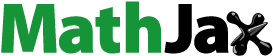
Abstract
Vortex generators (VGs) have shown the potential to mitigate the train's operational instability issues caused by strong wind. Numerical simulations are used to predict the flow structures around a train with VGs of different heights. The improved delayed detached eddy simulation (IDDES) hybrid modeling method is adopted to predict the trailing vortices on the leeward field. The numerical method is validated by reproducing wind tunnel test results. The study results reveal that VGs are capable of reducing the rolling moment coefficient around the leeward rail of a train by about 5% ∼ 15% while keeping the drag of the train still lower than its operational drag without crosswind. The control mechanism lies on that the streamwise vortices generated by VGs are attracted to the large-scale trailing vortices, resulting in the pressure on the leeward wall rising. The differences in the domain frequencies between VGs and Baseline cases in POD modes indicate that the VGs changed the periodicity and symmetry of the vorticity fluctuation. This study provides a new method to improve the safety of trains under crosswinds.
Introduction
The speed of high-speed trains has increased to 400 km/h (Park and Kim Citation2016), dramatically improving commuter efficiency. A higher speed accompanies a higher sensitivity of the operation of a high-speed train (Chen et al., Citation2022a and Citation2022b) and may cause a larger risk of unsteadiness affecting routine operation safety when a train experiences side forces caused by strong wind (Baker et al. Citation2009; Zhang et al. Citation2015), which is closely associated with economic losses and the potential for commuter casualties (Fujii et al. Citation1999; Gao et al. Citation2021). Consequently, it is a significant concern for scholars and institutions alike (Diedrichs et al. Citation2007; Liu et al. Citation2016b).
There are mainly three methods to deal with crosswind issues (Th. Tielkes et al. Citation2008; CEN European Standard Citation2018): using monitoring and early-warning systems (Gao et al. Citation2014), limiting the speed of high-speed trains, and constructing windbreaks along the rail lines (East Japan Railway Company Citation2022; Gautier et al. Citation2001). For example, along the most risky rail line of Southern Xinjiang Railway, 17 wind monitoring points are used to predict future wind speed based on transient data (Liu et al. Citation2022); European standard (CEN European Standard Citation2018) regulates a high-speed train must operate below 200 km/h under crosswind at 30 m/s; windbreaks with a height of more than 2 m are well recognized being beneficial for improving train’s stability under crosswind (Mohebbi and Rezvani, Citation2018; Mohebbi Masoud and Rezvani Mohammad Ali, Citation2021). However, massive funds are needed for wind monitoring or windbreaks, and reducing the train speed is not conducive to the railway’s efficient operation. Therefore, there are still some deficiencies in preventing the operational risks of trains responding to crosswinds.
Relative wheel unloading, which is influenced by the rolling moment, is an important indicator to measure the safety of a train under crosswinds (CEN European Standard Citation2018). The rolling moment is composed of a lift force and a side force. The side force rises because large-scale trailing vortices develop on the leeward of trains (Hemida and Baker Citation2010), which causes the appearance of negative-pressure regions (Niu et al. Citation2018). Therefore, reducing the size and intensity of the trailing vortices by flow control methods is an effective way to improve train operation safety under crosswinds.
Vortex generator (VG) (Schubauer and Spangenberg Citation1960) is a promising flow control device to overcome the adverse pressure gradient by manipulating the separation vortex, which has been widely studied in many fields, such as aerospace, automobile, vehicle (Kuya et al. Citation2009) industries. When VGs locate at NACA 4415, the maximum lift coefficient for an airfoil could increase by 21% (Fouatih et al. Citation2016). In terms of aerodynamic drag reduction, VGs also have a good performance. The helicopter drag decreases by 2% with VG array mounted on the rear ramp (Zanotti et al. Citation2016). Because the VG improves the boundary layer and triggers a smooth increase of the adverse pressure gradient, which is beneficial for preventing premature flow separation (Gibertini et al. Citation2015).
The purpose of adding VGs is to generate streamwise vortices that supply momentum from the higher region that has larger momentum to the near-wall region that quickly loses momentum (Gorton et al. Citation2002). This enables VGs to control the separation effectively (Koike et al. Citation2004) and weaken the strength of the counter-rotating vortex pair (Wortman Citation1999; Zhang et al. Citation2023). It has been observed that VGs can effectively reduce the size of the train wake vortices (Du et al. Citation2022), which inspired us to study the control effect of VGs on the trailing vortices of a train under crosswinds. The rolling moment of a train may be reduced if VGs are in the proper position with an appropriate shape.
The previous studies conducted a preliminary analysis of anti-rolling by conventional methods and drag reduction by installing VGs on high-speed trains (Li et al. Citation2023). However, the effectiveness of VGs in mitigating overturning of high-speed trains under crosswind conditions has not yet been investigated, resulting in that the applicability of VGs to address crosswind-induced issues of trains remains unclear. Thus, the main objective of this study is to determine the effectiveness and mechanism of VGs in reducing the rolling moments experienced by trains when subjected to crosswinds.
In this study, the effects of VGs with different heights on trains under crosswinds are predicted through numerical simulations to explore a new control method for improving the operational safety of high-speed trains under crosswinds. The flow control effects of train aerodynamic force caused by VGs are first examined. The pressure, vorticity, and vortices modes are then studied to understand the control mechanism of VGs on high-speed trains under crosswind. This study paves the way for applying VGs to reduce the rolling performance of high-speed trains in the future.
Numerical method
Model geometry
As shown in Figure , a three-car CR400BF model is chosen for the simulation. The pantograph, windshields, and bogies are ignored, which may lead to less obstacles of the airflow passing through the vehicle body resulting in a more regular boundary layer. The highly simplified model is more conducive to explore the relationship between VGs and the rolling moment, which has been used by other scholars (Östh et al. Citation2015). The characteristic parameters of the train are L × W × H = 80.6 m × 3.36 m × 4.05 m. The VGs are set at a uniform thickness e = 10 mm. The dimensionless distance determines the location information of the VGs, where λ is the distance between adjacent vanes’ trailing edges. The angle between the VGs and inflow velocity is
, which is set to 30°. An appropriate value of
can significantly improve the vortex strength of the VGs (Betterton et al. Citation2000; Godard and Stanislas Citation2006). The installation angle
is 50° since the yaw angle is 20°. The shape of the VG is rectangular in this study. The dimensionless device height of the VG is defined as
, and the dimensionless device length is defined as l/h, where h is the VG device height, l is the VG device length, and
is the thickness of the boundary layer. These parameters describe the geometric dimensions of the VG device.
According to a research review (Lin Citation2002), low-profile VGs with low device resistance, whose values are equal to 0.1 ∼ 0.5, can transmit enough wallward momentum to areas higher than several times the height of conventional VGs with
∼ 1. The VGs are relatively efficient when
is in the range of 0.1 ∼ 0.3 and can provide maximum pressure recovery with
∼ 1 (Lin et al. Citation1990b; Lin et al. Citation1990a). In this paper,
values of 0.2, 0.4, and 0.8 are chosen, corresponding to the lower VG (LVG), medium VG (MVG), and higher VG (HVG) cases, respectively. The flow around the roof of the CR400BF model under crosswinds is predicted by numerical simulations to obtain the specific
. The thickest portion of the boundary layer occurred at the arc from the leeward wall to the roof of the tail vehicle, which will be about 0.1 m at full-scale tests. Although the VGs are not sensitive to the value of l/h, which is 12, 6, and 3 respectively in this study, the minimum value should be greater than 2 (Godard and Stanislas Citation2006). It is reasonable for VGs to be within 2
upstream of the separation point to maintain the control effect of the VGs (Lin et al. Citation1990b). Therefore, the downstream end faces of the vans are near the boundary layer separation position. The dimensionless distance
∼ 2.5 is within the range studied by (Krajnovic Citation2009). The above parameters in different cases are shown in Table .
