousi et al., Citation2018). Since the angle of impact of the particle on the surface is equal to the reflect angle, calculating the probability of a particle from the surface of a tangential plane is 0.5 (Shamloo et al., Citation2020).
(8a)
(8a)
(8b)
(8b) Therefore, the position vector of the particle before contact and after contact is equal to
and
. The normal surface vector is
. Particle velocity vector before contact is
and after contact is
. Particle is reflected heterogeneously, so the velocity vector from the reflection surface can be obtained from Knudsen’s cosine (Alishiri et al., Citation2021; Ebrahimi, Shamloo, et al., Citation2021). According to the law of Cosines, the particle’s position in three dimensions is calculated according to the following Equation (9).
(9a)
(9a)
(9b)
(9b)
(9c)
(9c)
Reflect tangential velocities are and
, the instantaneous velocity of the particle hitting the surface is
, and normal velocity is
. The Angle (
) is considered between the range of 0–2
randomly; also, the angle (
) is obtained from the following equation (Shamloo et al., Citation2019).
(10)
(10) The probability distribution of the normal component of the velocity that is called
has the above sinusoidal relationship with the angle of
. This component is a random number. The differential equations governing the physics of the problem are based on the turbulence flow and obey the non-linear, unstable finite volume. The second-order method in tracking the particles and the time solver is used. For validation of our modelling, we used two previous experimental studies, which are given with details in the supplementary.
2.6. Mesh configuration and convergence analysis
An unstructured mesh was employed to discretize the geometry domain for the current abdominal aortic aneurysm model. The model was tested for four different grid numbers, i.e. 508,000, 755,000, 1,000,000, and 5,170,000 cells (Table ). Comparing the depicted velocity profiles (Supplementary Fig. S3) revealed less than a 1.66% difference in velocity magnitude on outlets between 1,000,000 and 5,170,000 grids. Thereby, we adopted the scheme that contained 1,000,000 grids.
Table 4. Mesh refinement study in the simulation.
Since the fluid flow in this geometry is complex and the results are sensitive to the regional turbulence effects, it is necessary to consider the sublayer and near-wall grid resolution to refine the mesh sufficiently. We generated a refined non-uniform grid with high smoothness quality near the wall. The mesh comprises five prism/wedge layers with an aspect ratio 1.2 near the wall. Tri/tetrahedral and pyramid computational cells were applied to discretize other geometry regions. Furthermore, to capture the viscous sublayer effects, turbulent boundary layer, and shear stresses of blood adjacent to the wall of the artery, the first layer height of the mesh was considered 0.1 mm (in the worst-case scenario) to reach a y + between 1 and 5, small enough for turbulent k-omega (SST) model.
Mesh elements quality was checked, and its metrics namely skewness, orthogonal quality, aspect ratio, and element quality, were calculated regarding cell number and type, have been shown Supplementary Fig. S4. Minimum orthogonal quality is reported as 1e-01, and 65% of the elements have a proper aspect ratio of 2.27, and the rest have less than 5, which minimize the numerical errors. The skewness of more than 72% of the elements was between 0 and 0.38. Overall, the important mesh quality factor had an average number of 0.858, which was close to an ideal number of 1 and demonstrated the high quality of the grid.
The local mesh size was set to 3 mm; also, the minimum curvature size and normal angle were established at 0.03 mm and 18 degrees, respectively, and the proximity minimum size was considered 0.03 mm to refine the mesh in some apex points like the artery bifurcation. The complete illustration of the discretized domain is provided for various mesh element sizes in the Figure . With this cell size criteria, it can be ensured that the DPM assumptions are met since the volume fraction of particles is estimated to be less than 10% in each cell, with particles counted as the dispersed phase. Thus, there are limitations in grid size, and if the grids become too small, the DPM gets grid dependent and needs to be studied.
3. Results and discussions
3.1. Blood flow behaviour
The key factor in the movement of particles and the forces acting on them, especially in their different shapes, is the pattern of blood flow inside the AAA. The stre amlines of blood flow velocity with the direction of the fluid flow, at the time (the beginning of the cardiac cycle),
(the first peak of the inlet velocity), and
(the minimum of the inlet velocity) is shown in Figures . Furthermore, a two-dimensional plot representing streamlines along with the magnitude of blood velocity for the three regions, inlet, middle, and outlet of AAA is given in Figures . In all cases, it can be seen that near the walls, the fluid flow velocity is close to zero, and in the middle of the artery, it has the highest value.
Figure 3. The streamlines of blood flow velocity with the direction of the fluid flow, at the time (the beginning of the cardiac cycle). A two-dimensional plot representing streamlines along with the magnitude of blood velocity for the three regions, inlet, middle, and outlet of AAA.
