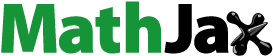
Abstract
To forecast the wake flow and power reduction affecting downstream turbines reliably and accurately, a hybrid wake model CFD(ALM)-IDWM was improved, in which the forecasting of Vθmax is improved from linear to cubic. Then, a partially overlapping computational domain is added behind the far-wake domain of upstream wind turbine to calculate the aerodynamic performance of a downstream wind turbine, and the numerical model of full CFD(ALM) and CFD(ALM)-IDWM for two in-line wind turbines are developed. Subsequently, a wind tunnel model test on two in-line wind turbines was carried out to validate the full CFD(ALM) model firstly. Subsequently, the hybrid model of CFD(ALM)-IDWM is numerically validated by the full CFD(ALM) simulations. The results show that CFD(ALM)-IDWM cannot only predict the wake characteristics such as vortices and wakes in the wake region more accurately, but can also accurately simulate the average values of the downstream turbine thrust and torque. By ignoring certain flow field details including acceleration, turbulent viscosity, and Reynolds stress during the simulation process, the computational time is reduced. Base on the same CFD(ALM), the computation time of CFD(ALM)-IDWM was approximately 60% of that of full CFD(ALM). The longer the computational domain of IDWM, the greater reduction in computational time.
1. Introduction
Wind energy plays a significant role in the current daily energy consumption and is expected to have a growing share in the future. Wind turbines are often employed in wind farms to optimise efficiency per unit of energy production. However, the wake generated by wind turbines can travel tens of kilometres (>20D); this is known as ‘wind theft.’ Moreover, it not only affects the power production but also the fatigue life of neighbouring wind turbines, thereby creating legal conflicts between wind farm operators and nations. Therefore, a fast and accurate simulation of the turbine far-wake is crucial in wind engineering. Wake models are required to accurately reproduce the downstream turbine wake, which can aid in assessing the performance of downwind turbines, such as in the design, operation, and maintenance of wind farms.
According to the simulated wake scale and calculation accuracy, wind turbine wake models can be classified into three categories: Analytical, simplified computational fluid dynamics (CFD)-based, and full CFD-based modelling.
Analytical modelling (Bastankhah & Porté-Agel, Citation2014; Frandsen et al., Citation2006; Jensen, Citation1983; Larsen, Citation1988) may have a lower simulation accuracy than complex numerical simulation tools for turbulence analysis; however, its programme implementation is relatively easy and has a low computational cost (10−3 CPU hours per simulation). Thus, analytical modelling is preferred for wind farm layout optimisation and control in flat terrain (e.g. grasslands and oceans). Meanwhile, Weather Research and Forecasting (WRF) models were used by Pryor et al. (Citation2020) for a broad range of atmospheric conditions and different climatic seasons. Analytical modelling is commonly used for the swift forecasting of wind-farm power production and is frequently employed in engineering design. However, its resolution is extremely low and it is rarely used to simulate two in-line wind turbines.
Simplified CFD-based modelling includes a two-dimensional Reynolds-averaged Navier-Stokes (RANS) equation and the dynamic wake meandering (DWM) model developed by Ainslie (Citation1988). Yoo and Oh (Citation2021) employed the open-source CFD code OpenFOAM to solve the unsteady two-dimensional RANS equation for flow simulation. In addition, the Spalart-Allmaras turbulence model was incorporated to enhance turbulence modelling as part of their methodology. The DWM model simplifies the time derivative term in the NS equation by assuming that the problem and wake are both stationary and symmetrical. By disregarding the tangential velocity and pressure gradient, the model obtained a set of control equations for solving the axial and radial velocity components of the wake. This model provides a method to quickly predict the wind farm power output and load. Larsen et al. (Citation2007) validated the DWM model by comparing model predictions with extensive full-scale measurements. Ennis et al. (Citation2015) made consistent comparisons of the DWM model with the high-fidelity vortex method (VM) and the actuator-line large vortex simulation (AL-LES). This model was extended by Jonkman and Shaler (Citation2021) to model wake advection (evolution), deflection and meandering in a quasi-steady manner.
