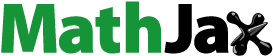
Abstract
The ventilation system significantly affects the energy saving, thermal comfort, and pollutant dispersion characteristics of high-speed trains. Using Computational Fluid Dynamics (CFD), the effects of combining side-wall air supply with side roof or ceiling air supply, on the performance of a high-speed train ventilation system is studied. The results show that the addition of side-wall air supply aids energy saving and emission reduction, resulting in an increase in energy utilisation coefficient of between 5% and 30%. The number of measurement points with wind speed less than 0.3 m/s increases by 3%∼9%, which can improve passenger comfort. Also, the concentration of pollutants can be reduced by 6%∼29%, and the diffusion distance along the length of the train can be reduced by 18%∼45%, which means more efficient ventilation and a shorter diffusion range of pollutants. However, it reduces temperature uniformity. The results of this study can be used for ventilation optimisation of high-speed trains, to reduce ventilation energy consumption, improve thermal comfort, and increase ventilation efficiency.
1. Introduction
In recent years, outbreaks of respiratory diseases, such as novel COVID-19 and influenza, have had a significantly negative impact on the economy, and on people’s health. As one of the most important means of transportation, high-speed trains have high passenger capacity, and employ well-developed transportation networks. The demand for high-speed trains is increasing, worldwide. However, these characteristics of high-speed trains also make it easy for respiratory diseases to spread among passengers and hence, rapidly across cities and countries. At the same time, considering carbon neutrality and sustainable development, the design of train ventilation systems, as part of a new era in public transportation, needs to consider energy-saving technology, technology to improve thermal comfort, and technology to improve air quality.
With economic and social development, and with the outbreaks of various respiratory diseases (SARS, H1N1, COVID-19, etc.) that have occurred in recent years, a large number of scholars have conducted a lot of research on the ventilation design of buildings and of public transportation, such as airplanes and high-speed trains (He et al., Citation2015; Liu et al., Citation2021; Zhao et al., Citation2021; Li et al., Citation2022a; Lu et al., Citation2023). Li et al. (Citation2022b) studied the diffusion and transmission of respiratory pollutants in great detail. It has also been concluded that poor ventilation aggravates the airborne transmission of respiratory diseases (Wei & Li, Citation2017; Li et al., Citation2022b). Studies have shown that different ventilation strategies can have a significant effect on the airborne capacity and transmission distance of viruses in confined spaces (Al-waked, Citation2010; Sun & Zhai, Citation2020; Shirzadi et al., Citation2022; Ho & Sandia, Citation2021; Rivas et al., Citation2022). Arjmandi et al. (Citation2022) used numerical simulation methods and multi-objective optimisation, to optimise the air supply opening size, air volume, and air supply temperature of classroom ventilation systems, in order to reduce the spread of respiratory pathogens, and improve thermal comfort. Xu et al. (Citation2022b) investigated the diffusion characteristics of cough pollutants in high-speed train compartments under four different ventilation modes, and showed that different ventilation modes have a significant impact on the diffusion trajectory and ventilation performance of pollutants. Qian et al. (Citation2009) combined the Wells-Riley (Riley et al., Citation1978) model with computational fluid dynamics, to predict the spatial distribution pattern of airborne infection risk, and investigated the effect of different ventilation schemes on the diffusion characteristics of the exhaled droplet nuclei of personnel on the ward. Hyeunguk et al. (Citation2018) concluded that different ventilation schemes simultaneously have a significant impact on air quality, thermal comfort, and energy performance in offices, due to uneven air mixing. The results of the study showed that proper ventilation design can be more energy efficient, and have higher ventilation efficiency. Aviv et al. (Citation2021) investigated a novel radiant cooling-assisted natural ventilation approach, to replace conventional air conditioning systems, a solution that could then reduce the risk of indoor transmission of infectious diseases, while significantly reducing the energy consumption of the building.
In summary, ventilation mode has a large impact on the flow field characteristics of an indoor environment. Currently, there are many studies on the flow field characteristics of aircraft, hospitals, and general indoor environments. However, there is still a lack of studies of trains, especially considering the combination of thermal comfort, energy consumption, and pollutant dispersion characteristics.
Based on computational fluid dynamics and tracer gas methods, this paper investigates the effects of combining side-wall air supply with either side roof air supply, or ceiling air supply, on the energy saving, thermal comfort, and respiratory pollutant dispersion characteristics of high-speed train ventilation, and evaluates the performance of the train ventilation system as a whole, using relevant evaluation indices.
2. Mathematical models
2.1. Turbulence model
The airflow in a train carriage is a low-speed turbulent flow, which can be regarded as incompressible flow. By solving the Reynolds-averaged Navier-Stokes (RANS) equations, the time-homogenized flow field inside the carriage can be obtained. In the calculation process, the energy equation is used to predict the heat transfer effect in the flow field, and gravitational and buoyancy effects are also considered.
In this paper, the Realisable k-ε turbulence model is used to solve the flow field in the compartment (Zhang et al., Citation2009; Cao et al., Citation2022; Li et al., Citation2022a), which relevant studies have verified to be reliable in predicting the flow field flow inside aircraft and train carriages. Also, the enhanced wall function was used (Cao et al., Citation2022; Duan et al., Citation2015). The governing equations for the turbulent kinetic energy k and turbulent dissipation rate ε are:
(1)
(1)
(2)
(2)
(3)
(3)
Where, uj is the velocity of the flow field in a direction; t is the physical time; ρ is the airflow density; p is the air pressure; k is the turbulent kinetic energy; ε is the turbulent dissipation rate; Gk represents the generation of turbulent kinetic energy due to the mean velocity gradients; Gb is the generation of turbulent kinetic energy due to buoyancy; YM represents the contribution of fluctuating dilatation incompressible turbulence to the overall dissipation rate; C2 and C1ε are constants; σk and σε are the turbulent Prandtl numbers for k and ε, respectively. Sk and Sε are user-defined source terms.
2.2. Pollutant dispersion model
In 1990, Sherman (Citation1990) proposed the tracer gas method, which uses tracers to predict the trajectory and distribution pattern of the object of study. Subsequently, scholars verified the feasibility of using the tracer gas method for pollutant prediction via both experimental (Bivolarova et al., Citation2017) and numerical simulation (Li et al., Citation2011) perspectives. Ai et al. (Citation2020) concluded that the use of CO2 and N2O as tracers, can be good proxies for human-exhaled gaseous pollutants.
Gaseous pollutants from passenger breathing have different gaseous properties than the ambient air in the cabin, such that they can be seen as different species. By solving the species transport equations, the mixing and transport between the species can be solved. The general governing equation, in its mathematical form, is:
(4)
(4) Where, u, v and w are the components of the velocity vector in the x, y and z directions, respectively; cs is the volume concentration of the species s; ρcs is the mass concentration of the species s; Ds is the diffusion coefficient of species s; Ss is the rate of creation by addition from the dispersed phase, plus any user-defined sources.
