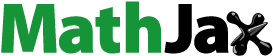
Abstract
Cavitation frequently arises in the safety valve of nuclear power plants’ secondary circuits operating under high pressure conditions. This study integrates valve flow characteristics and velocity strain rate corrections into the Zwart-Gerber-Belamri model to accurately simulate cavitation inside the valve, reducing the impact of physical empirical coefficient variations on cavitation length prediction. Subsequently, a visualisation test rig is developed to validate the accuracy of the numerical model, and experimental cavitation results are obtained using the grayscale detection method. The evaporation/condensation coefficients are optimised using the AES-MSI model and GA based on the experimental results. The accuracy of the constructed model is validated by comparing it with experimental results obtained under various operating conditions. Finally, the high-fidelity numerical model is employed to investigate the effects of pressure drop and valve openings on cavitation, elucidating the underlying mechanisms governing cavitation variations resulting from pressure drops. Furthermore, a comprehensive equation is derived to determine the effective flow area, aiding in the identification of cavitation locations and offering insights into the relationship between cavitation behaviour and valve openings. The modified cavitation model proposed in this study can be readily extended to investigate cavitation prediction in other valves or throttle elements.
1. Introduction
Nuclear power is a clean, efficient, and reliable form of energy that harnesses nuclear reactions to generate electricity (Bayramov, Citation2023). Safety valves play a crucial role in ensuring safe operation of nuclear power plants by serving as the last line of defense against dangerous pressure buildup in the steam, cooling water, and compressed air systems (Bjerre et al., Citation2017). Among these valves, the safety valve used in the second circuit is specifically designed to prevent excessive pressure generated by the water pump supplying water to the steam generator, as illustrated in Figure (Li et al., Citation2023b). Under high-pressure conditions, the valve opens and reduces the flow-through area, causing a decrease in pressure near the valve disc. When the local pressure falls below the saturated vapour pressure of the fluid medium, cavitation can occur, which is a phase change process that transforms liquid into gas (Nozawa, Citation2023). Cavitation bubbles then rapidly expand and release a huge pressure pulse when they collapse, causing material failure in the cavitation zone (Wang et al., Citation2021a). Cavitation damage inside the valve has been identified as a major cause of valve failure (Sreedhar et al., Citation2017). Therefore, studying cavitation is essential for safe engineering applications in flow systems, especially for the safe operation of nuclear power systems.
Figure 1. Safety valve in second loop of nuclear power plant. The red dotted line indicates the designated installation position of the safety valve within the secondary circuit of a nuclear power plant. In the event that the pump pressure exceeds the system's maximum permissible pressure, the safety valve promptly opens, releasing the medium to restore the system pressure to its normal level.

