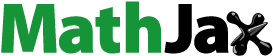
Abstract
To achieve maximum efficiency in organic photovoltaics (OPV), functional layers with uniform and exactly predefined thickness are required. An in-depth understanding of the coating process is therefore crucial for an accurate process control. In this paper, the meniscus-guided blade coating process, which is the most commonly used process for the manufacturing of organic electronics, is investigated by experimental and numerical methods. A computational fluid dynamics (CFD) model is created to simulate the coating behaviour of P3HT:O IDTBR, an industrial state-of-the-art active material system used in OPV, and its results' independence of numerical parameters is ensured. In particular, the influence of the coating velocity and the initially injected fluid volume on the resulting wet film thickness is studied. The developed CFD analysis is able to reproduce the experimental results with very high accuracy. It is found that the film thickness follows a power law dependence on the velocity (˜v2/3) and a linear dependence on the ink volume (˜V). Accordingly, an analytical expression based on our theoretical considerations is presented, which predicts the wet film thickness as a function of the coating velocity and the ink volume only based on easily accessible ink properties. Consequently, this CFD model can effectively substitute time-consuming and expensive experiments, which currently have to be performed manually in the laboratory for a multitude of novel material systems, and thus supports highly accelerated material research. Moreover, the results of this work can be used to achieve homogeneous large-area coatings by utilising accelerated blade coating.
1. Introduction
Organic photovoltaics (OPV) provide a huge potential to unlock novel applications for photovoltaics, because of their unique characteristics, for instance lightweight, mechanical flexibility, and semi-transparency (Hu et al., Citation2022). In lab experiments, OPV maximum efficiencies above 19% have been reported (Zhan et al., Citation2022; Zhu et al., Citation2022). The current world record efficiency of an OPV module with an area >200 cm is 11.7% (Distler et al., Citation2021). To further improve the device efficiency, an immense research effort is being invested, not only concerning practical innovations and optimisations, but also the utilisation of numerical simulations to gain a better understanding of the optoelectronic properties of printed thin-film photovoltaics and the underlying working mechanisms (Aeberhard et al., Citation2019; Chen et al., Citation2019; De Falco et al., Citation2012; Zandi & Razaghi, Citation2019), to optimise the device layout (Lo Sciuto et al., Citation2016; Lucera et al., Citation2015), or to explore the potential of performance-enhancing features (Lo Sciuto et al., Citation2020; Mann & Rastogi, Citation2020).
A thin-film solar cell consists of different layers with different functionalities, which in combination generate electrical energy from the absorbed light. In the case of printed photovoltaics, such as OPV, these layers are deposited from solution, i.e. an ink that comprises the functional material dissolved in a solvent. Upon upscaling from small-area lab cells to large-area modules, a drop in power conversion efficiency of OPV devices is observed. One major reason for this is related to the production process and the homogeneity of the active layer, which becomes harder to maintain with increasing device area (Ibrahim & Hassan, Citation2019). For solution-processed organic electronics, like OPV, meniscus-guided coating techniques are most commonly used to deposit the functional layers (Diao et al., Citation2014). For industrial scale applications with high throughputs, roll-to-roll (R2R) slot-die coating is the most relevant approach among these (Gu et al., Citation2017). For early-stage research, a very similar technique called blade coating (aka ‘horizontal dipping’, see Figure ) is used because of its minimal material consumption.
Figure 1. 3D illustration of the blade coating process. The 2D plane for the simplified CFD model is highlighted.

For blade coating, an applicator (red) is positioned over a substrate (e.g. glass) at a predefined distance, and a defined volume of ink is injected into the gap between the applicator and the substrate. The applicator (or the substrate) is then moved at a defined speed in one direction. While most of the fluid remains inside the gap between the applicator and the substrate, some of the fluid is deposited onto the substrate to leave a thin liquid film behind. A predefined uniform layer thickness is required to achieve maximum efficiency in an OPV device. However, the complex interplay of many different parameters (coating speed, fluid volume, gap height, temperature, fluid kinematic viscosity, fluid surface tension…) influences the process and makes it difficult to predict the resulting coating liquid film thickness for novel inks based on new material combinations.