2.3. Evaluation indicators
In this study, a total of seven evaluation indices were used to evaluate the flow field characteristics inside the vehicle. The energy utilisation coefficient (ηT) is used to evaluate the energy efficiency of the ventilation, where a larger index indicates more energy-efficient ventilation; The wind speed index (Iws) and the temperature uniformity index (Itu) are used to evaluate thermal comfort, where a larger index indicates better thermal comfort, which would preferably be close to 100%; The non-uniformity coefficient (kx) is used to evaluate the uniformity and stability of the flow field, where a smaller index indicates a more uniform and less fluctuating flow field; Pollutant equivalent concentration (Ct) and ventilation efficiency (Ev) are used to evaluate the purification capacity of ventilation. The lower the concentration and the higher the efficiency, the higher the purification capacity of ventilation; Dimensionless pollutant dispersion distance (Dpx) is used to evaluate the dispersal capacity of pollutants along the length of the carriage, the smaller the index, the shorter the dispersion distance of pollutants. The specific definition of each indicator is described in the following.
While studying wind speed index, temperature uniformity index, and non-uniformity coefficient, it is necessary to set up several monitoring points in the vehicle, and extract relevant data, as calculation samples. 228 monitoring points are arranged at each of four heights along the carriage, with a total of 912 monitoring points being used. The specific layout is shown in Figure . According to the recommendations and requirements of EN14750 (EN14750-2, Citation2006), the four heights correspond to the passengers’ ankles (0.1 m), the passengers’ knees (0.6 m), the heads of sitting passengers (1.1 m), and near the heads of standing passengers (1.7 m).
2.3.1. Energy utilisation coefficient
The energy utilisation coefficient is a ventilation index that analyzes temperature, which reflects the effectiveness of energy use in achieving airflow distribution. The larger the coefficient, the more energy-efficient the air conditioner. The energy utilisation coefficient is defined as in (Krajčík et al., Citation2012; Zhang et al., Citation2019b):
(5)
(5) Where, ηT denotes the energy utilisation coefficient; Te is the indoor exhaust air temperature, °C; Ts is the indoor supply air temperature, °C;
is the average indoor temperature, °C.
2.3.2. Wind speed index
The Iws is defined as the ratio of two statistical values, the first being the number of all measurement points in the vehicle, and the second being the number of measurement points that satisfy a specific condition. According to the standard EN14750 (EN14750-2, Citation2006), most people will feel comfortable when v < 0.3 m/s. The wind speed index is defined as:
(6)
(6) Where, n1 denotes the total number of measurement points satisfying the condition v < 0.3 m/s; n denotes the total number of measurement points.
2.3.3. Temperature uniformity index
The Itu is defined as the ratio of two statistical values, the first being the number of all measurement points in the vehicle, and the second being the number of measurement points that satisfy a particular condition. This particular condition requires that the temperature difference between the measurement points should all be less than 3°C. This is because, according to the standard EN14750 (EN14750-2, Citation2006), most people are comfortable when the temperature difference of airflow in the cabin is within 3°C. The temperature uniformity index is defined as:
(7)
(7) Where, n2max indicates the maximum sum of measurement points that meet the condition that
, where Tm is the reference temperature, in °C; Ti is the temperature of the monitoring point, in °C; ΔTm is the increment of Tm.
2.3.4. Non-uniformity coefficient
The non-uniformity coefficient includes the velocity non-uniformity coefficient and temperature non-uniformity coefficient, which reflect the degree of variation of velocity and temperature, respectively, in the vehicle. The smaller the coefficient, the more uniform the flow field. The velocity non-uniformity coefficient is also related to the turbulence intensity of the flow field inside the vehicle. A smaller coefficient indicates a lower turbulence intensity. The standard deviation reflects the degree of temperature dispersion and velocity dispersion, respectively, in the data set. The non-uniformity coefficient and standard deviation are defined as:
(8)
(8)
(9)
(9)
(10)
(10)
Where, kx denotes the velocity non-uniform coefficient (kv), or the temperature non-uniform coefficient (kT); is the variable at the sampling point, which can denote the velocity or temperature at the measurement point; σx is the root mean square deviation of the variable, which is the mean value of the variable.
2.3.5. Pollutant equivalent concentration
In theory, increased ventilation can reduce the concentration of pollutants. However, simply increasing the airflow will not only increase the energy consumption of the air conditioner, but will also have a negative impact on the thermal comfort of the compartment. Also, the flow of exhaled pollutants differs for passengers with different physiological characteristics. Therefore, this paper does not directly use the concentration of a characterised pollutant, but rather an equivalent concentration that characterises the ability to remove the pollutant (Zhang et al., Citation2019a). The equivalent concentration of a pollutant is defined as:
(11)
(11)
(12)
(12)
(13)
(13)
Where, Ct indicates the equivalent concentration of pollutants; q is the volume flow rate of the source, in m3/s; Q is the volume flow rate of air conditioning and ventilation, in m3/s; xs is the location of the source (x, y, z); Cx(xs, x) is the concentration of pollutants in the corresponding space; Vs represents the space volume.
2.3.6. Ventilation efficiency
Ventilation efficiency is used to assess the purification capacity of the system. The greater the coefficient of ventilation efficiency, the greater the ability of the system to remove pollutants. The ventilation efficiency is defined as in (Chen et al., Citation2021; Yang et al., Citation2023):
(14)
(14) Where, Ev denotes the ventilation efficiency; Cp denotes the exhaust air concentration; Cs denotes the supply air concentration;
denotes the average indoor pollutant concentration.
2.3.7. Dimensionless pollutant dispersion distance
In addition to reducing the concentration of pollutants in the carriage, it is also desirable to reduce the diffusion distance of pollutants, by as much as possible. Since train carriages are long and narrow, it is preferable to reduce the diffusion distance of pollutants along the length of the carriage (as opposed to the width), and define it on a dimensionless scale, with the following equation:
(15)
(15) Where, Dpx denotes the dimensionless distance of pollutant dispersion along the length of the carriage; x(xs) is the x-coordinate where the source of pollution is located.
3. Computational model
3.1. Numerical model
The standard electric multiple units (EMU), which is a train type developed in China, is the subject of this study. A full-size carriage model is established, as shown in Figure . The length of the carriage is about 18300 mm, the height is about 2350 mm, and the width is about 3000 mm. The carriage is loaded to its capacity, with 90 people, 5 columns of seats are arranged along the width direction, and 18 rows of seats are arranged along the length direction. During the study, passengers in the ninth row were considered as research objects, and the respiratory pollutants produced specifically by them were analyzed.