The study of cavitation can be traced back to 1753 (Sun et al., Citation2022). When Euler discovered that the pressure in a water pipe could decrease to a negative value, causing the water to separate from the pipe wall and create a cavity through vaporisation of the liquid. This vacuum should be avoided to prevent damage to the system. In the late nineteenth century, the shipbuilding industry encountered problems when propeller speed was increased continuously, leading to a decrease in a ship's speed driven by a steam turbine. This was due to the impact of cavitation, which led scholars to start recognising its effects, leading to increased attention towards the study of cavitation. In the available literature, the earliest investigation of valve cavitation dates back to the work of MacLellan et al. in Citation1960 (MacLellan et al., Citation1960). In their study, they detected the cavitation phenomenon during fluid flow through the spool valve orifice. Following this, numerous scholars focused on the study of valve cavitation. Advanced testing technology and improved computer performance have led to experimental testing and numerical simulation becoming the primary methods for studying the phenomenon in valves. Due to the intricate structure of the valve flow channel and the highly nonlinear nature of cavitation in fluid dynamics, it is impractical to rely solely on theoretical models to derive mathematical equations for the cavitation phenomenon in valves.
Studies on valve cavitation using experimental techniques are divided into two main categories. The first category involves the indirect study of cavitation through testing the mass flow rate of the valve, as cavitation significantly impacts valve throttling. For instance, Amirante et al. (Citation2014) conducted an experimental analysis to investigate the effect of cavitation on the performance of a proportional direct-acting hydraulic reversing valve. They used a constructed experimental rig and a high-precision gear flow metre to measure the mass flow rate under cavitation conditions. Valdés et al. (Citation2014) investigated the cavitation flow in ball check valves by measuring the mass flow to control various degrees of cavitation through pressure adjustments at the valve outlet. The characteristic flow coefficients of the valves were subsequently analyzed. However, while flow measurement provides some insight into cavitation, it does not constitute a comprehensive study of valve cavitation. The cavitation phenomenon should be more critically evaluated by considering the cavitation length, as the morphology of the cavitation bubble is directly related to the degree of corrosion experienced by the valve's key components. Consequently, visualisation experiments have become an essential approach to studying cavitation. Oshima and Ichikawa (Citation1985) observed the cavitation phenomenon of converging and diverging flows in the poppet valve using the experimental half-cut test model. They investigated the effects of cavitation on the mass flow rate, noise, pressure distribution, and cavitation onset boundary of the water hydraulic poppet valve.
Kumagai et al. (Citation2016) developed a visualisation system to analyze the mechanism of cavitation-induced spool vibration. They used a high-speed camera to capture cavitation inside a transparent cone valve (with the body made of PMMA). Li et al. (Citation2013) developed a visualisation experimental system to observe the cavitation phenomenon between the flapper and nozzle in an electro-hydraulic servo valve. They determined the location of the cavitation source and explored the cavitation evolution with respect to Reynolds number. Wang et al. (Citation2018) utilised a camera and a transparent throttle valve made of PMMA material to capture the inner bubble morphology of the valve. They employed convolutional neural networks for rapid detection of spool features and developed intelligent algorithms based on two-dimensional detection and three-dimensional reconstruction of the bubbles. Lu et al. (Citation2022) employed 46# anti-wear hydraulic oil as the working medium, and the valve body of the spool valve was fabricated using highly transparent Plexiglas material. They captured cavitation images inside the valve using a high-speed camera and adjusted the valve opening by manipulating bolts to obtain cavitation images at different openings. Yi et al. (Citation2015) observed the cavitation phenomenon inside the relief valve by using a camera and a transparent valve body. They experimentally investigated the interaction between the spool vibration characteristics and the cavitation characteristics.
Capturing cavitation cavities through high-speed cameras is a straightforward approach for conducting cavitation experiments. However, this method requires a transparent valve body, which limits the range of temperature and pressure of the medium during experimental tests. Moreover, the extensive time and economic costs associated with this method hinder the optimal valve design. As computer performance has improved, numerical simulation methods based on computational fluid dynamics (CFD) have become crucial tools for cavitation research. For example, Zong et al. (Citation2022) conducted a numerical simulation of heat-fluid-solid coupling for explosion-proof valves with complex flow paths using the CFD method. They proposed a reduced-dimensional computational fluid dynamics modelling method to simulate the barrier effect of the flame retardant sheet. Steady and transient simulations were conducted, leading to the conclusion that the computational accuracy of the equivalent volumetric method proposed in their study was higher than that of the equivalent porous method. Within the computational framework based on the theory of CFD, two models are commonly adopted for gas–liquid two-phase flow: the two-fluid model and the homogeneous equilibrium flow model (HEM) (Tahmasebi et al., Citation2017). HEM has gained widespread use in practical engineering due to its superior convenience and relatively mature method in practical applications (Salvador et al., Citation2017; Webber, Citation2011). Under the assumption of HEM, two mainstream cavitation models, the barotropic equation model (BEM), and the transport equation model (TEM), have been formed based on the determination of different density fields (Chen et al., Citation2016). However, researchers found that the BEM has certain limitations in predicting the convection and transport of cavitation as it does not reflect the phase change process in the physical model. As the understanding of cavitation improved, researchers turned to the TEM to capture the phase change process in cavitation with greater accuracy. The most representative models based on the assumption of TEM are the Schnerr-Sauer cavitation model (Schnerr-Sauer model), Singhal fully cavitation model (Singhal model), and Zwart-Gerber-Belamri cavitation model (Z-G-B model) (Schnerr & Sauer, Citation2001; Singhal et al., Citation2002; Zwart et al., Citation2004). Liu et al. (Citation2019) developed a CFD cavitation model for the regulating valve using the Schnerr-Sauer model and the standard k-ε turbulence model. They analyzed the changes in the location, shape, area, and density of cavitation in both the axial and radial directions at different inlet pressure conditions. Yaghoubi et al. (Citation2018) performed a numerical simulation of a globe control valve to examine the effects of orifice diameter and various trim types on cavitation delay performance. They found that using three trims resulted in the best economic and technical performance in terms of delaying cavitation. In addition, some scholars have also adopted new cavitation models or methods for the simulation of cavitation flow. For example, Cheng et al. (Citation2021) propose a novel Eulerian-Lagrangian cavitation model that considers the influence of non-condensable gases. The global flow field is solved using the Eulerian method, while the migration of non-condensable bubbles towards the vortex core is tracked employing the Lagrangian method. The mass source term in the original Schnerr-Sauer cavitation model is adjusted through modelling the relationship between the local gas concentration and its impact on cavitation. The model has been successfully applied to investigate the cavitation on NACA-16020 hydrofoils, and its effectiveness has been verified by comparing it with experimental results. Wang et al. (Citation2021b) employed a multiscale Eulerian-Lagrangian method to simulate cavitation flow around a Clark-Y hydrofoil. The method combines large vortex simulation with the fluid volume method and tracks the microscale Lagrangian bubbles by solving the compressible Rayleigh-Plesset equation and the equations of motion for bubbles. A two-way coupled simulation of the interaction between the flow field and bubbles is performed using Gaussian kernel functions. The validity of the method is finally confirmed through experimental verification.
In the study of cavitation length and mechanisms of cavitation evolution, Wang et al. (Citation2023) proposed a novel multiscale modelling approach to investigate numerically the cavitation formation mechanism of hydrofoils. In their study, a mixture model and a Lagrangian model were used to capture the macroscopic vapour structure and the behaviour of microbubbles, respectively. The study provides a comprehensive explanation of the cloud cavitation and its evolution process, as well as the changing law of bubble dynamics. Gao et al. (Citation2021) conducted numerical simulations of cage control valves using the Z-G-B cavitation model to compare the flow rate and cavitation strength of different valve cage structures. The comparison shows that the improved cage structure has the smallest cavitation strength, and the paper also deduces that the cavitation strength and flow coefficient gradually decrease with the increase in the number of cages and the height of grooves. Yuan et al. (Citation2019) conducted a numerical simulation of cavitation caused by a jet inside the poppet valve using the Schnerr-Sauer model. They analyzed the inhibitory effect of cavitation growth on the vortex and explained the interaction mechanism between cavitation and the vortex. Jin et al. (Citation2020) explored the effect of four typical valve core shapes on cavitation in valves using the Schnerr-Sauer model. They aimed to reduce cavitation in regulating valves and concluded that the cavitation strength was higher with ellipsoidal and cylindrical valve cores. Additionally, they studied the relationship between valve opening and vapour volume, finding that the vapour volume tends to increase and then decrease with the increase in valve core displacement. Liu et al. (Citation2021) investigated the characteristics of transient cavitation and pressure fluctuation inside a regulating valve using the Schnerr-Sauer model. They analyzed the effect of variations in the length-to-radius ratio of the valve spool on cavitation characteristics. Furthermore, other scholars have conducted numerous studies on valve cavitation using numerical simulation methods (Habibnejad et al., Citation2022; He et al., Citation2023; Qian et al., Citation2023).
From the literature summary above, it is evident that there is a wealth of literature on valve cavitation from experimental and numerical perspectives. However, there are still several shortcomings.
Currently, research on valve cavitation primarily focuses on regulating/control valves, with less attention paid to safety valves. The few studies on safety valves have primarily focused on two-dimensional (2-D) or simplified three-dimensional (3-D) modelling of the flow core region only, instead of utilising full-size three-dimensional modelling of the valve. These modelling approaches may result in inaccurate capture of cavitation compared to full-size 3-D modelling, as geometric boundaries can significantly impact fluid flow characteristics, particularly pressure drop, which is relevant to simulating cavitation.
Most researchers use cavitation models developed for hydrofoils and inducers, which may not be appropriate for simulating safety valve cavitation due to specific values of empirical coefficients. Adjustments to these coefficients are often necessary to account for specific operating conditions. For instance, Zwart et al. (Citation2004) recommended setting the evaporation and condensation coefficients to 50 and 0.01, respectively, while in another study he set the evaporation and condensation coefficients to 0.4 and 0.001, respectively. Therefore, it is necessary to propose a robust method capable of eliminating the effect of the variation of these empirical coefficients on the results or a method capable of efficiently determining these coefficients.
Moreover, although many researchers have provided general guidelines for the influence of certain parameters on valve cavitation, the definition of cavitation length on safety valve remains unclear, and the explanation for the cavitation evolution mechanism has not been widely accepted. Therefore, further research is required to address these issues.
Based on the analysis presented above, we have modified the mass transfer term in the Z-G-B model by incorporating the flow characteristics of the valve and the velocity strain rate. This modification effectively eliminates the effects of the bubble diameter and nucleation site volume fraction on the mass transfer during the phase change process. Subsequently, we combined an adaptive ensemble of surrogate models with the genetic algorithm to determine the optimal evaporation and condensation coefficients, based on the cavitation length observed in the visual experiment. Finally, the validated high-fidelity cavitation model is employed to explain the evolution mechanism of cavitation under different pressure drops and valve openings.
The paper is organised as follows: Section 2 presents the mathematical models used in the modified cavitation model and the adaptive ensemble of surrogate models. Section 3 provides a detailed account of the construction process of CFD cavitation models, including simplification of the fluid computational domain, verification of mesh independence, and setting boundary conditions. Additionally, we discuss the validation of the physical dimensionality reduction of the modified cavitation model and the optimisation of evaporation/condensation coefficients based on visualisation experiments. Section 4 explores the impact of essential parameters, such as pressure drop between the inlet and outlet and valve opening, on cavitation in safety valves and provides insightful explanations from a physical standpoint to elucidate this relationship. Finally, in Section 5, we summarise our findings and suggest future research considerations.
2. Mathematical models
In this section, a detailed description of the conservation equation for multiphase flow and the turbulence equation is provided to establish the theoretical foundation for simulating two-phase flow in the relief valve. Subsequently, the theory behind the Z-G-B model is presented, which is based on the Rayleigh-Plesset equation and describes mass transfer between the liquid and vapour phases. Furthermore, the modified cavitation model is explained in depth, incorporating the valve's internal flow characteristics and the velocity strain rate to account for the evaporation term. Finally, the construction concept and relevant theory of the adaptive ensemble of surrogate models are presented, which facilitates the establishment of the mapping relationship between evaporation/condensation coefficients and cavitation lengths.
2.1. Governing equations of multiphase flow
In multiphase flow, the conservation equations are fundamental in describing the behaviour of each phase. These equations encompass the continuity, momentum, and energy equations, and their interdependence results in coupling. Solving the conservation equations provides crucial information on the velocity field, phase distribution, and thermodynamic properties of each phase. Therefore, achieving accurate modelling and numerical simulation of multiphase flow is contingent on the proper formulation and thorough comprehension of the conservation equations. In this study, the hydraulic fluid constitutes the primary phase, while the secondary phase comprises the vapour formed through the vaporisation of the hydraulic fluid. As there is minimal temperature change during the phase transition process, the energy equation is omitted. The continuity equation and the momentum equation are presented below.
(1)
(1)
(2)
(2)
Here t is time;
is the mixture density;
is the mass-averaged velocity; P is the pressure;
is the mixture viscosity; and
represents the turbulence viscosity, modelled using the SST
model in our study. The mixture density
, viscosity
, and
are calculated as follows:
(3)
(3)
(4)
(4)
(5)
(5)
Here ,
,
and
are the volume fraction, density, viscosity, and velocity of the
phase, respectively.
The turbulence viscosity and the turbulent kinetic energy k and specific dissipation ω are presented as follows (Mustafa et al., Citation2023; Viitanen et al., Citation2022).
(6)
(6)
(7)
(7)
(8)
(8)
Here where γ, σk, σω, β, β⋆ are model constants. represents the productions of turbulence kinetic energy, which can be written as follows:
(9)
(9)
In Equation (6), the value of parameter a1 is 0.31. S represents the strain rate, which can be write as
, and the
can be wirte as
. The remaining critical parameters in Equations (6)–(8) can be expressed as follows:
(10)
(10)
(11)
(11)
(12)
(12)
2.2. Theory of the cavitation model based on flow scale and velocity strain rate correction
The Z-G-B model, which is a frequently utilised cavitation model in commercial software for cavitation prediction, was originally introduced in 2000 by Zwart, Gerber, and Belamri (Jena et al., Citation2023). The firstly purpose of this model is to accurately capture cavitation resulting from the operation of a two-dimensional hydrofoil and subsequently also has been verified in other fields. In this study, An enhancement Z-G-B model is proposed by incorporating the flow scale associated with valve opening and velocity strain rate. The objective of this enhancement is to mitigate the impact of physical empirical parameters such as bubble diameter and nucleation volume fraction on the cavitation prediction. The modification of the Z-G-B model provides a more accurate prediction of cavitation inception, particularly in systems with varying flow scales. The Z-G-B model has been developed based on the Rayleigh-Plesset equation. Equation (13) presents the expression of the Rayleigh-Plesset equation, which explains the expansion and contraction of a single bubble due to the difference between the internal and external pressure (Geng et al., Citation2020; Snyder et al., Citation2016). This equation serves as the foundation for the development of the Z-G-B model.
(13)
(13)
Here,
is the bubble radius,
is the surface tension coefficient, and
is the vapour pressure.
By neglecting the second-order terms and the effect of surface tension, A simplified expression that shows the change rate of the bubble radius over time is obtained, which can be seen in Equation (14). In order to calculate the mass change rate during the phase change, the nucleation site volume fraction, the evaporation coefficient, and the condensation coefficient are introduced, which can be expressed as Equation (15).
(14)
(14)
(15)
(15)
The parameters recommended for the above equation are
;
;
;
. These coefficients are determined empirically and are applicable only in specific engineering cases. The evaporation (
) and condensation coefficients (
) signify the relaxation time for the mutual transition process between the vapour and liquid phases. The relaxation time is derived in the mass transfer equation between the phases, which is based on bubble dynamics and obtained from the derivative of the bubble radius with respect to the time scale. The time scale is expressed as the ratio of the characteristic flow length to the fluid velocity. Consequently, a strong correlation exists between the evaporation and condensation coefficients and the local flow characteristics. Pope (Citation2000) highlighted that the symmetric part of the velocity strain rate correlates directly with the shear stress. By analogy, this symmetric part can substitute for the local flow characteristics, enabling the linkage of the velocity strain rate with the evaporation and condensation coefficients. Due to the typically elevated flow velocity and significant pressure drop gradient in the cavitation region, the velocity strain rate correction facilitates the rupture and fragmentation of bubbles in areas with higher velocity strain rates, thereby diminishing the bubble diameter. Moreover, regions with higher velocity strain rates experience relatively high shear stress, impeding bubble nucleation and growth, and reducing the nucleation site volume fraction. Furthermore, Asnaghi et al. (Citation2017) employed the velocity strain rate correction method in the Sauer cavitation model to examine the surface pressure distribution of a steady-state two-dimensional hydrofoil under cavitation conditions, successfully diminishing the impact of the empirical parameter (number of bubbles per unit volume, n) on the calculation outcomes. Likewise, a prior study by the authors (Li et al., Citation2023a) effectively eliminated the influence of bubble diameter and nucleation site volume fraction on the mass flow rate of a cavitation jet through an orifice plate, using a velocity strain rate correction within the Z-G-B model.
Building on this modified cavitation model, a characteristic length equation that considers the flow characteristics (valve opening) of the valve is derived. Figure depicts the principle of the calculation of the characteristic length of the relief valve investigated in our study. When the valve opening (xv) occurs, fluid exits the nozzle and forms a curtain surface shown in blue in Figure . This surface corresponds to the ring area with diameter dv, which represents the characteristic length corresponds to valve opening.
(16)
(16)
(17)
(17)
Here
is the characteristic length,
is the velocity of the fluid, davg is the average of the inner and outer diameters of the valve disc. and xv is the valve opening.
Figure 2. The schematic of the calculation of the characteristic length. When the valve is open at position xv, the space between the valve disc and the nozzle forms a ring-shaped region through which the fluid flows, represented by the transparent blue section. This annular region can be considered as a circular area with a characteristic diameter dv, which serves as the characteristic length for further analysis.