On one hand, there are some practical studies reported, which investigate the meniscus-guided coating of organic electronic materials and the influence of coating parameters on the properties of the deposited thin film (Chen et al., Citation2019; Lu et al., Citation2020). On the other hand, there are also a few simulative works, which numerically model the film formation process itself (Iliopoulos & Scriven, Citation2005; Singh & Ormiston, Citation2021) or the morphology formation upon drying of the solvent (Michels et al., Citation2020; Ronsin & Harting, Citation2022; Zhang & Wang, Citation2021), in order to contribute to a better process understanding of meniscus-guided coating techniques.
Time-consuming and expensive experiments need to be performed to experimentally establish correlations between process parameters and the resulting film thickness for a specific material system. In order to overcome this difficulty, we developed a computational fluid dynamics (CFD) model based on finite element analyses (FEM), which simulates the blade coating process. The model acts as a ‘digital twin’ of the process that can predict OPV active layer thicknesses as a function of fluid properties and process parameters. In this work, we describe the development of this first-of-its-kind model and validate it by comparing the results of simulation and experiment. An analytical expression is derived that allows for the prediction of the wet film thickness as a function of the initially injected fluid volume and the coating velocity of the applicator.
2. Materials and methods
2.1. CFD model
For the numerical modelling of the coating process, a CFD analysis is conducted. The simulations are performed in the COMSOL Multiphysics platform, which utilises FEM to solve differential equations. For the CFD analysis of the coating process, a moving mesh modelling approach is chosen, since it is superior compared to field-based methods in terms of computation time. However, the moving mesh method cannot handle topological changes. This limitation is overcome by the introduction of an artificial area in the simulation domain (see chapter 3.2.1). Two basic equations are solved in a coupled manner. The first equation is the Navier-Stokes equation:
(1)
(1) Here,
is the velocity vector (SI unit: m/s), ρ is the density (SI unit: kg/m
),
is the stress tensor of the fluid (SI unit: Pa), and
is the volume force vector (SI unit: N/m3). The stress tensor is defined as
, where p refers to the pressure (SI unit: Pa),
to the 3x3 identity matrix, and
to the viscous stress tensor (SI unit: Pa). The second equation is the continuity equation:
(2)
(2) Since the Mach number is very small (MA<<0.3), an incompressible flow can be assumed. Thus, the continuity equation can be rewritten as
(3)
(3) The fluid at the applicator surface is assumed to be stationary in the model. A no slip boundary condition is implemented for boundaries 7, 8, and 9 (see Figure )
(4)
(4) To simulate the movement of the applicator during the coating process, boundaries 2, 3, and 4 in Figure are set as Navier slip boundary conditions. This condition prescribes a no-penetration at the boundary
(5)
(5) with
being the unit normal vector of the boundary. An additional friction term
at the fluid-solid interface can be defined as
(6)
(6) Here, γ is a numerical parameter, which depends on the size of the mesh elements, μ is the dynamic viscosity (SI unit: Pa s), and
is the tangential velocity of the fluid at the boundary, which is defined as
(7)
(7) where
regards to the tangential velocity of the fluid which can be written as
,
to the magnitude of the wall movement which equals the coating velocity, and
to a unit vector, pointing parallel to the boundary in the positive x direction. In Figure , the boundaries 1, 5, and 6 represent the system boundaries and are set up with open boundary conditions
(8)
(8) with
being the magnitude of the stress in normal direction (SI unit: N/m
) at the boundary. For the CFD analysis, zero normal stress is assumed. The surface of the coating fluid is in contact with the surrounding air, making a fluid-fluid interface boundary condition for the boundaries of the menisci (10, 11, and 13 in Figure ) necessary. On the one hand, the fluid-fluid interface boundary condition connects the velocities in both phases, air and coating ink. Since no mass transfer across the interface is assumed, the continuity of both velocity fields across the interface can be expressed by
(9)
(9) where
and
represent the velocity fields in air and ink, respectively. On the other hand, the stress difference across the fluid-fluid interface can be written as
(10)
(10) with
being the unit normal vector at the interface pointing into the air domain,
being the surface gradient operator
, and σ being the surface tension coefficient (SI unit: N/m) (CitationCOMSOL Multiphysics®, Citationn.d.). The fluid-fluid interface boundary condition assumes a contact angle for the intersection points of the interface with solid surfaces. The contact angles of the air-ink interface with the applicator (α) and the glass substrate (β) are experimentally determined (see Table ). The contact angle at the intersection point of boundaries 5, 6, and 13 is assumed to be 90
.
