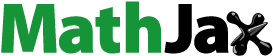
Abstract
Current work explores the intricacies of magnetohydrodynamic and mix convectional boundary-layer flow concerning couple stress Casson nanofluid (CSCNF) dynamics via a 3D stretchable surface. The addition of active and passive control mechanisms for nanoscales creates an innovative dimension to the exploration. Remarkably, the analysis incorporates the influence of non-Fourier and non-Fickian heat and mass flux, alongside the effects of thermophoresis and Brownian diffusion, to systematically investigate the heat and mass transportation phenomena. The governing equations (PDEs) describing the MHD-3DCSCNF model are converted into a set of ordinary differential equations to facilitate the ANN analysis. Employing the bvp4c technique, a dataset is systematically generated for the back propagation artificial neural network with Levenberg–Marquardt Algorithm (BANN-LMA) through four distinct scenarios. Via accurate testing, validation, and training, the BANN-LMA produces estimated results for the MHD-3DCSCNF problem. The performance validation of BANN-LMA is executed through several metrics, involving the mean squared error, error histogram and regression analysis. The training process, characterized by minimizing the MSE through a gradient descent methodology with optimized weights, exhibits a compelling correlation R = 1, between the target and network output. Furthermore, the consistent convergence observed highlights the method robustness and reliability.
1. Introduction
An important and cutting-edge technique to artificial intelligence is exemplified by artificial neural networks (ANNs). ANNs are remarkably adaptable, dynamically responding to the data that flows through the network while learning, whether it comes from within or outside the system. Artificial neural networks make use of the Back-Propagation approach for concurrent training to improve the performance of Multilayer Perceptron networks. This approach stands out as the most common, effective, and understandable framework for complex multi-layered networks. Backpropagation is a supervised learning technique that follows the gradient of the error curve to reduce network errors. Notably, Paul Werbos first introduced this algorithm in 1974. Rumelhart and Parker just revived it. In feedforward multilayer neural networks, backpropagation is now a frequently used learning technique. The Levenberg–Marquardt methodology offers computational solutions to a wide range of fluid flow problems in various technical disciplines by introducing a novel and convergent stability method for ANNs. Newtonian and non-Newtonian fluid systems have been the subject of recent research, which used the Levenberg–Marquardt artificial neural network (LMA-NN) for experimentation. To examine the impact of radiative heat transport on the Williamson fluid flow model, (Shoaib, Raja, et al., Citation2022) reported a ground-breaking use of the LMA-NN. By considering mixed convectional and slip impact between rotating disks, (Zhao et al., Citation2023) used LMA-NN to systematically examine the dynamics of Ree-Eyring fluid flow. Furthermore, led to a deeper knowledge of the fluid, (Asif Zahoor Raja et al., Citation2022) used the computational intelligence algorithms based on LMA-NN to learn more about ferrofluid flow, incorporating the effects of both magnetic forces and nonlinear thermal radiation effects. Recent studies (Dai et al., Citation2023; Karimmaslak et al., Citation2021; Z. Shah et al., Citation2022; B. K. Sharma et al., Citation2023; Shoaib, Kainat, et al., Citation2022; X. Zhang et al., Citation2022; Zuhra et al., Citation2022) that use the ANN approach to analyse heat transport mechanisms in various geometries while taking a variety of characteristics are presented in the literature.
In thermodynamic processes, irreversibility is caused by several phenomena, including fluid opposition, radiative heating, biological repetition, energy transmission, chemical changes, and electric and magnetic propagation. Various mechanisms such as energy transportation and movement of liquid inherently demonstrate irreversibility, supporting enhanced entropy and the loss of favourable properties. In these processes, the mechanism of entropy production remains constant during closed isothermal processes, but in case of irreversible, the entropy production increases. Such distinction is helpful in identifying reversible and irreversible mechanisms. When liquid flow experiences a drop in pressure, entropy is produced in any thermodynamic system. Bejan (Citation1980, Citation2013) first investigated the idea of irreversibility in convection liquid flow because of the viscous effects of thermal transport. Tayebi et al. (Citation2020) highlighted the numerical analysis of entropy production in the flow field of a nanofluid in presence of convectional heating. Khan et al. (Citation2020) explained on the statistical modelling of slip flow in a micropolar liquid. Hayat et al. (Citation2020) evaluated entropy-optimized flow with melting heat exchange. The rate of entropy production and nonlinear radiated thermal fluxes over a deformable surface with changeable properties were investigated by Nasir, Berrouk, Aamir, et al. (Citation2023). Entropy improvement in high-temperature surfaces, including nanoparticles, was studied by Boujelbene et al. (Citation2023). In the domain of literature, intriguing and contemporary flow-related challenges linked to the generation of entropy are explored in references (Mandal & Pal, Citation2023; Rafique et al., Citation2023; Sakkaravarthi & Reddy, Citation2023).
The quality of energy transmission becomes fundamental as distinct areas inside the same body undergo thermal variations. Fourier first proposed the idea of coolant temperature conduction, and Catteneo later improved it by adding a relaxation time to address the paradox of heat conduction. The Cattaneo–Christov heat flux model (CCHFM), which incorporates the notion of relaxation time, was created because of Christov’s further development of the Fourier model. The CCHFM was used by Nayak et al. (Citation2022) to investigate heat transmission in hybrid nanofluids and the subsequent entropy formation. The CCHFM was used by Gowda et al. (Citation2021) to examine heat transmission in a nanofluid flow. To study the movement of chemically reactive micropolar carbon nanotube-blood nanofluid through a constriction channel, (Shaw, Citation2021) applied the idea of CCHFM mechanism. Using the CCHFM, (Gowda et al., Citation2023) investigated the flow of liquid nanomaterial over a surface. The effect of the CCHFM on the flow of ferromagnetic Maxwell nanofluids across an extended geometry was explained by F. Shah et al, (Citation2023).