![Figure 3. The streamlines of blood flow velocity with the direction of the fluid flow, at the time t=2.65[s] (the beginning of the cardiac cycle). A two-dimensional plot representing streamlines along with the magnitude of blood velocity for the three regions, inlet, middle, and outlet of AAA.](/cms/asset/c71625ba-b3e0-4b77-9b1f-6d6f1db94d50/tcfm_a_2236166_f0003_oc.jpg)
Figure 4. The streamlines of blood flow velocity with the direction of the fluid flow, at the time (the first peak of the inlet velocity). A two-dimensional plot representing streamlines along with the magnitude of blood velocity for the three regions, inlet, middle, and outlet of AAA.
![Figure 4. The streamlines of blood flow velocity with the direction of the fluid flow, at the time t=2.97[s] (the first peak of the inlet velocity). A two-dimensional plot representing streamlines along with the magnitude of blood velocity for the three regions, inlet, middle, and outlet of AAA.](/cms/asset/9e8df9b7-15d0-4ee2-8435-18afb46143c1/tcfm_a_2236166_f0004_oc.jpg)
Figure 5. The streamlines of blood flow velocity with the direction of the fluid flow, at the time (the minimum of the inlet velocity). A two-dimensional plot representing streamlines along with the magnitude of blood velocity for the three regions, inlet, middle, and outlet of AAA.
![Figure 5. The streamlines of blood flow velocity with the direction of the fluid flow, at the time t=3.34[s] (the minimum of the inlet velocity). A two-dimensional plot representing streamlines along with the magnitude of blood velocity for the three regions, inlet, middle, and outlet of AAA.](/cms/asset/9324e58d-380e-4347-be10-e2180908e423/tcfm_a_2236166_f0005_oc.jpg)
At the beginning of the cardiac cycle time (), it can be seen that the magnitude of blood velocity in the AAA area has the lowest value compared to the other two states,
,
. Moreover, according to the two-dimensional Figure , it can be seen that in all the three regions, inlet, middle, and outlet of AAA, flow velocity vortices are formed. For the middle of AAA, the vortex is formed on the right side of AAA, and for the state of
, it can be seen that the Womersley profile is created at the beginning of the AAA. This profile is created due to the influence and increase of fluid flow velocity. It has also been observed that the flow velocity in the right side has the lowest in the middle region of AAA. The vortex formed in the inlet and middle region of the AAA is evident at the
. These vortices are due to the reduction of the inlet flow rate, and also, the vortex formed in the middle of the AAA is seen clearly in the right area of the AAA, which has a lower magnetude of blood velocity. The formation of vortices and the blood flow pattern inside the AAA have also been observed in previous numerical studies (Arzani & Shadden, Citation2012; Les et al., Citation2010; Shamloo et al., Citation2020), which are highly consistent with the present simulation.
3.2. Position of particles
In the present simulation, solid particles with different dimensions (at nano scales: ,
,
, and
and at micro scales
,
,
, and
) have been used with
particles entering the AAA per cardiac cycle. The position of spherical particles inside the AAA at three times,
,
, and
are given in Figure . It can be seen that before the third cycle, in which the third release of particles begins, the concentration of particles in the AAA region is higher than in the two bifurcations downstream of the aorta and the AAA entrance region. It can also be seen that inside the AAA, the concentration of particles is higher at the in front of the aneurysm bulge than in other parts of the AAA. The leading cause of this particle congestion is the fluid flow pattern inside it. As mentioned in the previous sections, the magnitude of blood velocity is the lowest compared to other parts of the aorta artery, which allows the particles to exist in that area. Furthermore, the vortex formed in the lower right area causes the rotation of those particles (as shown in Figure ), which increases the particles residence time in that area. This rotation under the effect of vortex dramatically increases the probability of particles hitting the wall, and it even causes the particles to hit the walls repeatedly. As the collision of particles increases, the probability of particles sticking to the target wall increases. Because if the ligand fails to attach to its receptor at first collision, this connection may occur in the following impacts. The drug-carrying particles generally stick to the AAA wall with the ligand–receptor connection, and adhesion of the drug-carrying particles depends on the way the particles hit the inner wall and the amount of shear stress and the ligands type coated on the particles.
3.3. The delivery efficiency based on the number of particles interaction
Another critical parameter in how particles move under the influence of fluid flow is their geometric shape. The drag and lift forces are necessary and effective in how particles move in AAA, depending on the particles’ geometric shape. Investigating the movement path of particles and the number of particles collisions in their different shapes can be very important in the optimal design of drug carrier particles. In this study, we investigated the number of particle interaction to the AAA wall in various shape factors and dimensions of nanoparticles and microparticles shown in Figure (A,B), respectively. According to Figure (A), for nanoparticles in shape factors of and 0
, particles with a diameter of
nm have the highest impacting rate with the AAA wall. The effect of the shape factor on the particles interaction with the inner wall of AAA is such that for nanoparticles with a diameter of
, the impact rate value is
higher for particles with shape factor of
than
. However, for nanoparticles with a diameter of
nm and shape factors of
and
, the number of particle interaction is the highest compared to other sizes. In addition, it can be seen that for nanoparticles with a diameter of
nm, a shape factor of
; for nanoparticles with a diameter of
nm, a shape factor of
; and for nanoparticles with a diameter of
nm, a shape factor of
has the highest amount of interaction with the AAA wall geometry. This issue can show the importance of the shape of the nanoparticles in drug delivery to the target wall inside the AAA, which should be considered in the design of drug-carrying nanoparticles.