2.3. Theory of AES-MSI
The use of surrogate models is prevalent in optimising designs as it replaces complicated and time-consuming numerical analyses (Elmisaoui et al., Citation2023). These models, also known as approximate models or response surface models, significantly enhance efficiency and reduce optimisation difficulty. Common surrogate models include polynomial response surface (PRS), Kriging model (KRG), radial basis function (RBF), and support vector regression (SVR). However, selecting the most suitable surrogate model for a specific problem can be challenging. A model's performance depends not only on its nature but also on the problem and experiment design. There is no single surrogate model that fits all engineering applications. Consequently, using an ensemble of surrogate models is vital to improve predictive capabilities with effective ensemble strategies. In our previous works, the authors proposed an adaptive surrogate model called the Adaptive Ensemble of Surrogate Models by Minimum Screening Index (AES-MSI) model, which demonstrated accuracy and robustness when tested with several test functions (Zhang et al., Citation2022). Therefore, in this study, AES-MSI is utilised to map the relationships between critical parameters in the Z-G-B cavitation model and cavitation lengths. AES-MSI consists of two parts. In part (I), individual surrogate models in the model library are updated based on the screening index to obtain a model subgroup with relatively high accuracy. In part (II), a weight calculation strategy is developed to determine the weight of each individual surrogate model, and AES-MSI is constructed using the obtained weights. The framework of AES-MSI is illustrated in Figure .
2.3.1. Part (I): update the model library
The surrogate models in the original model library mainly consist of four commonly used ones: PRS, RBF, SVR, and KRG. Since the adaptability of these models to different engineering problems varies based on dimensionality, degree of nonlinearity, etc., it is necessary to evaluate their applicability to the research object of this paper. To achieve this, a screening index based on the correlation coefficient and CV is proposed, as shown in Equation (18).
(18)
(18)
where rij is the correlation coefficient between i-th and j-th individual surrogates.
and
are the predictions of i-th and j-th individual surrogates, respectively.
and
are the predictions mean value of i-th and j-th individual surrogates, respectively.
Corresponding to the model library constructed in this paper, the correlation coefficient between i-th individual surrogate and the other four individual surrogates can be written as:
(19)
(19)
Then, construct the screening index as follow:
(20)
(20)
where SIi is the screening index of i-th individual surrogates.
represents max correlation coefficient in ri.
A larger indicates the i-th surrogate is highly correlated with an individual surrogate in the model library, which means that the model has relatively better prediction accuracy. Conversely, a smaller MCVi indicates better performance of the individual surrogate. Therefore, a smaller SIi value represents a better performance of the individual surrogate. To compare the performance and exclude the influence of large SIi values of individual surrogates, we calculate the normalised SIi using the following formula:
(21)
(21)
where SImax and SImin are the maximum and minimum value of SIi in the model library, respectively.
2.3.2. Part (II): calculation of adaptive weights
This methodology integrates global trends and local errors to determine the weights of each surrogate model in adaptive ensemble. The baseline surrogate model, with the smallest NSI, represents the global trend. The local error is calculated as the difference between the other surrogate models and the baseline surrogate model, as shown in Equation (22). A smaller value of eij indicates a greater weight of the individual surrogate in the ensemble.
(22)
(22)
where eij represents local error at j-th sample point of i-th individual surrogate.
and
are the prediction value of the i-th individual surrogate and the baseline surrogate at the j-th sample point, respectively.
The normalised error is then calculated by combining the local error of each surrogate, including the baseline surrogate. It is noted that e is a vector, which includes the local error of each predicted value. Thus, there is a weight for all predicted values of each surrogate.
(23)
(23)
The weight of each surrogate in the ensemble is composed of two parts: a global trend weight represented by the baseline surrogate, and a weight based on the local errors. To balance these two parts, we set the weights of the baseline surrogate.
(24)
(24)
(25)
(25)
where
and
are the weights of baseline surrogate and the rest of the surrogates at j-th sample point.
3. CFD cavitation simulation of the safety relief valve
In this section, we present a comprehensive description of the numerical simulation of the cavitation phenomenon in relief valves. Firstly, we discuss the simplification and meshing process of the computational domain of the relief valve. Next, we define the cavitation length as a criterion to quantify the cavitation phenomenon, taking into account the specific characteristics of the relief valve under study. Additionally, we construct a mesh-independent validation by examining this parameter, demonstrating that our proposed numerical method is not sensitive to the distribution of the computational grid. We also provide detailed information about the boundary conditions, which are set based on actual working conditions. Furthermore, we verify the effectiveness of the physical dimensionality reduction achieved through the use of the modified evaporation coefficient, which is corrected based on the velocity strain rate. The bubble diameter and nucleation site density serve as indicators to validate this reduction. Lastly, we establish a relationship between the evaporation/condensation coefficients and cavitation lengths, employing the AES-MSI method. Grayscale detection techniques are applied to visualise experiments, and the resulting cavitation lengths are utilised as targets for parameter optimisation using a Genetic Algorithm. Through these steps, we develop a high-fidelity cavitation model specifically tailored for relief valves.
3.1. Computational domain simplification and mesh generation
In our previous research, the computational domain modelling and mesh generation techniques were successfully employed to analyze safety valves in the second circuit of nuclear power plants for predicting fluid forces acting on the valve disc (Li et al., Citation2023b). In this study, we extend our previous work by increasing the valve inlet pressure and directing our attention to cavitation inside the valve. Since the only difference between the two operating conditions is the pressure, we can utilise the same fluid domain partitioning and mesh generation techniques used in the previous work. Figure (a) presents a 3D model of a direct-acting relief valve comprising five essential components: preload screws, spring, valve seat, valve body, and valve disc. In Figure (b), a simplified fluid domain model based on the 3D model depicted in Figure (a) is shown. To generate a pure hexahedral mesh, the fluid computational domain is divided into four crucial sections, namely the inlet, turbulent core, valve chamber, and outlet domains. The interfaces facilitate data transfer between different sub-fluid domains. Figure (c) illustrates the mesh that has been generated for the fluid domain. To enhance computational efficiency, all meshes have been partitioned into hexahedral meshes. Moreover, the O-block method has been employed to generate the mesh while ensuring that it satisfies the quality criteria for meshes with circular boundaries.
Figure 4. The calculation model configuration of the safety relief valve. (a) presents a semi-profile 3D model of the relief valve, offering a clear depiction of its key components. (b) presents the fluid computational domain obtained by employing the split computational domain processing method. Various sub-domains are highlighted using distinct colours, and data exchange between these sub-domains is facilitated through Interfaces. (c) showcases the resulting mesh division, where hexahedral meshes are employed throughout using the ‘O-block’ approach. This strategy not only optimises computational resources but also enhances computational convergence.

3.2. Independence verification of mesh and turbulence models
Based on the computational domain and mesh method described in the previous section, this section presents details of the grid independence verification and turbulence models. Numerical simulations’ outcomes are heavily impacted by these two factors. Two critical factors in the grid independence verification are the first layer height near the wall and the mesh density. The first layer height is crucial for accurate prediction of the flow near the wall, and its reasonableness is often evaluated using y+. In our study, the SST k-ω model is employed, and it requires the y+ value of the valve disc in the cavitation region to be close to 1. Therefore, a first layer height of 1E-6 m is selected for this region, and the distribution of y+ of the valve disc is presented in Figure . Subsequently, we performed grid independence verification using the cavitation length as the target, selecting five different grid sizes (2, 1, 0.5, 0.2, and 0.1 mm) based on the first layer height.
The cavitation region of the relief valve under investigation forms an approximate annular cavitation zone with a half-elliptical cross-section around the axis of the valve (as shown in Figure ). In our study, we define two cavitation lengths: the radial cavitation length (xlength) and the axial cavitation length (ylength). The xlength is calculated as the difference between the length at the start and end of cavitation, using Equation (26). The ylength is computed from the bubble's volume, which is the volume of the bubble swept by half of the elliptical section around the centre of the section, using Equation (27). The mesh independence verification was conducted under specific boundary conditions, including 1.5 MPa inlet pressure, 0 MPa outlet pressure, and 0.5 mm valve opening, while keeping the parameters of the Z-G-B cavitation model at their default values. Table compares the cavitation length under five different mesh densities. It shows that with increasing mesh density, both xlength and ylength slightly increase. However, when the mesh density reaches 0.2 mm, the two cavitation length stabilise with a variation rate of less than 1%. Hence, 0.2 mm is considered the optimal mesh density and is adopted as the final mesh density.
(26)
(26)
(27)
(27)
Turbulence models are crucial for accurately predicting cavitation in engineering applications. Accurate prediction of cavitation requires accounting for turbulence effects, particularly in modelling complex flows with vortices or high shear flows. Therefore, selecting and validating an appropriate turbulence model for specific flow conditions is essential to ensure accurate predictions of cavitation phenomenon. In this study, we discuss five different turbulence models (Standard k-ε, RNG k-ε, Realisable k-ε, Standard k-ω, and SST k-ω) to determine the most suitable turbulence model. Figure shows the cavitation lengths (xlength and ylength) predicted by various turbulence models for the analyzed relief valves. Based on Figure , the cavitation length predictions by the turbulence models can be grouped into two categories: the k-ε turbulence model and the k-ω turbulence model. Among the turbulence models developed from the k-ε model, the RNG k-ε model predicts the longest cavitation length, while the Standard k-ε model predicts the smallest. As for the turbulence model based on the k-ω model, both the xlength are 3.5 mm, while the ylength are larger than those of the Standard k-ω model. According to the comparison with experimental results, the cavitation lengths predicted based on the k-ω model are more accurate. This finding will be further emphasised in the subsequent experimental section. Therefore, we selected the SST k-ω model to predict the cavitation length due to its accurate prediction in our study.
Figure 6. Represents the radial dimension (xlength) and axial dimension (ylength) of the cavitation area in the relief valve. The blue region represents a portion of the fluid domain surrounding the valve disc and seat. As cavitation exclusively transpires within the gap formed between the valve disc and seat, this specific area is magnified for clarity. Meanwhile, the red area denotes the cavitation region, taking the form of a ring-shaped region obtained by sweeping an ellipse as a cross-section with the ellipse's centre serving as the radius.