Based on the typical layout of air vents, the air supply and exhaust air vents of the carriage are established. A ceiling air vent in the middle of the roof, with an air outlet size of 1000 mm × 18300 mm. Side roof air vents are located on both sides of the roof, each with an air outlet size of 40 mm × 18300 mm. Side-wall air vents are located under the luggage racks on both sides, each with air vents measuring 40 mm × 18300 mm. The exhaust air vent is located below the side-walls on both sides. The sizes of these air vents are both 150 mm × 18300 mm.
According to the different locations of air supply vents and flow distribution ratios, the effects of nine ventilation schemes, on the ventilation energy saving, thermal comfort, and respiratory pollutant dispersion characteristics of high-speed trains, are comparatively studied, as shown in Table . The calculation scheme includes three independent air supply schemes: side-wall air supply (scheme 1), side roof air supply (scheme 5), and ceiling air supply (scheme 9). Three mixed air supply schemes (scheme 2, scheme 3, and scheme 4) consisting of a combination of side-wall air supply and side roof air supply, with 25%, 50%, and 75% flow distribution of the side roof air supply, respectively, are considered, as well as three mixed air supply schemes (scheme 6, scheme 7 and scheme 8), consisting of a combination of side-wall and ceiling air supply with 25%, 50% and 75% of the flow distribution of the ceiling air supply, respectively.
Table 1. Ventilation scheme and ventilation parameters.
3.2. Boundary conditions
The numerical case study is carried out in Ansys Fluent, version 2021. The boundary condition of the air supply outlet is set to velocity-inlet, the total air supply flow rate is 1.33 m3/s, and the air supply temperature is 22°C. The boundary condition of the air exhaust outlet is set to outflow. The flow properties of the gas assume it to be incompressible.
In the calculation process, all passengers in the vehicle are considered to have an exhalation process, and continuously release respiratory pollutants into the vehicle. The exhalation process was simplified to a steady state, which is reliable when just studying the ventilation efficiency of the whole system (Qian et al., Citation2009; Li et al., Citation2022a), and the boundary conditions were set to velocity-inlet. According to the experimental results of Gupta et al. (Citation2010), the air velocity was set to 2.24 m/s, at an angle of 30 degrees to the vertical. CO2 was used as a tracer for passenger respiratory pollutants (Ai et al., Citation2020), with a 100% CO2 concentration, and with both human surface and exhaled gas temperature set to 32°C (Li et al., Citation2022a).
During train operation, the heat radiation effect of the sun will exchange heat between the surface of the carriage and the flow field inside and through the carriage, and affect the flow field. The outdoor ambient temperature when the train is in operation is considered to be 35°C, and the solar radiation intensity is 800 W/m2. Assuming that the sun is directly above the train, uneven solar radiation on the two sides of the train is ignored. Due to the different structural designs and material properties of each part of the train, the area-weighted method is used here, to calculate the convective heat transfer coefficient of the whole vehicle. Furthermore, the cold bridging phenomenon caused by the processing process, etc., is corrected using a correction factor. Here, the convective heat transfer coefficient of the carriage wall is set to 1.2 W/(m2·K), since this value can represent the properties of the wall material (Li et al., Citation2022b; Xu et al., Citation2022a, Citation2022b).
In solving the Navier-Stokes equations, the Realisable k-ε turbulence model and the enhanced wall function are used. The energy equation considers gravitational and thermal buoyancy effects. The SIMPLE algorithm solver is chosen, and the variables of momentum, energy, species, turbulent kinetic energy, and turbulent dissipation rate are in discrete format with second-order upwind, except for the pressure, which is by PRESTO! scheme. The energy residuals reach 10−6, the residuals of the remaining variables reach 10−3, and the flow field variables in the vehicle are considered to be converged after gradual stabilisation, with iteration steps.
3.3. Mesh independence
The computational model was meshed using the commercial software Fluent Meshing version 2021, with the mesh type Poly-Hexcore. To eliminate the influence of grid-scale size on the calculation results, three sets of grid models of different scales are devised. Figure shows the carriage cross-sections of grid models of different scales. The maximum sizes of the three sets of body grids are 32, 28, and 20 mm, giving body grid numbers of about 12.63, 16.8, and 21.62 million, respectively.
Figure 3. Carriage cross-sectional grid (a. the body grid is 32 mm; b. the body grid is 28 mm; c. the body grid is 20 mm).

Four vertical lines were selected as datum lines (x = 0 m, x = 2 m, x = 4 m, x = 6 m) on the centre surface of the compartment corridor, as shown in Figure . The velocity and temperature on the datum lines were selected as the test of grid independence, and the calculation results are shown in Figure . Furthermore, the relative error () is defined in Equations 16 and 17.
(16)
(16)
(17)
(17) Where,
indicates the relative error of the medium grid or coarse grid, relative to the fine grid at measurement point i-j;
indicates the simulation data of the medium or coarse grid at measurement point i-j;
indicates the simulation data of the fine grid at measurement point i-j;
indicates the average relative error of all measurement points; i denotes the number of the monitoring line, the total number being 4; j denotes the number of measurement points on each monitoring line, the total number of which is ns.
The results in Figure show that the average relative errors between the medium and fine grids, for air temperature and wind speed are 0.48% and 5.60%, respectively. The relative error of the coarse grid is about 5 times higher than that of the medium grid. Thus, the coarse grid increases the resolution error in the chamber flow field. Based on the comparison of the three grids, the fine and medium grids were able to produce grid-independent CFD results. To save computational time and ensure simulation accuracy, the grid parameters used in the medium grid were used for flow field discretization.
The specific division dimensions of the second set of grids are as follows: The maximum grid size of the passenger nostril area is 0.5 mm, and the maximum grid sizes for air vents, passenger, and cabin surfaces are 5, 20, and 30 mm, respectively, as shown in Figure . In addition, the maximum body mesh is 28mm, and the major y + of the model is less than 10 (Cao et al., Citation2022).
4. Numerical method validation
4.1. Validation of gaseous pollutant dispersion
To ensure the accuracy of the method for numerically predicting the dispersion of gaseous pollutants, the experiment of Zhang and Chen (Citation2006) is chosen, here, for numerical validation. The experimental environment in that study, is a room with dimensions of 4800 × 2440 × 4200 mm (x × y × z), as shown in Figure . The room is ventilated through air supply vents on the floor, with a total supply air flow rate of 0.0944 m3/s. In addition, the room is equipped with six lamps and four simplified manikins, for heating. More detailed information can be obtained from the study. During the experiment, particles with an average diameter of 0.7 µm were continuously injected into the room.
In the numerical calculations, CO2 was used as a particle tracer. Figure shows the comparison between the norm velocity and dimensionless temperature obtained from the numerical simulation, with the experimental data. Figure shows the comparison between the dimensionless concentration obtained from the numerical simulation, and the experimental data. Overall, the numerical simulation can predict the experimental data well, except that the randomness of the flow field may introduce some errors (Zhang & Chen, Citation2006). Therefore, the numerical method in that study can predict the flow field characteristics and the diffusion characteristics of gaseous pollutants in a confined space.