The phenomenon of thermal radiation refers to the transmission of thermal energy between two bodies in the absence of physical contact. Thermal radiation is used by pyrometers and infrared cameras to measure and regulate temperature in a variety of sensing processes. Thermal radiation plays a dynamic role in a wide range of engineering applications, especially at high temperatures. Convective heat transfer was examined by Jayaprakash et al. (Citation2022) together with radiative heat transmission. The impact of a homogenous heat source/sink on the flow of hybrid nanofluids was explored by Soumya et al. (Citation2022). In order to account for heat radiation (Nasir, Berrouk, Gul, et al., Citation2023), described the behaviour of nanofluids over a surface. The effects of heat sources and radiation on the convective flow of a nanoliquid in a cavity were discussed by Gul et al. (Citation2023). Thermal radiation has great uses in the field of medical sciences in addition to industrial and engineering domains. As a result, (Hsu et al., Citation2017) evaluated potential application in the field of medicine, providing illumination on how thermal radiation from the human body might result in both heating and cooling effects. The vital importance of maintaining a healthy body temperature as a basic requirement for living is emphasized by their research. In a similar fashion, (Wehinger & Flaischlen, Citation2019) established frameworks for thermal radiation in computational fluid dynamics, (Petela, Citation2010) concentrated on industrial thermodynamics, (Navarro et al., Citation2021; Wang et al., Citation2023) investigated thermal radiation within fluid dynamics. All the authors researched the novel and advanced uses of thermal radiation in various contexts.
The properties of mixed convective flow across an extended surface have numerous technological and industrial applications. When gravitational forces are at work, the effect of free convection is more prominent. The mechanisms of fluid flow and energy transmission are impacted by both the strained and buoyant forces. Thermal buoyancy, which affects the rate of heat movement in manufacturing environments, results from variations in the heat on the expanding surface. Applications of this phenomenon include precautionary stoppage of nuclear cooler systems, environmental fluxes, heaters, photovoltaic panels, conditioning of electrical gadgets, oceanic mechanics, low-velocity heat transfer mechanisms, precooler mechanisms, vehicle demisters, and more. According to (Patil et al., Citation2020), mixed convective nanoparticle transport along an exponentially expanded porous surface was influenced by suction/injection. Mixed convective radiative flow over an inclined permeability surface was explored by Moradi et al. (Citation2013). Time-dependent magneto Williamson nanoparticle flow through a stretchy surface was evaluated by Das et al. (Citation2022) considering velocity slips, nonlinear mixed convection, and radiative heat effects. In contrast to the focus on their Newtonian behaviour, the impact of nanofluids’ non-Newtonian properties on their heat transfer rate has not been adequately investigated. Many nanofluids display non-Newtonian characteristics, even though a lot of study has been devoted to employing Newtonian nanofluids to improve energy transmission (Elboughdiri et al., Citation2023; Rasool et al., Citation2023; J. Sharma et al., Citation2023; Wakif, Citation2023; Wakif et al., Citation2022; K. Zhang et al., Citation2023).
To the author’s knowledge, most of the literature in the area of Casson couple-stressed nanofluids concentrates on convective boundary conditions rather than active and passive control nanoparticle boundary conditions for examining thermal and concentration distributions. The analysis of the double-diffusive Cattaneo–Christov energy flux in couple stress nanofluids using the theory from reference (Hayat et al., Citation2017) while simultaneously considering passive and active control conditions has also not been the subject of any published study. Additionally, this theoretical and numerical studies have the potential to advance the field of bio-fluid dynamics and have applications in several engineering fields, including photovoltaic conversion, nuclear reactor implementation, cooling systems, and electronic generators. So, according to the authors, it helps to investigate thermal radiation, magnetohydrodynamic effects, and Darcy-analysis Forchheimer’s of couple stress nanofluids’ three-dimensional mixed convection flow. Because of this, authors feel it is appropriate to investigate the effects of passive and active control on magnetohydrodynamics, thermal radiation, Darcy-analysis Forchheimer’s of three-dimensional mixed convection flow of couple stress nanofluid with non-heat Fourier’s flux, and non-mass Fick’s flux theory. The couple stress nanofluid flow of magnetic, mix convectional, and double-diffusive Cattaneo–Christov energy flux within porous media is investigated in this research using the BANN-LMA approach. The importance of the constructed ANN procedure is emphasized through a comparison of convergence and accuracy measures, employing performance analysis, regression, correlation, histograms, and the Mean Squared Error. The reference dataset for the ANNs is established using the bvp4c technique for various model parameter variations. Additionally, using graphs and tables, the effects of various parameters on velocity, temperature, and concentration fields are thoroughly explored and explained.
2. Mathematical formulation
Here, a mathematical model for steady, incompressible Couple stress nanofluid flowing through a nonlinear extending sheet submerged in a porous media created by Darcy and Forchheimer is represented. In this analysis the surface is traced at and the fluid flow is produced as the extending of sheet surface in
and
directions. Also, we assume that the starching velocity along
direction is
and along
direction is
(Gowda et al., Citation2023; Ramzan et al., Citation2013). Where
and
are positive constants. Its also suggested that
constant surface and
ambient temperature such that
. Furthermore, we settled that
constant surface and
ambient temperature such that
. In this effort, the Buongiorno nanofluid model and Cattaneo–Christov heat and mass fluxes are also used (Figure ).