Table 1. Geometric parameters of VG in each case.
Computational domain and boundary conditions
In the calculation domain, a 20° angle is set between the train and the streamwise direction. The top and front views of the calculation domain are shown in Figure . The world coordinate system depends on the inlet boundary. The X-axis points downstream of the inlet boundary, and the Y-axis is parallel to the inlet boundary. The coordinate origin is located in the position where the train centre point is projected to the ground. At the same time, a rotating coordinate system is established which is obtained by rotating the world coordinate system clockwise by 20°. The new axes are denoted as ,
and
which is the same as the Z axis of the world coordinate system.
Figure 2. Calculation domain and its boundary conditions with the world coordinate system and the rotation coordinate system: (a) top view and (b) front view.

The computational domain reserves a length of 10H upstream of the train, and 30H is arranged downstream of the train to allow the wake vortices to fully develop (Gallagher et al. Citation2018). The height of the calculation domain is set as 10H, and the distance from the leading vehicle and tail vehicle to the wall on both sides is 10H. This ensures that the projected area of the train along the X direction is relatively small compared with the cross-sectional area of the computational domain, and the blocking ratio is about 3.2%. The gap between the train and the ground is 0.235 m (CEN), which is the cost of omitting the track and ballast.
The inlet boundary condition is set to a velocity inlet with a constant velocity. The turbulence intensity is 1%, the turbulent viscosity ratio is 10.0, and the resultant velocity is 60 m/s.
includes the velocity component in the X direction representing the train speed Ut = 51.96 m/s and the velocity component in the Y direction representing the crosswind speed Uc = 30 m/s. The Reynolds number Re =
= 2.1 × 106 meets the requirement of being greater than 2.5 × 105 (CEN), where the density of air at 15°C is
= 1.225 kg/m3 and the dynamic viscosity is
= 1.78 × 10−5 N·s/m2. The outlet boundary condition is set as a pressure outlet which assumes that the incoming flow enters the simulation along a vector that is orthogonal to the boundary surface to provide the most robust convergence. The train body surface is set as a no-slip wall. As the train running direction is inconsistent with the incoming flow direction, the ground also needs to be set as a no-slip wall to avoid the impact of the downstream region on the side wall caused by the ground moving along the train direction. The slip wall is used on both sides of the wall and on the top to reduce the interference of the wall to the flow field (Maleki et al. Citation2020).
Numerical model
The detached eddy simulation (DES) turbulence model, as a hybrid model, combines the Reynolds-averaged Navier–Stokes (RANS) and large eddy simulation (LES) methods. The DES uses the RANS mode for the boundary layers and the LES for the unsteady separated regions. However, the original DES equation, DES97, proposed by Spalart et al. (Citation1997), has problems with modeled-stress depletion (MSD) (Ashton Citation2017) and log-layer mismatch (Hamba Citation2009). Spalart et al. (Citation2006) then proposed the delayed detached eddy simulation (DDES), which improved the identification of the boundary layer and robustness of the irrotational domain by introducing parameters such as the kinematic eddy viscosity, velocity pressures, and distance to the wall into the formula of the length scale. The improved delayed detached eddy simulation (IDDES) proposed by Shur et al. (Citation2008) solves log-layer mismatch by combining the DDES and wall-modelled LES (WMLES) and applies them to mixed flows with both attached and separated regions. The IDDES considers both the calculation cost and accuracy and has been widely used in unstructured grids (Wang et al. Citation2020). In addition to the traditional Finite Volume Method, the Lattice Boltzmann Method can also achieve high fidelity flow field prediction (Mohebbi and Safaee Citation2022).
This paper applies IDDES as the computation method because it owns the advantages of RANS mode for the boundary layers and the LES for the unsteady separated regions. The shear-stress transport (SST) K-Omega model is used as it can more accurately predict strong adverse pressure gradients and separation (Menter et al. Citation2003). The segregated flow solver of the commercial software STAR CCM + is used for the simulation, which used the Semi-Implicit Method for Pressure-Linked Equations (SIMPLE) algorithm to achieve coupling of the pressure and velocity and then solve the mass and momentum conservation equations. This method applies to incompressible flows with Ma < 0.3. The convection scheme is a mixed bounded central difference (BCD) scheme, which mixes the second-order upwind scheme with the BCD to maintain the accuracy and stability of the calculation. The upwind blending factor is 0.15. The Quadratic Constitutive Relation (QCR) improves the prediction of secondary flows.
The transient term of the transfer equation is discretized to the second order to meet the requirements of a high-precision prediction. The time step is , where
is the characteristic time. The characteristic time is reduced 8 times due to the 1/8 mesh scaling. The convective Courant number, which represents the number of grid elements that the fluid crosses per time step, in the wake region of the tail vehicle is less than one in most regions (see Figure ). Only in the near wall region of the train model is the convective Courant number greater than one, whereas the maximum value is less than two.
In Figure , the variations of the rolling moment coefficient around the lee rail CMx,lee, drag force coefficient CFx, and side force coefficient CFy with time are displayed. The formula for CMx,lee is , the formula of CFx is
, and the formula of CFy is
, where Mx,lee is the rolling moment around the lee rail, Fx is the drag force, Fy is the side force, and S = 0.187 m2 is the reference area of the 1/8 model. First, the RANS method is used to initialize the flow field to accelerate the convergence, enhance the IDDES calculation's stability, and reduce the total calculation cost (Wang et al. Citation2020). The entire calculation time of the IDDES is
, where the time of flow field development is
and the sampling time is
. The fluctuations of CMx,lee of the train are stable after RANS initialization and IDDES flow field development. Therefore, the time to start sampling is reasonable.
These calculations were performed at the High Performance Computing Platform of Central South University. The CPU used was Intel(R) Xeon(R) Gold 6248R. Four nodes are used in each case, and each node has 48 cores. The calculation cost for each case, excluding the RANS initialization times, is about 13824 core hours. The grid generation utilizes a precision desktop workstation with an Intel(R) Xeon(R) Gold 6138 CPU and 128 GB RAM.
Meshing strategy
The mesh is made by the trimmed mesher of STAR CCM+, which is suitable for external flow field predictions. The high pressure gradient and vorticity regions are densified to generate a high-quality mesh adapted to the IDDES simulation (Maleki et al. Citation2020). These regions, which are consistent with previous research (Baker Citation2010), are predicted using a densified mesh. As shown in Figure , the mesh is mainly divided into four levels of refinement regions. The mesh size difference between adjacent refinement regions is double. Refinement 1 mainly densifies the gap between the trains and the ground (not shown) with Δx = Δy = Δz ≈ 0.01H. Refinement 2 mainly densifies the regions with a high pressure gradient near the train and the regions near the wall on the leeward with Δx = Δy = Δz ≈ 0.02H. Refinement 3 mainly densifies the near trailing vortex region with Δx = Δy = Δz ≈ 0.04H. Refinement 4 mainly densifies the trailing vortex region with Δx = Δy = Δz ≈ 0.08H.