Figure 7. The effect of different turbulence models on cavitation length. The figure depicts the cavitation dimensions with different turbulence models, with the red bars representing the radial dimension, xlength, and aligned with the left axis. Conversely, the blue bars represent the axial dimension, ylength, corresponding to the right axis.

Table 1. The comparison of cavitation length with different numbers of meshes.
3.3. Boundary conditions
Boundary conditions define the behaviour of fluids at the edges or boundaries of the analyzed domain, including walls, inlets, outlets, and interfaces. They dictate the flow parameters, such as velocity, pressure, and species concentration, at these boundaries. Properly selecting and specifying boundary conditions is crucial to ensure the accuracy and reliability of CFD simulations. Inaccurate or inappropriate boundary conditions can lead to erroneous outcomes or convergence issues, rendering the simulation invalid. Therefore, this section presents a comprehensive description of the boundary conditions based on real working conditions.
Half of the model is used to reduce computational resources due to its symmetry, and a symmetric boundary is employed for the fluid domain model. Pressure boundary conditions are set for both the inlet and outlet. The mixture model describes the phase transition process in the cavitation, where the primary phase is oil, and the secondary phase is oil-vapour. Table presents the physical properties of the two media. User Defined Functions (UDFs) are employed to compile the two-phase flow mechanism based on the Z-G-B cavitation model's mass transfer equations, with the mass transfer term modified by the velocity strain rate. Equations (15)–(17) present these equations. The acceleration in the y direction is set to 9.81 m/s2 to account for the fluid's gravity and the formation of bubbles. The coupled solver is used due to its robustness and accelerated convergence, especially for high-pressure cavitation jets. Second-order upwind schemes are used for discretization of momentum, turbulence kinetic energy, and turbulence dissipation rate. To monitor convergence, the continuity equation, x-directional velocity, y-directional velocity, z-directional velocity, turbulent kinetic energy, and turbulent dissipation rate are considered. Convergence is achieved when the residual values of these physical variables fall below 1E-4. The solution algorithm and spatial discretization method are listed in Table .
Table 2. Material properties of the flow medium.
Table 3. The solution algorithm and the spatial discretization method.
3.4. Validation of the implementation effect of physical dimensionality reduction
As discussed in Section 2.2, implementing the velocity strain rate correction to the mass transfer term in the Z-G-B cavitation model, in conjunction with the valve flow length, can significantly reduce physical dimensionality. This section aims to validate the effectiveness of this approach by examining the cavitation length, specifically the bubble diameter (Db) and nucleation site volume fraction (αnuc). Figure compares the cavitation length predicted by the modified and original cavitation models for the bubble diameter (Db). The radial and axial cavitation lengths are represented by Figure (a and b), respectively. The red and blue dotted lines in Figure (a) represent the variation curves of xlength calculated by the original and modified cavitation models, respectively. The red and blue bars show the change rate of the original and modified cavitation models compared to the recommended bubble diameter (Db = 1E-6 m), respectively. The results indicate that xlength decreases as Db increases. The gradient of change in the cavitation length is more drastic when Db is larger than the recommended value, and more moderate when it is smaller. The modified cavitation model effectively eliminates the effect of the Db on the xlength, reducing the maximum error from 57% to 11.2%. In Figure (b), the axial cavitation lengths (ylength) predicted by both models decrease when the Db is less than the recommended value. However, when the Db is larger than the recommended value, the two models predict opposite trends. The error of the modified cavitation models are smaller than those of the original cavitation model, reducing the maximum error from 12.5% to 8.7%. Overall, the modified cavitation model effectively mitigates the influence of the bubble diameter (Db) on the prediction of the cavitation length.
Figure 8. The effect of the modified cavitation model on the cavitation length compared with the original model for the bubble diameter. (a) and (b) represent the comparison between the original cavitation model and the modified cavitation model in terms of xlength and ylength for different bubble diameters, respectively. The bar charts in red and blue represent the cavitation lengths of the original and modified models, respectively, and are aligned with the left-hand axis. The dotted line plots in red and blue represent the cavitation lengths of the original and modified models, respectively, and are aligned with the right-hand axis.

Figure illustrates a comparison between the cavitation length predicted by the modified and original cavitation models for the αnuc. The dotted line plots and bar graphs represent the same features as in Figure , with the exception of the independent variable (Figure for αnuc). From Figure (a), it is evident that the xlength predicted by the original cavitation model gradually increases with an increase in αnuc, and the change is most significant when αnuc is less than the recommended value (αnuc = 5E-4). In contrast, the modified cavitation model shows only a slight decrease with an increase in αnuc. A comparison of the errors between the predicted and recommended values of the two cavitation models under different values of αnuc reveals that the modified cavitation model effectively suppresses the influence of αnuc on the xlength prediction and reduces the maximum error from 63% to within 5%. As shown in Figure (b), the prediction of ylength for both cavitation models increases with increasing αnuc, but the change in ylength prediction for the original model is more pronounced when αnuc is less than the recommended value (αnuc = 5E-4). A comparison of the bars reveals that the errors calculated by the original model relative to the recommended values at different αnuc are greater than those predicted by the modified cavitation model, and the maximum error is reduced from 22% to within 10%. In summary, the modified cavitation model effectively suppresses the effects of Db and αnuc variations on the prediction results of the cavitation length, and achieves a physical dimensionality reduction of the cavitation model.
Figure 9. The effect of the modified cavitation model on the cavitation length compared with the original model for the nucleation site volume fraction. (a) and (b) represent the comparison between the original cavitation model and the modified cavitation model in terms of xlength and ylength for different nucleation site volume fraction, respectively. The bar charts in red and blue represent the cavitation lengths of the original and modified models, respectively, and are aligned with the left-hand axis. The dotted line plots in red and blue represent the cavitation lengths of the original and modified models, respectively, and are aligned with the right-hand axis.

3.5. The construction of AES-MSI based on the evaporation and condensation coefficients
Based on the analysis in Section 3.4, the impact of Db and αnuc on the cavitation length is effectively reduced by correcting the evaporation term using the velocity strain rate correction. This section explores the relationship between the evaporation/condensation coefficients and cavitation lengths using the AES-MSI method. The evaporation coefficient's default value is 50. To explore a range of values, we varied it up or down by an integer factor from the default value, defining the sample space as [5E-1, 5E1]. The condensation coefficient has a default value of 1E-2, and we established a sample space of [1E-2, 1E0] by varying the coefficient up or down by an integer factor from its default value. To establish the relationship between evaporation/condensation coefficients and cavitation lengths, it is essential to effectively sample the design variables within the designated sample space. Subsequently, the cavitation lengths corresponding to the sample points are computed using CFD numerical models. The present study utilises the optimal Latin hypercube sampling (LHS) method to sample the evaporation and condensation coefficients. Since the design variables in this study are multiples of 10 and the ranges have an extreme difference of a factor of 100, the design space undergoes logarithmic transformation to achieve the highest possible sampling uniformity.
Figure displays the distribution of the training points and test points in the sample space using the logarithmic coordinate system. The study uses a dataset of 34 points, including 30 uniformly distributed sample points and 4 boundary points, represented by black and blue circles, respectively. The training points are chosen based on the principle criteria proposed by Forrester and Jones, which require a number of training points exceeding ten times the number of dimensions. Additionally, 10 uniformly distributed validation points, represented by red circles, are employed to evaluate the surrogate model's accuracy. The CFD calculations for both the training and validation datasets utilise specific boundary conditions, namely, a valve opening of 0.5 mm and an inlet pressure of 1.5 MPa. The remaining settings align with those described in Section 3.2–3.3.
Figure 10. The distribution of the training points and validation points in the sample space. The black points in the figure represent the training points utilised to construct the surrogate model. The red points indicate the validation points employed to assess the accuracy of the surrogate model. To avoid extrapolation, the authors introduced four additional boundary points, illustrated by the blue points.

The relationship between evaporation/condensation coefficients and cavitation length is established using the AES-MSI method, as described in Section 2.3, based on the CFD numerical simulation results at specific sample points. The evaporation and condensation coefficients are treated as input variables, while the cavitation lengths are considered as the output variable. To assess the performance of AES-MSI, four commonly used engineering surrogate models (PRS, RBF, KRG, and SVR) are compared. Since relying on a single indicator for evaluation can be limiting, this study employs three evaluation indicators to assess the surrogate model's accuracy. These indicators are the coefficient of determination (R2), root mean square error (RMSE), and mean absolute percentage error (MAPE). R2 is a widely used metric to visually evaluate the model fit, ranging between 0 and 1, with values closer to 1 indicating a better fit to the data. Both RMSE and MAPE visually represent the magnitude of the model's prediction error, with smaller values indicating better prediction performance. The results of the prediction accuracy evaluation for the five surrogate models are presented in Tables and . The surrogate model constructed by AES-MSI have the highest R2 value (closest to 1), the smallest RMSE and MAPE values, demonstrating the accuracy of AES-MSI in constructing the mapping relationship between the evaporation/condensation coefficient and cavitation lengths.
Table 4. Three evaluation indexes of five surrogate models for the prediction of xlength.
Table 5. Three evaluation indexes of five surrogate models for the prediction of ylength.
The constructed surrogate model provides a rapid way to access the cavitation length at specific evaporation and condensation coefficients, which is crucial for determining the optimal coefficients for both processes. Figure show the surface correlation among the cavitation lengths, evaporation coefficient, and condensation coefficient, generated using AES-MSI. Figure (a and b) depict xlength and ylength as functions of evaporation and condensation coefficients, respectively. From Figure (a), it can be observed that the relationship between evaporation coefficient, condensation coefficient, and xlength is bowl-shaped. When either the evaporation or condensation coefficient remains constant while the other parameter gradually increases, xlength increases in a quadratic trend with a decreasing gradient of change, indicating a positive correlation between xlength and evaporation/condensation coefficients. The maximum xlength value appears in the upper left corner of Figure (a) (looking at the xlength – Lg(Fcond) plane), corresponding to the position where the evaporation/condensation coefficient are at their maximum. Conversely, the minimum xlength value appears in the lower right corner of Figure (a) (looking at the xlength – Lg(Fcond) plane), corresponding to the position where the evaporation/condensation coefficient are at their minimum. The relationship between evaporation and condensation coefficients and ylength shown in Figure (b) differs slightly from Figure (a). The maximum value appears at the same location as in Figure (a), although the ylength in the lower right corner is smaller. However, the global minimum value appears in the lower left corner. ylength tends to increase as the condensation coefficient decreases when the evaporation coefficient is small, while ylength tends to decrease as the condensation coefficient decreases when the evaporation coefficient is larger.
Figure 11. The surface correlation among the cavitation lengths, evaporation coefficient, and condensation coefficient. (a) and (b) represent the evaporation/condensation coefficients plotted against xlength and ylength, respectively, using a logarithmic scale for improved visualisation. The colour gradient ranging from red to blue indicates the variation of the coefficients, with red indicating the maximum value and blue indicating the minimum value. The accompanying colour bar provides a reference for the magnitude of the coefficients.