Figure 7. The number of particle interaction to the AAA wall in various shape factors and dimensions of (A) nanoparticles and (B) microparticles.

For microparticles, as shown in Figure (B), it can be seen that the maximum number of microparticles interaction is at a shape factor of for a diameter of
micrometers. For a diameter of
, 3, and 4 micrometer, a shape factor of
,
, and
, respectively, has better performance in delivering the drug towards the inner wall of AAA in comparison with other shape factors in the same microparticle diameter.
A decrease in the number of spherical nanoparticles (shape factor ) between
,
,
,
nm diameters has been observed in our previous study (Ebrahimi, Vatani, et al., Citation2021) on the effect of interaction of spherical drug carriers (liposome). However, in the present study, a turbulence model was used to simulate the blood flow inside the AAA; the number of released particles in AAA is different compared to the previous study. In addition, the inlet fluid flow velocity of the AAA is considered different in the previous study from the present study.
In the current study, a patient-specific geometry of AAA has been investigated in order to investigate the effects of shape factors in the delivery of drug-carrying particles to the AAA wall. Although we observed in our previous study (Ebrahimi, Vatani, et al., Citation2021) that the change in the AAA geometry changes the number of the particles interaction with the AAA wall, it did not affect on the trend of these changings concerning the diameters of the particles. Because the flow pattern inside the AAA is the same in all AAA geometries, due to the increase in the diameter of the artery in the aneurysm area, which decreases the flow velocity and forms vortices in that area. Therefore, the present simulation results are also valid for all other AAA geometries.
3.4. The delivery efficiency based on the number of particles exiting from AAA
Another important parameter in the design of drug-carrying particles to deliver to the inner wall of the AAA is the rate of their transfer to the downstream of the aorta artery, which, if it exceeds a specific dosage, will cause toxicity and dysfunction of the downstream arteries. Here, we investigated the number of particles transported downstream of the artery. The number of particles exiting the downstream area in the bifurcation outlets for particles in nanometer and micrometer dimensions is shown in Figure (A,B), respectively. For nanoparticles, according to Figure (A), it can be seen that the lowest number of nanoparticles exiting from AAA belongs to particles with a diameter of nm and a shape factor of
. For the shape factors of
and
, particles with a diameter of
nm have the lowest output. For a shape factor of
, particles in diameters of
,
, and
nm have the lowest amount of AAA outlet, and in shape factor 1, particles with a
nm diameter also have the lowest value. The results for nanoparticles show that the more particles hit the wall, the fewer particles leave the aorta and more tend to stay in the area. Therefore, it can be concluded that nanoparticles with a high interaction number in each shape factor have the lowest outlet from the AAA region, which can significantly decrease toxicity.
Figure 8. The number of particles exiting the downstream area in the bifurcation outlets for particles in (A) nanometer and (B) micrometer dimensions.

In the microparticles sample, according to Figure (B), it can be seen that in the diameters of micrometer, particles with shape factors of
,
, and
have the lowest outlet of microparticles from the branch of the abdominal aorta. Nonetheless, microparticles with shape factors of
,
, and diameters of
and
micrometer, respectively, have the highest outlet of microparticles from AAA, which can produce low toxicity in the patient's body. According to the number of microparticles interaction to the inner wall of AAA (Figure (B)), the highest number collisions are in different shape factors that can be used as drug carriers.
3.5. Optimizing nano-microparticles for efficient drug delivery to AAA wall
Optimizing the geometric shapes of nano-microparticles for efficient drug delivery has significant practical implications for various medical applications. The effect of shape and dimensions of drug carriers on their performance has been extensively studied in recent years, including their application in drug delivery, tissue engineering, and medical imaging (Cooley et al., Citation2018; Hinde et al., Citation2017; Shachar-Berman et al., Citation2019). The results of these studies have shown that the shape of drug carriers significantly affects drug delivery efficiency and penetration into cells. These findings highlight the potential for optimizing the shape and size of drug carriers to enhance drug delivery rates while reducing the risk of toxicity in blood vessels with disorders.
The present study has demonstrated that the shapes of drug carriers in the micrometer range significantly affect drug delivery to the AAA wall. Our simulation results have identified various geometric shapes, including spheres, cylinders, cylinders with spherical heads, ellipses, oval cylinders, and octahedral, that can be utilized to design drug carriers and optimize their manufacture for efficient drug delivery. The geometrical parameters of these shapes are presented in Tables and for nanoparticles and microparticles, respectively.
Table 5. Geometric shapes that can be designed include spheres, cylinders, cylinders with spherical heads, ellipses, oval cylinders, and octahedral with geometrical parameters for nanoparticles.
Table 6. Geometric shapes that can be designed include spheres, cylinders, cylinders with spherical heads, ellipses, oval cylinders, and octahedral with geometrical parameters for microparticles.