2.1. System assumptions and limitations
In the current flow system, we have implemented the following assumptions and constraints:
Three-dimensional flow
Time independent flow
Incompressible fluid
Couple stress fluid model
Dorcy Forchheimer porous medium
Cattaneo–Christov heat and mass fluxes models
The heat flux and mass flux
model is presented as follows (F. Shah et al., Citation2023):
(5)
(5) where
relaxation time heat flux and
relaxation time mass flux. Equation reduces classical Fouriers and Fick’s law for
(Shaw, Citation2021; Zuhra et al., Citation2022). Since the fluid flow in this suggested model is mass-conserving, or steady, at
, Equation (5) become.
(6)
(6)
For the isotropic and incompressible flow of a Casson fluid, the rheological equation of state is as follows (J. Sharma et al., Citation2023; Wang et al., Citation2023; Zuhra et al., Citation2022).
(7)
(7) Here
is the product of the component of deformation rate with itself, where
and
is the
component of the deformation rate,
is the critical value of this product based on the non-Newtonian model,
is the plastic dynamic viscosity of the non-Newtonian fluid and
is the yield stress of the fluid (Sakkaravarthi & Reddy, Citation2023), but for Casson fluid we supposed
and
, the dynamic viscosity could be described as
(8)
(8)
We obtain by inserting the value of
(9)
(9)
The high order system of partial differential equations formed from the controlling problem are as follows, based on the above-mentioned explanations (Gowda et al., Citation2023; Ibrahim et al., Citation2022; Ramzan et al., Citation2013; Shaw, Citation2021; Zuhra et al., Citation2022):
(10)
(10)
(11)
(11)
(12)
(12)
(13)
(13)
(14)
(14) where
and
(velocities components),
(Casson parameter),
(electrical conductivity),
(density),
(magnetic field),
(porous medium permeability),
(porous medium inertia coefficient),
(Drag force),
and
(thermal and concentration relaxation time coefficient),
,
(thermal diffusivity and conductivity),
(heat capacities ratio),
(effective heat capacity of fluid),
(Brownian diffusivity),
(thermophoretic diffusion coefficient).
2.2. Boundary conditions
The relevant boundary conditions are as follows (Elboughdiri et al., Citation2023; Ibrahim et al., Citation2022; Rasool et al., Citation2023):
(15)
(15)
The arising terms and
from Equations (13) and (14) are estimated as:
(16)
(16)
Considering the appropriate similarity transformations as (Ibrahim et al., Citation2022):
(17)
(17) where the velocity components for present investigations are represented as
(18)
(18)
The aforementioned equations can be substituted in governing partial differential equations which transform them into couplet ODEs of the following form:
(19)
(19)
(20)
(20)
(21)
(21)
(22)
(22)
With transform boundary conditions:
(23)
(23) where the term
and
are
(24)
(24)
(25)
(25) where the several model parameters obtained after transformation are presented in Table .
Table 1. Details regarding the dimensions and arrangement of the proposed network.
The engineering aspects for the current analysis are and
are given by Gowda et al. (Citation2023), Ibrahim et al. (Citation2022), Ramzan et al. (Citation2013) and Zuhra et al. (Citation2022):
(26)
(26) Here terms
surface heat flux and
mass flux mathematically stated as:
(27)
(27)
In non-dimensionless form is
(28)
(28)
When the mass flux is equal to zero, then the Sherwood number will also equal zero.
(29)
(29) Equation (29) signifies the Local Reynolds number.
3. Solution methodology
3.1. Formation of data set
Assuming and organizing the relevant information for training the BANN-LMA or for resolving fluid mechanics related problems is referred to as the process of constructing a dataset for projected solutions. Such data set operates as the foundation for training and evaluating the performance of several algorithms, containing neural networks. The choice of representative models, data preparation, and ensuring data integrity and quality are all carefully considered. The dataset’s extensiveness and structure play a crucial role in assisting the model to generalize and produce dependable results. Consequently, the creation of a well-crafted dataset stands as a critical initial phase when utilizing neural networks for successful solutions. With regards to addressing the nonlinear couple stress Casson fluid model (CSCFM), the strategies and techniques presented here are characterized into the following three parts. The classification of present nonlinear CSCFM fluid model contains three categories, specifically velocity field, temperature field, and concentration field. Within each classification, there are two distinct cases. The first phase offers the principal data for the numerical solver bvp4c. This solver is working to establish the dataset based on final couplet system of equations. This consists of transforming higher-order nonlinear ODEs into first-order ODEs while containing the boundary conditions. The succeeding phase presents a suggested computational framework, BANN-LMA, which involves elements like the backpropagation procedure, layer layout, and network plan. The concluding phase offers guidelines for applying the suggested BANN-LMA methodology (Asif Zahoor Raja et al., Citation2022; Z. Shah et al., Citation2022; Shoaib, Raja, et al., Citation2022) to address the tasks of the nonlinear CSCFM. The resulting higher-order ODEs after transformation are (Ibrahim et al., Citation2022):
(30)
(30)
(31)
(31)
(32)
(32)
(33)
(33)
(34)
(34)
With transform boundary conditions:
(35)
(35)
3.2. Creating artificial neural network
In programming courses, artificial neural networks (ANNs) are a crucial tool. Artificial neural networks, which were originally utilized in the middle of the 20th century, gained popularity because of their improved ability to handle complex nonlinear functions (Dai et al., Citation2023; Shoaib, Raja, et al., Citation2022). The most well-known multilayer perceptron (MLP) systems are used in artificial neural network (ANN) models (Zhao et al., Citation2023). The neural network is made up of (i) weights, (ii) input/output, (iii) activation mechanism, and hidden layer. Backpropagation feed-forward (BPFF) is the most popular approach used in multilayer perceptron networks (Shoaib, Kainat, et al., Citation2022). The network then creates a relationship with at least one hidden layer, where the weighted connection’s structure is doing critical computations. Towards the end of training, error eventually decreases. Four ANN models were created in the current study to forecast the movement of a CSCFM through an expanding medium utilizing the Cattaneo–Christov and Darcy–Forchheimer models. Weights described as an input limitation in the involvement of neurons in the hidden layer as well as the prediction values collected in shape of system performance, gradient and histogram investigation at the output layer of the artificial neural networks model developed for the prediction of and
against
and
. In Figure (a,b) we see the basic framework for setting up the proposed ANN models. Only 15% of the input used in the developed ANN models is intended for use in the testing process, 15% is intended for use in the validation stage, and 70% is intended for use in ANN model training. A MLP network model was designed to estimate the variables
and
against
and
using 410 data sets from 8 distinct data components. 349 samples were used for training in this analysis, whereas 61 data sets were used for testing and validation.