3.6. Visualisation experiments and genetic algorithms for determining evaporative condensation coefficients
Once the relationship between the evaporation/condensation coefficients and cavitation lengths has been established, it becomes crucial to determine these coefficients based on the cavitation lengths obtained under actual operating conditions. This section provides a detailed description of the experimental procedure and the independently built test rig used to measure cavitation phenomena. The cavitation lengths measured during the experiment are used as the target to find the optimal values for the evaporation/condensation coefficients of the relief valve using genetic algorithms. In our previous study (Li et al., Citation2023b), we conducted experimental measurements on the fluid forces exerted on the valve disc of a relief valve using a hydraulic bench. These experiments yielded high test accuracy and demonstrated good experimental repeatability. Building upon this hydraulic test rig setup, we further investigated the cavitation phenomenon occurring within the valve by integrating visualisation equipment. The hydraulic test rig are depicted in Figure .
Figure 12. The test rig for observing cavitation. (a) depicts the comprehensive layout of the experimental setup, with the control cabinet, represented by the white cabinet, serving as the central unit for oil pressure regulation. The oil supply system comprises the motor and oil tank, while the monitor enables the visualisation of the cavitation area surrounding the valve disc and valve seat. (b) represents the visualisation system, encompassing the CCD camera, industrial microscope, and the valve system under examination. (c) showcases the data acquisition system, which consists of the signal amplifier and an NI acquisition card. (d) depicts the valve opening adjustment mechanism, primarily comprises a linear motion system and a laser displacement sensor. (e) provides a detailed view of the transparent test valve and the valve opening adjustment mechanism.

The experimental setup consists of various components, such as a tank and motor-driven oil supply system, an oil pressure control system based on PID regulation, a data acquisition system using NI data acquisition cards, and a visualisation system that includes a transparent test valve and a high-definition (HD) industrial microscope. This study specifically focuses on the visualisation experimental system, which includes transparent test valves, an electronic magnifier system, a valve opening adjustment mechanism, and upstream and downstream pressure monitoring systems.
The transparent test valves are made of PMMA material, providing excellent visualisation capabilities to observe the flow conditions inside the valves. The visualisation system consists of a high-definition industrial microscope, a high-performance CCD camera, and brackets. The system allows adjustable optical magnification ranging from 21x to 135x, adjustable lens magnification from 0.7x to 4.5x, and can capture images with up to 4800 w pixels. The valve opening adjustment mechanism primarily consists of a rack-and-pinion-based linear motion mechanism and a laser displacement sensor. The linear motion mechanism features a scale bar with an adjustment accuracy of 0.01 mm. By rotating the adjustment knob, the valve opening can be adjusted within a maximum range of 5 mm, and the opening can be fixed using the locking nut. The laser displacement sensor measures the valve disc opening by projecting a laser onto the iron piece mounted on the spool, allowing for precise verification of the valve opening set by the linear mechanism.
The experimental procedure is exemplified using a valve opening of 0.5 mm and an inlet pressure of 1.5 MPa. Firstly, the power should be turned on, and a small pressure should be entered to allow the equipment to run for a while. This will ensure that there are no fluid leaks in the pipeline and that the pressure values displayed on the sensor match those from the data acquisition system. In the second step, the initial position of the adjustment mechanism relative to the scale when the valve disc is at 0 mm valve opening should be confirmed. The adjusting screw should then be rotated to adjust the displacement of the valve spool to 0.5 mm, and the laser displacement sensor value should be compared to confirm the accuracy of the valve opening. In the third step, the pressure sensor in front of the valve should be stable, and the cavitation image detected from the display should not change. Once this condition is met, the cavitation image should be recorded, marking the end of the cavitation test process. Figure (a) presents the cavitation region of the relief valve, observed through an electron magnifying lens. In this image, the yellow area represents oil, the upper portion of the bright gray area corresponds to the valve disc, the lower portion represents the nozzle, and the black area below the valve disc indicates the cavitation zone. To determine the cavitation length, our study employs digital image processing techniques based on grayscale detection, commonly used for identifying two-phase flows (Liu et al., Citation2019; Soyama & Hoshino, Citation2016). To reduce the number of pixels requiring calculation, images are cropped to appropriate regions. The average gray value (G) is determined by calculating the average value of the gray values along the same horizontal coordinate, as shown in Equation (28). And a threshold for defining the cavitation initiation and collapse termination points is defined follows the method proposed by Liu et al. (Citation2019), namely, λs = (λmax + λmin)/2. If the gray value (λ) is greater than or equal to λs, the distance from the collapse to the starting boundary represents the cavitation length.
(28)
(28)
(29)
(29)
Figure (b) depicts the grayscale imagine obtained using the aforementioned method, where the white region represents the cavitation area determined through grayscale detection. In Figure (c), the grayscale values are plotted against the x-axis coordinates to illustrate their variation. Subsequently, we present the dimensional measurements of the cavitation region. It exhibits a radial length of 3.67 mm (referred to as xlength) and an axial length of 0.22 mm (referred to as ylength). Next, the genetic algorithm is employed to optimise the evaporation and condensation coefficients based on the relationship constructed by AES-MSI. The optimisation objectives and constraints are presented in Equation (29). Specifically, the optimisation of evaporation and condensation coefficients involves two objectives and two constraints. The two objectives are to minimise the difference between the experimental values and the predicted values of xlength and ylength, which are surrogate model outputs. The two constraints are to limit the range of values of evaporation and condensation coefficients. In this context, xlength represents the radial cavitation dimension that influences the cavitation length on the valve disc in the engineering problem. Moreover, ylength represents the axial cavitation length that directly affects the mass flow rate of the valve by obstructing the passage of fluid. Given these objectives and constraints, both objectives are weighted equally at 0.5 to select the optimal solution from the pareto solution set. Accordingly, the final evaporation and condensation coefficients are determined to be 5E0.757 and 1E-1.830, respectively. Figure presents the vapour volume contour (the cavitation region) of the relief valve under seven different calculation conditions, including the Standard k-ε, Realisable k-ε, RNG k-ε, SST k-ω, Standard k-ω, MSST k-ω (a modified cavitation model corrected by velocity strain rate), and OMSST k-ω (an optimised cavitation model combining the flow characteristics of the valve, velocity strain rate correction, AES-MSI surrogate model, and genetic algorithm). Since cavitation only occurs in the valve disc region, Figure displays only the valve disc region, with the cavitation region highlighted in red. The highlighted area represents an iso-surface with a gas-phase volume fraction greater than 0.5.
Figure 13. The method of cavitation length calculation. (a) is the cavitation region of the relief valve, observed through an electron magnifying lens. In this image, the yellow area represents oil, the upper portion of the bright gray area corresponds to the valve disc, the lower portion represents the nozzle, and the black area below the valve disc indicates the cavitation zone. (b) represents the grayscale map obtained using the grayscale detection method, where the bright white region represents the cavitation area. (c) represents the variation of the grayscale values with the x-axis coordinates to illustrate the cavitation length.

Figure 14. Comparison of cavitation regions for different turbulence models. (a) Standard k-ε. (b) Realisable k-ε. (c) RNG k-ε. (d) SST k-ω. (e) Standard k-ω. (f) MSST k-ω. (g) OMSST k-ω.

Table shows the cavitation lengths predicted by these seven calculation methods. The results indicate that k-ω-based models predict cavitation lengths that are in better agreement with experimental values than k-ε-based models. Among the four calculation methods based on the k-ω turbulence model, our proposed cavitation prediction method exhibits the highest prediction accuracy, with prediction errors of xlength and ylength reduced to 0.81% and 1.72%, respectively. Furthermore, to further validate the accuracy of the simulation, we compared the cavitation lengths obtained from experiments and simulations under two operating conditions: valve openings of 0.7 and 0.9 mm. These evaporation/condensation coefficients were chosen based on the optimised coefficients mentioned above. The comparison results are presented in Figure .
Figure 15. Comparison of experimental and simulated cavitation lengths at different valve openings. (a1)-(a3) represent the simulated cavitation length, the experimental cavitation length, and grayscale curve at 0.7 mm opening, respectively. (b1)-(b3) represent the the simulated cavitation length, the experimental cavitation length, and grayscale curve at 0.9 mm opening, respectively.