The practical implications of our findings are significant. The optimized nano-microparticles can be utilized in a variety of drug delivery systems, such as solid nano-microparticles, liposomes, lysosomes, microbubbles, and metal–organic frameworks, to enhance drug delivery to the AAA wall. This has important implications for the treatment of AAA, which is a serious and potentially life-threatening condition.
3.6. Limitations and future works
DPM provides an acceptable approximation of particle interaction; nevertheless, due to the limitation of experimentation, acquiring information about this interaction is challenging. Therefore, these methods have been widely used in industry and academia for many years and have been shown to produce valuable results in various applications. Additionally, the DEM can be combined with computational fluid dynamics (CFD) to depict fluid and particle interaction systems. Regarding accuracy in particle interactions and travelling path lines in DPM and DEM, calibration and validation against various experiments can accuarate these modelling approaches and numerical errors. The simulation provides a thorough and precise outcome, but it does not exactly replicate real-world scenarios. Moreover, the DPM overlooks interactions between particles, which is reasonable in situations involving sparse particle transportation. However, when dealing with multi-phased systems that involve dense particles, using DPM may result in significant errors that are unacceptable (Yan et al., Citation2020).
Two factors can explain the difference in results between experiments and simulations. Initially, the numerical methods employed in the regions near the wall of obtained CT-Scan geometries would diverge. As a result, it is necessary to smooth these regions by designing software to carry out simulations. The simulations assume a perfectly smooth surface, while microscale roughness exists on actual surfaces, leading to inexactitudes in near-wall interactions. Secondly, when dealing with complex geometries that exhibit significant pressure gradients, flow separation, and recirculatory effects, slight errors may arise due to using RANS turbulence models (Kalitzin et al., Citation2005; Mohammadian & Pourmehran, Citation2019). In summary, although there are some critical issues due to the limitations of these modellings, this study acknowledges the drawbacks and limitations and shows the results consistent with previous research supporting the validity of our findings. Since the main purpose of this study is to count the number of nano-microparticles colliding with the artery’s wall, highlighting details of particle-particle or wall-particle interactions was not considered. On the other hand, when analysing the flow of particles that are not very dense, the DPM approach provides a satisfactory representation of calculations. However, using the DEM method will enhance the reliability of simulation results.
Recently, CFD-DEM has gained popularity as the go-to approach to examining fluid-particle interaction systems due to its computational benefits compared to DNS-DEM, LBM-DEM, SPH-DEM, and PPM-DEM. Moreover, CFD-DEM excels at capturing microscopic details related to particles compared to the two-fluid model (TFM) (Zhou et al., Citation2019). Researchers have extensively used the CFD-DEM model to investigate complex problems involving continuous and discontinuous elements in various fields. These include fluidization, pneumatic conveying, blast furnace operations, hydro cyclone operations (Tang et al., Citation2018), saturated granular characteristics (El Shamy & Zeghal, Citation2007), hopper flow, and piling processes in water (Zhao & Shan, Citation2013), hydraulic structure stability (Dai et al., Citation2019; El Shamy & Aydin, Citation2008; Mao et al., Citation2018), and sand production (Climent et al., Citation2014).
As another limitation of the current study, it is recognized that the geometry and boundary conditions of blood vessels can vary depending on the type and location of the complication, thus making it difficult to examine all complications in a single study. However, it is acknowledged that the methods utilized in this study could be applied in the future to investigate the effect of nano-microcarrier size and shape on other types of blood vessels, such as coronary, carotid, and cerebral blood vessels with aneurysm and stenosis complications, thus providing valuable insights into the potential of targeted drug delivery for treating a wider range of vascular complications.
4. Conclusions
In the present study, the delivery of solid particles to the inner wall of the AAA, in different sizes of nanometers and micrometers in different shapes, has been investigated. Modelling based on finite volume method on the Patient-Specific geometry of AAA by applying boundary conditions close to reality (pulsatile velocity and pressure of blood) was performed considering non-Newtonian blood based on the Carreau Yasuda model and turbulence model. Finally, based on the number of particles hitting the internal wall of the AAA, the number of particles exiting the bifurcations of the abdominal aorta has been obtained to investigate the toxicity of the delivery of nano-microcarriers and the optimal size and shape of the drug carriers in the delivery of nano-microcarriers to the wall of the AAA. The present study's simulation results have highlighted the significant impact of drug carrier shape and dimensions on drug delivery efficiency, with non-spherical particles showing higher interaction with the target wall. Optimal shapes and geometrical parameters for drug carriers in the nano-micrometer range were identified for efficient drug delivery systems targeting AAA. These findings can be extended to other drug delivery applications, and future research should explore the potential of geometric shapes of nano-microcarriers in other types of blood vessels, including those with aneurysm and stenosis complications, such as coronary, carotid, and cerebral blood vessels. Ultimately, improving the efficiency and safety of drug delivery systems can have a significant impact on the treatment of various medical conditions and improve patient outcomes.
Acknowledgements
The authors declare that they have no known competing for financial interests or personal relationships that could have appeared to influence the work reported in this paper.