In contrast, full CFD-based modelling aims to solve the NS equations in the time and space domains with turbulence models using large eddy simulations (LES) or RANS. Although LES models can generate more detailed wake structures and wake evolution procedures, their realistic simulation domains are currently relatively small because of their high computational costs (Berg et al., Citation2017; Vanderwende et al., Citation2016; Yu et al., Citation2023). For example, Churchfield et al. (Citation2012) utilised a grid of 1–2 m around a turbine, which required approximately 6 × 1010 grids to calculate10 km × 6 km × 1 km domains. In addition, a recent review by Porté-Agel et al. (Citation2020) indicates that the computational cost involved in LES of wind farm flow is typically ‘103–104 CPU hours per simulation.’ Hence, Yu et al. (Citation2023) used the actuator line model (ALM) to simplify the wind turbine blades and utilised the RANS model rather than LES to improve the computational efficiency and obtain better results for fixed and floating wind turbine simulations. Wang et al. (Citation2020) used the RANS turbulence model in Star CCM + to simulate the aerodynamic performance of a fixed wind turbine at different yaw angles, which has a significant engineering application value. However, owing to computational efficiency limitations, the number of numerical examples investigated was limited. In summary, the computational efficiency of a full model based on CFD is insufficient for engineering design (Porté-Agel et al., Citation2020).
Analytical modelling is computationally more efficient but ignores crucial physical information such as the wake vortex structure. Although simplified CFD models consider more physical information, including the radial velocity and wake evolution behaviour (Jonkman & Shaler, Citation2021), their computational efficiency depends on empirical parameters and does not produce spirals in the wake because the tangential velocity is neglected. High-resolution CFD models can capture all physical phenomena accurately but are limited by their computational efficiency. The hybrid wake method inherits the advantages of the two model types, overcomes the disadvantages of each model, and is realistic and effective.
Yuan et al. (Citation2023) proposed a new wind turbine wake hybrid method, CFD(ALM)-IDWM, to study the different tip speed ratios of a single fixed turbine, which achieved enhanced computational efficacy while still achieving similar results. According to Feng et al. (Citation2022), the flow in the near-wake region is influenced by the specific details of the turbine design. Specifically, the flow in the near-wake is characterised by the presence of tip, root, and hub vortices. Conversely, the flow in the far-wake region is influenced by various operating conditions including the thrust and power coefficients. These operating conditions include the incoming wind speed and the ambient turbulence intensity.
In this study, the CFD (ALM)-IDWM is further extended. The near wake is simulated using a complete CFD model based on the NS equation, and the wind turbine is represented by an actuator line model (ALM). The far wake is modelled using a new improved dynamic wake model, which is still called the IDWM. The wake predicted by the IDWM serves as the inlet boundary of the downstream viscous domain and was simulated via CFD, coupling the two models and two wind turbines. To avoid duplicate naming, this method was unified as CFD(ALM)-IDWM. To validate the newly developed numerical model, a model test on two in-line turbines was performed in a wind tunnel based on the method of Hao et al. (Citation2023), and the aerodynamic performance and wake properties of the model-scale turbines were studied.
2. Numerical models
This study focuses on the CFD(ALM)-IDWM method, which was used to calculate the affect of upstream wind turbine to the aerodynamic loads of downstream wind turbines. The simulation employs the CFD(ALM) model to simulate the aerodynamic loads of the turbines. The CFD(ALM) model will simulate the near wake of the upstream wind turbine, while the IDWM model will predict the far wake of the same, in which the forecasting of maximum tangential velocity Vθmax was improved from linear to cubic.
To prevent the potential bias introduced by other advanced CFD(ALM) methods on readers’ evaluation of the computational efficiency improvement achieved by CFD(ALM)-IDWM in this study, the hybrid model CFD(ALM)-IDWM is developed based on the conventional ALM method proposed by Sørensen and Shen (Citation2002). The efficiency of computations is evaluated in comparison to the full CFD(ALM).
2.1. CFD(ALM) model for upstream wind turbine
In CFD(ALM) model, as shown in Figure (a), the wake simulation in computational domain ΩCFD(ALM) uses interDyMFoam in OpenFOAM based on the combined PISO-SIMPLE (PIMPLE) algorithm, combined with the traditional k-ε turbulence model to become a high-fidelity wake solver. Specific details can be found in the OpenFOAM documentation. The governing equation used by interDyMFoam was the RANS equation
(1a)
(1a)
(1b)
(1b) where, p is the pressure; τij and τtij are the viscous and turbulent stresses, respectively; g is the acceleration of gravity; fσi is the surface tension; ρ is the fluid density; and fUALM is a source term for modelling blade effects on fluids (Yu et al., Citation2023).
Figure 1. Comparison between CFD(ALM) and CFD(ALM)-IDWM (CFD(ALM) model is used in the solid-line area while the IDWM is used in the dashed-line area; the lower boundary represents the water surface; the upper boundary is the artificial cut-off boundary). (a) CFD(ALM) model (b) CFD(ALM)-IDWM model.

