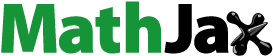
ABSTRACT
Aim
Our aim was to compare the prevalence and load of nine pathobionts in subgingival samples of healthy individuals and periodontitis patients from four different countries.
Methods
Five hundred and seven subgingival biofilm samples were collected from healthy subjects and periodontitis patients in Belgium, Chile, Peru and Spain. The prevalence and load of Eubacterium brachy, Filifactor alocis, Fretibacterium fastidiosum, Porphyromonas endodontalis, Porphyromonas gingivalis, Selenomonas sputigena, Treponema denticola, Tannerella forsythia and Treponema socranskii were measured by quantitative PCR.
Results
The association with periodontitis of all species, except for T. socranskii, was confirmed in all countries but Peru, where only P. endodontalis, P. gingivalis and T. denticola were found to be significantly associated. Moreover, most species showed higher loads at greater CAL and PPD, but not where there was BOP. Through Principal Component Analysis, samples showed clearly different distributions by diagnosis, despite observing a smaller separation in Peruvian samples.
Conclusions
Unlike prevalence, relative load was found to be a reliable variable to discriminate the association of the species with periodontitis. Based on this, F. alocis, P. endodontalis, P. gingivalis, T. denticola and T. forsythia may be biomarkers of disease in Belgium, Chile and Spain, due to their significantly higher abundance in periodontitis patients.
Introduction
The oral microbiome comprises more than 700 microorganisms [Citation1]. In periodontally healthy individuals, the host and its oral microbiota maintain a symbiotic relationship [Citation2]. However, a number of disturbances can alter the host-microbiota equilibrium [Citation2,Citation3] and interrupt the microbial homeostasis, and if extended over time, may lead to periodontitis [Citation2,Citation4].
The use of methods like culture or DNA–DNA hybridisation first associated periodontitis with bacteria such as Porphyromonas gingivalis, Tannerella forsythia and Treponema denticola [Citation5]. Soon thereafter, species like Filifactor alocis, Selenomonas sputigena, Porphyromonas endodontalis and Treponema socranskii were also related to periodontitis using PCR [Citation6]. Nonetheless, the advent of high-throughput sequencing (HTS) allowed for the comparison of whole microbial communities, confirming previous observations [Citation7,Citation8], stressing the importance of species like F. alocis or P. endodontalis [Citation9,Citation10], and describing new putative periodontopathogens such as Fretibacterium fastidiosum and Eubacterium brachy [Citation9,Citation10]. The use of HTS, mainly 16S metagenomics, unveiled a complex scenario where periodontitis was seen to result not from individual pathogens but rather from polymicrobial synergy and dysbiosis [Citation3,Citation4,Citation7]. Most of the above-mentioned publications are single-country studies from a rather low number of countries (to our knowledge, about 55% used samples from the USA, 14% from Brazil, and the remaining percentage of publications used samples from the following countries in an equal distribution: Germany, the UK, Spain, Italy, Sweden, Turkey, China, Japan, Korea, Taiwan, Saudi Arabia, United Arab Emirates, India and Chile) [Citation7,Citation9,Citation11]. However, it should be considered that microbial composition can be influenced by host genotype, environment and habits [Citation9]. In fact, one study showed significant microbial divergence between African Americans and Caucasians who shared geographic location, lifestyle and nutrition [Citation12]. Therefore, different human populations and ethnicities can harbour different microbiota.
Recently, we used 16S metagenomics to characterise the microbiota associated with periodontitis in subgingival samples from Belgium, Chile, Peru and Spain (data not shown). Nonetheless, 16S metagenomics is based on sequencing the subregions of the 16S rRNA gene, which can result in misclassification at the species-level [Citation13,Citation14]. Indeed, quantitative PCR (qPCR) would be a more suitable approach to analyse particular species, because it is a highly specific and sensitive method [Citation8]. Therefore, this study used qPCR to add to our 16S metagenomics analysis by determining the degree of association between periodontitis and several species in Belgium, Chile, Peru and Spain. Based on a literature search [Citation5,Citation6,Citation8,Citation10,Citation15], nine species were selected for the analysis (E. brachy, F. alocis, F. fastidiosum, P. endodontalis, P. gingivalis, S. sputigena, T. denticola, T. forsythia and T. socranskii). To our knowledge, this is the first study aiming to assess and compare the degree of association of certain bacteria with periodontitis in different countries.
Methods
Subjects and sample collection
This study was designed as a case–control trial that included healthy subjects (HS) and periodontitis patients (PP). Volunteers were recruited from 2017 to 2019 among those attending the dental clinics at Katholieke Universiteit Leuven (Belgium), Pontificia Universidad Católica de Chile (Chile), Universidad Científica del Sur (Peru) and Universitat Internacional de Catalunya (Spain). The design was approved in advance by their corresponding Ethics Committees, which assigned the study numbers S60696 (Belgium), 180111004 (Chile), 002-DACE-DAFCS (Peru) and ODO-2014-01 (Spain), all complying with the Declaration of Helsinki. All participants gave their informed consent before being included in the study.