Table 1. P3HT:O-IDTBR fluid properties.
2.2. Coating liquid
For all investigations, an OPV ink based on the photoactive material combination of the donor polymer poly(3-hexylthiophene) (P3HT) and the non-fullerene acceptor (5Z,5'Z)-5,5'-((7,7'-(4,4,9,9-tetraoctyl-4, 9-dihydro-s-indaceno[1,2-b:5,6-b']dithiophene-2,7- diyl)bis(benzo[c][1,2,5]thiadiazole-7,4-diyl))bis(methanylylidene bis(3-ethyl-2-thioxothiazolidin-4-one))) (O-IDTBR) was selected, a material system that is of very high relevance for large-area OPV and its industrial application due to processing and stability reasons (Armin et al., Citation2021; Strohm et al., Citation2018). The ink consists of the solute P3HT:O-IDTBR in a 1:1.25 (w/w) ratio, which is dissolved in a solvent mixture of o-xylene and 1-methylnaphthalene in a 19:1 (v/v) ratio. The solid content of the ink is 36 mg/ml and the resulting ink density is 0.92 g/cm.
After depositing a thin film of the OPV ink on a glass substrate by blade coating (using a Proceq/Zehntner ZAA 2300 thin film coater), the formed wet film dries quickly and leaves a P3HT:O-IDTBR dry film behind (i.e. the active layer of an organic solar cell). After measuring the dry film thickness (in our case with a confocal microscope), the respective original wet film thickness can be calculated. This method is used in this work to determine the experimentally obtained wet film thicknesses.
In addition, the dynamic viscosity μ and the surface tension σ of the ink, as well as its contact angles with the blade applicator (α), and the glass substrate (β) are measured experimentally. All fluid-related properties that are used as input data for the simulations are summarised in Table .
3. Results and discussion
3.1. Experimental motivation
For blade coating of low-viscosity inks (as being used in OPV manufacturing), our experimental results show that the deposited wet film thickness is a function of coating speed and injected ink volume (see Figure ). On one hand, the film thickness strongly increases with increasing velocity. On the other hand, the film thickness also significantly increases with increasing ink volume, which has an important implication: Upon coating, the ink volume inside the gap between substrate and applicator steadily decreases (because part of the fluid is deposited on the substrate), which means that coating over longer distances at constant speed will inevitably lead to a thickness gradient, i.e. a decreasing film thickness along the coating direction. However, if there were a mathematical expression that exactly described the film thickness as a function of coating speed and ink volume, it would potentially be possible to compensate this thickness gradient caused by the ink loss with a defined acceleration of the blade movement, in order to obtain films with constant thickness over large distances. In the following, such a theoretical correlation will be established based on simulations and verified by experiments.
Figure 2. Contour plot of experimentally determined wet film thickness of blade coated P3HT:O-IDTBR layers as a function of coating velocity v and injected ink volume V. The plot comprises a total of 40 data points (a variation matrix of eight different coating speeds and five different ink volumes) and each data point is the average of two measured samples coated with identical parameters.

3.2. Development of the CFD model
3.2.1. Design of the modelling domain
Figure shows the modelling domain of the CFD analysis. Regions A and C are air domains, whereas regions B and D represent the domains of the coating liquid. The trapezoid shape on the top stems from the applicator. Boundaries 7, 8, and 9 depict the interface of the applicator. The surface of the substrate on which the coating liquid is applied is represented by the boundaries 2, 3, and 4.
For the investigation, the geometry of the model is developed in a two-step procedure. This improves the solvability of the problem and reduces its computing time. The initial shape of the air-fluid interface is assumed to be circular. Different centres and radii of the underlying circles correspond to different injected volumes (50–90 µl). The exact shape of the menisci (boundaries 10 and 11 in Figure ) is then modelled by the quasi-stationary study in the first step. In the second step, the geometry is supplemented with an artificial region (D in Figure ) to avoid topology changes of the model and maintain a fine enough discretisation.