The aerodynamic forces on the turbine blades are estimated by using a well-known blade element method. The effect of the turbine blades on the flow dynamics is modelled by distributing the aerodynamics forces as a body force (ρfUALM) into the flow domain. The NS model combined with ALM is named as CFD(ALM) hereafter.
2.2. CFD(ALM)-IDWM model for upstream wind turbine
In their recent study, Jonkman and Shaler (Citation2021) developed an extension of the DWM that enables the simulation of wake advection, deflection, and meandering in a quasi-steady manner. In this study, an improved dynamic wake model (IDWM) is presented to predict the far wake of upstream wind turbine, as shown in Figure (b). The governing equations utilised in the IDWM are as follows:
(2)
(2)
(3)
(3) where, vt is the empirical kinematic eddy viscosity, which varies spatially to approximate the turbulent effects in the wake flow. In the IDWM, it can be represented as
(4)
(4) Where IAmb is the turbulence intensity at the hub centre; MIN|r{Vx(x, r)} denotes the minimum value of Vx along the radius at a given downstream section; kvAmb and kvShr are coefficients, specified as 0.05 and 0.016, respectively. In the equations above, RWake is the wake half-width, estimated as
(5)
(5) where, Vwind is the incoming wind speed. For convenience, the axial velocity (Vx) in the wake can be expressed as
(6)
(6) Coefficient kvShr was evaluated using the CFD(ALM) results. To achieve this, Equation (2) is integrated with respect to the radial coordinate, denoted as x = xc1, at the entrance of the IDWM, yielding:
Then, kvShr can be expressed by
(7)
(7) At x = xc1 (IDWM inlet), the parameters involved in obtaining the equations are easily obtained from the solution in the CFD(ALM). The coefficients kvShr determined in this manner way correspond to the simultaneous turbine conditions and vary with time.
The proposed that a model for the tangential velocity downstream of a wind turbine can be described as
(8)
(8) where r2 = y2 + z2 is in the coordinate system used in this study and the scaling constant λ is assumed to be 1.26 following Devenport et al. (Citation1996). The first part of Devenport et al. (Citation1996) is almost identical to that in Equation (8) when λ = α ≈ 1.26 but with (1 + 0.5/α) replaced by 1/[1−exp(−λ)].
To use this equation, the values of Vθmax and rc must be determined. In the CFD(ALM)-IDWM used by Yuan et al. (Citation2023), a linear fitting method was used to calculate the maximum tangential velocity. In contrast, we found that Vθmax(x) for x is a nonlinear change process through the verification of additional wind turbine examples. We compared the linear, quadratic, and cubic results and found that they had a certain impact on the calculation error of the aerodynamic performance of the downstream turbines. Equations (9)–(11) are provided for the reader.
(9)
(9)
(10)
(10)
(11)
(11) where a, b, c and d are four coefficients. Our results show that the coefficients can be determined using the data immediately before the DWM inlet. They were estimated using the maximum tangential velocity from CFD(ALM) at several sections between the IDWM inlet and the section (x = xd) denoted by the dashed-dotted line in Figure . rc in Equation (12) is evaluated as follows
(12)
(12) where rc1 is the radial coordinate of Vθmax at the IDWM-inlet (xc1), and xc is the axial coordinate of the section where rc is evaluated. The value of β in Equation (11) should be 0 < β < 1. This can be determined in different ways, but is proposed to be estimated by
(13)
(13) where rc0 /R is the ratio of the radial coordinate of Vθmax at the turbine section to the radius of the turbine. Based on the numerical tests, Equation (12) indicates that rc1 ≤ rc < Rwake. The values of a, b, c, d, β, rc1 and Rwake(xc1) in Equations (9)–(13) were simultaneously estimated using the results obtained by CFD(ALM). These values correspond to the flow conditions of the turbines, which change over time.
2.3. Full CFD(ALM) and CFD(ALM)-IDWM models for two in-line wind turbines
For two wind turbines, a CFD(ALM) model of the downstream wind turbine will be incorporated into the numerical model of the upstream wind turbine to assess the aerodynamic performance of the downstream wind turbine. In fact, the original CFD(ALM)-IDWM model for the upstream turbine supplies the velocity distribution to the CFD(ALM) model for the downstream wind turbine. The numerical model of two wind turbines is not limited to the arrangement of wind turbines. When two wind turbines are positioned in-line, the downstream turbine is significantly influenced, as shown in Appendix A. For ease of discussions, a case of two in-line turbines will be considered, as shown in Figure .
When the upstream wind turbine is simulated using the CFD(ALM) model, the downstream turbine model can be directly added, forming a full CFD(ALM) model as shown in Figure (a). If the the upstream wind turbine is simulated using the CFD(ALM)-IDWM model, a partially overlapping computational domain ΩCFD(ALM)-T2 will be added to the behind of far wake domain ΩIDWM, also forming the CFD(ALM)-IDWM model as shown in Figure (b). As shown in the figure, the CFD(ALM) model is used in the solid-line area, whereas the IDWM model is used in the dashed-line area, the lower and upper boundaries represent the water surface and artificial cut-off boundary, respectively.
In CFD(ALM)-IDWM model, the downstream wake simulation is achieved by directly interpolating the velocity distribution at the same position ΩIDWM to the inlet boundary of ΩCFD(ALM)-T2. In contrast to Yuan et al. (Citation2023), the coupling between ΩIDWM-ΩCFD(ALM)-T2 does not require a relaxation zone. The impact of the distance between the inlet boundary and downstream turbine is discussed in the following text. The reference coordinate system used in this study is consistent with that described by Yuan et al. (Citation2023). The CFD(ALM)-IDWM hybrid method for two in-line wind turbines is shown in the flowchart in Figure . In the same time-step calculation, CFD(ALM)-T1 and CFD(ALM)-T2 are the NS solvers. CFD(ALM)-T2 can only be calculated after the iteration calculations of CFD(ALM)-T1 and IDWM are completed, and the CFD(ALM)-T2 inlet boundary is updated.
To verify the numerical model presented in this study, a model test on two in-line wind turbines was conducted to estimate the aerodynamic performance and wake characteristics of the model-scale wind turbines. Because the CFD(ALM) model serves as the foundation for CFD(ALM)-IDWM, the two in-line wind turbines simulated by full CFD(ALM) model are verified firstly. Furthermore, the performance of CFD(ALM)-IDWM will be investigated by comparing it with the performance of the full CFD(ALM) model.
3. Experimental validation of full CFD(ALM) model
The model test was conducted in a boundary-layer wind tunnel with dimensions of 18 m (length) × 4 m (width) × 3 m (height).
3.1. Experimental model and setup
As previously mentioned, the flow in the far-wake region is influenced by various operating conditions including the thrust and power coefficients. To simulate the upstream turbine wake flow and its effect on the downstream turbine's aerodynamic performance (particularly the power), achieving the thrust and torque similarities through the entire range of wind speeds is crucial, particularly after the rated wind speed, to maintain performance. To ensure that a model-scale wind turbine achieves the desired scale thrust and torque simultaneously throughout the range of wind speeds, Hao et al. (Citation2023) proposed a novel wind turbine model-test method for achieving similarity in both model- and full-scale thrusts and torques. The results of the wind tunnel model test demonstrated that the desired scale thrust and torque could be achieved simultaneously at the test wind speed for a single model-scale wind turbine.
The model-scale wind turbine tested in this study corresponds to the DTU 10MW offshore wind turbine, but it is not geometrically similar. Instead, it was redesigned using the low Reynolds airfoil SD7032. This presents a novel model-test method for floating offshore wind turbines. In order to achieve the similar aerodynamic thrust and torque, the cross section of blade needs be changed from high Reynolds number airfoils to the low Reynolds number airfoils. As a result, the blade will not geometrically similar precise. The rotor diameter was scaled down to a ratio of 1:100. The lift-drag coefficient curve of the SD7032 airfoil was used for this model at a Reynolds number of 12.5 × 104, as presented in Figure . Distribution of the geometrical properties of model scale blade are shown in Table .
Table 1. Distribution of the geometrical properties of model scale blade.
Figure (a and b) illustrates the arrangement of the model test in the wind tunnel, and Figure (c) shows the installed model. The model-scale wind turbines are installed on the front and back turntables of a wind tunnel, and the yawing of the turbine can be performed by turning the turntables. The turbines were spaced 10 m (5.56D) apart. The front turbine was positioned 3.0 m (1.67D) from the wind tunnel inlet, with the centre of its hub positioned 1.15 m above the wind tunnel floor. The height of the second turbine hub was 1.08 m. Three wake measurement profiles were designed between the two turbines, each parallel to the turbine rotation plane. These profiles were located at distances of 3.6 m (2D), 5.4 m (3D), and 7.2 m (4D) from the front turbine's rotation plane.
Figure 5. Experimental setup. (a) Arrangement of the model test (b) Arrangement of the wind velocity measurement (c) Installed in wind tunnel.