Disclosure statement
No potential conflict of interest was reported by the author(s).
References
- Alishiri, M., Ebrahimi, S., Shamloo, A., Boroumand, A., & Mofrad, M. R. K. (2021). Drug delivery and adhesion of magnetic nanoparticles coated nanoliposomes and microbubbles to atherosclerotic plaques under magnetic and ultrasound fields. Engineering Applications of Computational Fluid Mechanics, 15(1), 1703–1725. https://doi.org/10.1080/19942060.2021.1989042
- Arzani, A., & Shadden, S. C. (2012). Characterization of the transport topology in patient-specific abdominal aortic aneurysm models. Physics of Fluids, 24(8). https://doi.org/10.1063/1.4744984
- Awad, A. M., Mekheimer, K. S., Elkilany, S. A., & Zaher, A. Z. (2022). Leveraging elasticity of blood stenosis to detect the role of a non-Newtonian flow midst an arterial tube: Mazumdar and Keller models. Chinese Journal of Physics, 77. https://doi.org/10.1016/j.cjph.2022.04.006
- Biglari, H., Razaghi, R., Ebrahimi, S., & Karimi, A. (2019). A computational dynamic finite element simulation of the thoracic vertebrae under blunt loading: Spinal cord injury. Journal of the Brazilian Society of Mechanical Sciences and Engineering, 41(2), 84. https://doi.org/10.1007/s40430-019-1588-z
- Burmasheva, N., & Prosviryakov, E. (2022). Exact solutions to Navier–Stokes equations describing a gradient nonuniform unidirectional vertical vortex fluid flow. Dynamics, 2(2). https://doi.org/10.3390/dynamics2020009
- Climent, N., Arroyo, M., Osullivan, C., & Gens, A. (2014). Sand production simulation coupling DEM with CFD. European Journal of Environmental and Civil Engineering, 18(9). https://doi.org/10.1080/19648189.2014.920280
- Cooley, M., Sarode, A., Hoore, M., Fedosov, D. A., Mitragotri, S., & Sen Gupta, A. (2018). Influence of particle size and shape on their margination and wall-adhesion: Implications in drug delivery vehicle design across nano-to-micro scale. Nanoscale, 10(32). https://doi.org/10.1039/c8nr04042g
- Dai, X., Zheng, G., Cheng, X., & Huo, H. (2019). Numerical simulation of ground movement induced by leakage of groundwater and sand in excavations based on the DEM-CFD method. Yanshilixue Yu Gongcheng Xuebao/Chinese Journal of Rock Mechanics and Engineering, 38(2). https://doi.org/10.13722/j.cnki.jrme.2018.0933
- Dufresne, M., Vazquez, J., Terfous, A., Ghenaim, A., & Poulet, J. B. (2009). Experimental investigation and CFD modelling of flow, sedimentation, and solids separation in a combined sewer detention tank. Computers and Fluids, 38(5). https://doi.org/10.1016/j.compfluid.2008.01.011
- Ebrahimi, S., Shamloo, A., Alishiri, M., Mozhdehbakhsh Mofrad, Y., & Akherati, F. (2021). Targeted pulmonary drug delivery in coronavirus disease (COVID-19) therapy: A patient-specific in silico study based on magnetic nanoparticles-coated microcarriers adhesion. International Journal of Pharmaceutics, 609, Article 121133. https://doi.org/10.1016/j.ijpharm.2021.121133
- Ebrahimi, S., Vatani, P., Amani, A., & Shamloo, A. (2021). Drug delivery performance of nanocarriers based on adhesion and interaction for abdominal aortic aneurysm treatment. International Journal of Pharmaceutics, 594. https://doi.org/10.1016/j.ijpharm.2020.120153
- El Shamy, U., & Aydin, F. (2008). Multiscale modeling of flood-induced piping in river levees. Journal of Geotechnical and Geoenvironmental Engineering, 134(9). https://doi.org/10.1061/(asce)1090-0241(2008)134:9(1385)
- El Shamy, U., & Zeghal, M. (2007). A micro-mechanical investigation of the dynamic response and liquefaction of saturated granular soils. Soil Dynamics and Earthquake Engineering, 27(8), 712–729. https://doi.org/10.1016/j.soildyn.2006.12.010
- Forouzandehmehr, M., & Shamloo, A. (2018). Margination and adhesion of micro- and nanoparticles in the coronary circulation: A step towards optimised drug carrier design. Biomechanics and Modeling in Mechanobiology, 17(1), 205–221. https://doi.org/10.1007/s10237-017-0955-x
- Ghalambaz, M., Saleem, A., Nadeem, S., & Akhtar, S. (2021). Microphysical analysis for peristaltic flow of SWCNT and MWCNT carbon nanotubes inside a catheterised artery having thrombus: Irreversibility effects with entropy. International Journal of Exergy, 34(3). https://doi.org/10.1504/ijex.2021.10036473