3.3. ANN optimization
The sigmoid function, whose expression is is the weighted sum of input connection has been included in the present research as the transformation function /activation function of the MLP network. Among the most popular transformation functions is the sigmoid function. Its precise performance on nonlinear regression issues in various applications leads to its adoption in MLP networks. During the training phase, errors were evaluated using a performance measure. In the present research, the determination MSE and R2 (correlation coefficient) have been used to assess the network’s accuracy. Which is define as (Asif Zahoor Raja et al., Citation2022; Z. Shah et al., Citation2022; Shoaib, Raja, et al., Citation2022; Zhao et al., Citation2023):
(36)
(36)
(37)
(37)
4. Results and discussion
Using MATLAB ‘bvp4c’ numerical solver, the nonlinear differential equations and their related boundary conditions are solved numerically. The original differential equations are changed into first-order form to facilitate this procedure. By changing the values of the physical model parameters, as shown in Table , several scenarios are investigated. Figure depicts the full computing process of current analysis.
Table 2. Non-dimensional model parameters.
4.1. Effect of model parameters on heat and concentration profile
Following the implementation of the MATLAB ‘bvp4c’ numerical solver to acquire the solution of the nonlinear ODEs, the solution is drawn to show the presence of numerous significant factors, including the and
, on the
and
fields. Results are obtained for both passive and active control of nanoparticles. Figure (a,b) displays the variation in
and
for varying values of
. An enhancement in
signifies an augmentation in the
and
profiles for both the active and passive control cases. The magnetic field, which lowers the liquid’s movement and increases the heat and concentration profiles, causes the body force to diminish as would be expected. Additionally, from Figure (a) the
distribution for passive control is smaller than it is for active control. Figure (b) illustrates how the
affect the
profile. By increasing the
, the
profile is enhanced for both active and passive controller scenarios. Figure (c) shows the effect of the
stimulation on
field. To produce stronger convection, the
can be elevated. As a result,
field and thermal layer thickness rise under control situations. Figure (d) is developed to demonstrate how the
impacts the
profile. In this situation, the
profile shows a propensity to rise in both active and passive nanoparticle control conditions. The effect of
on the
and
field is shown in Figure (e,f) for both cases. The collision of fluid atoms or molecules will result in an arbitrary motion known as the Brownian motion of suspended (pendulous) particles, which will enlarge the boundary layer in both active and passive control scenarios, as shown in Figure (e). Figure (f) demonstrates that choosing a higher
parameter value results in a weaker
field for both types of nanoparticles (Table ).
Figure 4. Effect of various parameters on and
profile. (a) Effect of
on
. (b) Effect of
on
. (c) Effect of
on
. (d) Effect of
on
. (e) Effect of
on
. (f) Effect of
on
.

Table 3. Variation in different parameters of ANN for various scenarios.
4.2. Analysis of arterial neural network model results
To implement the suggested solution methodology, reference solutions in the form of the numerical data set produced via bvp4c against the and
are delivered to the neural network input. The neural network is trained with 75% of the points, with the remaining 15% being used for testing and validating the neural network. Figure (a,b) depicts the solution technique design and bi-layer architecture. A visual comparison of a neural network’s output to the output of a fixed domain can be seen in time-series charts. These graphical representations are crucial for recognizing the consistency between the desired and actual values during the network’s training, validation, and testing phases. Figure (a) displays the transient stability for case (1) in scenario (I). An error histogram, which breaks down the full error range into 20 bins, is used to analyse the dispersion of errors. The total number of errors for the network’s training, validation, and testing stages is shown as a vertical line for each error range. The reliability and correctness of the solution outcomes are indicated by the presence of maximum error values near to the zero-error line is
. The error distribution for case (1) in scenario (I) is displayed in Figure (b). Another helpful technique for evaluating the consistency between desired and actual values for the training, validation, and testing phases separately is regression analysis. This evaluation can be done mathematically or visually. A straight line is used to represent the reference solution in graphical representations, while BANN-LMA results are represented by little rings or spots. An
denotes a lack of correlation between the targeted and obtained results, whereas an
indicates outstanding correlation. A regression plot for case (1) in scenario (I) is shown in Figure (c). When analysing the quality and reliability of the machine learning model, the MSE (mean square error) is an essential aspect. MSE is calculated numerically by averaging the squares of the discrepancies between the values that were produced and a benchmark solution. A lower MSE value indicates greater accuracy and reliability in the findings, demonstrating the dependability of the solution technique. The MSE plot for case (1) in scenario (I) over the training, validation, and testing data sets is shown in Figure (d) after 445 epochs in total time 28s with MSE is
.
For scenarios II, III, and IV, the subfigures of Figures – contain time-series response plots (Figures (a), (a), (a)), error histograms (Figure (b) with maximum error values near to the zero-error line is −7.11, 7(b) with −1.5
, 8(b) with −9.6
), regression graphs (Figures (c), (c) and (c)) and MSE plots (Figure (d) after 336 epochs in total time 22s with MSE is 1.804
, 7(d) after 180 epochs in total time 17s with MSE is 2.6915
, 8(d) after 78 epochs in total time 21s with MSE is 3.7068
) respectively.