In the experiment, the rotor was driven at a set rotational speed by using a motor behind the hub. Thrust was measured using a 6-component balance under the bottom of the tower. The tower was installed on a 6-component balance. The power of the wind turbine was measured indirectly using the aerodynamic torque. The dynamic torque was measured using a contactless torque sensor installed coaxially between the hub and the motor. In the constant-temperature mode (CTA), the average velocity in the wake behind the upstream turbine T1 was measured through a pitot tube. The gauge point of the pitot tube was installed at the same height as the hub of T1. Each measurement point was sampled for 30 s at 3.33 kHz, resulting in a total of 10,000 samples at a time. The signals were amplified and filtered to avoid noise distortion. Before each measurement, 20,000 samples of the inflow wind time series were taken at the entrance of the wind tunnel within a 60-second change period. Reference speed Uref was then obtained and displayed on the monitor in real time. The test turbines did not rotate until the inflow wind speed became relatively stable, and their stable thrust and torque were measured. Simultaneously, the pressure difference around the two defined cross-sections for each measuring point was recorded. The air density (ρ) in the experiment was calculated according to the air temperature and atmospheric pressure measured in the test section of each measuring point.
The model test in this study primarily evaluates the rated wind speed, as well as the working conditions beyond it, using real wind speeds of 11.4 m/s and 16.0 m/s respectively. The model test used a wind speed scaling ratio of 2, with different yaw angles employed for the front turbine to establish six sets of testing conditions. The experimental conditions are listed in Table . The rotor speed and blade pitch angle of the model-scale turbines were determined using the model test method proposed by Hao et al. (Citation2023). This approach ensured that both the scaled thrust and torque were obtained at the set test wind speed, which was consistent with the requirements of the model test.
Table 2. Cases of the wind tunnel model test on two in-line wind turbines.
3.2. Convergent tests
In this section, we first validated the CFD(ALM) model against experimental data and ensured that it converged with respect to the chosen grid. And, Case 6 was chosen as a representative example for the grid convergence analysis.
The computational domain size followed the same specifications as those in the wind tunnel model test presented in Figure . T1 was located at the origin, T2 was placed at 5.56D, and the outlet boundary was set to 7.8D. The k-ε turbulence model was employed with the inlet boundary conditions derived from the turbulence intensity TI = 0.1% of k = 0.464 and ε = 2.84. According to Shen et al. (Citation2005), the tip loss is an important phenomenon in wind turbine aerodynamics, and it must be modelled separately using the CFD(ALM) method to predict the aerodynamic performance of the wind turbine; it exhibits good robustness in situations involving high angles of attack and axial interference factors. This makes the model attractive for tip loss correction in CFD(ALM). Therefore, the Shen end-effect model is more appropriate for blade-tip loss correction in this study. where c1 and c2 are 0.125 and 21, respectively. Moreover, a zero velocity and pressure gradient was imposed on the outlet boundary, whereas no-slip boundaries were used at the top, sidewalls, and bottom of the simulated wind tunnel environment. In addition, we generated meshes that followed the specifications presented by Yuan et al. (Citation2023). Table lists three different meshes: coarse, medium, and fine. The symbol R/no.mesh represents the number of grids distributed in each turbine radius. For instance, G2 has a grid size near ALM consisting of approximately 72 points distributed in each turbine radius. The selected time step Δt corresponds to the angular displacement of the turbine (1°), resulting in an average Courant number of < 1. To ensure relatively stable turbine aerodynamics, we calculated 35 rotation periods (5 s) for all cases.
Table 3. Mesh generation scheme.
Figure illustrates the thrust and torque of T1 for all the grids. The results show that the mean values of the coefficients and oscillation amplitude were almost identical for the medium (G2) and fine (G3) grids, whereas significant differences were observed for the coarse grid. The relative differences in the mean thrust and torque values for G2 and G3 were negligible. However, the corresponding differences for G1 were 0.4% and 0.1%. Figure shows the dimensionless mean axial velocity distributions for the 2D, 3D, and 4D models. The results show that the medium grid (G2) and fine grid (G3) were indistinguishable in terms of their velocity distributions, whereas the fine grid (G1) consistently yielded higher velocity values at the wake disk centre.
3.3. Validation of aerodynamic loads
As an application, the wind tunnel model test cases in Table were simulated using full CFD(ALM). The thrust and torque of the two in-line wind turbines were evaluated, and the results are presented in Table . The full CFD(ALM) error remained within 10%, providing results that were consistent with the experimental results. For example, when the inlet wind speed was 5.56 m/s, an increase of 5° in the yaw angle of T1 decreased the thrust and torque of the upstream turbine by 1.2% and 2.8%, respectively. Simultaneously, the thrust and torque of the downstream turbine increased by 10.6% and 2.3%, respectively. Consequently, the total torque increased by 1.16%. As the yaw angle of T1 approached 10°, the thrust and torque of the upstream turbine decreased by 2.3% and 3.9%, respectively. However, the thrust and torque of the downstream turbine increased by 7.6% and 31.6%, respectively, resulting in a 6.54% increase in the total torque.
Table 4. Aerodynamic loads of upstream and downstream turbines.
However, when the inlet wind speed was 8 m/s, an increase of 5° in the yaw angle of T1 reduced the thrust and torque of the upstream turbine by 2.2% and 0.9%, respectively. Simultaneously, the thrust and torque of the downstream turbine increased by 0.4% and 1.2%, respectively. When the yaw angle of T1 was changed to 10°, the thrust and torque of the upstream turbine decreased by 3.1% and 1.9%, respectively. The thrust and torque of the downstream turbine increased by 0.6% and 2.6%, respectively. Because the experimental results were limited, the significance of the 8 m/s yaw increase on the turbine torque could not be confirmed. However, numerical simulations could aid in completing the results when the yaw angle was greater than 10°.
3.4. Validation of the wake (deficit axial velocity)
Figures and compare the wake results obtained from the full CFD(ALM) simulations and experimental measurements, where the error bar range is 20% of the test value. To avoid the contingency of the test, two wind tunnel tests were conducted, as shown in Figure (a), and the results of the two groups were consistent. When the wake had a bimodal Gaussian distribution shape, the velocity gradient near the bimodal distribution was large, and the velocity changed significantly, making it difficult to measure the location of the peak; the smaller the velocity, the more difficult it is to measure. As the yaw angle increased, the centre point of the axial velocity distribution shifted towards the negative y-axis. The numerical simulation accurately reproduced this shift as observed in the experiments. The numerical results obtained using full CFD(ALM) were in good agreement with the experimental measurements.
Figure 8. Deficit velocity profiles (||/
) averaged over 6–7 s along the horizontal line of −2R < y < 2R and z = 1.15 m for
= 5.56 m/s, (circles: Experimental measurements (Error bar 20%); dashed line: CFD(ALM); Black inverted triangle: Repeated experimental measurements). (a) γ = 0° (b) γ = 5° (c) γ = 10°.