Table 6. Three evaluation indexes of five surrogate models for the prediction of ylength.
4. Analysis of cavitation evolution based on modified cavitation model
In this section, we investigate the impact of two crucial operating parameters, namely the pressure difference between inlet and outlet (hereafter referred to as ) and valve opening, on cavitation in relief valves. Using the high-fidelity CFD cavitation model, we provide insights into the influence of
on valve cavitation by examining factors such as fluid flow obstruction caused by vortices and turbulence. This analysis is supported by velocity contours and streamlines. Additionally, employing the same high-fidelity CFD cavitation model, we simulate the cavitation phenomenon for various valve openings and analyze the corresponding changes in the cavitation area. To facilitate this analysis, we propose a flow area calculation formula.
4.1. Effect of 
on the cavitation evolution
is a critical factor that affects the cavitation phenomenon of safety valves. Variations in
can result in different fluid flow velocities through valve openings, thereby creating diverse local low-pressure zones and inducing varying degrees of cavitation. By conducting a comprehensive investigation of the impact of
on the cavitation phenomenon, it is possible to optimise the design parameters and operating conditions of safety valves to ensure the secure operation of equipment. The investigation for various
is conducted using a valve opening of 0.5 mm, while keeping the other parameter settings for the numerical simulations consistent with those presented above. Figure displays the cavitation contours for various
ranging from 0.5 to 1.5 MPa. For space constraints, only four of them (0.6, 0.8, 1.0, and 1.2 MPa) are presented in the main text, while the remainder will be presented in Appendix A. As evident from Figure and Appendix A, the area of cavitation (highlighted in red) occurs in the gap formed between the valve disc and seat. The reduction in fluid flow area at this point causes an increase in flow velocity, leading to a local pressure drop below the saturation vapour pressure, ultimately resulting in cavitation. Observing these cavitation clouds reveals that the cavitation region gradually expands with an increase in
. However, the cavitation area does not change beyond a certain critical pressure, and the degree of cavitation differs between the left and right sides of the valve disc due to the valve's asymmetric structure, having only one side outlet. To gain a deeper understanding of the correlation between the cavitation region and pressure, the cavitation length defined in Section 3.2 is employed to measure the extent of cavitation at varying pressure drop, as presented in Figure .
Figure 17. Cavitation length for various . The figure categorises the cavitation length into two groups based on their proximity to the outlet on either side of the valve disc, as indicated by the red and black colour. By examining the characteristics of the curves, the cavitation phenomenon can be classified into three distinct stages: the initial cavitation stage, the development cavitation stage, and the stable cavitation stage.

In previous literature, the valves cavitation process with varying pressure has been categorised into three stages: the no-cavitation stage, the initial cavitation stage, and the stable cavitation stage. In this study, the three stages mentioned above have been further refined based on the varying degrees of cavitation around the valve disc. Figure considers the rate of change of the radial cavitation length (xlength) and the difference in xlength between the two sides of the valve disc (i.e. close/far away from the valve outlet) to classify cavitation into three stages: initial cavitation, developing cavitation, and stable cavitation. When is less than 0.7, if the xlength is less than 0.1 mm, which considered that xlength remains unchanged with
. Additionally, xlength is consistent on both sides of the valve disc within this range of
, thus defining it as the initial stage of cavitation. When
is greater than 0.7 and less than 1.3, the xlength exceeds 0.1 mm, which exhibits a significant increasing gradient with
. At this time, xlength on the left and right sides of the valve disc starts to differ, and this difference progressively amplifies. Consequently, this range of
is designated as the development stage of cavitation. When
exceeds 1.3, the rate of change of xlength is less than 2%, which remains constant with
. Additionally, the xlength on the left and right sides of the valve disc tends to equalise. Hence, this range of
is categorised as the cavitation stabilisation stage.
To investigate the reasons why cavitation lengths on both sides of the valve disc differ in the developing and stable cavitation stages, yet ultimately show consistency, and why the scales gradually increase and then stabilise with rising pressure, we analyzed the flow characteristics of the valve at various pressures based on the high-fidelity CFD cavitation model. Figure displays the velocity contours, streamlines, pressure contours and vapour contours of the valve at 0.7, 1.2, and 1.5 MPa. From the velocity contours, it can be observed that the flow velocity at the three different follow a similar trend. As the fluid enters the nozzle, the flow area decreases, leading to an increase in velocity. As the fluid exits the nozzle and enters the valve disc groove region, the through-flow area increases, leading to a decrease in flow velocity. When the fluid reaches the bottom of the valve disc groove, it is divided into two pathways based on the centre of the valve disc (represented by the black centre line). The fluid is accelerated as it passes through the bottom of the valve disc groove (black solid line) and flows into the gap formed by the valve disc and seat (jet flow region) through the valve disc wall (purple solid line). In this region, the flow velocity increases due to the reduction in flow area, leading to a relatively large pressure drop (as shown in Figure (a3–c3)) and the formation of a cavitation area (as shown in Figure (a4–c4)). The velocity contours (as shown in Figure (a1–c1)) at various pressures with the primary difference being the size of the high-speed region (as indicated by the black line) that increases with higher pressure. At pressures of 0.7 and 1.5 MPa, the size of the high-speed region on both sides of the valve disc is equal, whereas at 1.2 MPa, the size of the high-speed region on the left side is smaller than that on the right side. A larger high-speed region corresponds to a greater pressure gradient drop, resulting in a larger cavitation length. This is consistent with Figure , which shows that the size of the cavitation region on either side of the valve disc is different at 1.2 MPa but the same at 0.7 and 1.5 MPa.
Figure 18. The velocity contours, streamlines, pressure contours and vapour contours of the valve for three kinds of . where (a), (b) and (c) represent the three
(0.7, 1.2, and 1.5 MPa), respectively. Numbers 1, 2, 3 and 4 represent velocity contours, streamlines, pressure contours and vapour contours, respectively.
The analysis above indicates that differences in flow characteristics are the primary cause of varying cavitation lengths on either side of the valve disc. To investigate the causes of the different high-speed zones on either side of the valve disc, Figure (a2–c2) shows the streamlines at three typical . At a
of 0.7 MPa, the fluid flows through the gap formed between the valve disc and the valve seat on the left side and then moves upwards along the wall. Due to the shear force of the wall and the viscous force of the fluid, the fluid gradually decelerates and forms a vortex, which subsequently flows to the outlet through the upper part of hole 1. As the
increases to 1.2 MPa, the flow velocity of the fluid on the left side also increases, resulting in the formation of more vortices, and hence, obstruction of the flow (Martin & Bhushan, Citation2014). This obstructive effect leads to a shorter high-speed area on the left side of the valve disc. Meanwhile, Most of the fluid on the right side of the valve disc flows directly from hole 1, and only a small portion of it flows through hole 2. As a result, the high-speed area on the right side of the valve disc is larger than that on the left side, leading to different cavitation lengths on the two sides of the valve disc. With further increase in pressure, the fluid on the left side of the valve disc no longer moves upward along the wall due to the higher speed. Instead, it directly impacts the wall and flows into the lower part of hole 1, as shown in Figure (c2), resulting in an increased flow capacity due to the lack of vortex obstruction. At this stage, the left and right sides of the valve disc present almost the same high-speed area size, leading to the formation of the same cavitation length.
By employing our developed high-fidelity CFD cavitation model, together with velocity contours and streamlines, we have discovered a strong correlation between the length of cavitation and the extent of the high-speed zone in the gap region (formed by the valve disc and seat). Moreover, it has been determined that high-speed flow plays a critical role in initiating turbulence, wherein turbulence intensity serves as a valuable parameter for assessing and controlling turbulence dynamics. Considering the aforementioned perspective, it becomes feasible to establish a correlation between turbulence intensity and cavitation. Turbulence intensity is defined by the ratio of the root-mean-square of velocity fluctuations to the mean flow velocity, as expressed in Equation (30).
(30)
(30)
where
is the turbulence intensity,
is the root-mean-square of the velocity fluctuations,
is the mean flow velocity.
Figure (a) depicts 9 circular faces intercepted in the cavitation region along the flow direction, with each face spaced 0.5 mm apart. Figure (b) shows the distribution of turbulence intensity on the 9 surfaces at different pressures. It can be observed from Figure (b) that the overall trend of turbulence intensity increases with the rise of pressure. When the pressure is constant, the turbulence intensity tends to increase and then decrease from face 1 to face 9, reaching a maximum between 1 and 2 mm, and then gradually decreasing as the pressure rises, causing the degree of cavitation to decrease. Two critical points of cavitation length are 0.7 and 1.3 MPa in Figure . From Figure (b) it is evident that the flow can be classified into three categories based on turbulence intensity. When the pressure is below 0.7 MPa, the turbulence intensity is almost flat, corresponding to the initial cavitation stage in Figure . The turbulence intensity begins to show a clear trend of increasing and then decreasing when the pressure is between 0.7 and 1.3 MPa. The turbulence intensity at the tail of the flow (distance of 3.5–4 mm) shows a parallel trend at all pressures in this range, corresponding to the developing cavitation stage in Figure . When the pressure is greater than 1.3 MPa, the maximum turbulence intensity gradually shifts backward and appears in the region of 1.5–2 mm, while at the tail of the flow (distance of 3.5–4 mm), the turbulence intensity appears to rise abruptly (no longer parallel trend). The effect of turbulence inhibits the further development of cavitation because the flow becomes turbulent, which corresponds to the stable cavitation stage in Figure .
4.2. Effect of valve opening on the cavitation evolution
The valve opening is a critical parameter in regulating fluid velocity and pressure distribution in a pipeline. Decreased valve opening results in reduced fluid flow and an increase in fluid velocity, which in turn alters the pressure distribution. These conditions may create localised pressure reductions, potentially leading to liquid vaporisation. Conversely, fully opening the valve allows for maximum fluid circulation through the valve cross-section, resulting in more uniform fluid velocity and pressure distribution, reducing the risk of vaporisation due to a more even pressure and temperature distribution. However, the complex flow dynamics of the relief valve nozzle and valve disc area complicate the relationship between valve opening and cavitation. Therefore, a more in-depth study is necessary to fully comprehend the impact of valve opening on cavitation. The investigation for various valve openings is conducted using the of 1.5 MPa, while keeping the other parameter settings for the numerical simulations consistent with those presented above. Figure displays the vapour phase contour distribution at valve openings between 0.5 and 1.5 mm. However, due to spatial constraints, only partial valve openings are presented, while the vapour phase contours for all valve openings can be found in Appendix B. This paper divides the cavitation region into two sections: region 1 and region 2, which are determined based on the location of cavitation occurrence. Region 1 (as shown in Figure (a1) marked by red) is formed between the valve disc and the valve seat, while region 2 (as shown in Figure (a3) marked by red) refers to the nozzle region. Analysis of Figure and Appendix B indicates that as the valve opening increases, the cavitation length in region 1 gradually shortens and disappears entirely. Additionally, with valve opening increases, cavitation emerges in region 2. The cavitation length of region 2 increases gradually before stabilising.
Figure 20. The vapour phase contour distribution at different valve openings. (a1) 0.6 mm. (a2) 0.8 mm. (a3) 0.9 mm. (a4) 1.0 mm. (a5) 1.2 mm. (a6) 1.5 mm.