Figure 5. Top views of mesh densified region: (a) leading vehicle, (b) near wake region, and (c) wall y+ of train surface.

It is generated 17 prism layers around the train surface, with a target growth rate of 1.2, and five prism layers are generated for the ground surface. The ground surface is densified to reduce the volume variation rate between the prism layer and the volume mesh and improve the stability and convergence of the calculation. The location where the boundary layer separates due to the reverse pressure gradient requires a low dimensionless wall distance y+ to predict the boundary layer velocity accurately. The exact value of the wall y+ in this study is shown in Figure (c), which is within an allowable scope according to (CEN).
Three sets of meshes are generated for mesh convergence verification. and
values represent the grid resolutions of the flow and spread directions, respectively (Davidson Citation2009). The parameters of the three meshes are shown in Table . Because the mesh type is an isotropic trimmed volume mesh, the
and
are the same. Between different meshes, such as coarse and medium meshes, the difference in the grid resolution in each spatial direction is greater than or equal to a factor of 1.5 (CEN European Standard Citation2018).
Table 2. Mesh parameters for mesh convergence verification.
Numerical method validation
The values of the train aerodynamic forces for the three meshes are shown in Table . The CFx and CFz values show good consistency for the three meshes, and the relative error is less than 1%. Only CMx,lee and CFy show significant errors in the coarse mesh, which may be caused by the rough volume mesh on the leeward. In this study, a medium mesh configuration is adopted.
Table 3. Calculation results of train aerodynamic forces on three meshes.
The correct prediction of the trailing vortices is the key to this study. Note that the meshes discussed now are those outside the prism layer. During the initialization of the flow field with the RANS method, the length scale ratio is adopted to determine the relationship between the turbulence length scale
and the grid size
, where
, k is turbulent kinetic energy,
is the specific dissipation rate,
, and
is the cell volume.
should be greater than 2 in the region so that the vortex can be resolved rather than modeled (Siemens Citation2021), which means that turbulence could be captured by two or more cells in a period. Figure (a) shows the isosurface of
= 2 and the
distribution in the Z plane. Regions with a length scale greater than 2 are mainly concentrated downstream of the lee. This is within our expectation and the degree of coincidence with the region of high turbulent kinetic energy
is at a high level, as shown in Figure (b). In the flow field, velocity standard deviations of many regions are close to zero. Weak pulsations must be matched with high computational costs, so they do not enter the analysis scope.
Figure 6. Scalar contour plots of the Z plane and isosurface: (a) Reynolds-averaged Navier–Stokes (RANS) length ratio (lRANSRatio = 2) and (b) the turbulent kinetic energy ( = 8 J/kg).

The above steps ensured that the large-scale vortices can be captured by the mesh, even when the LES branch of the DDES is not triggered. In addition, whether the trailing vortices are resolved by the LES is analyzed here. The length scale of the IDDES is given by the following formula (Shur et al. Citation2008):
(1)
(1) where
is the IDDES elevating function, and
(2)
(2) depends on
. fe1 determines whether elevation occurred, fe2 controls the elevating intensity, dw is the distance to the wall, and
is the maximum local grid spacing. For the cells outside the prism layer, fe1 < 1 and fe = 0. Thus, the length scale of the IDDES is simplified to
, and the WMLES branch of the IDDES stops working. The following equations are applied
(3)
(3)
(4)
(4)
(5)
(5)
(6)
(6) where
and
are the length scales of the RANS and LES modes, respectively,
is similar to fe1 and tends to 0 when it is outside the prism layer,
is the kinematic turbulent viscosity,
is the turbulent viscosity, and
is the von Karman constant.
Figure shows the distribution of the IDDES blending function in the Z plane and the white translucent isosurface with
= 0.5. From the Z plane, the low
regions are mainly near the train, on the leeward and downstream. Moreover, as the height increases, the area with
of 0 gradually expands. Therefore, the RANS mode predominantly governs the upstream region of the train and positions near the sidewalls, while the LES mode primarily governs the surrounding and downstream areas of the train. The mesh size in the wake and leeward regions that we are concerned with is sufficient to trigger the LES mode.
Withsss the same meshing strategy and numerical method described above, a comparison between the numerical results and wind tunnel test data for CR400BF at the yaw angle of 30° is shown in Table . The model used for numerical validation is same with the wind tunnel experiments, which is a generic reduced-scaled (1:8) high-speed train consisting of a leading vehicle and an adjacent half trailing car. The wind tunnel study took place in a large-scale low-speed wind tunnel of the China Aerodynamic Research and Development Center (CARDC), as illustrated in Figure . The test section is 20, 8 and 6 m in in length, width, height, respectively. Wind velocities within the tunnel ranged from 20 m/s to 100 m/s, maintaining a corresponding turbulence intensity below 0.12%. To mimic yaw effects, a freely rotating 7-meter diameter disk was positioned on the testing platform. A specialized floor design elevated the setup approximately 1 m above the tunnel floor, reducing boundary layer development. A scaled-down model of a high-speed train, reduced to 1:8 in scale and comprising both a leading vehicle and an adjacent half-trailing car, was centrally placed on a single ballastless track.
Table 4. Comparison of aerodynamic coefficients between wind tunnel tests and numerical simulations.
Figure 8. Test configuration in FL-13: (a) top view; (b) general view; (c) side view and (d) test view. (Li et al. Citation2022).

Incoming flow velocity measurements were obtained using a Pitot tube positioned at the front of the test section. Aerodynamic forces and moments in three Cartesian directions were captured via a six-component internal dynamometric balance located within the train cabin. This balance exhibited a precision level of less than 0.09% and an accuracy error of less than 0.24%. Pressure taps with a 1mm diameter were typically installed on the vehicle. Transient pressure data was recorded through a pressure scanning valve connected to metal tubes via transmission pipes. The tests employed a high-frequency miniature pressure scanner (ESP-64HD) produced by Pressure System Inc., characterized by a precision level of approximately ±0.03%. During the test, a sampling frequency of 1 kHz was employed, the sampling duration extending to 30 s. You can refer to the reference articles for more details (Huo et al. Citation2023).
The predictions of the rolling moment coefficient, , and CFy are accurate, and the errors with the wind tunnel test are within 1%, where
is the rolling moment. Although the relative error of CFx is 11.1%, the absolute error is only 0.01, which is also an accurate result. Only CFz has a significant error of −7.5% because the track in the wind tunnel test is a scale model of the real track and the track is not continuous to allow the supporting pillars to be installed. In contrast, the track used in the numerical simulation is a simplified track conforming to CEN standards, which can lead to an inconsistent flow at the bottom of the train, which is reflected in the error in CFz. This error is inevitable because the characteristic size of the real track is far smaller than the characteristic size of the train and can not be retained. Because of the model differences, the error in CFz has a low reference value. Based on the results of CMx, CFx, and CFy, the meshing strategy and numerical method adopted in this study are suitable.
Figure shows the predicted train surface pressure coefficient of Loop 1 and Loop 3, which are highly consistent with the wind tunnel test data. Only on the leeward does the pressure coefficient line of the numerical simulation deviate slightly from the data points. In Loop 1, the maximum absolute error of 0.032 indicates a relative error of 4%. In Loop 3, the maximum absolute error of 0.013 indicates a relative error of 1.9%. In general, the pressure coefficient line also proves the accuracy and reliability of the prediction results.