Figure 9. Deficit velocity profiles (||/
) averaged over 6–7 s along the horizontal line of −2R < y < 2R and z = 1.15 m for
= 8.0 m/s, (circles: Experimental value (Error bar 20%); dashed line: CFD(ALM)). (a) γ = 0° (b) γ = 5° (c) γ = 10°.

There are two main reasons for the insufficient prediction of full CFD(ALM). First, the deficit velocity is a no-zero value beyond the range of the wake, and there is a blocking effect in wind tunnel tests. Second, the aerodynamic parameters applicable to turbines under undisturbed inflow conditions are used for downstream turbines operating in the wake of upstream turbines.
4. Numerical validation of CFD(ALM)-IDWM model
Comparisons with the experimental results presented in the previous section proved that the full CFD(ALM) method used in this study is accurate. Because CFD(ALM)-IDWM has not yet considered as the function of wall reflection, new examples must be established based on the full CFD(ALM) to verify CFD(ALM)-IDWM. Simultaneously, based on the CFD(ALM)-based method, a set of cases using coarse grids in the wake region were added (hereafter referred to as CFD(ALM) – coarse grids).
To validate the hybrid method of CFD(ALM)-IDWM, two in-line wind turbines were simulated, and their results were compared with those obtained by full CFD(ALM). As shown in Table , turbine T2 is located 7–10D behind turbine T1 and the sidewall width extends from 2.2D to 8D. The computational parameters for CFD(ALM)-IDWM are as follows: The inlet and outlet boundaries of ΩNS1 were set at −2.2D and 3D, respectively; Turbine T1 was located at the coordinate origin. The inlet boundary of the IDWM was set at 2.5D, the relaxation zone was set from 2.5D to 3D, and the inlet and outlet boundaries of ΩNS2 were set at 5D to 9.8D in Cases 7 and 8 and 8D to 12.8D in Cases 13 and 14,respectively, and the other working conditions were similar. The computational mesh parameters used were dx = 0.1, dy = 0.03, NX = 100, and NR = 52 in ΩIDWM for 5,200 grids, which was sufficient based on our numerical testing. With the backward movement of T2, the physical time required for convergence of the numerical calculation was longer.
Table 5. Specifications of tests.
4.1. Vθmax interpolation scheme
The tangential velocity variation in the wake is another feature that must be examined. Take Cases 1 and 4 for example, Figure shows the average tangential velocity changes at various axial positions between 6 and 7 s for rotations along the horizontal line (−2R < y < 2R, z = 1.15 m). At each position, the distribution features a maximum tangential velocity denoted as Vθmax, which change linearly according to Yuan et al. (Citation2023). However, as more examples and experiments were conducted, it was observed that Vθmax tended to be nonlinear. An important improvement in the interpolation scheme for Vθmax in this paper is extending it from linear to cubic. And Equations (9)–(11) are proposed based on the reader's choice.
Figure 10. Profiles of the tangential velocity (Vz) averaged over 6–7 s along the horizontal line of −2R < y < 2R and z = 1.15 m. (a) = 5.56 m/s, γ = 0° (b)
= 8.0 m/s, γ = 0°.

Figure shows the variation of VZmax in Cases 1 and 4, which are predicted by different interpolation scheme. Compared to the results obtained by full CFD(ALM), the cubic is significantly better than linear and quadratic.
Figure 11. Variation in the maximum of the averaged tangential velocity over 6–7 s on the line of γ = 0° along the axial direction. (a) = 5.56 m/s, γ = 0° (b)
= 8.0 m/s, γ = 0°.

In this section, the impact on the calculation accuracy for different forecasting formats of Vθmax for Cases 7 and 8 is discussed while disregarding their effect on the upstream wind turbine. To illustrate this, Figure shows the thrust and torque of the downstream wind turbine, calculated by employing various forecasting methods of calculating Vθmax. The higher the format order, the closer is the estimated average value to the CFD(ALM). The linear format was ineffective in predicting an accuracy of 11.1% for the torque calculation, as shown in Figure (a). Hence, we can infer that the linear forecasting format is not ideal for predicting the results of Case 7. This inference is consistent with the conclusions presented in Figure .
4.2. Performance of the CFD(ALM)-IDWM
According to the results presented in Section 4.1, the cubic equation is used for the calculation in the CFD(ALM)-IDWM. In this section, the detailed results for Cases 7 and 8 are compared using the four methods: full CFD(ALM), CFD(ALM)- IDWM CFD(ALM)-DWM, and full CFD(ALM)-coarse grids. The CFD(ALM)-DWM uses a default value of kvShr = 0.001 and does not consider tangential velocity correction, with all other settings being consistent with the CFD(ALM)-IDWM. The time history of the thrust and torque of the corresponding wind turbine, as shown in Figures and , respectively, exhibited a maximum error of 1.7% for turbine T1. However, the error of the CFD(ALM)-DWM in predicting the torque of turbine T2 in Case 7 significantly exceeds the acceptable range. In Case 8, the thrust and torque results of T2 by the full CFD(ALM) – coarse grids were both unacceptable, indicating that coarse grids could not effectively simulate the evolution process of the upstream wind turbine wake. Hence, the improvement in the CFD(ALM)-DWM makes the hybrid model more universal.
Figures and show the time histories of the torque and thrust of T2 for Cases 13 and 14; the mean values of the results are relatively consistent, but there is no uniform pattern for the amplitude. This is because the prediction of the tangential velocity relies on interpolation prediction, and as the distance increases, the interpolation results inevitably deviate from the full CFD(ALM) results. The inconsistency of the velocity components directly affects the angle of attack of the inflow, thereby affecting the amplitude of the aerodynamic performance.
Figures and show the average thrust torque values of T2 at different distances and wind speeds. Results revealed that the CFD(ALM)-IDWM and full CFD(ALM) methods were consistent, with errors within 5%. Moreover, even if the downstream turbine was arranged at 10D without yaw, its thrust and torque were severely affected by the wake. Compared with the upstream turbine that is not affected by the wake, the wind speed is 5.56 m/s, the thrust decreased by 36.5% and the torque decreased by 75.6%; when the wind speed was 8 m/s, the thrust decreased by 26.4% and the torque decreased by 39.3%.
Figure 18. At = 5.56 m/s, T2 is arranged at different distances to obtain the average thrust torque value (Error bar 5%).

Figure 19. At = 8.0 m/s, T2 is arranged at different distances to obtain the average thrust torque value (Error bar 5%).

Figure compares the average axial wake distributions predicted using the different methods. For Cases 7 and 8, x/d = 5 is the inlet boundary, and x/d = 6 is the wake evolution process from the inlet boundary to turbine T2 at x/d = 7. The thrust and torque were calculated using the full CFD(ALM) method based on the flow field near x/d = 7. In contrast, the wake contour lines simulated by the CFD(ALM)-DWM were significantly different from those simulated by the other methods because the average axial wake distribution dominated in the wake, which provided the wind load for turbine T2. The wake profile with the CFD(ALM)-IDWM method coincides with the full CFD(ALM) in the velocity distribution of the x/d = 7 profile, accurately calculating the average value of the aerodynamic performance of turbine T2. Cases 13 and 14 are similar.
Figure 20. Deficit velocity profiles (||/
) averaged over 6–7 s along the horizontal line of −2R < y < 2R and z = 1.15 m (Solid line: Experimental value; circles: CFD(ALM)-IDWM; dashed line: CFD(ALM)-DWM; x- dashed line: CFD (ALM) – coarse grids). (a) Case 7 (b) Case 8 (c) Case 13 (d) Case 14.

Figure illustrates the average tangential wake distributions predicted using different methods. In full CFD(ALM), thrust and torque calculations rely on the velocity vector of the flow field. The results show that the magnitude of the tangential velocity cannot be ignored because it directly affects the calculation of the attack angle. The analysis reveals that the axial wake velocity primarily influences the average value of the aerodynamic performance turbine T2, whereas the tangential velocity affects the amplitude.
Figure 21. Tangential velocity profiles (normalised by ) averaged over 6–7 s along the horizontal line of −2R < y < 2R and z = 1.15 m (Solid line: Experimental value; circles: CFD(ALM)-IDWM; dashed line: CFD(ALM)-DWM; x- dashed line: CFD (ALM) – coarse grids). (a) Case 7 (b) Case 8 (c) Case 13 (d) Case 14.