To further quantify the variation in cavitation length with valve opening and investigate the cause of the shift in cavitation location, Figure plots the radial cavitation length of region 1 (as shown the black dimension line in Figure (a1)) and the axial cavitation size of region 2 (as shown the black dimension line in Figure (a3)) as a function of valve opening. As depicted in Figure , the cavitation length in region 1 gradually diminishes as the valve opening increases. Moreover, once the valve opening reaches 0.8 mm, cavitation becomes apparent in region 2. This phenomenon can be attributed to the fluid's through-flow area. With an increase in valve opening, the through-flow area expands, resulting in a decrease in flow velocity and a subsequent reduction in pressure drop. Consequently, the cavitation length diminishes. However, when the valve opening reaches 0.8 mm, the through-flow area in region 1 continues to enlarge, surpassing the through-flow area of the fluid passing through the nozzle. This leads to the emergence of cavitation in region 2. Considering the hindering effect of cavitation bubbles on the flow in the vicinity of region 1, when the valve opening is xv, the actual effective circulation opening can be determined by subtracting the axial cavitation length ylength from xv. Referring to the method of calculating the effective circulation area in Figure , the formula for calculating the effective circulation area in region 1 is obtained as shown in Equation (31).
(31)
(31)
Similarly, in the vicinity of region 2, the obstruction of flow by cavitation bubbles is represented by the radial cavitation length xlength. The actual effective circulation diameter can be determined by subtracting xlength from the nozzle diameter. Therefore, the formula for calculating the effective circulation area for region 2 is obtained as shown in Equation (32).
(32)
(32)
Combining Equations (26) and (27), the formula for calculating the final effective area of region 1 and region 2 can be obtained as shown in Equation (33).
(33)
(33)
Figure demonstrates the relationship between the area of region 1 and region 2 and the valve openings calculated using Equation (33), which indicates that the cavitation length of region 1 decreases as valve opening increases, resulting in a quadratic trend for the through-flow area of region 1 at valve openings between 0.5 and 1.3 mm. Once valve opening exceeds 1.3 mm, cavitation disappears completely, causing the through-flow area to increase linearly with valve opening. Conversely, as valve opening increases, the cavitation size of region 2 increases, leading to a decrease in the through-flow area, which stabilises once the cavitation region reaches a fixed size. By comparing the through-flow areas of region 1 and region 2 (i.e. values of S1 and S2), the cavitation location pattern can be inferred. When S1 is greater than S2, cavitation is likely to occur in region 2 due to the relatively small through-flow area of this region.
Figure 21. The cavitation length as a function of valve opening in region 1 and region 2. The cavitation region of the nozzle and the gap (between the valve disc and the valve seat) are represented by region1 and region2, respectively. The variation curves of these two regions with respect to the valve disc opening are depicted in red and black, respectively.

Figure 22. The relationship between the area of region 1 and region 2 and the valve openings. The location of cavitation can be determined by comparing the through-flow areas. The through-flow areas of the gap region and nozzle region, represented by S1 and S2 respectively, are calculated using Equation 33. These areas are depicted by the black and red curves, respectively. Based on the characteristics of these curves, they are categorised into three stages, each corresponding to different positions of cavitation.