Results and analysis
Aerodynamic force and moment
The aerodynamic forces and moments of the train with the lower, medium, and higher VGs are shown in Figure . The difference from the train in the Baseline case is shown in Table . LVG, MVG, and HVG represent the cases corresponding to the three kinds of VGs, as described above, and Baseline represents the case without VGs. VGs are conducive to reducing CMx,lee and CFy while increasing CFx and CFz. With the increase in the h values of the VGs, CMx,lee and CFy decrease, while CFx and CFz increase.
Figure 10. Aerodynamic force and moment coefficients of the train with different vortex generators (VGs).

Table 5. Aerodynamic force and moment coefficients of the train with different VGs and their differences.
VGs decrease the CMx,lee of a train with two methods, which are generating anti-rolling moment by the vanes and reducing the CMx,lee of the vehicle itself. The contribution of the anti-rolling moment directly generated by the vanes accounts for about 10% ∼ 20% of the net decrease value. The leading vehicle in the Baseline is dominant in CMx,lee with a value of −1.210, which contributes the most to the vehicle rolling moment compared with that of the middle and tail vehicles. However, the leading vehicle CMx,lee of the three VG cases decrease by only 3.3% ∼ 9.3%, while the middle and tail vehicles CMx,lee can decrease more significantly by 15% ∼ 23%. It is because the CFy of the leading vehicle decreases slowly leading to three vehicles performing different CMx,lee values, even with VGs of equal h. With an increase in the height of VGs, the CMx,lee of the leading and tail vehicles decreases, however, the change in CMx,lee for the middle vehicle is almost not discernable. This means that VGs with h ≤ 0.2δ could be a great design for the middle vehicle.
At the same time, the increase in CFx could not be ignored. The CFx of the train with VGs for the LVG, MVG, and HVG cases increase by 0.019, 0.041, and 0.106, respectively, from the Baseline case. The CFx of the vehicles themselves is identical in the three cases. The increase in the total CFx mainly attributes to the VGs whose device resistance accounts for 90% ∼ 98% of the total change. The CFx of the VGs on the middle vehicle is higher than others because the number of vanes on the middle vehicle is 63 while that of the leading and tail vehicles are 43. It is noticed that the CFx of the train is 0.232 without crosswind, which is higher than the CFx of the train in the LVG and MVG cases. This indicates that for trains in crosswind conditions, the VGs can perform an anti-rolling effect while keeping the drag at a lower level than that without crossflow.
The CFy of the vehicles significantly decreases except for the tail vehicle in the LVG case. The CFy of the middle vehicle decreases by 53.6% compared to the Baseline case. But the CFy of the leading vehicle decreases only by 10.6% requiring a 26.4% increase in resistance as a cost. This indicates that there is still significant room in improving configuration of the VGs of the leading vehicle.
On the contrary, The CFz of the vehicles significantly increases except for the tail vehicle in the LVG case. The CFz of the middle vehicle shows the largest changes. The CFz of the middle vehicle increases from −0.020–1.489 after installing the VGs with a height of 0.8 h/δ.
Surface pressure distribution
As the changes of CFy and CFz are essential factors for the reduction of CMx,lee, it is straightforward to investigate the difference in the pressure distribution on the train surface affecting CFy and CFz values. Figure shows the surface pressure distribution on the train’s leeward wall and the corresponding line plot around the horizontal profile of the train. The horizontal profile is marked with black solid lines in the contour plots. The contour plots are flipped horizontally to connect with the line plot. Thus, the incoming flow direction is from the left.
Figure 11. Surface pressure coefficient of the train: contour plots for the (a) Baseline, (b) lower VG (h/δ = 0.2), (c) medium VG (h/δ = 0.4), and (d) higher VG (h/δ = 0.8) cases and (e) line plots.

The contours are coloured by the pressure coefficient Cp, whose formula is , where P is the gauge pressure. The pressure contours on the windward side of the train are not shown since the differences are tiny and barely related to the VG installation. There are mainly 3 negative pressure regions on the leeward side of the train named N1, N2 and N3.
In the /L ∼ (−0.5, −0.3) range, there is little difference in the pressure contours, and the line plot is coincident. This is because the section is upstream of the VGs. In the
/L ∼ (−0.3, −0.1) range, the differences between the four cases gradually become apparent. In the Baseline case, the pressure exhibits a lower value in the yellow region (N1). By adding VGs, the pressure in the low-pressure region has risen with the height of VGs. As shown in the line plot, the difference in the Cp increases gradually as moving downstream due to the effect of the VGs.
In the /L ∼ (−0.1, 0.1) range, the Baseline case is quite different from the VG cases. The Cp in the LVG case in the line plot has an absolute increase of 0.1 with a relative increase of 38% compared with the Baseline case. What's more, the Cp demonstrates some lags occurring in the Baseline case because the negative pressure region (N2) invades downstream. On the pressure coefficient contours, this negative pressure region's range and absolute value decrease with the increase in h. The red positive pressure region near the roof has widened in the VG cases compared with the Baseline case.
In the /L ∼ (0.1, 0.5) range, the differences between the three VG cases gradually decrease. In the line plot, the lag between the Baseline case and VG cases is more significant due to the changes in N2; along the positive
direction, the Cp in the Baseline case rises later and the descent phase also occurs later. The lag between Baseline case and HVG case is approximately manifested as 0.1
/L in spatial distance. Due to the phase difference introduced by the lag between subfigure (a) to (d), it results in a reduction in difference among pressure line plots of the 4 cases in the
/L ∼ (0.2, 0.3) range. As the pressure develops downstream, the contour shows a stable pressure region (N3), where the pressure no longer changes drastically downstream until approaches the streamlined regions of the tail vehicle. The pressure near the roof is far greater in the VG cases in the constant cross-section regions of the train body, which significantly reduces the CFy of the train. In the streamlined regions at the train tail, the Cp in the Baseline case shows a stronger pressure recovery, whereas the values of Cp near the nose are close in all cases.
The direct effect of VGs on the surrounding flows is reflected by the surface pressure coefficient contours of the train roof, shown in Figure . In the /L ∼ (−0.1, 0.1) range, there is a zero-pressure region in the Baseline case, whereas the regions are affected by VGs and the Cp drops in an extensive range. Because of the acceleration of the fluid at the edge of the vanes, the local velocity near the VGs increases, resulting in the Cp drop. As the h/δ increases, the Cp decreases which is equivalent to the CFz increases. The cause of this phenomenon will be explained with the help of Figure in the next section. The Cp in the streamlined regions at the head and tail of the train do not change significantly in the VG cases, which indicates that the influence scope of the VGs in the
direction is small. The regions near and downstream of the VGs in the
direction are greatly affected.