3.2.2. Numerical discretisation
During the transient simulation, the geometry will experience a deformation. A ‘roller’ feature is applied to all boundaries where the air-liquid interface intersects with the ambient boundaries of the simulation domains (boundaries 2–9) to allow this modification. This feature allows the discretisation elements to move tangentially along the boundary and prevents mesh elements from moving perpendicular to the boundary. For the discretisation of the simulation domain, particular emphasis was put on meshing domain D since film formation takes place in this area. The mesh that is used for the investigation of the wet film thickness is shown in Figure .
A conventional triangular discretisation type is chosen for the air domains (A and C) and for the domain of the injected fluid volume (domain B). The geometry of domain D will undergo significant changes during the transient study focussing on the wet film thickness. The height of domain D will decrease during the study, along with the liquid film thickness. Using triangular elements, the shrinking of this domain in y-direction would cause deformed mesh elements. Resulting from this suboptimal discretisation, only simulation results with poor spatial resolution and/or challenging numerical convergence behaviour would be possible. As a better approach, distribution features are set up for boundaries 5 and 12 to define a fixed number of mesh elements at these boundaries. Afterwards, a mapped mesh is applied to domain D, resulting in a rectangular discretisation (inset in Figure ). To quantify the impact of the numerical discretisation on the simulation results, a mesh refinement study is conducted. This means that multiple studies were performed with different numbers of mesh elements along boundaries 5 and 12. The considered injected fluid volume was 90 µl and the coating velocity 90 mm/s, since these parameters result in the largest film thicknesses. Thus, dependencies of the simulation results on the numerical discretisation should be most distinct. In Figure , the result of the mesh refinement study is shown.
Figure 5. Influence of the degrees of freedom on the liquid film thickness (indicated by blue squares) and the computing time (plotted as orange circles).

Since the size of the mesh elements in domain D has been varied, the number of elements has increased, which corresponds to more degrees of freedom (DOF). The DOF is a typical measure of the magnitude of a numerical problem; generally, the smaller the DOF, the less computing time is necessary. The linear dependency of the computing time on the DOF can be seen in Figure .
In our specific case, the simulation runs on an Intel(R) Xeon(R) Gold 6142 CPU @ 2.60GHz machine with 128 GB of RAM. For a specific discretisation, the liquid film thickness depends highly on the DOF until ˜150k DOF. At larger values, the film thickness is relatively constant: it varies by only 0.1 µm, which is a 0.3% relative variation of the total film thickness.
In order to efficiently investigate all further wet film thicknesses, a discretisation of ˜150k DOF is chosen, as it results in the same wet film thickness as simulations with finer discretisation, while having a reduced computational time.
3.3. Comparison of simulation and experimental results
In order to validate the developed CFD model, the simulated results are compared to the experimental results of the blade coating process. To this end, firstly, cross-sectional photographs of the OPV ink in the gap between the applicator and the glass substrate were taken at rest and at the maximal coating speed of 90 mm/s (see Figure (a ,b)). The reddish trapezoid in all images is the lower part of the applicator, which holds the coating liquid (black). At rest (a)), the coating liquid is distributed symmetrically with respect to the centre of the applicator, forming equal menisci on both sides. In the case of a moving applicator (b)), the fluid is no longer symmetrically centred underneath the applicator, but is rather dragged behind it due to the friction at the liquid-substrate interface. Consequently, the higher the coating speed, the greater the difference in fluid geometry between the idle state and the steady state of movement.
Figure 6. Cross-sectional view of the blade coating process with 90 µl injected fluid volume. Figures (a) and (b) show photographs taken from the experiments. The red trapezoid is the applicator, the black area is the coating ink, and the remaining two areas (left and right) are air. The substrate surface equals the bottom edge of the photographs. In each image, the coating direction is indicated by an arrow labelled with the corresponding velocity. Images (c) and (d) show the simulated counterparts of the experiments. In (a) and (c), the applicator is at rest (0 mm/s); (b) and (d) show the steady state for an applicator movement of 90 mm/s. In images (c) and (d), the artificial simulation domain (domain D) is indicated by coloured arrows.