5. Conclusion
This study conducts a comprehensive examination of cavitation characteristics in a safety valve located in the secondary circuit of a nuclear power plant. To analyze the phenomenon, a high-fidelity cavitation model is proposed. It integrates the valve characteristic length, velocity strain rate correction mass transfer term, adaptive ensemble of surrogate models, and genetic algorithm. The model is then compared with experimental data obtained from a self-built visualisation test rig, and a remarkable agreement is observed. This research primarily focuses on two key control parameters of the relief valve: and valve opening While investigating the variations in cavitation, the influence of
on cavitation is elucidated through an analysis of vortex dynamics and turbulence intensity. Additionally, a formula is introduced to calculate the effective flow area under cavitation conditions, elucidating the impact of valve opening on cavitation. The principal findings of this study are summarised as follows.
The integration of the valve flow characteristic length and the velocity strain rate-based correction method for the evaporation term in the Zwart-Gerber-Belamri (Z-G-B) model effectively mitigates the influence of variations in bubble diameter (Db) and nucleation site volume fraction (αnuc) on the cavitation length. This approach results in a significant reduction in the maximum error of Db from 12.5% to 8.7% and limits the maximum error of αnuc from 22% to within 10%. As a result, it achieves a substantial reduction in dimensional complexity and ensures physical accuracy.
The visualisation test bench built in this study allows clear observation of the cavitation phenomenon in the valve, and the cavitation length can be accurately measured using the gray scale detection method. Furthermore, the AES-MSI model accurately establishes the relationship between the evaporation/condensation coefficients and the cavitation length. Experimental verification confirms that the above coefficients, when optimised by genetic algorithm, enable accurate prediction of the cavitation length.
Pressure drop and valve opening are two critical parameters that influence the cavitation phenomenon. Concerning the impact of pressure drop on cavitation, this study concludes that the cavitation length gradually increases with pressure until it reaches a steady state. This pressure-dependent cavitation process can be divided into three stages: initial cavitation stage, developing cavitation stage, and stable cavitation stage. During the developing cavitation stage, a significant difference in cavitation length exists between the left and right sides of the valve disc due to the presence of vortices within the fluid. With a further increase in pressure, the transition to the stable cavitation stage occurs, leading to changes in the flow pattern. As a result, the flow resistance on the left side of the valve disc decreases, and the cavitation length becomes consistent. Additionally, turbulence intensity can serve as a reliable indicator to distinguish the three cavitation stages.
Concerning the impact of valve opening on cavitation, this study concludes that the cavitation region around the valve disc decreases with increasing opening, and simultaneously shifts towards the nozzle region. This change can be attributed to the relative alteration in the effective flow area of the aforementioned region. The potential mechanism behind this change in cavitation area can be elucidated by adopting the effective flow area calculation formula proposed in this study.
5.1. Limit of this study
Although this study constructs a cavitation model for a nuclear power second circuit safety valve and explores the cavitation evolution law, there is a limitation in the visualisation test bench. As a result, the pressure set in this study deviates from the actual operating conditions of the valve to some extent. In future studies, using visualisation materials with higher pressure-bearing capacity or adopting more advanced testing methods for cavitation phenomenon will be considered to overcome the current pressure limitation. Furthermore, the valve disc shape studied in this study belongs to the classical cylindrical type, featuring a relatively simple structure. In the subsequent research, a valve disc with a more complex shape will be investigated to further validate the applicability of the improved cavitation model proposed in this study.
Author statement
The research was conceptualised and guided by Xueguan Song and Qingye Li. Shuai Zhang was responsible for constructing the surrogate model, MuChen Wang conducted the visualisation experiments and handled the image processing. Chaoyong Zong and Xuyang Li undertook the CFD modelling, calculations, and data analysis.
Acknowledgments
This work was supported by the National Natural Science Foundation of China (No. 52075068). We would also like to thank the editors and the reviewers for their constructive comments and helpful suggestions.
Disclosure statement
No potential conflict of interest was reported by the author(s).
Additional information
Funding
References
- Amirante, R., Distaso, E., & Tamburrano, P. (2014). Experimental and numerical analysis of cavitation in hydraulic proportional directional valves. Energy Conversion and Management, 87, 208–219. https://doi.org/10.1016/j.enconman.2014.07.031
- Asnaghi, A., Feymark, A., & Bensow, R. E. (2017). Improvement of cavitation mass transfer modeling based on local flow properties. International Journal of Multiphase Flow, 93, 142–157. https://doi.org/10.1016/j.ijmultiphaseflow.2017.04.005
- Bayramov, A. N. (2023). Comprehensive assessment of system efficiency and competitiveness of nuclear power plants in combination with hydrogen complex. International Journal of Hydrogen Energy, 48(70), 27068–27078. https://doi.org/10.1016/j.ijhydene.2023.03.314
- Bjerre, M., Eriksen, J. G. I., Andreasen, A., Stegelmann, C., & Mandø, M. (2017). Analysis of pressure safety valves for fire protection on offshore oil and gas installations. Process Safety and Environmental Protection, 105, 60–68. https://doi.org/10.1016/j.psep.2016.10.008
- Chen, Y., Chen, X., Gong, Z., Li, J., & Lu, C. (2016). Numerical investigation on the dynamic behavior of sheet/cloud cavitation regimes around hydrofoil. Applied Mathematical Modelling, 40(11–12), 5835–5857. https://doi.org/10.1016/j.apm.2016.01.031
- Cheng, H., Long, X., Ji, B., Peng, X., & Farhat, M. (2021). A new Euler-Lagrangian cavitation model for tip-vortex cavitation with the effect of non-condensable gas. International Journal of Multiphase Flow, 134, 103441. https://doi.org/10.1016/j.ijmultiphaseflow.2020.103441
- Elmisaoui, S., Benjelloun, S., Chkifa, M. A., & Latifi, A. M. (2023). Surrogate model based on hierarchical sparse polynomial interpolation for the phosphate ore dissolution. Computers & Chemical Engineering, 173, 108174. https://doi.org/10.1016/j.compchemeng.2023.108174
- Gao, Z., Yue, Y., Wu, J., Li, J., Wu, H., & Jin, Z. (2021). The flow and cavitation characteristics of cage-type control valves. Engineering Applications of Computational Fluid Mechanics, 15(1), 951–963. https://doi.org/10.1080/19942060.2021.1932604
- Geng, L., Chen, J., & Escaler, X. (2020). Improvement of cavitation mass transfer modeling by including Rayleigh–Plesset equation second order term. European Journal of Mechanics – B/Fluids, 84, 313–324. https://doi.org/10.1016/j.euromechflu.2020.05.008
- Habibnejad, D., Akbarzadeh, P., Salavatipour, A., & Gheshmipour, V. (2022). Cavitation reduction in the globe valve using oblique perforated cages: A numerical investigation. Flow Measurement and Instrumentation, 83, 102110. https://doi.org/10.1016/j.flowmeasinst.2021.102110
- He, J., Zhang, Y., Liu, X., Li, B., Sun, S., Peng, J., & Liu, W. (2023). Experiment and simulation study on cavitation flow in pressure relief valve at different hydraulic oil temperatures. Flow Measurement and Instrumentation, 89, 102289. https://doi.org/10.1016/j.flowmeasinst.2022.102289
- Jena, S. K., Bose, S., & Patle, S. D. (2023). Comparison of the performance of propane (R290) and propene (R1270) as alternative refrigerants for cooling during expansion in a helical capillary tube: A CFD-based insight investigation. International Journal of Refrigeration, 146, 300–313. https://doi.org/10.1016/j.ijrefrig.2022.11.009
- Jin, Z., Qiu, C., Jiang, C., Wu, J., & Qian, J. (2020). Effect of valve core shapes on cavitation flow through a sleeve regulating valve. Journal of Zhejiang University-Science A, 21(1), 1–14. https://doi.org/10.1631/jzus.A1900528
- Kumagai, K., Ryu, S., Ota, M., & Maeno, K. (2016). Investigation of poppet valve vibration with cavitation. International Journal of Fluid Power, 17(1), 15–24. https://doi.org/10.1080/14399776.2015.1115648
- Li, Q., Zong, C., Liu, F., Xue, T., Zhang, A., & Song, X. (2023a). Numerical and experimental analysis of the cavitation characteristics of orifice plates under high-pressure conditions based on a modified cavitation model. International Journal of Heat and Mass Transfer, 203, 123782. https://doi.org/10.1016/j.ijheatmasstransfer.2022.123782
- Li, Q., Zong, C., Liu, F., Zhang, A., Xue, T., Yu, X., & Song, X. (2023b). Numerical and experimental analysis of fluid force for nuclear valve. International Journal of Mechanical Sciences, 241, 107939. https://doi.org/10.1016/j.ijmecsci.2022.107939
- Li, S., Aung, N. Z., Zhang, S., Cao, J., & Xue, X. (2013). Experimental and numerical investigation of cavitation phenomenon in flapper–nozzle pilot stage of an electrohydraulic servo-valve. Computers & Fluids, 88, 590–598. https://doi.org/10.1016/j.compfluid.2013.10.016
- Liu, X., Wu, Z., Li, B., Zhao, J., He, J., Li, W., Chi, Z., & Fangwei, X. (2019). Influence of inlet pressure on cavitation characteristics in regulating valve. Engineering Applications of Computational Fluid Mechanics, 14(1), 299–310. https://doi.org/10.1080/19942060.2020.1711811
- Liu, X., He, J., Li, B., Zhang, C., Xu, H., Li, W., & Xie, F., (2021). Study on Unsteady Cavitation Flow and Pressure Pulsation Characteristics in the Regulating Valve. Shock and Vibration, 2021. http://doi.org/10.1155/2021/6620087
- Lu, L., Wang, J., Li, M., & Ryu, S. (2022). Experimental and numerical analysis on vortex cavitation morphological characteristics in u-shape notch spool valve and the vortex cavitation coupled choked flow conditions. International Journal of Heat and Mass Transfer, 189, 122707. https://doi.org/10.1016/j.ijheatmasstransfer.2022.122707
- MacLellan, D. G., Mitchell, A. E., & Turnbull, D. (1960). Flow characteristics of piston-type control valves. Proceedings of the symposium on recent mechanical engineering developments in automatrc control.
- Martin, S., & Bhushan, B. (2014). Fluid flow analysis of a shark-inspired microstructure. Journal of Fluid Mechanics, 756, 5–29. https://doi.org/10.1017/jfm.2014.447
- Mustafa, J., Abdullah, M. M., Ahmad, M. Z., Husain, S., & Sharifpur, M. (2023). Numerical study of two-phase turbulence nanofluid flow in a circular heatsink for cooling LEDs by changing their location and dimensions. Engineering Analysis with Boundary Elements, 149, 248–260. https://doi.org/10.1016/j.enganabound.2023.01.029
- Nozawa, T. (2023). Considering agitation in ultrasonic electroplating through observation of cavitation. Ultrasonics Sonochemistry, 96, 106432. https://doi.org/10.1016/j.ultsonch.2023.106432
- Oshima, S., & Ichikawa, T. (1985). Cavitation phenomena and performance of oil hydraulic poppet valve. Bulletin of JSME, 28(244), 2272–2279. https://doi.org/10.1299/jsme1958.28.2264
- Pope, S. B. (2000). Turbulent flows. Cambridge university press.
- Qian, J., Xu, J., Fang, L., Zhao, L., Wu, J., & Jin, Z. (2023). Effects of throttling windows on cavitation flow of sleeve control valve. Annals of Nuclear Energy, 189, 109841. https://doi.org/10.1016/j.anucene.2023.109841
- Salvador, F. J., Jaramillo, D., Romero, J. V., & Roselló, M. D. (2017). Using a homogeneous equilibrium model for the study of the inner nozzle flow and cavitation pattern in convergent–divergent nozzles of diesel injectors. Journal of Computational and Applied Mathematics, 309, 630–641. https://doi.org/10.1016/j.cam.2016.04.010
- Schnerr, G. H., & Sauer, J. (2001). Physical and numerical modeling of unsteady cavitation dynamics. 4th international conference on multiphase flow, 1(1), 1–12.
- Singhal, A. K., Athavale, M. M., Li, H., & Jiang, Y. (2002). Mathematical basis and validation of the full cavitation model. Journal of Fluids Engineering-Transactions of the Asme, 124(3), 617–624. https://doi.org/10.1115/1.1486223
- Snyder, T. A., Braun, M. J., & Pierson, K. C. (2016). Two-way coupled Reynolds and Rayleigh–Plesset equations for a fully transient, multiphysics cavitation model with pseudo-cavitation. Tribology International, 93, 429–445. https://doi.org/10.1016/j.triboint.2015.08.040
- Soyama, H., & Hoshino, J. (2016). Enhancing the aggressive intensity of hydrodynamic cavitation through a Venturi tube by increasing the pressure in the region where the bubbles collapse. Aip Advances, 6(4), 45113. https://doi.org/10.1063/1.4947572
- Sreedhar, B. K., Albert, S. K., & Pandit, A. B. (2017). Cavitation damage: Theory and measurements – A review. Wear, 372–373, 177–196. https://doi.org/10.1016/j.wear.2016.12.009
- Sun, X., Liu, S., Zhang, X., Tao, Y., Boczkaj, G., Yoon, J. Y., & Xuan, X. (2022). Recent advances in hydrodynamic cavitation-based pretreatments of lignocellulosic biomass for valorization. Bioresource Technology, 345, 126251. https://doi.org/10.1016/j.biortech.2021.126251
- Tahmasebi, E., Lucchini, T., D’Errico, G., Onorati, A., & Hardy, G. (2017). An investigation of the validity of a homogeneous equilibrium model for different diesel injector nozzles and flow conditions. Energy Conversion and Management, 154, 46–55. https://doi.org/10.1016/j.enconman.2017.10.049
- Valdés, J. R., Rodríguez, J. M., Monge, R., Peña, J. C., & Pütz, T. (2014). Numerical simulation and experimental validation of the cavitating flow through a ball check valve. Energy Conversion and Management, 78, 776–786. https://doi.org/10.1016/j.enconman.2013.11.038
- Viitanen, V., Sipilä, T., Sánchez-Caja, A., & Siikonen, T. (2022). CFD predictions of unsteady cavitation for a marine propeller in oblique inflow. Ocean Engineering, 266, 112596. https://doi.org/10.1016/j.oceaneng.2022.112596
- Wang, C., Duan, A., Xu, J., Liu, X., Jin, H., & Ou, G. (2021a). Cavitation failure analysis of 90-degree elbow of mixing point in ethylene glycol recovery and concentration system. Engineering Failure Analysis, 125, 105400. https://doi.org/10.1016/j.engfailanal.2021.105400
- Wang, H., Xu, H., Pooneeth, V., & Gao, X. (2018). A novel one-camera-five-mirror three-dimensional imaging method for reconstructing the cavitation bubble cluster in a water hydraulic valve. Applied Sciences, 8(10), 1783. https://doi.org/10.3390/app8101783
- Wang, Z., Cheng, H., & Ji, B. (2021b). Euler–Lagrange study of cavitating turbulent flow around a hydrofoil. Physics of Fluids, 33(11), 112108. https://doi.org/10.1063/5.0070312
- Wang, Z., Cheng, H., Ji, B., & Peng, X. (2023). Numerical investigation of inner structure and its formation mechanism of cloud cavitating flow. International Journal of Multiphase Flow, 165, 104484. https://doi.org/10.1016/j.ijmultiphaseflow.2023.104484
- Webber, D. M. (2011). Generalising two-phase homogeneous equilibrium pipeline and jet models to the case of carbon dioxide. Journal of Loss Prevention in the Process Industries, 24(4), 356–360. https://doi.org/10.1016/j.jlp.2011.01.010
- Yaghoubi, H., Madani, S. A. H., & Alizadeh, M. (2018). Numerical study on cavitation in a globe control valve withdifferent numbers of anti-cavitation trims. Journal of Zhejiang University-Science A: Applied Physics & Engineering, 25(11), 2677–2687.
- Yi, D., Lu, L., Zou, J., & Fu, X. (2015). Interactions between poppet vibration and cavitation in relief valve. Proceedings of the Institution of Mechanical Engineers. Part C, Journal of Mechanical Engineering Science, 229(8), 1447–1461. https://doi.org/10.1177/0954406214544304
- Yuan, C., Song, J., Zhu, L., & Liu, M. (2019). Numerical investigation on cavitating jet inside a poppet valve with special emphasis on cavitation-vortex interaction. International Journal of Heat and Mass Transfer, 141, 1009–1024. https://doi.org/10.1016/j.ijheatmasstransfer.2019.06.105
- Zhang, S., Pang, Y., Liang, P., & Song, X. (2022). On the ensemble of surrogate models by minimum screening index. Journal of Mechanical Design, 144(7), 71701–71707. https://doi.org/10.1115/1.4054243
- Zong, C., Li, Q., Li, K., Song, X., Chen, D., Li, X., & Wang, X. (2022). Computational fluid dynamics analysis and extended adaptive hybrid functions model-based design optimization of an explosion-proof safety valve. Engineering Applications of Computational Fluid Mechanics, 16(1), 296–315. https://doi.org/10.1080/19942060.2021.2010602
- Zwart, P. J., Gerber, A. G., & Belamri, T. (2004). A two-phase flow model for predicting cavitation dynamics. Proceeding of the 5th International Conference on Multiphase Flow, 152(1), 1–11.