4.2. Validation of airflow field
To further validate the accuracy of the current numerical method for predicting the carriage flow field, the experimental data of Xu et al. (Citation2022b) is chosen. Defining the train height as the characteristic length, H = 2240 mm, the length and width of the experimental model in the study are about 2.25 and 1.16 H. The specific carriage model, the layout of the air vents, and the layout of the monitoring points are shown in Figure . The experiments were conducted with full occupancy, and the total air flow of the carriage was 0.27 m3/s. More information can be obtained from the literature (Xu et al., Citation2022b).
Figure 10. Experimental carriage model and monitoring points (a. cabin model and air vent layout; b. monitoring points layout of top view; c. monitoring points layout of side view).

The experimental values of velocity and temperature at the monitoring points were compared, and the calculated results are shown in Figure . Overall, the velocities and temperatures of the monitoring points predicted by the simulation agree well with the experimental measurements. The slight deviations at some monitoring points may be due to positioning errors of the human bodies and sensors, during the experiments (Xu et al., Citation2022b). Based on the above analysis, it can be concluded that the numerical method used in that study can predict the flow field in the train car, well.
5. Results
By solving the Reynolds Average Navier-Stokes (RANS), information on the physical quantities of the flow field in the train were obtained. The results were analyzed, looking at five aspects: flow field characteristics, energy utilisation coefficient, thermal comfort, flow field uniformity, and respiratory pollutant dispersion characteristics in the cabin.
5.1. Flow field characteristics
To analyze the flow field inside the vehicle in more detail, three vertical planes, and one longitudinal plane were created. Figure shows the detailed locations and names of the individual cross-sections, where each vertical plane is located 0.1 m in front of the nearest passenger, while the longitudinal plane is located in the plane of symmetry of the passenger in column A.
Figure shows the velocity flow field distribution in a vertical section inside the train, which is intercepted in the cross-section of the car (Plane 3, x = 0.5 m). The results are analyzed in terms of four aspects: From the initial flow direction of airflow, vortex distribution of flow field, velocity magnitude, and airflow impact location.
As shown in Figure , when airflow enters the carriage from the side roof air supply and side-wall air supply, it spreads horizontally by adhering to the ceiling surface and the lower surface of the luggage rack, respectively, and forms a separation point after the airflow collision at the middle position of the carriage, after which, it spreads downward. This phenomenon is more common in adherent jets, and is called the Coanda effect. When the airflow is sent into the car from the ceiling air supply, it tends to diffuse directly downward, under gravity. The airflow diffusing downward from the top of the compartment causes the surrounding airflow to converge toward the top, a phenomenon known as the entrainment effect. As an example, this phenomenon can be observed in scheme 5 and scheme 9, as shown in the black dashed circle in Figure , and diminishes as the top air supply flow rate decreases.
From the vortex distribution of the flow field, we can see that the airflow under different ventilation schemes will flow into the corridor of the compartment, and the airflow in the passenger area will easily spread upward, under the action of the thermal plume, thus forming a large airflow vortex on each side of the corridor. As high-speed trains generally adopt a 3 + 2 seating layout scheme, the vortices on opposite sides tend to be asymmetrical.
From the velocity distribution of the flow field, we can see that the relatively large air velocity in the compartment is concentrated in three areas, being the air supply area, the corridor area, and the area near the floor. Overall, the airflow velocity is relatively lower when using a ceiling air supply in combination with a side-wall air supply. This is due to the larger air vent area and more uniform airflow distribution when ceiling air is used.
Due to the asymmetrical seating layout of the train, the space structure on both sides of the carriage is different, and the intensity of the heat plume is different. The flow field in the carriage is asymmetric, and the direction of airflow in the carriage is varied. From the black dashed box in the figure, it can be seen that the airflow impact location is mainly concentrated in the passenger and corridor areas of column C. Taking scheme 1, scheme 5, and scheme 9 as examples; in scheme 1, the airflow impact location is mainly in the passenger area of column C; with scheme 5, the airflow impact location is mainly in the corridor area; with scheme 9, the airflow impact location includes both the corridor area and the passenger area of column C.
5.2. Energy utilisation coefficient
The average temperature in the carriage can reflect the cooling capacity of the ventilation scheme. The lower the temperature, the stronger the temperature reduction capacity. Moreover, the larger the energy utilisation coefficient, the more conducive the scheme is to saving energy in ventilation.
Figures and show the average temperature and ventilation energy utilisation coefficients inside the vehicle, for different ventilation schemes. It can be seen that the average temperature inside the vehicle is 25.06°C, 25.95°C and 25.54°C for the three independent air supply cases of side-wall air supply (scheme 1), side roof air supply (scheme 5), and ceiling air supply (scheme 9), respectively. The energy utilisation coefficients are 1.18, 0.90 and 0.98, respectively. Overall, the energy-saving effect is relatively the worst when a side roof air supply is used.
With side roof air supply, the average temperature inside the vehicle decreases by 0.99°C, 0.84°C, and 0.31°C, respectively when combined with side-wall air supply (scheme 2, scheme 3, and scheme 4), and the energy utilisation coefficients increase by 0.27, 0.22 and 0.05, respectively. When using a combination of ceiling and side-wall air supply (scheme 6, scheme 7 and scheme 8), the average temperature inside the vehicle decreases by 0.62°C, 0.60°C and 0.54°C, respectively, and the energy utilisation coefficients increase by 0.20, 0.19, and 0.20, respectively. Overall, when using a mixed air supply, the average temperature inside the vehicle can be effectively reduced, and the energy utilisation efficiency of ventilation can be improved.
Taking three independent air supplies (scheme 1, scheme 5, and scheme 9) and three mixed air supplies (scheme 3, scheme 4, and scheme 8) as examples, Figure shows the temperature field distribution on the cross-section and longitudinal section of the carriage, intercepted at x = −4.4 m, x = 0.5 m, x = 5.4 m and z = −1.2 m, respectively. Overall, the airflow temperature in the passenger area is relatively high, due to heat dissipation from the human body surface, and heat dissipation from exhaled airflow, while the airflow temperature in the corridor area is relatively low, due to the direct influence of fresh airflow. Compared to only side roof air supply (scheme 5), and only ceiling air supply (scheme 9), the temperature in the passenger area is significantly cooler when using a mixed air supply, meaning when combined with the side-wall air supply (shown in the black circle area in Figure ). This indicates that the airflow is more effective in cooling the passenger area when combined with the side-wall air supply, and is, therefore, more conducive to ventilation and energy-saving.
Figure 16. Temperature field distribution in the cross-section and longitudinal section of the carriage.

It can also be seen in Figure , that the temperature field along the length of the carriage does not exhibit complete uniformity, with varying degrees of similarity between the cross-sections. In scheme 1, for example, the temperature at the C1 circle is significantly higher than at the C2 circle. This non-uniform characteristic of the flow field will be described in detail, in section 5.4.