- Ghodousi, M., Shahgholi, M., & Payganeh, G. (2018). Analysis of nonlinear vibrations and stability of rotating asymmetrical nano-shafts incorporating surface energy effects. Continuum Mechanics and Thermodynamics, 30(4). https://doi.org/10.1007/s00161-018-0640-z
- He, H., Wang, J., Li, Q., Li, X., Li, M., Wang, T., Li, J., Wang, L., & Shu, C. (2022). Endovascular repair combined with adjunctive procedures in the treatment of tuberculous infected native aortic aneurysms. Journal of Vascular Surgery, 76(2). https://doi.org/10.1016/j.jvs.2022.01.136
- Hinde, E., Thammasiraphop, K., Duong, H. T. T., Yeow, J., Karagoz, B., Boyer, C., Gooding, J. J., & Gaus, K. (2017). Pair correlation microscopy reveals the role of nanoparticle shape in intracellular transport and site of drug release. Nature Nanotechnology, 12(1). https://doi.org/10.1038/nnano.2016.160
- Huang, K., Wu, Y., Zhang, Y., Youn, J. Y., & Cai, H. (2022). Combination of folic acid with nifedipine is completely effective in attenuating aortic aneurysm formation as a novel oral medication. Redox Biology, 58. https://doi.org/10.1016/j.redox.2022.102521
- Huynh, A. T., & Miller, K. (2022). Towards accurate measurement of abdominal aortic aneurysm wall thickness from CT and MRI. In P. M. Nielsen, M. P. Nash, X. Li, K. Miller, & A. Wittek (Eds.), Computational biomechanics for medicine. Springer. https://doi.org/10.1007/978-3-031-09327-2_1
- Kalitzin, G., Medic, G., Iaccarino, G., & Durbin, P. (2005). Near-wall behavior of RANS turbulence models and implications for wall functions. Journal of Computational Physics, 204(1). https://doi.org/10.1016/j.jcp.2004.10.018
- Kang, T., Mukherjee, D., & Ryu, J. (2021). Numerical investigation of carotid stenosis in three-dimensional aortic-cerebral vasculature: Pulsatility index, resistive index, time to peak velocity, and flow characteristics. Engineering Applications of Computational Fluid Mechanics, 15(1). https://doi.org/10.1080/19942060.2021.1984993
- Khanafer, K. M., Bull, J. L., Upchurch, G. R., & Berguer, R. (2007). Turbulence significantly increases pressure and fluid shear stress in an aortic aneurysm model under resting and exercise flow conditions. Annals of Vascular Surgery, 21(1). https://doi.org/10.1016/j.avsg.2006.10.009
- Kwon, O. (2008). Effect of blood viscosity on oxygen transport in residual stenosed artery following angioplasty. Journal of Biomechanical Engineering. https://doi.org/10.1115/1.2838029
- Lan, L., Liu, H., Ip, V., Soo, Y., Abrigo, J., Fan, F., Ma, S. H., Ma, K., Ip, B., Liu, J., Fan, Y., Zeng, J., Mok, V., Wong, L., Liebeskind, D., Leung, T., & Leng, X. (2020). Regional high wall shear stress associated with stenosis regression in symptomatic intracranial atherosclerotic disease. Stroke, 51(10), 3064–3073. https://doi.org/10.1161/STROKEAHA.120.030615
- Lee, C., Duong, W., Kabutey, N.-K., Farzaneh, C., Tohmasi, S., Fujitani, R., Chau, A. H., & Kuo, I. (2023). Endovascular inframesenteric aortic aneurysm repair in cross fused renal ectopia. Annals of Vascular Surgery – Brief Reports and Innovations, 3(1). https://doi.org/10.1016/j.avsurg.2022.100151
- Les, A. S., Shadden, S. C., Figueroa, C. A., Park, J. M., Tedesco, M. M., Herfkens, R. J., Dalman, R. L., & Taylor, C. A. (2010). Quantification of hemodynamics in abdominal aortic aneurysms during rest and exercise using magnetic resonance imaging and computational fluid dynamics. Annals of Biomedical Engineering, 38(4). https://doi.org/10.1007/s10439-010-9949-x
- Li, R., Liu, Y., & Jiang, J. (2023). Research advances in drug therapy for abdominal aortic aneurysms over the past five years: An updated narrative review. International Journal of Cardiology, 372. https://doi.org/10.1016/j.ijcard.2022.11.058
- Lovato, S., Keetels, G. H., Toxopeus, S. L., & Settels, J. W. (2022). An eddy-viscosity model for turbulent flows of Herschel–Bulkley fluids. Journal of Non-Newtonian Fluid Mechanics, 301. https://doi.org/10.1016/j.jnnfm.2021.104729
- Ma, D., Wang, Y., Azhar, M., Adler, A., Steinmetz, M., & Uecker, M. (2022). In silico modeling for personalized stenting in aortic coarctation. Engineering Applications of Computational Fluid Mechanics, 16(1), 2056–2073. https://doi.org/10.1080/19942060.2022.2127912