4.3. Analysis of comparison of suggested and ref solutions with absolute errors
In comparison to the reference dataset of CSCFM, specifically the parameter , Figure shows the resultant graphs and evaluation of the variation in
using BANN-LMA. We investigate the effect of the
on
in Figure (a). The behaviour of
depends on the couple stress viscosity, which acts as a barrier to flow and increases fluid density, which in turn increases fluid motion. Figure (a) demonstrates clearly how fluid velocity inside the flow zone accelerates as value of
increases. An assessment of
s absolute errors is shown in Figure (b), with the absolute errors lying within the range 10−4 and 10−8. While observing Figure (a,b), it becomes evident that
profile experience a reduction as the value of
increases, and this is accompanied by absolute error ranges between 10−3 and 10−7. This decline in
profile is mostly because of the Lorentz force produced by the applied magnetic field. It’s significant that the Lorentz force resists the motion of liquid, preceding to a reduction in its overall motion. Furthermore, such force creates additional heat, identified as Joule heating, within the system. Therefore, the employment of higher value of
in the system results in an expansion in
profile within the flow region, which is spotted in both active and passive control cases, as indicated in Figure (a,b) with absolute error ranges ranging from 10−5 to 10−8. In Figure (a,b), we display the visual interpretations of how
impact the
in both scenarios, while simultaneously operating an AE analysis within the range of 10−5 to 10−8.
plays an extensive role in governing the transfer of heat energy within and between the liquid and its surroundings. The
serves as a quantification of the impact of radiation in the situation of heat transport. A higher value
specifies an elevated status of radiation effects, indicating to higher rates of heat transport and alterations in thermal distribution. As the
escalates, the surface temperature also experiences an increase, then ensuing a rise in both the thickness of the thermal boundary layer and thermal resistance. Also, observing Figure (a,b), it becomes evident that
profile experience a reduction as the value of
increases in case of active control while for passive control present opposite behaviour, and this is accompanied by AE ranges between 10−5 and 10−7. Similarly, the employment of higher value of
in the system results in an expansion in
profile within the flow region, which is spotted in both active and passive control cases, as indicated in Figure (a,b) with absolute error ranges ranging from 10−3 to 10−8.
Figure (a) shows comparisons between the current study and existing literature (Ramzan et al., Citation2013), whereas Figure (b) emphasizes the numerical comparison between numerical and ANN outcomes.
Tables show the significance of each variation within the suggested methodology and were individually prepared for each of the four scenarios (1–4). (a) illustrates the contrast between our present work and the existing literature (Ramzan et al., Citation2013), whereas (b) demonstrates the validation of our findings by comparing the ANN results with the numerical dataset. Both figures exhibit a high level of concordance, thereby guaranteeing the precision of our current work and preventing any potential confusion or misinterpretation.
Figure 15 (a) Assessment of result with published work (Ramzan et al., Citation2013) for
, when
, (b) Comparison of
numerical and ANN results.

Table 4. Outcome of LMBP-NNs (Levenberg–Marquardt backpropagation neural networks) technique scenario 1.
Table 5. Outcome of LMBP-NNs (Levenberg–Marquardt backpropagation neural networks) technique scenario II.
Table 6. Outcome of LMBP-NNs (Levenberg–Marquardt backpropagation neural networks) technique scenario III.
Table 7. Outcome of LMBP-NNs (Levenberg–Marquardt backpropagation neural networks) technique scenario IV.
5. Conclusions
The present investigation aims to explore the impacts of coupling stress Casson fluid models with convectional properties under active and passive control using advanced AI-based computational methodologies. These approaches power the neural networks enhanced with the Levenberg–Marquardt Algorithm (NN-LMA). The investigation incorporates the Cattaneo–Christov heat and mass flux models and employs the Rosseland approximation to account for thermal radiation effects. The basic partial differential equations (PDEs) explaining flow patterns are transformed into ODEs through appropriate conversion manner. This system of equations are then solved using the robust bvp4c solver to produce datasets for ANN analysis. Subsequently, the BANN-LMA method is applied to the reference dataset to approximate solutions for the CSCFM models. As shown by a minimal MSE of about 10−10 in the training charts, the findings of this study show a high degree of agreement between the approximations and the real data. In error histograms, around 90% of the output dynamics are centred around the zero line, demonstrating a strong agreement between expected and actual values. Additionally, the regression value constantly equals 1 in each case, highlighting the precision of the results. The main objective of the current analysis is described below, focusing on how the physical characteristics of couple stress Casson fluid models change.
The
parameter reduces
profile and results in a thinner momentum boundary layer, while enhances the fluid’s thermal behaviour.
Increasing the
and
parameter leads to an acceleration of fluid motion.
distribution can be controlled by adjusting the
and
to finer values.
As the
increases, the mass transfer rate declines, while the
exhibits the opposite trend.
A higher
signifies increased convective effects, which enhance the temperature.
In the future, one could employ the remarkable capabilities of artificial intelligence-based methodologies, utilizing both supervised and unsupervised neural networks, in various applications within the field of fluid mechanics such as (Nasir, Alghamdi, et al., Citation2023; Ramzan et al., Citation2013; Wang et al., Citation2023; K. Zhang et al., Citation2023). Future study may also expand on the current research to include non-Newtonian liquids and unsteady behaviour under diverse physical conditions. To ensure that the study continues to make significant contributions and remains current in its specific field, these suggested areas for extension might serve as a road map for future research directions.