Vortex structures
In order to further explore the reasons for the change in the surface pressure coefficient, the vortex structures are compared and analyzed. Figure shows the isosurface generated by the third-generation vortex identification criterion called the -method. Compared with second-generation vortex identification criteria, such as Q-criterion and
, the Ω-method has the advantages of having an insensitive threshold and weak vortex capture (Wang and Gui Citation2019). Therefore, parameter
is introduced, representing the ratio of the vortical vorticity over the whole vorticity inside a vortex core. The formula of
(Liu et al. Citation2016a) is as follows
(7)
(7) where
is a small positive number used to avoid division by zero, and A and B are symmetric tensors and antisymmetric tensors of the second-order tensor
decomposition, respectively. In the Cartesian coordinate system, these are the strain rate tensors and vortex tensors in fluid mechanics. The
decomposition is as follows:
(8)
(8) When
, the antisymmetric tensor is superior to the symmetric tensor, which can be used as a criterion for vortex identification. Generally,
is selected as a fixed threshold to identify vortex structures, which many users have verified. In addition,
can be determined by a formula to avoid artificial selection (Dong et al. Citation2018):
(9)
(9) The vortices of the four cases shown in Figure are similar. The isosurface in Figures (a), (b), and (d) is coloured with V/U∞, while the isosurface in Figure (c) is coloured with ωyH/U∞. The formula of the vorticity is
(10)
(10) The primary vortices are represented by V, and V1 ∼ V4 represent four vortices. V1 is derived from the obstacle catcher of the leading vehicle, V2 is derived from the streamlined region of the leading vehicle, V3 is derived from the bottom of the middle vehicle, and V4 is derived from the roof of the tail vehicle. Their shapes are similar to those summarized by (Baker Citation2010). V1 is far from the train body, it would not have a significant impact on the pressure on the train. The strengths of V2, V3 and V4 determine whether the pressure on the leeward decreases.
Because the derived region of V1 is near the obstacle catcher, V1 in the VG cases is not affected by the VG vanes. The velocity and scale of the secondary vortex are similar to those of the Baseline case. The rotation direction of V1 is clockwise.
Although V2 is separated from the streamlined region of the leading vehicle, which is located upstream of the VGs, it is deeply affected by the separated airflow from the top of the train. V2 has a higher velocity and more secondary vortices in the Baseline case compared with the other three VG cases. In the enlarged view of Figure (a), the airflow separating from the roof is attracted by V2, which drives the vortex intensity of V2 to increase. In the VG cases, the vortices generated by VGs are attracted by V2 instead of forming a steady separated airflow without block in the Baseline case, which leads to a decrease in the velocity of V2. Moreover, with the increase in the h of the VGs, the decrease in velocity is more evident in the HVG case. Therefore, VGs will lower the strength and stability of V2 and correspondingly increase the pressure on the lee, mainly in the upstream region dominated by V2.
As shown in Figure and Figure , the pressure of the leeward of the tail section is conquered by V4 instead of V2. The V2 is far away from the leeward wall while the V4 gradually expands along the downstream direction. This is why the pressure on the roof is higher in the middle vehicle (See Figure ) as weak vortices are affecting the local area.
The secondary vortices around V1 moving downstream are all rotating in the positive Y-axis direction, while the rotation direction of the secondary vortices around V2 and V3 are periodically switching. Thus, the speeds of the adjacent secondary vortices around V2 and V3 are not the same. The periodic shedding of the wake vortices causes the secondary vortex of V4 to exhibit alternating vorticity directions. The secondary vortices around V4 which are composed of periodically shedding wake vortices are similar to vortex street.
Although the vortices of the three VG cases are different, it is more interesting to compare and analyze the cases between baseline and VG cases. Therefore, the two-dimensional contour of the time-averaged vorticity is plotted to distinguish differences more easily, as shown in Figure . The green streamlines represent the vortex cores, the red contour represents the clockwise rotating vortices, and the blue contour represents the counter-clockwise rotating vortices. Because the simple shear flow contains ω, the ground boundary layer is inevitably identified. Some ground boundary layer data are isolated to reduce the interference of the ground boundary layer.
V1 is generated by the separation of airflow at the end of the cowcatcher, and the vortex size is small. V2 starts from the end of the streamlined region of the leading vehicle and is formed by the air rolling from the roof. V3 starts from the constant cross-section region of the leading vehicle and is formed by the air rolling from the bottom. V4 extends downstream and gradually lefts the train body at the middle position of the train.
When the trailing vortex is in close proximity to the vehicle, it exhibits slight irregularity. In the upstream of P0, the pressure distribution on the leeward side is predominantly governed by V2 and V3. Conversely, in the downstream P0, the leeward pressure field is primarily regulated by V4. The scales of V2, V3 and V4 in the MVG case are smaller than those in the Baseline case. One can see V4 is broken and dissipated faster in the MVG case, which indicates the VGs significantly reduce the strength of the dominant vortices. This must be the key factor in increasing the pressure on the leeward by adding VGs.
Figure shows the velocity distribution in the plane at the central position of the train. One can observe that the kinetic energy of V2 and V3 gradually decreases as the VG height increases. This phenomenon arises due to the delayed flow separation caused by VGs, resulting in the airflow passing over the roof having a more pronounced downward tendency. This could be the key to the VGs being able to weaken the large-scale trailing vortex on the leeward side. The flow separation control by VGs has been widely used for drag reduction (Lin Citation2002) and heat dissipation (Awais and Bhuiyan Citation2018).
Proper orthogonal decomposition
The proper orthogonal decomposition decomposes the flow field into a linear superposition of basis functions and correlation coefficients, which can effectively extract the important coherent structures of the flow field. This is helpful to better describe and analyze the evolution characteristics of V2 and V3. There are two methods: the classical method and the snapshot method (Sirovich Citation1987). This study adopted the snapshot method because it is more effective at high spatial resolutions with the appropriate number of snapshots (Chen et al. Citation2013). The velocity (excluding the X component of the velocity) and time series of P0 in Figure are expressed in matrix form, and the following proper orthogonal decomposition is performed:
(11)
(11) where k is the snapshot index. According to the proper orthogonal decomposition (POD) method, the flow field is then decomposed into a linear combination of M spatial basis functions and a set of mode coefficients as follows:
(12)
(12) where
is the basis function,
is the mode coefficient. The mathematical procedures of the POD method are reported by (Chen et al. Citation2013). It is worth noting that when the sampled cell volumes are inconsistent, as in this paper, the temporal correlation matrix of the singular value decomposition (SVD) requires volume weights to be introduced (Muld et al. Citation2012a).
Convergence verification is a precondition of applying the POD method. The converted modes require a reasonable time step and a sufficient number of snapshots. The mode that reaches the convergence state will not change with the time step and the number of snapshots. There are two methods to evaluate mode differences (Muld et al. Citation2012b). The first method is to compute the scalar product of the modes, and the second method is to compute the
-norm of the difference between modes.
and
are defined as follows:
(13)
(13)
(14)
(14) where 1 and 2 indicate different data sets.
The two methods are consistent. For the POD mode, an orthogonality test is recommended (Muld et al. Citation2012a). Figure shows the orthogonal test results of POD modes 1 ∼ 7 with different sampling numbers and frequencies. Figure (a) is resolved with = 0.1
, and Figure (b) is resolved with
. When the sampling time
is higher than 250 and the sampling interval is less than 0.2
, POD modes 1 ∼ 5 converge. Therefore, the accuracy can be guaranteed by selecting the sampling time
and sampling time interval
= 0.1
.
Figure 16. Scalar product of the difference between the sets of modes: (a) different snapshot numbers and (b) different /
values.

V can be decomposed by the SVD method, , where S is the singular value matrix corresponding to V or
, where the elements are arranged according to the energy of the corresponding orthogonal basis functions. The energy ratio of POD mode k to the total energy of the flow field is.
(15)
(15) According to the formula, the energy fraction of POD mode 1 is about 99.2%, which is much higher than the sum of the other modes. This is a POD characteristic of the flow type dominated by the average flow. As shown in Figure , The blue bar represents the energy fraction of POD modes 2 ∼ 20, and the orange bar and line with pink points represent the cumulative energy fraction. The fraction of each mode in the remaining energy is less than 0.2%. The cumulative energy fraction of POD modes 2 ∼ 5 is higher than 0.4% which is more than half of the residual energy. The energy fractions of the higher modes are low, and thus, they are not worthy of further analysis.