5.3. Breeze velocity and thermal comfort
To study the breeziness of the vehicle under different ventilation schemes, we have arranged several measurement points in the vehicle. According to the requirements from EN14750 (EN14750-2, Citation2006), 228 monitoring points at a height of 1.1 m from the floor, and all monitoring points inside the vehicle were selected as data sample points for analysis, using 0.3 m/s as the velocity threshold. Figure shows the percentage of airflow velocity in the compartment that were less than 0.3 m/s, for each of these two cases. For the monitoring points at 1.1 m height, the percentages of velocity values below 0.3 m/s were 82%, 84%, and 97%, when independent air supply schemes (scheme 1, scheme 5, and scheme 9, repectively), were used. The percentage of airflow velocity below 0.3 m/s increases by 5%, 12%, and 9%, respectively, when using the combination of side roof with side-wall air supply schemes (scheme 2, scheme 3, and scheme 4, respectively). The percentage of velocity values below 0.3 m/s is 90%, 98%, and 100%, when using a combination of ceiling and side-wall air supply (scheme 6, scheme 7, and scheme 8, respectively).
Figure 17. Percentage of velocity below 0.3 m/s for different ventilation schemes. (a) 1.1 m height measurement points. (b) All measurement points in the carriage.

For all measurement points in the vehicle, the percentages of velocity values below 0.3 m/s were 92%, 90%, and 99%,when independent air supply schemes (scheme 1, scheme 5, and scheme 9, respectively,), were used. With side roof air supply, the percentage of airflow velocity below 0.3 m/s increases by 3%, 9%, and 5%, when using combinations with side-wall air supply (scheme 2, scheme 3, and scheme 4, respectively). The percentages of velocity values below 0.3 m/s were 93%, 99%, and 100%, when combinations of ceiling air supply and side-wall air supply was used (scheme 6, scheme 7, and scheme 8, respectively). Overall, the breeze velocity in the compartment can be improved significantly when any combination of ceiling or side roof, with side-wall air supply is used. The in-vehicle breeze velocity is better when the ceiling air supply is used, than when the side roof air supply is used.
To specifically study the distribution characteristics of the wind speed inside the vehicle, three independent air supplies (scheme 1, scheme 5, and scheme 9), and three mixed air supplies (scheme 3, scheme 4, and scheme 8), are used as examples. Figure shows the velocity distribution regularity of all measurement points in the vehicle, which are spread across all cross-sections of the cabin, as shown in Figure , in Section 2.3. Furthermore, the blue horizontal line in Figure represents the dividing line of v = 0.3 m/s. At 0.6, 1.1, and 1.7 m heights, relatively large airflow velocities are present in the passenger area of column C, and in the corridor area. From the perspective of the flow field, this is because the airflow of the air supply enters the passenger area, and tends to converge in the middle of the passenger area. From the perspective of the car layout, it is because the train has an asymmetric layout scheme of 3 + 2, resulting in a large airflow velocity in column C. Conversely, at 0.1 m height, there is a relatively small airflow velocity in column C and in the corridor area, and a relatively large airflow velocity on both sides. The relatively small velocity is due to the flow separation of the airflow here, while the relatively large velocity is influenced by the exhaust port on both sides, and by the velocity of the airflow moving toward the ground.
Taking the side roof air supply (scheme 5), with relatively poor breeziness, as an example, the regions with velocity values exceeding v = 0.3 m/s are mainly found in the regions of columns D and F at 0.1 m height, and in the regions of column C and the corridor at 0.6 m height and 1.1 m height, respectively. When using a combination of side roof and side-wall air supply, the airflow velocity in these areas also gradually decreases, and the overall breeziness of the vehicle gradually increases.
To study the temperature uniformity inside the train, the number of measurement points with a relative temperature difference of 3°C or less, as a percentage of the total number of monitoring points, is counted here. The ‘relative temperature difference’ refers to the temperature differences between each of the monitoring points in the analysis. Here, the monitoring point at a height of 1.1 m, and all the monitoring points in the train are used for analysis, respectively, as shown in Figure . The layout of the monitoring points is shown in Figure , in section 3.2. For the measurement points at 1.1 m height, the percentages of temperature differences within 3°C were 82%, 98%, and 93% with independent air supply schemes (scheme 1, scheme 5, and scheme 9, respectively). With the side roof air supply, the percentage of temperature difference within 3°C decreases by 4%, 13%, and 3%, when using a combination with side-wall air supply (scheme 2, scheme 3, and scheme 4, respectively). For ceiling air supply, the percentage of temperature difference within 3°C decreases by 4%, 0%, and 3%, when using a combination with side-wall air supply (scheme 6, scheme 7, and scheme 8, respectively).
Figure 19. Percentage of temperature difference within 3°C for different ventilation schemes. (a) 1.1 m height measurement points. (b) All measurement points in the carriage.

For all measurement points in the vehicle, the percentages of temperature differences within 3°C were 84%, 94%, and 88%, when independent air supply was used, (scheme 1, scheme 5, and scheme 9, respectively). For side roof air supply, the percentage of temperature difference within 3°C decreases by 8%, 13%, and 1%, when using a combination with side-wall air supply (scheme 2, scheme 3, and scheme 4, respectively). For ceiling air supply, the percentage of temperature difference within 3°C decreases by 5%, 6%, and 4%, when using a combination with side-wall air supply (scheme 6, scheme 7, and scheme 8, respectively). Unlike breeziness, the temperature uniformity inside the vehicle performs better when a side roof air supply is used. However, when combined with the side-wall air supply, it tends to reduce the temperature uniformity of the flow field inside the vehicle.
To specifically study the distribution characteristics of the temperature inside the vehicle, Figure shows the temperature distribution regularity of all measurement points inside the vehicle, which are spread across all cross-sections of the cabin, as shown in Figure , in Section 2.3. The two blue horizontal lines in Figure represent the threshold dividing line, within 3°C of the temperature difference. As can be seen from the figure, there are a total of three areas prone to large temperature differences, including a high-temperature area and two low-temperature areas. The high-temperature region appears in column A at 0.6 m height; the low-temperature regions appear in column C and the corridor region at 1.1 m height, and in column C and the corridor region, in the 1.7 m height region.
Comparing scheme 3 and scheme 5, scheme 8 and scheme 9 in Figure , respectively, it can be seen that when using a combination of ceiling or side roof, with side-wall air supply, the temperature in the two low-temperature regions mentioned above is further reduced, thus worsening the temperature non-uniformity inside the train. Therefore, there is room for further optimisation regarding the side-wall air supply scheme. It would also be useful to attempt to increase the temperature in the column C area, and thus improve the overall temperature uniformity in the vehicle.