- Manchester, E. L., Pirola, S., Salmasi, M. Y., O’Regan, D. P., Athanasiou, T., & Xu, X. Y. (2022). Evaluation of computational methodologies for accurate prediction of wall shear stress and turbulence parameters in a patient-specific aorta. Frontiers in Bioengineering and Biotechnology, 10, Article 836611. https://doi.org/10.3389/fbioe.2022.836611
- Mao, H., Wang, X., Wang, Z., & Wu, H. (2018). Seepage of saturated coarse soil multi-scale coupling simulation with dynamic water level. Yanshilixue Yu Gongcheng Xuebao/Chinese Journal of Rock Mechanics and Engineering, 37. https://doi.org/10.13722/j.cnki.jrme.2016.1529
- Moawad, A. M. A., Abdel-Wahab, A. M., Mekheimer, K. S., Ali, K. K., & Sweed, N. S. (2022). Effects of electro-osmotic and double diffusion on nano-blood flow through stenosis and aneurysm of the subclavian artery: Numerical simulation. Waves in Random and Complex Media. https://doi.org/10.1080/17455030.2022.2100945
- Mohammadian, M., & Pourmehran, O. (2019). CFPD simulation of magnetic drug delivery to a human lung using an SAW nebulizer. Biomechanics and Modeling in Mechanobiology, 18(3). https://doi.org/10.1007/s10237-018-1101-0
- Pedram, M. Z., Shamloo, A., Alasty, A., & Ghafar-Zadeh, E. (2016). Optimal magnetic field for crossing super-para-magnetic nanoparticles through the brain blood barrier: A computational approach. Biosensors, 6(2), 25. https://doi.org/10.3390/bios6020025
- Rashidi, S., Esfahani, J. A., & Ellahi, R. (2017). Convective heat transfer and particle motion in an obstructed duct with two side by side obstacles by means of DPM model. Applied Sciences, 7(4). https://doi.org/10.3390/app7040431
- Ruysschaert, N. (2009). (Self) hypnosis in the prevention of burnout and compassion fatigue for caregivers: Theory and induction. Contemporary Hypnosis, 26(3). https://doi.org/10.1002/ch.382
- Saleem, A., Akhtar, S., Alharbi, F. M., Nadeem, S., Ghalambaz, M., & Issakhov, A. (2020). Physical aspects of peristaltic flow of hybrid nano fluid inside a curved tube having ciliated wall. Results in Physics, 19. https://doi.org/10.1016/j.rinp.2020.103431
- Saleem, A., Akhtar, S., Nadeem, S., Issakhov, A., & Ghalambaz, M. (2020). Blood flow through a catheterized artery having a mild stenosis at the wall with a blood clot at the centre. CMES – Computer Modeling in Engineering and Sciences, 125(2). https://doi.org/10.32604/cmes.2020.011883
- Sankar, D. S., & Hemalatha, K. (2007). A non-Newtonian fluid flow model for blood flow through a catheterized artery-steady flow. Applied Mathematical Modelling, 31(9). https://doi.org/10.1016/j.apm.2006.06.009
- Shachar-Berman, L., Bhardwaj, S., Ostrovski, Y., Das, P., Koullapis, P., Kassinos, S., & Sznitman, J. (2020). In silico optimization of fiber-shaped aerosols in inhalation therapy for augmented targeting and deposition across the respiratory tract. Pharmaceutics, 12(3). https://doi.org/10.3390/pharmaceutics12030230
- Shachar-Berman, L., Ostrovski, Y., Koshiyama, K., Wada, S., Kassinos, S. C., & Sznitman, J. (2019). Targeting inhaled fibers to the pulmonary acinus: Opportunities for augmented delivery from in silico simulations. European Journal of Pharmaceutical Sciences, 137. https://doi.org/10.1016/j.ejps.2019.105003
- Shamloo, A., Amani, A., Forouzandehmehr, M., & Ghoytasi, I. (2019). In silico study of patient-specific magnetic drug targeting for a coronary LAD atherosclerotic plaque. International Journal of Pharmaceutics. https://doi.org/10.1016/j.ijpharm.2018.12.088
- Shamloo, A., Boroumand, A., Ebrahimi, S., Kalantarnia, F., Maleki, S., & Moradi, H. (2022). Modeling of an ultrasound system in targeted drug delivery to abdominal aortic aneurysm: A patient-specific in silico study based on ligand-receptor binding. IEEE Transactions on Ultrasonics, Ferroelectrics, and Frequency Control, 69(3). https://doi.org/10.1109/TUFFC.2021.3138868
- Shamloo, A., Ebrahimi, S., Amani, A., & Fallah, F. (2020). Targeted drug delivery of microbubble to arrest abdominal aortic aneurysm development: A simulation study towards optimized microbubble design. Scientific Reports. https://doi.org/10.1038/s41598-020-62410-3
- Shamloo, A., Ebrahimi, S., Ghorbani, G., & Alishiri, M. (2022). Targeted drug delivery of magnetic microbubble for abdominal aortic aneurysm: An in silico study. Biomechanics and Modeling in Mechanobiology, 21(2). https://doi.org/10.1007/s10237-022-01559-4