Figure 17. Energy fractions (left axis) and cumulative energy fractions (right axis) in residual energy for the proper orthogonal decomposition (POD) modes in the Baseline case.

is dealt with by the POD method to obtain
. The curl of the two-dimensional
is calculated to show the vortex shape more clearly. P0 (see Figure ) is coloured by the curl of
, as shown in Figure , which shows POD modes 1 ∼ 3 of the Baseline and MVG cases. Blue colour indicates the vortices that rotate counterclockwise, while red indicates the vortices that rotate clockwise. Line integral convolution provides additional information about the direction of the vector. The same colour for different POD modes may indicate different curl values.
Figure 18. Curl of for POD modes 1 ∼ 3 at a middle section of the train body in the Baseline and MVG cases.

POD mode 1 shows the average flow field of P0 and is the most important mode. The Baseline case has three primary vortices similar to the MVG case, whereas B2 is farther away from the train body compared with A2 because the airflow across the roof of the train with a stronger downwash trend has been delayed for its separation in the MVG case.
POD modes 2 and 3 represent the unsteady characteristics of the vortex structures. With the increase in the mode order, the scale of the vortex decreases gradually, and the quantity of vortical structures increases. The number and size of spatial structures in modes 2 and 3 are the same near the leeward wall in the Baseline case and are the same near the downstream in the MVG. The rotation directions of C1 ∼ C4 are opposite compared with E1 ∼ E4 as well as D1 ∼ D4 compared with F1 ∼ F4. The similar shape and the opposite rotation direction indicated that the vortex pair alternately generate and shed. In the MVG case, the modes 2 and 3 of the vortices near the leeward wall are quite different which means the vorticity fluctuation of the B2 and B3 is aperiodic and irregular. In other words, the VGs destroyed the stability of the trailing vortices.
Power spectral density (PSD) analyses of are indispensable, showing the corresponding dominant frequency of each POD mode or the frequency characteristics of the vortices. The independent variable is the Strouhal number, St =
, where H is the height of the train, f is the frequency, and
is the incoming flow speed. Figure shows the power spectral densities of POD modes 2 and 3 in the Baseline and MVG cases. This flow field characteristics of the mode 2 or 3 represent the main oscillation mode of the train wake. The frequency for POD model includes the pulsation frequency of vortices.
Figure 19. Power spectral density diagrams of different POD modes: (a) POD mode 2 and (b) POD mode 3.

In the Baseline case, there is indiscernible domain frequency in mode 2 which means the is random without periodicity. Although the PSD of
has an obvious dominant frequency St = 1.15, the energy of mode 3 is less than mode 2 (refer to Figure ) and the periodic fluctuations of the vorticity are weak. In contrast, one can see a dominant frequency at St = 1.06 in mode 2 in the MVG case, indicating that the VGs induce a regular pulsation of the trailing vortices. It should be noted that the dominant frequencies of modes 2 and 3 are different in Baseline or MVG cases, reflecting that the trailing vortices affected by VGs with an unclear periodicity are dissimilar to the wake vortices without crosswinds (Liu et al. Citation2020).
Conclusions
This paper has preliminarily explored the anti-crosswind effect of vortex generators (VGs) on high-speed trains, mainly focusing on the aerodynamic forces, surface pressure, and trailing vortices. The conclusions are summarized as follows:
The VGs are capable of reducing the train's side force and rolling moment while keeping the drag of the train still lower than its operational drag without crosswind. When the dimensionless device height of the VGs is
∼ 0.4, the rolling moment coefficient around the leeward rail CMx,lee is reduced by 10.4%. While
≤ 0.8, as the h increases, CMx,lee decreases and CFx increases.
The anti-rolling effect of the VGs is mainly achieved by increasing the pressure downstream of the leading vehicle streamlined region on the leeward which is controlled by the trailing vortices V2 and V4. VGs also significantly reduce the pressure on the roof, which is not conducive to reducing the rolling moment.
The velocity of the trailing vortices and secondary vortices decreases because the small-scale vortices generated by the VGs are attracted to the large-scale trailing vortices. The reduction of the vortex intensity is the main factor causing the pressure rise on the leeward. Moreover, with the increase in the height of the VGs, the reduction of the vortex intensity becomes more significant.
The POD modes show the differences in the generating and shedding of the vortices between the Baseline and VG cases. In the MVG case, the PSD of
displays a clear dominant frequency with a larger amplitude. The VGs change the periodicity and symmetry of the trailing vortex fluctuations.
This study provides a new method to improve train operation safety under crosswinds. In other words, under the same wind speed and direction, the VGs can increase the upper limit of a train’s safety operation speed. The results of this study lead to an important concept: methods that can produce the same effect as VGs, including air blowing, synthetic jets, and plasmas, may also work for achieving an anti-rolling effect. In practical applications, VGs with a fixed orientation may encounter challenging situations in its effectiveness because a train operates bidirectionally. Also, sharp vanes can also pose safety concerns to personnels in trains’ daily operation.
The above research was conducted on a simplified model of CR400BF, the VG parameters may need a revision in according to the flow features of other types of trains; the drag reduction effect of VGs on a train under different wind speeds and directions is not yet clear. In addition, most parameters of VGs are fixed due to the limited computing power. The current design of VG structure is not the optimal design scheme. Future work will include the optimization of various geometric and layout parameters of the VGs, and relationships between the parameters and the aerodynamic performance of the train will be studied. In the end, an optimized VG design with higher anti-rolling performance and lower device resistance will be proposed.
Acknowledgments
This work is supported by the National Key R&D Program of China (Grant No. 2020YFA0710903 and 2022YFB4301104), the Natural Science Foundation of Hunan Province (Grant No. 2020JJ4737), Technology Research and Development Program of China Railway Group (Grant No. P2023J001 and P2021J036), and the Postdoctoral International Exchange Program in China(Grant No. YJ20220218). We are grateful for resources from the High Performance Computing Center of Central South University.
Disclosure statement
No potential conflict of interest was reported by the author(s).
Additional information
Funding
References
- Ashton, N. (2017). Recalibrating Delayed Detached-Eddy Simulation to eliminate modelled-stress depletion. 23rd AIAA computational fluid dynamics conference, Denver, Colorado. 0605. American Institute of Aeronautics and Astronautics, Reston, Virginia.
- Awais, M., & Bhuiyan, A. A. (2018). Heat transfer enhancement using different types of vortex generators (VGs): A review on experimental and numerical activities. Thermal Science and Engineering Progress, 5, 524–545. https://doi.org/10.1016/j.tsep.2018.02.007
- Baker, C. (2010). The flow around high speed trains. Journal of Wind Engineering and Industrial Aerodynamics, 98(6-7), 277–298. https://doi.org/10.1016/j.jweia.2009.11.002
- Baker, C., Cheli, F., Orellano, A., Paradot, N., Proppe, C., & Rocchi, D. (2009). Cross-wind effects on road and rail vehicles. Vehicle System Dynamics, 47(8), 983–1022. https://doi.org/10.1080/00423110903078794
- Betterton, J. G., Hackett, K. C., Ashill, P. R., Wilson, M. J., Woodcock, I. J., & Tilman, C. P. (2000). Laser Doppler anemometry investigation on Sub boundary layer vortex generators for flow control. 10th Intl. Symp. on. 10th Intl. Symp. on, Lisbon. July 10–13 2000.