When simulating wall turbulence, such as turbulent channel flow, researchers and engineers are interested in the distribution of the wall-normal component of the Reynolds stress (Lee & Moser, Citation2015). In statistically steady turbulence, the Reynolds stress field is obtained by calculating the prime-squared mean of the velocity field (UPrime2Mean in OpenFOAM). As the inlet boundary ensures only the input of the velocity field, the Reynolds stress field belongs to a second-order small quantity of velocity. CFD(ALM)-IDWM and CFD(ALM)-DWM results displayed in Figure represent a recalculation of the Reynolds stress field in the viscous domain based on the velocity boundary conditions. Although CFD(ALM)-IDWM has some amplitude errors, compared to full CFD(ALM), its trend is consistent. In future research, we will consider further modifications to the IDWM model to compensate for this error.
Figure 22. Reynolds stress field profiles (UPrime2Mean magnitude) along the horizontal line of −2R < y < 2R and z = 1.15 m (Solid line: Experimental value; circles: CFD(ALM)-IDWM; dashed line: CFD(ALM)-DWM; x- dashed line: CFD (ALM) – coarse grids). (a) Case 7 (b) Case 8.

Figures and show that CFD(ALM)-IDWM generates an excellent far-wake region, whereas CFD(ALM)-DWM fails to capture the main wake characteristics, despite having dynamic wake models.
5. Behaviours of CFD(ALM)-IDWM model
5.1. Effects of the inlet boundary locations (L) of ΩCFD(ALM)-T2
The above analysis demonstrates the reliability and superiority of CFD(ALM)-IDWM. To further elucidate the speed of the method, distance L (Figure ) from the downstream inlet boundary to turbine T2 was examined. The time histories of the thrust and torque for various values of L are presented in Figure , where xD denotes distance L from the inlet boundary to turbine T2. The predicted aerodynamic performance exhibited slight changes in both the average and amplitude, owing to the unavoidable differences between the changes in the wake simulated by IDWM along the wind speed direction and the wake calculated through full CFD(ALM). Nonetheless, the level of variation still fell within the acceptable range, and the error in all examples was less than 5%. CFD(ALM)-IDWM cannot be applied when the distance is less than 0.5D. Thus, the shortest distance L should be set to 0.5D.
Figure 25. Time histories of the thrust and torque of T2 under different inlet boundary locations. (a) Case 7 (b) Case 8.

The velocity-vector magnitude map based on Case 8 with x/D = 7 is presented in Figure , in which the outcomes for L at 0.5D and 2D exhibit substantial similarity. Hence, using L at 0.5D can significantly reduce the computation time for the aerodynamic performance of turbine T2.
5.2. Analysis of wind turbine wake characteristics
In this section, the first-generation eddy current identification method ‘vorticity’ and the second-generation eddy current identification method ‘Q criterion’ are used to study the aerodynamics of wake propagation and interaction with turbine, to determine the source of power and thrust changes discussed in Section 4.1–4.3.
Figure (a) shows the x-vorticity, which represents the direction of the incident wind. According to the Biot–Savart law, eddy currents in the x-direction are directly caused by the evolution of the axial velocity deficit distribution, . The formation mechanism of a counter-rotating vortex pair (CVP) is as follows (Wang et al., Citation2023): the pressure gradient in the normal direction of the blade changes significantly, and the fluid is compressed and detached from the blade tip when passing through the blade surface, forming a blade tip vortex. The periodic rotation of the blade tip resulted in the shedding of the blade tip vortex cycle, and the velocity cycle carried by the blade tip vortex in the flow direction was equal to the vortex flux of the CVP.
Figure 27. x and y vorticity for Case 8 simulated by different methods. (a) x vorticity (b) y vorticity. (A): full CFD(ALM), (B): CFD(ALM)-IDWM, (C): CFD(ALM)-DWM).

The vortex system consisted of blade root and blade tip vortexes. The root vortex formed a cylindrical positive vortex zone at the centre of the wake, whereas the tip vortex formed a negative vortex zone near the centre. As the wake developed, the overall distribution of the vortex structure remained almost unchanged; however, the vortex intensity decreased. Under the influence of the vortex at the tail of the upstream turbine, the downstream turbine increased the intensity of the entire vortex system, removing more energy and resulting in less energy for the downstream turbine to convert into electrical energy. The improved CFD(ALM)-IDWM ensured that the vorticity in the x-direction was not numerically dissipated, capturing the physical phenomenon of strength weakening of the negative vortex region of the blade root vortex after being affected by the circular positive vortex region of the upstream blade root vortex, whereas CFD(ALM)-DWM did not exhibit this phenomenon.
Figure (b) shows the y-vorticity. The Lift-induced drag generated by the vorticity in the y- and z-directions directly affected the thrust of the wind turbine. The vorticity of the wake simulated by CFD(ALM)-DWM in the y-direction was relatively high, generating greater vorticity in the y-direction after acting on the downstream turbine. Based on the analysis shown in Figure , this may be one of the reasons for the decrease in the thrust amplitude of the downstream turbine.
Figure shows the vorticity magnitude for Case 14. A nonphysical rectangular positive vortex zone appeared on the ground of the downstream turbine, which did not occur within the downstream turbine disk and had little effect on the vorticity of the downstream turbine. However, IDWM used in this study did not handle the ground boundary well; thus, it must be improved in future studies.
Figure 28. Vorticity magnitude for Case 14 simulated by different methods. (A): full CFD(ALM), (B): CFD(ALM)-IDWM.