- Shamloo, A., Manuchehrfar, F., & Rafii-Tabar, H. (2015). A viscoelastic model for axonal microtubule rupture. Journal of Biomechanics, 48(7), 1241–1247. https://doi.org/10.1016/j.jbiomech.2015.03.007
- Shamloo, A., Mohammadaliha, N., & Mohseni, M. (2015). Integrative utilization of microenvironments, biomaterials and computational techniques for advanced tissue engineering. Journal of Biotechnology, 212, 71–89. https://doi.org/10.1016/j.jbiotec.2015.08.005
- Shamloo, A., Nejad, M. A., & Saeedi, M. (2017). Fluid–structure interaction simulation of a cerebral aneurysm: Effects of endovascular coiling treatment and aneurysm wall thickening. Journal of the Mechanical Behavior of Biomedical Materials, 74, 72–83. https://doi.org/10.1016/j.jmbbm.2017.05.020
- Sun, H. T., Sze, K. Y., Chow, K. W., & On Tsang, A. C. (2022). A comparative study on computational fluid dynamic, fluid-structure interaction and static structural analyses of cerebral aneurysm. Engineering Applications of Computational Fluid Mechanics, 16(1), 262–278. https://doi.org/10.1080/19942060.2021.2013322
- Tang, Z., Yu, L., Wang, F., Li, N., Chang, L., & Cui, N. (2018). Effect of particle size and shape on separation in a hydrocyclone. Water, 11(1). https://doi.org/10.3390/w11010016
- Tsuji, Y., Kawaguchi, T., & Tanaka, T. (1993). Discrete particle simulation of two-dimensional fluidized bed. Powder Technology, 77(1). https://doi.org/10.1016/0032-5910(93)85010-7
- Vali, A., Aristova, M., Vakil, P., Abdalla, R., Prabhakaran, S., Markl, M., Ansari, S. A., & Schnell, S. (2019). Semi-automated analysis of 4D flow MRI to assess the hemodynamic impact of intracranial atherosclerotic disease. Magnetic Resonance in Medicine, 82(2). https://doi.org/10.1002/mrm.27747
- Vosswinkel, N., Kouyi, G. L., Ebbert, S., Schnieders, A., Maus, C., Laily, A. G., Mohn, R., & Uhl, M. (2012). Influence of transient behaviour on the settling of solids in storm water tanks. In Proceedings of the 9th Urban Drainage Modelling International Conference (pp. 3–7).
- Wang, J., Tian, X., Yan, C., Wu, H., Bu, Y., Li, J., Liu, D., & Han, Y. (2023). TCF7L1 accelerates smooth muscle cell phenotypic switching and aggravates abdominal aortic aneurysms. JACC: Basic to Translational Science, 8(2). https://doi.org/10.1016/j.jacbts.2022.07.012
- Wilcox, D. C. (2008). Formulation of the k-w turbulence model revisited. AIAA Journal, 46(11), 2823–2838. https://doi.org/10.2514/1.36541
- Yan, H., Vosswinkel, N., Ebbert, S., Lipeme Kouyi, G., Mohn, R., Uhl, M., & Bertrand-Krajewski, J. L. (2020). Numerical investigation of particles’ transport, deposition and resuspension under unsteady conditions in constructed stormwater ponds. Environmental Sciences Europe, 32(1). https://doi.org/10.1186/s12302-020-00349-y
- Yin, C., Rosendahl, L., Knudsen Kær, S., & Sørensen, H. (2003). Modelling the motion of cylindrical particles in a nonuniform flow. Chemical Engineering Science, 58(15). https://doi.org/10.1016/S0009-2509(03)00214-8
- Yousefsani, S. A., Shamloo, A., & Farahmand, F. (2018). Micromechanics of brain white matter tissue: A fiber-reinforced hyperelastic model using embedded element technique. Journal of the Mechanical Behaviour of Biomedical Materials, 80, 194–202. https://doi.org/10.1016/j.jmbbm.2018.02.002
- Zastawny, M., Mallouppas, G., Zhao, F., & van Wachem, B. (2012). Derivation of drag and lift force and torque coefficients for non-spherical particles in flows. International Journal of Multiphase Flow, 39. https://doi.org/10.1016/j.ijmultiphaseflow.2011.09.004
- Zhan, J., Lu, T., Yang, Z., Hu, W., & Su, W. (2022). Influence of the flow field and vortex structure of patient-specific abdominal aortic aneurysm with intraluminal thrombus on the arterial wall. Engineering Applications of Computational Fluid Mechanics, 16(1), 2100–2122. https://doi.org/10.1080/19942060.2022.2131628
- Zhao, J., & Shan, T. (2013). Coupled CFD-DEM simulation of fluid-particle interaction in geomechanics. Powder Technology, 239. https://doi.org/10.1016/j.powtec.2013.02.003
- Zhou, H., Wang, G., Jia, C., & Li, C. (2019). A novel, coupled CFD-DEM model for the flow characteristics of particles inside a pipe. Water, 11(11). https://doi.org/10.3390/w11112381