Nomenclature
List of symbols
= | Components of velocities | |
= | Magnetic field strength | |
= | Dimensional velocity profile | |
= | Fluid and wall temperature | |
= | Free surface temperature | |
= | Inertia coefficient | |
= | Magnetic parameter | |
= | Rosseland means absorption constant | |
= | Prandtl number | |
= | Local Reynolds number | |
= | Drag force | |
= | Porous medium permeability | |
= | Brownian diffusivity | |
= | Thermophoretic diffusion coefficient | |
= | Radiation parameter | |
= | Fluid, wall, free space concentration | |
= | Specific heat fluid | |
= | Thermal conductivity of fluid | |
= | Couple stress parameter | |
= | Thermophoresis parameter | |
= | Lewis number | |
= | Eckert number |
Greek symbols
= | Dynamic viscosity | |
= | Kinematic viscosity | |
= | Density | |
= | Dimensional thermal, concentration profiles | |
= | Similarity variable | |
= | Nanoparticle volume fraction | |
= | Ratio parameter | |
= | Electrical conductivity | |
= | Stefan Boltzmann constant | |
= | Thermal relaxation time coefficient | |
= | Concentration relaxation time coefficient | |
= | Casson parameter |
Abbreviation
ANN | = | Artificial neural network |
BANN-LMA | = | Back propagation artificial neural network with Levenberg–Marquardt Algorithm |
CSCNF | = | Couple stress Casson nanofluid |
MSE | = | Mean square error |
AE | = | Absolute error |
MLP | = | Multi-Layer perception |
ARD | = | Average relative deviation |
PEDs | = | Partial differential equations |
MHD | = | Magneto-hydrodynamics |
Disclosure statement
No potential conflict of interest was reported by the author(s).
Additional information
Funding
References
- Asif Zahoor Raja, M., Shoaib, M., Tabassum, R., Khan, N. M., Kehili, S., & Bafakeeh, O. T. (2022). Stochastic numerical computing for entropy optimized of Darcy–Forchheimer nanofluid flow: Levenberg–Marquardt Algorithm. Chemical Physics Letters, 807, 140070. 10.1016j.cplett.2022.140070
- Bejan, A. (1980). Second law analysis in heat transfer. Energy, 5(8–9), 720–732. doi:10.1016/0360-5442(80)90091-2
- Bejan, A. (2013). Entropy generation minimization: The method of thermodynamic optimization of finite-size systems and finite-time processes. CRC Press.
- Boujelbene, M., Rehman, S., Alqahtani, S., Alshehery, S., & Eldin, S. M. (2023). Thermal transport and magnetohydrodynamics flow of generalized Newtonian nanofluid with inherent irreversibility between conduit with slip at the walls. Engineering Applications of Computational Fluid Mechanics, 17(1), 2182364. doi:10.1080/19942060.2023.2182364
- Dai, Z., Li, T., Xiang, Z.-R., Zhang, W., & Zhang, J. (2023). Aerodynamic multi-objective optimization on train nose shape using feedforward neural network and sample expansion strategy. Engineering Applications of Computational Fluid Mechanics, 17(1), 2226187. https://doi.org/10.1080/19942060.2023.2226187
- Das, M., Kumbhakar, B., & Singh, J. (2022). Analysis of unsteady MHD Williamson nanofluid flow past a stretching sheet with nonlinear mixed convection, thermal radiation and velocity slips. J. Comput. Anal. Appl, 30(1), 176–195.
- Elboughdiri, N., Reddy, C. S., Alshehri, A., Eldin, S. M., Muhammad, T., & Wakif, A. (2023). A passive control approach for simulating thermally enhanced Jeffery nanofluid flows nearby a sucked impermeable surface subjected to buoyancy and Lorentz forces. Case Studies in Thermal Engineering, 47, 103106. doi:10.1016/j.csite.2023.103106
- Gowda, R. J. P., Al-Mubaddel, F. S., Kumar, R. N., Prasannakumara, B. C., Issakhov, A., Rahimi-Gorji, M., & Al-Turki, Y. A. (2021). Computational modelling of nanofluid flow over a curved stretching sheet using Koo–Kleinstreuer and Li (KKL) correlation and modified Fourier heat flux model. Chaos, Solitons & Fractals, 145, 110774. doi:10.1016/j.chaos.2021.110774
- Gowda, R. J. P., Kumar, R. N., Kumar, R., & Prasannakumara, B. C. (2023). Three-dimensional coupled flow and heat transfer in non-Newtonian magnetic nanofluid: An application of Cattaneo–Christov heat flux model. Journal of Magnetism and Magnetic Materials, 567, 170329. doi:10.1016/j.jmmm.2022.170329
- Gul, T., Nasir, S., Berrouk, A. S., Raizah, Z., Alghamdi, W., Ali, I., & Bariq, A. (2023). Simulation of the water-based hybrid nanofluids flow through a porous cavity for the applications of the heat transfer. Scientific Reports, 13(1), 7009. doi:10.1038/s41598-023-33650-w
- Hayat, T., Aziz, A., Muhammad, T., & Alsaedi, A. (2017). Active and passive controls of Jeffrey nanofluid flow over a nonlinear stretching surface. Results in Physics, 7, 4071–4078.