5.4. Flow and temperature field uniformity
The carriages of high-speed trains are narrow and long, and it can be found in Figure that the temperature field along the length of the train does not appear to be completely uniform. The large variability of the flow field not only affects the thermal comfort inside the vehicle, but more importantly, increases the challenge of predicting, analyzing, and optimising the flow field. The reason for this is that the flow field varies greatly between individual sections, making it difficult to select a representative section for analysis.
To analyze the similar characteristics of the flow field along the length of the car, three independent air supplies (scheme 1, scheme 5, and scheme 9) and three mixed air supplies (scheme 3, scheme 4, and scheme 8) are used as examples. Figure illustrates the velocity field distribution pattern inside the vehicle. From the figure, it can be seen that the flow field in the three circles in the figure has a large variability when using scheme 1 and scheme 3, especially the diffusion direction of the air supply airflow after entering the compartment. The flow fields in each cross-section are somewhat more similar when the other schemes, scheme 4, 5, and 8 are used, and this phenomenon is similar to the temperature field distribution pattern in Figure .
To quantify and analyze the similar properties of the flow field along the length of the carriage, a constraint is added, here, to the calculation method of the non-uniform coefficients. Firstly, the measurement points are divided into 48 groups, and the coordinates of each measurement point in the same group are the same along both the height direction and width direction of the compartment. They are also equally spaced along the length direction. Then, the non-uniform coefficients of each dataset are calculated, and the average value is taken as the final non-uniform coefficient. The calculation results are shown in Figure .
Figure 22. Non-uniform coefficient along the length of the carriage. (a) Velocity non-uniformity coefficient. (b) Temperature non-uniformity coefficient.

From Figure (a), it can be seen that the velocity non-uniformity coefficient does not accurately reflect the similarity or difference of the flow field. Taking scheme 1, scheme 3, and scheme 5 as examples, it can be shown from the flow field perspective, that the flow field similarity of scheme 5 is better, but that the reflected velocity non-uniformity coefficient is larger. On the other hand, the velocity non-uniformity coefficients of scheme 1 and scheme 3 are smaller. It is shown in Figure (b) that the temperature non-uniformity coefficient can reflect the variability of the flow field quite well. This is due to the relatively small airflow velocity in the vehicle, with the average velocity being between 0.13 and 0.18 m/s, under different ventilation schemes, and as this is the denominator for calculating the velocity non-uniformity coefficient, small changes can have a large impact on the results. The average temperature, also used as the denominator of the calculated temperature non-uniformity factor, is mainly distributed between 25.0°C and 25.6°C, which does not affect the calculation results. Therefore, the use of temperature non-uniformity coefficients can accurately reflect the similarity of the flow field along the length of the train. The smaller the coefficient, the better the similarity of the flow field. Looking at Figures and , it is shown that the temperature non-uniformity coefficients are relatively smaller, and the flow and temperature fields along the length of the train are more similar, when using scheme 5 and scheme 8. When scheme 1 is used, the temperature non-uniformity coefficient is relatively large, and the flow field along the length of the train is relatively more varied, and unstable. Furthermore, the flow field non-similarity under scheme 1 is similar to the phenomenon found in the study of Cao et al. (Citation2014). The reasons for this phenomenon are complex. On the one hand, it may be related to the shape of the train. For example, in scheme 1, when two jets collide, the surrounding space is more open and not constrained by walls, which may increase the possibility of the jets flowing in more directions after the collision. On the other hand, it may also be influenced by the Archimedes number (Ar), which is defined as the ratio of the Grashof number (Gr) to the square of the Reynolds number (Re) (Cao et al., Citation2014), where Gr characterises the strength of thermal buoyancy.
5.5. Pollutant dispersion characteristics
For indoor pollutants, we want to reduce both the average concentration of pollutants, and the dispersion distance of pollutants, as much as possible. Therefore, here we analyze the average concentration of pollutants, and the dispersion distance, separately.
Figures and show the average concentration of pollutants and ventilation efficiency in the vehicle, under different ventilation schemes, which can visually reflect the elimination ability of each ventilation system for respiratory pollutants. It is shown that the average concentration of pollutants in the vehicle is 1.16, 1.40, and 1.40 when side-wall air supply (scheme 1), side roof air supply (scheme 5), and ceiling air supply (scheme 9) are used, respectively. The ventilation efficiency is 0.89, 0.69 and 0.71, respectively. Considering that the similarity of the flow field along the length of the carriage is poor when the side-wall air supply is used, the respiratory pollution of the 9th row of passengers is not selected to be sufficiently representative for analysis, and so is not analyzed in detail.
Based on the pollutant concentration Ct = 1, the average in-vehicle pollutant concentration decreases by 17%, 29%, and 6%, respectively, and the ventilation efficiency increases by 0.11, 0.19, and 0.05, respectively, when using a combination of side-wall air and side roof air supply (scheme 2, scheme 3 and scheme 4). With ceiling air supply, combined with side-wall air supply (scheme 6, scheme 7, and scheme 8), the average concentration of pollutants in the vehicle decreased by 24%, 16%, and 32%, respectively, and the ventilation efficiency increased by 0.13, 0.06 and 0.30, respectively. Based on Figures and , it is shown that the combination with the side-wall air supply can effectively reduce the concentration of pollutants in the train, and improve the ventilation efficiency of the air conditioner. The reason for this is that the airflow from the side-wall air vents can reach the passenger area more efficiently, which is more conducive to contaminant removal. This is similar to the reason described in section 5.3, that adding side-wall air supply can improve the energy utilisation coefficients.
Figure shows the perspective view of the respiratory pollutants of the 9th-row passengers in the compartment, analyzed in terms of the near diffusion characteristics and long-range diffusion characteristics of the pollutants, respectively. When analyzing the near dispersion characteristics of pollutants, it was found that the concentration of pollutants in row 9 was relatively the largest, followed by rows 8 and 10, while the concentration of pollutants in the rest of the area was significantly lower, as shown in the circled area. Analysis from the perspective of long-range dispersion characteristics reveals that the pollutants disperse relatively the farthest when scheme 5 and scheme 9 are used, as shown in the C2 region of the figure, followed by scheme 4 and scheme 8, as shown in the C1 region of the figure. As the flow rate of the side and ceiling air vents decreases, the diffusion distance of pollutants along the length of the compartment becomes shorter. From the perspective of the flow field (Figure ), it is known that the respiratory pollutants are subjected to the entrainment effect of the supply airflow, and diffuse above the compartment, away from the exhaust air outlet, thus increasing the diffusion distance in the passenger compartment.