- CEN European Standard. (2018). Railway applications - Aerodynamics Part 6: Requirements and test procedures for cross wind assessment. BSI British Standards. https://doi.org/10.3403/02796008U.
- Chen, H., Reuss, D. L., Hung, D. L. S., & Sick, V. (2013). A practical guide for using proper orthogonal decomposition in engine research. International Journal of Engine Research, 14(4), 307–319. https://doi.org/10.1177/1468087412455748
- Chen, X, Zhong, S, Ozer, O, & Weightman, A. (2022a). Control of afterbody vortices from a slanted-base cylinder using sweeping jets. Physics of Fluids, 34(7), 0785. http://dx.doi.org/10.1063/5.0094565
- Chen, X, Zhong, S, Ozer, O, & Weightman, A. (2022b). Drag reduction of a slanted-base cylinder using sweeping jets. Physics of Fluids, 34(10), 491. http://dx.doi.org/10.1063/5.0118386
- Davidson, L. (2009). Large Eddy simulations: How to evaluate resolution. International Journal of Heat and Fluid Flow, 30(5), 1016–1025. https://doi.org/10.1016/j.ijheatfluidflow.2009.06.006
- Diedrichs, B., Sima, M., Orellano, A., & Tengstrand, H. (2007). Crosswind stability of a high-speed train on a high embankment. Proceedings of the Institution of Mechanical Engineers, Part F: Journal of Rail and Rapid Transit, 221(2), 205–225. https://doi.org/10.1243/0954409JRRT126
- Dong, X., Wang, Y., Chen, X., Dong, Y., Zhang, Y., & Liu, C. (2018). Determination of epsilon for Omega vortex identification method. Journal of Hydrodynamics, 30(4), 541–548. https://doi.org/10.1007/s42241-018-0066-x
- Du, H., Zhou, D., Meng, S., & Luo, C. (2022). Effect of vortex generators on the aerodynamic performance of high-speed trains. Flow, Turbulence and Combustion, 109(3), 627–645. https://doi.org/10.1007/s10494-022-00349-3
- East Japan Railway Company. (2022). JR East Group Report INTEGRATED REPORT 2022. https://www.jreast.co.jp/e/environment/pdf_2022/all.pdf.
- Fouatih, O. M., Medale, M., Imine, O., & Imine, B. (2016). Design optimization of the aerodynamic passive flow control on NACA 4415 airfoil using vortex generators. European Journal of Mechanics - B/Fluids, 56, 82–96. https://doi.org/10.1016/j.euromechflu.2015.11.006
- Fujii, T., Maeda, T., Ishida, H., Imai, T., Tanemoto, K., & Suzuki, M. (1999). Wind-Induced accidents of train/vehicles and their measures in Japan. Quarterly Report of RTRI, 40(1), 50–55. https://doi.org/10.2219/rtriqr.40.50
- Gallagher, M., Morden, J., Baker, C., Soper, D., Quinn, A., Hemida, H., & Sterling, M. (2018). Trains in crosswinds – Comparison of full-scale on-train measurements, physical model tests and CFD calculations. Journal of Wind Engineering and Industrial Aerodynamics, 175, 428–444. https://doi.org/10.1016/j.jweia.2018.03.002
- Gao, G., Zhang, J., & Xiong, X.-H. (2014). Location of anemometer along Lanzhou-Xinjiang railway. Journal of Central South University, 21(9), 3698–3704. https://doi.org/10.1007/s11771-014-2353-1
- Gao, H., Liu, T., Gu, H., Jiang, Z., Huo, X., Xia, Y., & Chen, Z. (2021). Full-scale tests of unsteady aerodynamic loads and pressure distribution on fast trains in crosswinds. Measurement, 186, 110152. https://doi.org/10.1016/j.measurement.2021.110152
- Gautier, P.-E., Sacre, C., Delaunay, D., Parrot, M., Dersigny, C., & Bodere, S. (2001). “TGV méditerranée” high speed line safety against cross-winds: A slow-down system based on anemometric measurements and spatial short-term meteorological prediction. Rapport technique, SNCF.
- Gibertini, G., Boniface, J. C., Zanotti, A., Droandi, G., Auteri, F., Gaveriaux, R., & Le Pape, A. (2015). Helicopter drag reduction by vortex generators. Aerospace Science and Technology, 47, 324–339. https://doi.org/10.1016/j.ast.2015.10.004
- Godard, G., & Stanislas, M. (2006). Control of a decelerating boundary layer. Part 1: Optimization of passive vortex generators. Aerospace Science and Technology, 10(3), 181–191. https://doi.org/10.1016/j.ast.2005.11.007
- Gorton, S., Jenkins, L., & Anders, S. (2002). Flow control device evaluation for an internal flow with an adverse pressure gradient. 40th AIAA aerospace sciences meeting & exhibit, Reno, NV, U.S.A. 14 January 2002 - 17 January 2002. American Institute of Aeronautics and Astronautics, Reston, Virigina.
- Hamba, F. (2009). Log-layer mismatch and commutation error in hybrid RANS/LES simulation of channel flow. International Journal of Heat and Fluid Flow, 30(1), 20–31. https://doi.org/10.1016/j.ijheatfluidflow.2008.10.002
- Hemida, H., & Baker, C. (2010). Large-eddy simulation of the flow around a freight wagon subjected to a crosswind. Computers & Fluids, 39(10), 1944–1956. https://doi.org/10.1016/j.compfluid.2010.06.026
- Huo, X.-S., Liu, T.-H., Chen, Z.-W., Li, W.-H., Niu, J.-Q., & Gao, H.-R. (2023). Aerodynamic characteristics of double-connected train groups composed of different kinds of high-speed trains under crosswinds: A comparison study. Alexandria Engineering Journal, https://doi.org/10.1016/j.aej.2022.09.011
- Koike, M., Tsunehisa, N., & Naoki, H. (2004). Research on aerodynamic drag reduction by vortex generators. Mitsubishi Motors Technical Review, 16, 11–16.
- Krajnovic, S. (2009). Shape optimization of high-speed trains for improved aerodynamic performance. Proceedings of the Institution of Mechanical Engineers, Part F: Journal of Rail and Rapid Transit, 223(5), 439–452. https://doi.org/10.1243/09544097JRRT251
- Kuya, Y., Takeda, K., Zhang, X., Beeton, S., & Pandaleon, T. (2009). Flow physics of a race car wing with vortex generators in ground effect. Journal of Fluids Engineering, 131(12), 121103. https://doi.org/10.1115/1.4000423
- Li, T., Liang, H., Zhang, J., & Zhang, J. (2023). Numerical study on aerodynamic resistance reduction of high-speed train using vortex generator. Engineering Applications of Computational Fluid Mechanics, 17(1), e2153925. https://doi.org/10.1080/19942060.2022.2153925
- Li, W., Liu, T., Martinez-Vazquez, P., Chen, Z., Huo, X., Liu, D., & Xia, Y. (2022). Correlation tests on train aerodynamics between multiple wind tunnels. Journal of Wind Engineering and Industrial Aerodynamics, 229, 105137. https://doi.org/10.1016/j.jweia.2022.105137
- Lin, J. C. (2002). Review of research on low-profile vortex generators to control boundary-layer separation. Progress in Aerospace Sciences, 38(4-5), 389–420. https://doi.org/10.1016/S0376-0421(02)00010-6
- Lin, J. C., Howard, F. G., Bushnell, D. M., & Selby, G. V. (1990a). Investigation of several passive and active methods for turbulent flow separation control. 21st fluid dynamics, plasma dynamics and lasers conference, Seattle, WA, U.S.A. 18 June 1990 - 20 June 1990. American Institute of Aeronautics and Astronautics, Reston, Virigina, p. 17.