- Hayat, T., Shah, F., Alsaedi, A., & Ahmad, B. (2020). Entropy optimized dissipative flow of effective Prandtl number with melting heat transport and Joule heating. International Communications in Heat and Mass Transfer, 111, 104454. doi:10.1016/j.icheatmasstransfer.2019.104454
- Hsu, P.-C., Liu, C., Song, A. Y., Zhang, Z., Peng, Y., Xie, J., Liu, K., Wu, C.-L., Catrysse, P. B., & Cai, L. (2017). A dual-mode textile for human body radiative heating and cooling. Science Advances, 3(11), e1700895. doi:10.1126/sciadv.1700895
- Ibrahim, W., Gamachu, D., & Bedada, B. (2022). Entropy generation analysis of three dimensional mixed convection flow of couple stress nanofluid with non-Fourier’s heat and non-Fick’s mass flux model. Alexandria Engineering Journal, 61(11), 8843–8857. 10.1016j.aej.2022.02.021
- Jayaprakash, M. C., Alsulami, M. D., Shanker, B., & Varun Kumar, R. S. (2022). Investigation of Arrhenius activation energy and convective heat transfer efficiency in radiative hybrid nanofluid flow. Waves in Random and Complex Media, 1–13. doi:10.1080/17455030.2021.2022811
- Karimmaslak, H., Najafi, B., Band, S. S., Ardabili, S., Haghighat-Shoar, F., & Mosavi, A. (2021). Optimization of performance and emission of compression ignition engine fueled with propylene glycol and biodiesel–diesel blends using artificial intelligence method of ANN-GA-RSM. Engineering Applications of Computational Fluid Mechanics, 15(1), 413–425. doi:10.1080/19942060.2021.1880970
- Khan, M. I., Alzahrani, F., & Hobiny, A. (2020). Simulation and modeling of second order velocity slip flow of micropolar ferrofluid with Darcy–Forchheimer porous medium. Journal of Materials Research and Technology, 9(4), 7335–7340. doi:10.1016/j.jmrt.2020.04.079
- Mandal, G., & Pal, D. (2023). Estimation of entropy generation and heat transfer of magnetohydrodynamic quadratic radiative Darcy–Forchheimer cross hybrid nanofluid (GO + Ag/kerosene oil) over a stretching sheet. Numerical Heat Transfer, Part A: Applications, 84(8), 853–876. https://doi.org/10.1080/10407782.2022.2163944
- Moradi, A., Ahmadikia, H., Hayat, T., & Alsaedi, A. (2013). On mixed convection–radiation interaction about an inclined plate through a porous medium. International Journal of Thermal Sciences, 64, 129–136. doi:10.1016/j.ijthermalsci.2012.08.014
- Nasir, S., Alghamdi, W., Gul, T., Ali, I., Sirisubtawee, S., & Aamir, A. (2023). Comparative analysis of the hydrothermal features of TiO2 water and ethylene glycol-based nanofluid transportation over a radially stretchable disk. Numerical Heat Transfer, Part B: Fundamentals, 83(5), 276–291.
- Nasir, S., Berrouk, A. S., Aamir, A., & Shah, Z. (2023). Entropy optimization and heat flux analysis of Maxwell nanofluid configurated by an exponentially stretching surface with velocity slip. Scientific Reports, 13(1), 2006. doi:10.1038/s41598-023-29137-3
- Nasir, S., Berrouk, A. S., Gul, T., Zari, I., Alghamdi, W., & Ali, I. (2023). Unsteady mix convectional stagnation point flow of nanofluid over a movable electro-magnetohydrodynamics Riga plate numerical approach. Scientific Reports, 13(1), 10947. doi:10.1038/s41598-023-37575-2
- Navarro, J. M. A., Hinojosa, J. F., & Piña-Ortiz, A. (2021). Computational fluid dynamics and experimental study of turbulent natural convection coupled with surface thermal radiation in a cubic open cavity. International Journal of Mechanical Sciences, 198, 106360. doi:10.1016/j.ijmecsci.2021.106360
- Nayak, M. K., Shaw, S., Waqas, H., & Muhammad, T. (2022). Numerical computation for entropy generation in Darcy–Forchheimer transport of hybrid nanofluids with Cattaneo–Christov double-diffusion. International Journal of Numerical Methods for Heat & Fluid Flow, 32(6), 1861–1882. doi:10.1108/HFF-04-2021-0295
- Patil, P. M., Kulkarni, M., & Hiremath, P. S. (2020). Effects of surface roughness on mixed convective nanofluid flow past an exponentially stretching permeable surface. Chinese Journal of Physics, 64, 203–218. doi:10.1016/j.cjph.2019.12.006
- Petela, R. (2010). Engineering thermodynamics of thermal radiation: For solar power utilization. McGraw-Hill Education.
- Rafique, K., Mahmood, Z., Saleem, S., Eldin, S. M., & Khan, U. (2023). Impact of nanoparticle shape on entropy production of nanofluid over permeable MHD stretching sheet at quadratic velocity and viscous dissipation. Case Studies in Thermal Engineering, 45, 102992. doi:10.1016/j.csite.2023.102992
- Ramzan, M., Farooq, M., Alsaedi, A., & Hayat, T. (2013). MHD three-dimensional flow of couple stress fluid with Newtonian heating. The European Physical Journal Plus, 128(5), 1–15. doi:10.1140/epjp/i2013-13049-5
- Rasool, G., Wakif, A., Wang, X., Shafiq, A., & Chamkha, A. J. (2023). Numerical passive control of alumina nanoparticles in purely aquatic medium featuring EMHD driven non-Darcian nanofluid flow over convective Riga surface. Alexandria Engineering Journal, 68, 747–762. 10.1016j.aej.2022.12.032
- Sakkaravarthi, K., & Reddy, P. B. A. (2023). Entropy generation on Casson hybrid nanofluid over a curved stretching sheet with convective boundary condition: Semi-analytical and numerical simulations. Proceedings of the Institution of Mechanical Engineers, Part C: Journal of Mechanical Engineering Science, 237(2), 465–481. doi:10.1177/09544062221119055