Due to the narrow shape of the trains, we prefer to control the dispersion distance of pollutants along the length of the trains. Table shows the dimensionless pollutant dispersion distance (Dpx) along the length of the compartment, for different ventilation schemes. It is shown that Dpx is 0.9851 and 0.8155 when side roof air supply (scheme 5) and ceiling air supply (scheme 9) are used, respectively. Dpx is 0.6507, 0.5413, and 0.7716 when using a combination of side-wall air supply and side roof air supply (scheme 2, scheme 3, and scheme 4, respectively), decreasing by 33.95%, 45.05%, and 21.67%, respectively, compared to scheme 5. The Dpx is 0.5760, 0.6285, and 0.6606 when using a combination of side-wall air supply and ceiling air supply (scheme 6, scheme 7, and scheme 8, respectively), decreasing by 29.37%, 22.93%, and 18.99%, respectively, compared to scheme 9.
Table 2. Dpx and its reduction rate relative to independent air supply (schemes 5 and 9).
6. Conclusion
In this study, a computational model of the airflow of a standard EMU was established. The effects of combining side-wall air supply with side roof air supply, or ceiling air supply, on the energy efficiency, thermal comfort, flow field similarity, and respiratory pollutant dispersion characteristics of air conditioning ventilation in high-speed trains, were investigated and evaluated using relevant indices. The following main conclusions were drawn from the study:
As trains generally adopt a 3 + 2 seating layout, the airflow entering the passenger compartment is likely to have a direct impact on the column C area, and the corridor area, and form airflow vortices of different strengths on each of the two sides. When side roof air supply, or ceiling air supply are used, the airflow tends to spread above the compartment, under the effect of entrainment.
By adding a side-wall air supply scheme, and adjusting the flow ratio between air supply outlets, the energy utilisation efficiency of ventilation can be effectively improved, and the effect of energy saving in ventilation can be achieved. Under the same conditions of air supply flow and temperature, the temperature inside the compartment can be reduced by 0.31°C∼0.99°C, and the energy utilisation coefficient can be increased by 5%∼30%.
Combination with side-wall air supply can effectively improve the air speed index inside the carriage, but it is not conducive to improving the temperature uniformity index inside the carriage. By adjusting the flow ratio between air vents, the number of measurement points with wind speed less than 0.3 m/s can be increased by 3%∼9%, while the maximum number of measurement points with temperature difference within 3°C may be decreased by 1%∼13%.
The similarity of the flow field along the length of the compartment can be effectively described by using a constrained temperature non-uniformity coefficient. It can be shown that the similarity of the flow field is relatively poor when the side-wall ventilation scheme is used.
Combining the side-wall air supply solution with other air supply sources, not only reduces the average concentration of pollutants in the car, but also effectively reduces the diffusion distance of pollutants along the length of the car. By adjusting the flow ratio between air vents, the pollutant concentration can be reduced by between 6% and 29%, and the diffusion distance can be reduced by between 18% and 45%.
The results show that the addition of side-wall air supply will only adversely affect the temperature uniformity of the train, so this solution can be improved on this front, by optimising the flow distribution ratio, and the exit angle of the side-wall air supply vents, and the shape of the luggage rack, etc. These research results can provide some reference for design of the new generation of high-speed train ventilation systems. Furthermore, the internal flow field of high-speed trains is a complex system, influenced by multiple parameters and constrained by multiple objectives. The individual parameters for certain models can be further adjusted, utilising multi-objective optimisation techniques, targeted air supply optimisation, and so on, based on each evaluation index.
Acknowledgements
This work was supported by National Natural Science Foundation of China (No. 12372049), Science and Technology Program of China National Accreditation Service for Conformity Assessment (No. 2022CNAS15), the Fundamental Research Funds for the Central Universities (No. 2682021ZTPY124) and Independent Project of State Key Laboratory of Rail Transit Vehicle System (No. 2023TPL-T06).
Disclosure statement
No potential conflict of interest was reported by the author(s).
Additional information
Funding
References
- Ai, Z. T., Mak, C. M., Gao, N., & Niu, J. (2020). Tracer gas is a suitable surrogate of exhaled droplet nuclei for studying airborne transmission in the built environment. Building Simulation, 13(3), 489–496. https://doi.org/10.1007/s12273-020-0614-5
- Al-waked, R. (2010). Effect of ventilation strategies on infection control inside operating theatres. Engineering Applications of Computational Fluid Mechanics, 4(1), 1–16. https://doi.org/10.1080/19942060.2010.11015295
- Arjmandi, H., Amini, R., Khani, F., & Fallahpour, M. (2022). Minimizing the respiratory pathogen transmission: Numerical study and multi-objective optimization of ventilation systems in a classroom. Thermal Science and Engineering Progress, 28, 101052. https://doi.org/10.1016/j.tsep.2021.101052
- Aviv, D., Chen, K. W., Teitelbaum, E., Sheppard, D., Pantelic, J., Rysanek, A., & Meggers, F. (2021). A fresh (air) look at ventilation for COVID-19: Estimating the global energy savings potential of coupling natural ventilation with novel radiant cooling strategies. Applied Energy, 292, 116848. https://doi.org/10.1016/j.apenergy.2021.116848
- Bivolarova, M., Ondracek, J., Melikov, A., & Ždímal, V. (2017). A comparison between tracer gas and aerosol particles distribution indoors: The impact of ventilation rate, interaction of airflows, and presence of objects. Indoor Air, 27(6), 1201–1212. https://doi.org/10.1111/ina.12388
- Cao, Q., Liu, M. X., Li, X. Y., Lin, C.-H., Wei, D., Ji, S., Zhang, T., & Chen, Q. (2022). Influencing factors in the simulation of airflow and particle transportation in aircraft cabins by CFD. Building and Environment, 207, 108413. https://doi.org/10.1016/j.buildenv.2021.108413
- Cao, X. D., Liu, J. J., Pei, J. J., Zhang, Y., Li, J., & Zhu, X. (2014). 2D-PIV measurement of aircraft cabin air distribution with a high spatial resolution. Building and Environment, 82, 9–19. https://doi.org/10.1016/j.buildenv.2014.07.027
- Chen, Z., Aganovic, A., Cao, G. Y., & Bu, Z. (2021). Experimental and simulated evaluations of airborne contaminant exposure in a room with a modified localized laminar airflow system. Environmental Science and Pollution Research, 28(24), 30642–30663. https://doi.org/10.1007/s11356-021-12685-4
- Duan, R., Liu, W., Xu, L. Y., Huang, Y., Shen, X., Lin, C.-H., Liu, J., Chen, Q., & Sasanapuri, B. (2015). Mesh type and number for the CFD simulations of air distribution in an aircraft cabin. Numerical Heat Transfer Part B-Fundamentals, 67(6), 489–506. https://doi.org/10.1080/10407790.2014.985991
- EN14750-2. (2006). Railway applications-air conditioning for urban and suburban rolling stock-part 1: comfort parameters.