The respective simulated counterparts of the images are shown in Figure (c ,d), in which the liquid domains are indicated in black. In both cases, i.e. the idle (c)) and the moving (d)) state, the simulated menisci of the coating fluid match the experimental observations very well. In Figure (c), where the applicator is at rest, the supplemented artificial domain D from the geometry initialisation procedure, as described in Section 3.2.1, can be seen (indicated by the blue arrow). It is important to note that, in this state (i.e. before the coating started), the height of this area does not yet represent the experimentally observed wet film thickness at this position, because there is no deposited wet film yet. However, for the simulation of a moving blade, as depicted in (d), this area will change and adapt to the steady-state condition for the respective velocity, here 90 mm/s. In this case, the height of the artificial domain equals the deposited wet film thickness that is predicted by the simulation (indicated by the orange arrow).
The wet film thickness, generated by the blade coating process, is investigated in a transient, two-dimensional CFD study. In the beginning of the transient study, the liquid film thickness oscillates due to the rapid height reduction of domain D. Therefore, the time domain of the simulation is chosen in such a way that a steady state and a stable coating process are established, which is the case after a coating distance of 20 mm. Thus, the time domain depends on the coating velocity. Interestingly, also in case of the experiment, stable coating with homogeneous film thicknesses only starts after 10–20 mm coating distance, depending on the coating speed.
To compare the wet film thickness values obtained by simulation and experiment, a variation matrix of different coating speeds ranging from 1 to 90 mm/s and different volumes of injected ink ranging from 50 to 90 µl is chosen and investigated. The experimental wet film thickness is obtained as described in Section 2.2. The experimentally determined dependency of the wet film thickness on the coating velocity for different injected volumes (i.e. the same data set as initially presented in Section 3.1) is shown in Figure by square data points and error bars. Moreover, the respective values obtained by our CFD simulation model are plotted in the same figure as solid lines.
Figure 7. Simulated and experimentally determined wet film thickness as a function of coating speed for different injected fluid volumes. Simulation results are plotted as solid lines, whereas experimental data is represented by squares with corresponding error bars.

An excellent agreement between simulation and experimental results is observed for injected volumes of 50 to 80 µl. For 90 µl, the experimental and simulation data match well for small coating velocities, while they slightly deviate at the higher velocities. This mismatch is due to experimental values that are lower than the simulated ones, which is most probably caused by the applicator's inability to properly drag the injected fluid at the start of the coating process for high fluid volumes in combination with high accelerations. Consequently, an inordinately large amount of the coating fluid remains at the starting position, which has the same effect on the resulting layer thickness as an initially smaller injected volume V. Both simulation and experiment show the same trend of increasing wet film thickness with increasing coating speed. Furthermore, the increase in wet film thickness with higher injected ink volumes predicted by the simulations matches very well the observed experimental results.
3.4. Comparison of simulation and experiment with theory
In 1940s, Landau and Levich (Landau & Levich, Citation1988) and Derjaguin (Derjaguin, Citation1993) developed the theory to describe the deposition of a thin liquid film on a solid. The theoretical considerations hold only true for Newtonian fluids with a capillary number (so-called Landau-Levich-regime). In this case, the wet film thickness h as a function of the coating velocity v can be described by the power-law, derived by Landau and Levich
(11)
(11) In previous literature reports, the predicted power-law dependency of the wet film thickness versus the coating speed has already been experimentally verified for blade coating of OPV inks (Nickel et al., Citation2012; Park & Han, Citation2009). However, the dependency of Equation (Equation11
(11)
(11) ) on the injected volume V has not been studied directly yet. Since the deposited wet film thickness is determined by the radius of the formed down-stream meniscus, its dependence on the injected ink volume depends itself on the applicator geometry. For example, Park et al. derived an analytical expression for the wet film thickness for a cylindrical applicator (i.e. a circular cross-section) (Park & Han, Citation2009). In our setup, which is a commonly used blade coater for OPV devices, the applicator has a trapezoidal cross-section. In order to determine the wet film thickness as a function of ink volume, Equation (Equation11
(11)
(11) ) can be written as:
(12)
(12) where
is a constant (SI unit: m1/3s2/3), which depends on the injected fluid volume V. Accordingly, Equation (Equation12
(12)
(12) ) is used to fit our simulated and experimental data to the theoretical predictions. The results are shown in Figure (a,b), respectively. Remarkably, both the simulation and the experiment match very well with the theoretical correlation.