This study introduced the Q-criterion to study the evolution of wind turbine wakes, such as generation, evolution, and fluid dissipation. The Q-criterion (Jeong & Hussain, Citation1995) is defined as:
(14)
(14) where Ω and S are the antisymmetric and symmetric parts of the velocity gradient tensor
, corresponding to deformation and rotation in the flow field, respectively; thus, the Q-criterion has the physical meaning that the rotation motion of fluid microelements are stronger than the deformation as follows:
(15)
(15)
(16)
(16) As shown in Figure , the second-generation eddy current identification method ‘Q criterion’ captures the eddy current structures in the plane of two turbines. The helix-shaped vortex (red dot-shaped area at the blade tip) was released from the blade tips of all upstream turbines. The weak eddy currents at the trailing edges of the blades gradually weakened and disappeared. Because of the influence of the upstream wake, no helix-shaped vortex was observed after T2. The Q-criterion captured the tip and root vortices in the plane of the wind rotor in both the upstream and downstream wind turbines.
As shown in Figure , the second-generation eddy current identification method ‘Q criterion’ captures the eddy current structures in the plane of the downstream turbine. Comparing CFD(ALM)-IDWM and CFD(ALM)-DWM, CFD(ALM)-IDWM can accurately simulate the location, size, and shape of upstream wake hub vortices. After the wake interacts with the downstream turbine, the Q amplitude at the root of the blade tip is relatively large; however, the phenomenon is physical.
5.3. Computational efficiency of the hybrid method
As previously mentioned, the main goal of developing new methods is to conserve the grid for the intermediate calculation wake and enhance the computational efficiency when computing two in-line wind turbines. To verify that CFD(ALM)-IDWM requires less computational time than full CFD(ALM), we used the scenario outlined in Section 5.1. All cases were computed on workstations equipped with 24 Intel (R) Xeon (R) CPU E5-2660 [email protected] GHz processors and were used to calculate all the cases listed in Table . As stated in Section 5.1, the downstream inlet was located 0.5D away from the wind turbine.
Figure shows the computational times required by the four methods. For two in-line wind turbines, specifically case 7 and 8, the computational time required by CFD(ALM)-IDWM is approximately 60% less than that of the full CFD(ALM). The longer the computational domain of IDWM, the greater reduction in computational time. From the above results, it can be seen that after using IDWM instead of CFD(ALM) to simulate the far wake of upstream wind turbine, the downstream turbines can still obtain thrust and torque mean values with error less than 5%. This shows that the hybrid method proposed in this paper is reasonable.
In fact, compared with full CFD(ALM), the computational time reduced by CFD(ALM)-IDWM means that when calculating the flow field between two wind turbines, IDWM method is used to replace part of CFD(ALM) to calculate the far wake of upstream wind turbine, while the computational time used by IDWM is negligible relative to CFD.
As previously mentioned, the CFD(ALM) utilised in this paper is the traditional version. Replacing it with an improved CFD(ALM) would further enhance the calculation efficiency.
6. Summary and conclusions
In this study, a new hybrid model, which is an extension of CFD(ALM)-IDWM proposed by Yuan et al. (Citation2023), was proposed to simulate the wake and aerodynamic loads of two in-line wind turbines. Firstly, the CFD(ALM)-IDWM was improved by improving the forecasting of Vθmax from linear to cubic. After that, two numerical models for two in-line wind turbines are developed by adding a partially overlapping computational domain behind the far-wake domain of upstream wind turbine. They are the full CFD(ALM) and CFD(ALM)-IDWM, in which the far wake of upstream wind turbine is respectively simulated by CFD(ALM) directly and forecasted by improved IDWM.
The full CFD(ALM) was first validated using a wind tunnel model test on two in-line wind turbines. The obtained results indicated that the thrust and torque of the two in-line wind turbines simulated by full CFD(ALM) agreed well with the experimental measurements. Furthermore, consistent results were obtained for the deficit axial velocity of the wake behind the upstream wind turbine.
Subsequently, the hybrid model of CFD(ALM)-IDWM was numerically validated using full CFD(ALM) simulations, and similar numerical results were obtained. Furthermore, it can be concluded that higher-order interpolation methods for calculating the wake result in more precise predictions of the thrust and torque of the downstream turbines.
Furthermore, the behaviours of CFD(ALM)-IDWM were investigated. For the inlet boundary location of ΩCFD(ALM)-T2, it revealed that the simulation results agreed well with those of the full CFD(ALM) simulation when the distance from inlet boundary to wind turbine was 0.5D. For two in-line wind turbines, even if the distance of them was 10D, the thrust and torque of the downstream turbine were still severely affected by the wake of the upstream turbine. When the the distance of them is 7D, the CFD(ALM)-IDWM required approximately 40% less CPU time than the full CFD(ALM) simulation. The larger the distance between the two turbines, the longer the computational domain of IDWM, the greater reduction in computational time.
Regrettably, the hybrid model fails to capture certain flow field details including acceleration, turbulent viscosity, and Reynolds stress during the simulation process. Consequently, the accuracy of this information in the downstream calculation domain is compromised. To address this issue in future research, it is suggested to explore the inclusion of appropriate manual numerical corrections to enhance this particular data. This will ensure consistency between the thrust and torque amplitudes of downstream wind turbines and the results obtained from CFD (ALM).
Acknowledgments
This work is sponsored by the National Natural Science Foundation of China (No.51739001), the RISUD project of the Hong Kong Polytechnic University (no. 1-BBWT). The research was financially supported by the Key Laboratory Fund for Equipment Pre-research (6142223180210). A part of the research related to this paper was carried out when the first author was a visiting student at City, University of London under the support of CSC studentship
Disclosure statement
No potential conflict of interest was reported by the author(s).
Additional information
Funding
References
- Ainslie, J. F. (1988). Calculating the flow field in the wake of wind turbines. Journal of Wind Engineering and Industrial Aerodynamics, 27(1–3), 213–224. https://doi.org/10.1016/0167-6105(88)90037-2
- Bastankhah, M., & Porté-Agel, F. (2014). A new analytical model for wind-turbine wakes. Renewable Energy, 70(1), 116–123. https://doi.org/10.1016/j.renene.2014.01.002