- Gupta, J. K., Lin, C. H., & Chen, Q. (2010). Characterizing exhaled airflow from breathing and talking. Indoor Air, 20(1), 31–39. https://doi.org/10.1111/j.1600-0668.2009.00623.x
- He, K. S., Chen, D. Y., Sun, L. J., Liu, Z.-l., & Huang, Z.-y. (2015). The effect of vent openings on the microclimate inside multi-span greenhouses during summer and winter seasons. Engineering Applications of Computational Fluid Mechanics, 9(1), 399–410. https://doi.org/10.1080/19942060.2015.1061553
- Ho, C. K., & Sandia, N. L. (2021). Modelling airborne transmission and ventilation impacts of a covid-19 outbreak in a restaurant in Guangzhou, China. International Journal of Computational Fluid Dynamics, 35(9), 708–726. https://doi.org/10.1080/10618562.2021.1910678
- Hyeunguk, A., Donghyun, R. L., & James, L. (2018). Ventilation and energy performance of partitioned indoor spaces under mixing and displacement ventilation. Building Simulation, 11(3), 561–574. https://doi.org/10.1007/s12273-017-0410-z
- Krajčík, M., Simone, A., & Olesen, B. W. (2012). Air distribution and ventilation effectiveness in an occupied room heated by warm air. Energy and Buildings, 55, 94–101. https://doi.org/10.1016/j.enbuild.2012.08.015
- Li, T., Wu, S. B., Yi, C., Zhang, J., & Zhang, W. (2022a). Diffusion characteristics and risk assessment of respiratory pollutants in high-speed train carriages. Journal of Wind Engineering and Industrial Aerodynamics, 222, 104930. https://doi.org/10.1016/j.jweia.2022.104930
- Li, X. P., Niu, J. L., & Gao, N. P. (2011). Spatial distribution of human respiratory droplet residuals and exposure risk for the co-occupant under different ventilation methods. HVAC&R Research, 17(4), 432–445.
- Li, Y. G., Cheng, P., & Jia, W. (2022b). Poor ventilation worsens short-range airborne transmission of respiratory infection. Indoor Air, 32(1), e12946. https://doi.org/10.1111/ina.12946.
- Liu, H., He, S. D., Shen, L., & Hong, J. R. (2021). Simulation-based study of COVID-19 outbreak associated with air-conditioning in a restaurant. Physics of Fluids, 33(2), 023301. https://doi.org/10.1063/5.0040188
- Lu, Y. B., Wang, T. T., Zhao, C. L., Zhu, Y., Jia, X. P., Zhang, L., Shi, F. C., & Jiang, C. (2023). An efficient design method of indoor ventilation parameters for high-speed trains using improved proper orthogonal decomposition reconstruction. Journal of Building Engineering, 71, 106600. http://dx.doi.org/10.1016/j.jobe.2023.106600
- Qian, H., Li, Y., Nielsen, P. V., & Huang, X. (2009). Spatial distribution of infection risk of SARS transmission in a hospital ward. Building and Environment, 44(8), 1651–1658. https://doi.org/10.1016/j.buildenv.2008.11.002
- Riley, E. C., Murphy, G., & Riley, R. L. (1978). Airborne spread of measles in a suburban elementary school. American Journal of Epidemiology, 107(5), 421–432. https://doi.org/10.1093/oxfordjournals.aje.a112560
- Rivas, E., Santiago, J. L., Martin, F., & Martilli, A. (2022). Impact of natural ventilation on exposure to SARS-CoV 2 in indoor/semi-indoor terraces using CO2 concentrations as a proxy. Journal of Building Engineering, 46, 103725. https://doi.org/10.1016/j.jobe.2021.103725
- Sherman, M. H. (1990). Tracer-gas techniques for measuring ventilation in a single zone. Building and Environment, 25(4), 365–374. https://doi.org/10.1016/0360-1323(90)90010-O
- Shirzadi, M., Tominaga, Y., & Mirzaei, P. A. (2022). CFD modeling of airborne pathogen transmission of COVID-19 in confined spaces under different ventilation strategies. Sustainable Cities and Society, 76, 103397. https://doi.org/10.1016/j.scs.2021.103397
- Sun, C. J., & Zhai, Z. Q. (2020). The efficacy of social distance and ventilation effectiveness in preventing COVID-19 transmission. Sustainable Cities and Society, 62, 102390. https://doi.org/10.1016/j.scs.2020.102390
- Wei, J. J., & Li, Y. G. (2017). Human cough as a two-stage jet and its role in particle transport. European Physical Journal C, 12(1), e0169235. https://doi.org/10.1371/journal.pone.0169235.
- Xu, R. Z., Qian, B., Wu, F., Li, X., Zhang, G., & Wang, L. (2022a). Dispersion of evaporating droplets in the passenger compartment of high-speed train. Journal of Building Engineering, 48, 104001. https://doi.org/10.1016/j.jobe.2022.104001
- Xu, R. Z., Wu, F., Li, X. L., Yu, C., Li, H., Wu, R., & Wu, Y. (2022b). Numerical comparison of ventilation modes on the transmission of coughing droplets in a train compartment. Journal of Wind Engineering and Industrial Aerodynamics, 231, 105240. https://doi.org/10.1016/j.jweia.2022.105240
- Yang, Y. W., Yang, H. T., Li, Q. F., Zhang, L., & Dong, Z. (2023). Simulation study on airflow organization and environment in reconstructed fangcang shelter hospital based on CFD. Buildings, 13(5), 1269. https://doi.org/10.3390/buildings13051269
- Zhang, D. D., Cai, Y., Liu, D., Zhao, F.-Y., & Li, Y. (2019a). Dual steady flow solutions of heat and pollutant removal from a slot ventilated welding enclosure containing a bottom heating source. International Journal of Heat and Mass Transfer, 132, 11–24. https://doi.org/10.1016/j.ijheatmasstransfer.2018.11.121
- Zhang, H. J., Dai, L., Xu, G. Q., Li, Y., Chen, W., & Tao, W.-Q. (2009). Studies of air-flow and temperature fields inside a passenger compartment for improving thermal comfort and saving energy. Part I: Test/numerical model and validation. Applied Thermal Engineering, 29(10), 2022–2027. https://doi.org/10.1016/j.applthermaleng.2008.10.005
- Zhang, S., Cheng, Y., Oladokun, M. O., Huan, C., & Lin, Z. (2019b). Heat removal efficiency of stratum ventilation for air-side modulation. Applied Energy, 238, 1237–1249. https://doi.org/10.1016/j.apenergy.2019.01.148
- Zhang, Z., & Chen, Q. (2006). Experimental measurements and numerical simulations of particle transport and distribution in ventilated rooms. Atmospheric Environment, 40(18), 3396–3408. https://doi.org/10.1016/j.atmosenv.2006.01.014
- Zhao, B., An, N., & Chen, C. (2021). Using an air purifier as a supplementary protective measure in dental clinics during the coronavirus disease 2019 (COVID-19) pandemic. Infection Control and Hospital Epidemiology, 42(4), 493–493. https://doi.org/10.1017/ice.2020.292