- Shah, F., Hayat, T., & Momani, S. (2023). Non-similar analysis of the Cattaneo–Christov model in MHD second-grade nanofluid flow with Soret and Dufour effects. Alexandria Engineering Journal, 70, 25–35. doi:10.1016/j.aej.2022.10.035
- Shah, Z., Raja, M. A. Z., Khan, W. A., Shoaib, M., Asghar, Z., Waqas, M., & Muhammad, T. (2022). Application of Levenberg–Marquardt technique for electrical conducting fluid subjected to variable viscosity. Indian Journal of Physics, 96(13), 3901–3919. https://doi.org/10.1007/s12648-022-02307-1
- Sharma, B. K., Sharma, P., Mishra, N. K., Noeiaghdam, S., & Fernandez-Gamiz, U. (2023). Bayesian regularization networks for micropolar ternary hybrid nanofluid flow of blood with homogeneous and heterogeneous reactions: Entropy generation optimization. Alexandria Engineering Journal, 77, 127–148. 10.1016j.aej.2023.06.080
- Sharma, J., Ahammad, N. A., Wakif, A., Shah, N. A., Chung, J. D., & Weera, W. (2023). Solutal effects on thermal sensitivity of casson nanofluids with comparative investigations on Newtonian (water) and non-Newtonian (blood) base liquids. Alexandria Engineering Journal, 71, 387–400. doi:10.1016/j.aej.2023.03.062
- Shaw, S. (2021). Impact of cattaneo–Christov heat flux On Al2O3–Cu/H2O–(CH2OH)2 hybrid nanofluid flow between two stretchable rotating disks. Energy Systems and Nanotechnology, 329–368. doi:10.1007/978-981-16-1256-5_17
- Shoaib, M., Kainat, R., Ijaz Khan, M., Prasanna Kumara, B. C., Naveen Kumar, R., & Zahoor Raja, M. A. (2022). Darcy–Forchheimer entropy based hybrid nanofluid flow over a stretchable surface: intelligent computing approach. Waves in Random and Complex Media, 1–24. https://doi.org/10.1080/17455030.2022.2122627
- Shoaib, M., Raja, M. A. Z., Zubair, G., Farhat, I., Nisar, K. S., Sabir, Z., & Jamshed, W. (2022). Intelligent computing with Levenberg–Marquardt backpropagation neural networks for third-grade nanofluid over a stretched sheet with convective conditions. Arabian Journal for Science and Engineering, 47(7), 8211–8229. https://doi.org/10.1007/s13369-021-06202-5
- Soumya, D. O., Gireesha, B. J., Venkatesh, P., & Alsulami, M. D. (2022). Effect of NP shapes on Fe3O4–Ag/kerosene and Fe3O4–Ag/water hybrid nanofluid flow in suction/injection process with nonlinear-thermal-radiation and slip condition; Hamilton and Crosser’s model. Waves in Random and Complex Media, 1–22.
- Tayebi, T., Öztop, H. F., & Chamkha, A. J. (2020). Natural convection and entropy production in hybrid nanofluid filled-annular elliptical cavity with internal heat generation or absorption. Thermal Science and Engineering Progress, 19, 100605. doi:10.1016/j.tsep.2020.100605
- Wakif, A. (2023). Numerical inspection of two-dimensional MHD mixed bioconvective flows of radiating Maxwell nanofluids nearby a convectively heated vertical surface. Waves in Random and Complex Media, 1–22. doi:10.1080/17455030.2023.2179853
- Wakif, A., Abderrahmane, A., Guedri, K., Bouallegue, B., Kaewthongrach, R., Kaewmesri, P., & Jirawattanapanit, A. (2022). Importance of exponentially falling variability in heat generation on chemically reactive von Kármán nanofluid flows subjected to a radial magnetic field and controlled locally by zero mass flux and convective heating conditions: A differential quadrature. Frontiers in Physics, 10, 988275. doi:10.3389/fphy.2022.988275
- Wang, F., Sajid, T., Katbar, N. M., Jamshed, W., Eid, M. R., Abd-Elmonem, A., Mohamed Isa, S. S. P., & El Din, S. M. (2023). Computational examination of non-Darcian flow of radiative ternary hybridity Casson nanoliquid through moving rotary cone. Journal of Computational Design and Engineering, 10(4), 1657–1676. https://doi.org/10.1093/jcde/qwad057
- Wehinger, G. D., & Flaischlen, S. (2019). Computational fluid dynamics modeling of radiation in a steam methane reforming fixed-bed reactor. Industrial & Engineering Chemistry Research, 58(31), 14410–14423. doi:10.1021/acs.iecr.9b01265
- Zhang, K., Shah, N. A., Alshehri, M., Alkarni, S., Wakif, A., & Eldin, S. M. (2023). Water thermal enhancement in a porous medium via a suspension of hybrid nanoparticles: MHD mixed convective Falkner’s-Skan flow case study. Case Studies in Thermal Engineering, 47, 103062. doi:10.1016/j.csite.2023.103062
- Zhang, X., Liu, X., Wang, X., Band, S. S., Bagherzadeh, S. A., Taherifar, S., Abdollahi, A., Bahrami, M., Karimipour, A., & Chau, K.-W. (2022). Energetic thermo-physical analysis of MLP-RBF feed-forward neural network compared with RLS Fuzzy to predict CuO/liquid paraffin mixture properties. Engineering Applications of Computational Fluid Mechanics, 16(1), 764–779. doi:10.1080/19942060.2022.2046167
- Zhao, T., Khan, M. I., & Chu, Y. (2023). Artificial neural networking (ANN) analysis for heat and entropy generation in flow of non-Newtonian fluid between two rotating disks. Mathematical Methods in the Applied Sciences, 46(3), 3012–3030. doi:10.1002/mma.7310
- Zuhra, S., Raja, M. A. Z., Shoaib, M., Khan, Z., Nisar, K. S., Islam, S., & Khan, I. (2022). Numerical analysis of Cattaneo–Christov heat flux model over magnetic couple stress Casson nanofluid flow by Lavenberg–Marquard backpropagated neural networks. Waves in Random and Complex Media, 1–28. https://doi.org/10.1080/17455030.2022.2062484