- Berg, J., Troldborg, N., Sørensen, N. N., Patton, E., & Sullivan, P. (2017). Large-eddy simulation of turbine wake in complex terrain. Journal of Physics: Conference Series, 854, 012003. https://doi.org/10.1088/1742-6596/854/1/012003
- Churchfield, M. J., Lee, S., Michalakes, J., & Moriarty, P. J. (2012). A numerical study of the effects of atmospheric and wake turbulence on wind turbine dynamics. Journal of Turbulence, 13. https://doi.org/10.1080/14685248.2012.668191
- Devenport, W. J., Rife, M. C., Liapis, S. I., & Follin, G. J. (1996). The structure and development of a wing-tip vortex. Journal of Fluid Mechanics, 312, 67–106. https://doi.org/10.1017/S0022112096001929
- Ennis, B. L., Kelley, C. L., & Maniaci, D. C. (2015). Dynamic wake meandering model comparison with varying fidelity models for wind turbine wake prediction. 4(January 2015), 2885–2894. https://www.osti.gov/servlets/purl/1331655
- Feng, D. H., Li, L. K. B., Gupta, V., & Wan, M. P. (2022). Componentwise influence of upstream turbulence on the far-wake dynamics of wind turbines. Renewable Energy, 200, 1081–1091. https://doi.org/10.1016/j.renene.2022.10.024
- Frandsen, S. T., Barthelmie, R. J., Pryor, S. C., Rathmann, O., Larsen, S. E., Højstrup, J., & Thøgersen, M. (2006). Analytical modelling of wind speed deficit in large offshore wind forms. Wind Energy, 9(1–2), 39–53. https://doi.org/10.1002/we.189
- Hao, H. B., Liao, K. P., Ma, Q. W., Zheng, X., Sun, H. B., & Khayyer, A. (2023). Wind turbine model-test method for achieving similarity of both model- and full-scale thrusts and torques. Applied Ocean Research, 130(January 2023), 103444. https://doi.org/10.1016/j.apor.2022.103444
- Jensen, N. O. (1983). A note on wind turbine interaction. Risoe National Laboratory.
- Jeong, J., & Hussain, F. (1995). On the identification of a vortex. Journal of Fluid Mechanics, 285, 69–94. https://doi.org/10.1017/S0022112095000462
- Jonkman, J., & Shaler, K. (2021). FAST. Farm user's guide and theory manual. Tech. Rep. NREL/TP-5000-7848. National Renewable Energy Laboratory. https:// www.nrel.gov/docs/fy21osti/78485.pdf
- Larsen, G. C. (1988). A simple wake calculation procedure. Tech note Risø-M-2760. Risø National Laboratory.
- Larsen, G. C., Madsen, H. A., Bingol, F., Mann, J., Ott, S., Sorensen, J. N., Okulov, V., Troldborg, N., Nielsen, M., Thomsen, K., Larsen, T. J., & Mikkelsen, R. (2007). Dynamic wake meandering modeling. Denmark. https://www.osti.gov/etdeweb/servlets/purl/20941220
- Lee, M., & Moser, R. D. (2015). Direct numerical simulation of turbulent channel flow up to Reτ ≈ 5200. Journal of Fluid Mechanics, 774, 395–415. https://doi.org/10.1017/jfm.2015.268
- Porté-Agel, F., Bastankhah, M., & Shamsoddin, S. (2020). Wind-turbine and wind-farm flows: A review. Boundary-Layer Meteorology, 174(2020), 1–59. https://doi.org/10.1007/s10546-019-00473-0
- Pryor, S. C., Shepherd, T. J., Volker, P., Hahmann, A. N., & Barthelmie, R. J. (2020). “Wind theft” from onshore wind turbine arrays: Sensitivity to wind farm parameterization and resolution. Journal of Applied Meteorology and Climatology (1). https://doi.org/10.1175/JAMC-D-19-0235.1
- Shen, W. Z., Mikkelsen, R., Sørensen, J. N., & Bak, C. (2005). Tip loss corrections for wind turbine computations. Wind Energy, 8(4), 457–475. https://doi.org/10.1002/we.153
- Sørensen, J. N., & Shen, W. Z. (2002, June). Numerical modeling of wind turbine wakes. Journal of Fluids Engineering, 124(2), 393–399. https://doi.org/10.1115/1.1471361
- Vanderwende, B. J., Kosovic´, B., Lundquist, J. K., & Mirocha, J. D. (2016). Simulating effects of a wind-turbine array using LES and RANS. Journal of Advances in Modeling Earth Systems, 8(3), 1376–1390. https://doi.org/10.1002/2016MS000652
- Wang, Q., Liao, K. P., & Ma, Q. W. (2020). The influence of tilt angle on the aerodynamic performance of a wind turbine. Applied Sciences, 10(15), 5380. https://doi.org/10.3390/app10155380
- Wang, T., Cai, C., Wang, X., Wang, Z., Chen, Y., Hou, C., Zhou, S., Xu, J., Zhang, Y., & Li, Q. (2023). Evolution mechanism of wind turbine wake structure in yawed condition by actuator line method and theoretical analysis. Energy Conversion and Management, 281, 116852. https://doi.org/10.1016/j.enconman.2023.116852
- Yoo, S., & Oh, S. (2021). Flow analysis and optimization of a vertical axis wind turbine blade with a dimple. Engineering Applications of Computational Fluid Mechanics, 15(1), 1666–1681. https://doi.org/10.1080/19942060.2021.1989041
- Yu, Z. Y., Ma, Q. W., Zheng, X., Liao, K. P., Sun, H. B., & Khayyer, A. (2023). A hybrid numerical model for simulating aero-elastic-hydro-mooring-wake dynamic responses of floating offshore wind turbine. 268(15 January 2023), 113050. https://doi.org/10.1016/j.oceaneng.2022.113050
- Yuan, Y. M., Ma, Q. W., Yan, S. Q., Zheng, X., Liao, K. P., Ma, G., Sun, H. B., & Khayyer, A. (2023). A hybrid method for modelling wake flow of a wind turbine. Ocean Engineering, 281(1 August 2023), 114770. https://doi.org/10.1016/j.oceaneng.2023.114770
Appendix A
Effect of horizontal offset distance on downstream wind turbine
For two parallel wind turbines with different horizontal offset distances, as shown in Figure , the full CFD(ALM) and CFD(ALM)-IDWM simulations were conducted for the cases listed in Table . In these simulations, B1 represents the offset distance in the positive direction of the y-axis, and R indicates the wind turbine radius, which is 0.9 m as stated in the paper.
When T2 is arranged at different horizontal distances (B1), Figure presents the average thrust and torque values of T2 as a percentage of T1. Compared to the full CFD(ALM), It is observed that the relative errors of the results simulated by CFD(ALM)-IDWM are below 5%. Additionally, Figure illustrates the dimensionless axial velocity of the wake in ΩCFD(ALM)-T2. This observation suggests a strong agreement between the simulations conducted by CFD(ALM)-IDWM and the full CFD(ALM) model. Consequently, these findings attest to the high accuracy of employing CFD(ALM)-IDWM to explore the horizontal layout of turbines.
Figure A2. T2 is arranged at different horizontal distances (B1) to obtain the average thrust and torque values as a percentage of T1 (Error bar 5%).

Figure displays the time histories of the aerodynamic thrust and torque simulated by CFD(ALM)-IDWM. In the figures, the aerodynamic thrust and torque of upstream turbine are denoted as T1, which remains unaffected by B1. With the increasing of B1, the effect of upstream wind turbine on the aerodynamic thrust and torque of downstream wind turbine gradually diminishes. When B1 increases to 1.5R, the average thrust and torque of T2 exceed 95% of T1.
Figure illustrates the x-vorticity of the wake in ΩCFD(ALM)-T2 with Q = 0.1. The influence of the upstream wind turbine on this wake gradually diminishes as B1 increases. When B1 reaches 1.5R, only the tip vortex originating from the wake of the upstream turbine moderately affects the tip of the downstream turbine.
Table A1. Numerical simulation of staggered arrangement of two wind turbines.