- Lin, J. C., Howard, F. G., & Selby, G. V. (1990b). Small submerged vortex generators for turbulent flow separation control. Journal of Spacecraft and Rockets, 27(5), 503–507. https://doi.org/10.2514/3.26172
- Liu, C., Wang, Y., Yang, Y., & Duan, Z. (2016a). New omega vortex identification method. Science China Physics, Mechanics & Astronomy, 59(8), https://doi.org/10.1007/s11433-016-0022-6
- Liu, T., Wang, L., Gao, H., Xia, Y., Guo, Z., Li, W., & Liu, H. (2022). Research progress on train operation safety in Xinjiang railway under wind environment. Transportation Safety and Environment, 4, tdac005. https://doi.org/10.1093/tse/tdac005
- Liu, T.-H., Su, X., Zhang, J., Chen, Z.-W., & Zhou, X. (2016b). Aerodynamic performance analysis of trains on slope topography under crosswinds. Journal of Central South University, 23(9), 2419–2428. https://doi.org/10.1007/s11771-016-3301-z
- Liu, W., Guo, D.-L., Zi-Jian, Z., & Guo-Wei, Y. (2020). Study of dynamic characteristics in wake flow of high-speed train based on POD. Journal of the China Railway Society, 42, 49–57.
- Maleki, S., Burton, D., & Thompson, M. C. (2020). On the flow past and forces on double-stacked wagons within a freight train under cross-wind. Journal of Wind Engineering and Industrial Aerodynamics, 206, 104224. https://doi.org/10.1016/j.jweia.2020.104224
- Masoud, M., & Ali, R. M. (2021). 2D and 3D numerical and experimental analyses of the aerodynamic effects of air fences on a high-speed train. Wind and Structures, 32, 539–550. https://doi.org/10.12989/WAS.2021.32.6.539
- Menter, F. R., Kuntz, M., & Langtry, R. (2003). Ten years of industrial experience with the SST turbulence model. Turbulence, Heat and Mass Transfer, 4.
- Mohebbi, M., & Rezvani, M. A. (2018). Two-dimensional analysis of the influence of windbreaks on airflow over a high-speed train under crosswind using lattice Boltzmann method. Proceedings of the Institution of Mechanical Engineers, Part F: Journal of Rail and Rapid Transit, 232(3), 863–872. https://doi.org/10.1177/0954409717699502
- Mohebbi, M., & Safaee, A. M. (2022). The optimum model determination of porous barriers in high-speed tracks. Proceedings of the Institution of Mechanical Engineers, Part F: Journal of Rail and Rapid Transit, 236(1), 15–25. https://doi.org/10.1177/0954409721995323
- Muld, T. W., Efraimsson, G., & Henningson, D. S. (2012a). Flow structures around a high-speed train extracted using Proper Orthogonal Decomposition and Dynamic Mode Decomposition. Computers & Fluids, 57, 87–97. https://doi.org/10.1016/j.compfluid.2011.12.012
- Muld, T. W., Efraimsson, G., & Henningson, D. S. (2012b). Mode decomposition on surface-mounted cube. Flow, Turbulence and Combustion, 88(3), 279–310. https://doi.org/10.1007/s10494-011-9355-y
- Niu, J., Zhou, D., & Liang, X. (2018). Numerical investigation of the aerodynamic characteristics of high-speed trains of different lengths under crosswind with or without windbreaks. Engineering Applications of Computational Fluid Mechanics, 12(1), 195–215. https://doi.org/10.1080/19942060.2017.1390786
- Östh, J., Kaiser, E., Krajnović, S., & Noack, B. R. (2015). Cluster-based reduced-order modelling of the flow in the wake of a high speed train. Journal of Wind Engineering and Industrial Aerodynamics, 145, 327–338. https://doi.org/10.1016/j.jweia.2015.06.003
- Park, H., & Kim, J. (2016). Electromagnetic induction energy harvester for high-speed railroad applications. International Journal of Precision Engineering and Manufacturing-Green Technology, 3(1), 41–48. https://doi.org/10.1007/s40684-016-0006-6
- Schubauer, G. B., & Spangenberg, W. G. (1960). Forced mixing in boundary layers. Journal of Fluid Mechanics, 8((01|1)), 10–32. https://doi.org/10.1017/S0022112060000372
- Shur, M. L., Spalart, P. R., Strelets, M. K., & Travin, A. K. (2008). A hybrid RANS-LES approach with delayed-DES and wall-modelled LES capabilities. International Journal of Heat and Fluid Flow, 29(6), 1638–1649. https://doi.org/10.1016/j.ijheatfluidflow.2008.07.001
- Siemens. (2021). Simcenter STAR-CCM+ 2021.3 User Guide.
- Sirovich, L. (1987). Turbulence and the dynamics of coherent structures. Quarterly of Applied Mathematics, 45, 561–571. https://doi.org/10.1090/qam/910462
- Spalart, P. R., Deck, S., Shur, M. L., Squires, K. D., Strelets, M. K., & Travin, A. (2006). A new version of detached-eddy simulation, resistant to ambiguous grid densities. Theoretical and Computational Fluid Dynamics, 20(3), 181–195. https://doi.org/10.1007/s00162-006-0015-0
- Spalart, P. R., Jou, W.-H., Strelets, M., & Allmaras, S. R. (1997). Comments on the feasibility of LES for wings, and on a hybrid RANS/LES approach, in: First AFOSR International Conference on DNS/LES approach. Advances in DNS/LES, Louisiana Tech University. Greyden Press.
- Th. Tielkes, Ch. Heine, Möller, M., & Driller, J. (2008). A Probabilistic Approach to Safeguard Cross Wind Safety of Passenger Railway Operation in Germany: The new DB Guideline Ril 80704.
- Wang, J., Minelli, G., Dong, T., He, K., Gao, G., & Krajnović, S. (2020). An IDDES investigation of Jacobs bogie effects on the slipstream and wake flow of a high-speed train. Journal of Wind Engineering and Industrial Aerodynamics, 202, 104233. https://doi.org/10.1016/j.jweia.2020.104233
- Wang, Y., & Gui, N. (2019). A review of the third-generation vortex identification method and its applications. Chinese Journal of Hydrodynamics, 34.
- Wortman, A. (1999). Reduction of fuselage form drag by vortex flows. Journal of Aircraft, 36(3), 501–506. https://doi.org/10.2514/2.2484
- Zanotti, A., Droandi, G., Auteri, F., Gibertini, G., & Le Pape, A. (2016). Robustness and limits of vortex generator effectiveness in helicopter drag reduction. Journal of the American Helicopter Society, 61(3), 32007. https://doi.org/10.4050/JAHS.61.032007
- Zhang, J., Gao, G., Liu, T.-H., & Li, Z. (2015). Crosswind stability of high-speed trains in special cuts. Journal of Central South University, 22(7), 2849–2856. https://doi.org/10.1007/s11771-015-2817-y
- Zhang, J, Huang, F, Yu, Y, Han, S, Ding, Y, & Gao, G. (2023). A novel wake flow control method for drag reduction of a high-speed train with vortex generators installing on streamlined tail nose. Physics of Fluids, 35(10), 337. http://dx.doi.org/10.1063/5.0173350