Figure 8. In (a), simulated wet film thicknesses for different injected volumes are displayed by solid lines, the regarding fit of Equation (Equation12(12)
(12) ) is shown by dashed lines. In (b), the experimentally determined wet film thicknesses for different injected volumes are indicated by square data points with associated error bars, while the regarding power-law fits are plotted as dashed lines.

In order to further determine the dependency on the injected ink volume, the values of the coefficient a, which correspond to the fits of the simulation and experimental data, are plotted versus the injected volume V in Figure . Again, the experimentally and simulatively determined coefficients a are in great agreement, with only a mismatch at 90 µl (experimental data point too low), because of the previously described insufficient ability of the applicator to drag the high amount of injected fluid at the start of the process
Figure 9. The power-law coefficient from Equation (Equation12
(12)
(12) ) as extracted from the fits to the simulated (blue) and experimental data (orange) data with corresponding error bars as a function of the injected volume V. The blue dashed line represents a linear fit to the data obtained from simulation.

Furthermore, the values of a suggest a linear dependency on the injected volume V, which is confirmed by a linear fit to the simulation data (dashed blue line in Figure ). Consequently, a can be written as , where b refers to the intersection and c to the slope, respectively. The values corresponding to the linear fit in Figure are listed in Table . Note: The fact that for V = 0,
is > 0, as implied by the positive intersection value b, suggests that this correlation cannot be valid for very small volumes. Due to the trapezoidal applicator geometry, there is also a practical minimum volume that always has to be present between the applicator and the substrate (filling the gap underneath boundary 8 in Figure ) in order to cover the whole substrate width upon coating, which is ˜23 µl in our case (gap height (400 µm) * substrate width (25 mm) * applicator bar length (2.3 mm)). Therefore, the validity range of the correlation is also limited to
23 µl.
Table 2. Parameters of the linear fit to the simulatively determined coefficient a.
To the best of our knowledge, the expression of the film thickness h as a function of V is a novel establishment. This correlation is certainly determined by the applicator geometry as well as the contact angle at the fluid-vapour-applicator interface, since both have an effect on the shape of the down-stream meniscus. In our case, the contact angle does not vary for the different injected volumes, due to the trapezoidal geometry of the applicator. Thus, the determination of the coefficients b and c enables an analytical expression to predict the wet film thickness h for an ink, as a function of the coating velocity v and the injected volume V:
(13)
(13) With our developed CFD simulation model, we have shown to be able to precisely determine these coefficients only based on the ink properties density, viscosity, surface tension, and contact angles. Consequently, this approach is able to predict wet film thicknesses and to suggest coating parameters for desired wet film thicknesses, which saves a lot of temporal and economical expenditure, when it comes to the qualification and process development of new ink formulations, and thus enables highly accelerated material research.
Moreover, based on Equation (Equation13(13)
(13) ) and the corresponding parameters in Table , the deposited wet film thickness can be calculated for every velocity-volume combination. This allows for the determination of an acceleration profile that is able to compensate for the loss of ink volume during coating, in order to prevent a thickness gradient as described in Section 3.1. Consequently, this approach enables the fabrication of large-area coatings with constant film thickness.
4. Conclusions
The CFD analysis of the blade coating process developed and presented in this work shows an excellent agreement with the experimental data and is able to precisely predict the wet film thickness as a function of coating velocity and ink volume only based on easily accessible ink properties. This approach has been demonstrated and verified for one OPV active material ink and will be extended to further material systems with different fluid properties in the future, in order to prove the general applicability of our simulations to model the coating behaviour of novel inks. By this, our CFD model can effectively substitute time-consuming and expensive experiments to establish such correlations for new material systems and thus constitutes a resource-efficient approach that supports the necessary high-throughput optimisations in a rapidly evolving technology field such as OPV. Moreover, the development of an accelerated blade coating process based on the results of this work to achieve homogeneous coatings over large distances, is currently ongoing and will be published in due course. Finally, our model is not limited to OPV or other types of printed electronics, but can be utilised for blade coated films of all kind, which require a precise and homogeneous layer thickness.
Disclosure statement
No potential conflict of interest was reported by the author(s).
Additional information
Funding
References
- Aeberhard, U., Altazin, S., Stepanova, L., Stous, A., Blülle, B., Kirsch, C. M., Knapp, E., & Ruhstaller, B. (2019). Numerical optimization of organic and hybrid multijunction solar cells. https://doi.org/10.1109/pvsc40753.2019.8980824
- Armin, A., Li, W., Sandberg, O. J., Xiao, Z., Ding, L., Nelson, J., Neher, D., Vandewal, K., Shoaee, S., Wang, T., Ade, H., Heumueller, T., Brabec, C. J., & Meredith, P. (2021). A history and perspective of non–Fullerene electron acceptors for organic solar cells. Advanced Energy Materials, 11(15), 2003570. https://doi.org/10.1002/aenm.202003570
- Chen, M., Peng, B., Huang, S., & P. K. L. Chan (2020). Understanding the meniscus–guided coating parameters in organic field–effect–transistor fabrications. Advanced Functional Materials, 30(1), 1905963. https://doi.org/10.1002/adfm.201905963
- COMSOL Multiphysics® (n.d.). CFD module user's guide. Version 5.4. https://doc.comsol.com/5.4/doc/com.comsol.help.cfd/CFDModuleUsersGuide.pdf
- De Falco, C., Porro, M., Sacco, R., & Verri, M. (2012). Multiscale modeling and simulation of organic solar cells. Computer Methods in Applied Mechanics and Engineering, 245-246, 102–116. https://doi.org/10.1016/j.cma.2012.06.018
- Derjaguin, B. (1993). On the thickness of the liquid film adhering to the walls of a vessel after emptying. Progress in Surface Science, 43(1-4), 134–137. https://doi.org/10.1016/0079-6816(93)90022-n
- Diao, Y., Shaw, L., Bao, Z., & S. C. B. Mannsfeld (2014). Morphology control strategies for solution-processed organic semiconductor thin films. Energy and Environmental Science, 7(7), 2145–2159. https://doi.org/10.1039/c4ee00688g
- Distler, A., Brabec, C. J., & Egelhaaf, H. (2021). Organic photovoltaic modules with new world record efficiencies. Progress in Photovoltaics, 29(1), 24–31. https://doi.org/10.1002/pip.3336
- Gu, X., Zhou, Y., Gu, K. L., Kurosawa, T., Guo, Y., Li, Y., Lin, H., Schroeder, B. C., Yan, H., Molina-Lopez, F., Tassone, C. J., Wang, C., Mannsfeld, S. C. B., Yan, H., Zhao, D., Toney, M. F., & Bao, Z. (2017). Roll–to–roll printed large–area all–polymer solar cells with 5% efficiency based on a low crystallinity conjugated polymer blend. Advanced Energy Materials, 7(14), 1602742. https://doi.org/10.1002/aenm.201602742
- Hu, Y., Wang, J., Yan, C., & Cheng, P. (2022). The multifaceted potential applications of organic photovoltaics. Nature Reviews Materials, 7(11), 836–838. https://doi.org/10.1038/s41578-022-00497-y
- Ibrahim, M. L. I., & Hassan, H. H. M. (2019). Influence of active layer thickness on the performance of organic photovoltaics with light trapping. IEEE Transactions on Electron Devices, 66(7), 3124–3128. https://doi.org/10.1109/ted.2019.2917594
- Iliopoulos, I., & L. E. Scriven (2005). A blade-coating study using a finite-element simulation. Physics of Fluids, 17(12), 127101. https://doi.org/10.1063/1.2140226
- Landau, L., & Levich, B. (1988). Dragging of a liquid by a moving plate. In Esevier eBooks (pp. 141–153). https://doi.org/10.1016/b978-0-08-092523-3.50016-2
- Lo Sciuto, G., Capizzi, G., Coco, S., & Shikler, R. (2016). Geometric shape optimization of organic solar cells for efficiency enhancement by neural networks. In Lecture notes in mechanical engineering (pp. 789–796). Springer Nature. https://doi.org/10.1007/978-3-319-45781-9_79
- Lo Sciuto, G., Coco, S., Gotleyb, D., & Shikler, R. (2020). Finite element numeric simulation of organic solar cells with gold thin film. International Journal on Advanced Science, Engineering and Information Technology, 10(6), 2478. https://doi.org/10.18517/ijaseit.10.6.13782
- Lu, Z., Wang, C., Deng, W., Achille, M. T., Jie, J., & Zhang, X. (2020). Meniscus-guided coating of organic crystalline thin films for high-performance organic field-effect transistors. Journal of Materials Chemistry C, 8(27), 9133–9146. https://doi.org/10.1039/d0tc01887b
- Lucera, L., Kubis, P., Fecher, F. W., Bronnbauer, C., Turbiez, M., Forberich, K., Ameri, T., Egelhaaf, H., & Brabec, C. J. (2015). Guidelines for closing the efficiency gap between hero solar cells and roll-to-roll printed modules. Energy Technology, 3(4), 373–384. https://doi.org/10.1002/ente.201402192
- Mann, V., & Rastogi, V. (2020). FDTD simulation studies on improvement of light absorption in organic solar cells by dielectric nanoparticles. Optical and Quantum Electronics, 52(5). https://doi.org/10.1007/s11082-020-02328-2
- Michels, J. J., Zhang, K., Wucher, P., Beaujuge, P. M., Pisula, W., & Marszalek, T. (2021). Predictive modelling of structure formation in semiconductor films produced by meniscus-guided coating. Nature Materials, 20(1), 68–75. https://doi.org/10.1038/s41563-020-0760-2
- Nickel, F., Sprau, C., Klein, M. L., Kapetana, P., Christ, N., Liu, X., Klinkhammer, S., Lemmer, U., & Colsmann, A. (2012). Spatial mapping of photocurrents in organic solar cells comprising wedge-shaped absorber layers for an efficient material screening. Solar Energy Materials and Solar Cells, 104, 18–22. https://doi.org/10.1016/j.solmat.2012.04.026
- Park, B., & Han, M. A. (2009). Photovoltaic characteristics of polymer solar cells fabricated by pre-metered coating process. Optics Express, 17(16), 13830. https://doi.org/10.1364/oe.17.013830
- Ronsin, O., & Harting, J. (2022). Formation of crystalline bulk heterojunctions in organic solar cells: insights from phase-field simulations. ACS Applied Materials & Interfaces, 14(44), 49785–49800. https://doi.org/10.1021/acsami.2c14319
- Singh, A. R., & Ormiston, S. J. (2022). CFD analysis of blade coating from a reservoir onto a horizontal substrate using a homogeneous two–phase model. Canadian Journal of Chemical Engineering, 100(2), 349–362. https://doi.org/10.1002/cjce.24096
- Strohm, S., Machui, F., Langner, S., Kubis, P., Gasparini, N., Salvador, M., McCulloch, I., Egelhaaf, H., & Brabec, C. J. (2018). P3HT: Non-fullerene acceptor based large area, semi-transparent PV modules with power conversion efficiencies of 5%, processed by industrially scalable methods. Energy & Environmental Science,, 11(8), 2225–2234. https://doi.org/10.1039/c8ee01150h
- Zandi, S., & Razaghi, M. (2019). Finite element simulation of perovskite solar cell: A study on efficiency improvement based on structural and material modification. Solar Energy, 179, 298–306. https://doi.org/10.1016/j.solener.2018.12.032
- Zhan, L., Yin, S., Li, Y., Li, S., Chen, T., Sun, R., Min, J., Zhou, G., Zhu, H., Chen, Y., Fang, J., Yang, J., Xia, X., Lu, X., Qiu, H., Fu, W., & Chen, H. S. (2022). Multiphase morphology with enhanced carrier lifetime via quaternary strategy enables high–efficiency, thick–film, and large–area organic photovoltaics. Advanced Materials, 34(45), 2206269. https://doi.org/10.1002/adma.202206269
- Zhang, L., & Wang, X. (2021). Evaporation-driven nanoparticles motion and deposition on a textured surface in the inkjet process. Engineering Applications of Computational Fluid Mechanics, 15(1), 644–655. https://doi.org/10.1080/19942060.2021.1902859
- Zhu, L., Zhang, M., Xu, J., Li, C., Yan, J., Zhou, G., Zhong, W., Hao, T., Jiali, S., Xue, X., Zhou, Z., Zeng, R., Zhu, H., Chen, C., MacKenzie, R. C. I., Zou, Y., Nelson, J., Zhang, Y., Sun, Y., & Liu, F. (2022). Single-junction organic solar cells with over 19% efficiency enabled by a refined double-fibril network morphology. Nature Materials, 21(6), 656–663. https://doi.org/10.1038/s41563-022-01244-y