The volunteers were grouped as HS (individuals never diagnosed with periodontitis and free of gingivitis) or PP (patients diagnosed with stage II–IV and grade B or C generalised periodontitis according to the latest classification) [Citation16–18]. Healthy periodontium was defined as non-erythematous and non-oedematous gingiva, absence of bleeding on probing (BOP) or less than 10% of sites with BOP, and no probing pocket depths (PPD) of ≥3 mm, in a non-reduced or reduced periodontium. Periodontitis defined as stage II–IV and grade B or C was diagnosed as interdental clinical attachment loss (CAL) at sites with ≥3 mm, radiographic bone loss of 15% or higher of root length, and a maximum PPD of ≥5 mm. Patients diagnosed with gingivitis, which is defined as >10% of sites with bleeding on probing and PPDs of no more than 3 mm, in a non-reduced or reduced periodontium, were not included in the study [Citation18]. The inclusion criteria comprised systemic health, non-smokers or light smokers (<10 cigarettes/day), retention of at least 18 natural teeth, no previous periodontal treatment, no antibiotic intake in the previous 6 months, no anti-inflammatory intake in the previous 4 months, no regular use of oral antiseptics, and no status of pregnancy or breastfeeding.
The demographic and clinical parameters recorded were age, gender, PPD, CAL and BOP. The probing depth and gingival recession/overgrowth (with the cementoenamel junction [CEJ] as a reference point) were measured to the nearest 1 mm (buccally and orally of each root, and at each approximal site, both buccally and orally) by means of a periodontal probe. CAL was calculated using the sum of the PPD and the recession. BOP was evaluated 20 s after probing the depth of the pocket; the scores were 0 (absent) and 1 (present) [Citation19]. Subgingival samples were taken from a total of 4 sites per individual using sterile endodontic paper points (size 30; 2 paper points per site), which were inserted for 30 s in the deepest pocket of each quadrant, following isolation and supragingival biofilm removal. The paper points were pooled in 2 ml screw cap microcentrifuge tubes, frozen at −80°C and shipped without interrupting the cold chain to the DENTAID Research Center (Spain). Similarly, the values of CAL and PPD of the four sites were averaged for each individual, and BOP was marked as positive in the presence of at least one bleeding site.
Quantitative PCR
The amount of each bacterial species and of eubacteria was evaluated by qPCR using specific primers (Life Technologies) and TaqMan probes (Life Technologies or Roche; Table S1). First, DNA was purified with the QIAamp DNA Mini Kit (Qiagen GmbH) following the manufacturer’s instructions for buccal swabs. Then, reactions were performed as previously described [Citation20], and the resulting crossing points were transformed to number of copies/µl through plasmid standard curves. Each standard plasmid was prepared by cloning the target PCR products in pGEMT [Citation21] with the specific primers from Table S1, using DNA from E. brachy DSM 3990, F. alocis ATCC 35,896, F. fastidiosum DSM 25,557, P. endodontalis ATCC 35,406, P. gingivalis ATCC 33,277, S. sputigena ATCC 35,185, T. forsythia ATCC 43,037, T. denticola DSM 14,222 and T. socranskii ATCC 35,534 as templates. The number of copies/µl was estimated using a Nanodrop ND-1000 UV – vis spectrophotometer (Nanodrop Technologies).
Eubacterial load was used to normalise the data between individuals. The standard curve was prepared as in Àlvarez et al. [Citation20], using DNA from Streptococcus gordonii ATCC 49,818, Veillonella parvula NCTC 11,810, Actinomyces naeslundii DSM 17,233, Fusobacterium nucleatum DSM 20,482 and P. gingivalis ATCC 33,277 as templates.
Statistical analysis
Data processing and statistical analysis were performed in R v4.0.2 [Citation22]. The additional R packages used were tidyverse [Citation23], ggpattern [Citation24] and Rmisc [Citation25] to transform and plot the data and to calculate statistical parameters; gvlma [Citation26] to determine the normal distribution of each variable; car [Citation27] and emmeans [Citation28] for the statistical analysis; and factoextra [Citation29] to plot Principal Component Analyses (PCA) from multivariant analyses.
Prior to the statistical analysis, when a sample tested negative for a certain species, its concentration was imputed to half the value considered its limit of detection [Citation30]. Then, data was transformed to log10 scale, and relative bacterial load () was created to adjust for differences in the total bacterial quantity per sample. CAL and BOP were transformed to categorical variables according to several publications [Citation16,Citation31,Citation32]: samples were grouped into Low CAL (≤3 mm), Medium CAL (3–5 mm) and High CAL (≥5 mm); Low PPD (≤3 mm), Medium PPD (3–6 mm) and High PPD (≥6 mm).
The principal response variables were the prevalence and relative load of each species. The principal explanatory variables were country and diagnosis, and the secondary explanatory variables were age, gender, BOP, CAL and PPD. The homogeneity of each secondary explanatory variable through the groups explained by country and condition was explored with Linear Models (LM; age), Generalised Linear Models (GLM; gender and BOP) and Fisher’s exact tests (CAL and PPD). Statistical differences between groups in the principal response variables were assessed with LM (for bacterial load) and GLM (for prevalence). In all tests, alpha was set at 0.05.
The relative load of each of the nine species was also evaluated with multivariant analyses through PCA using the principal explanatory variables as grouping factors.
Results
Demographic and clinical variables
Data from the 507 participants was sorted according to country of origin and diagnosis (38 HS and 38 PP from Belgium, 38 HS and 43 PP from Chile, 64 HS and 108 PP from Peru, and 58 HS and 120 PP from Spain). Gender was found to be homogenous across most groups, but age, BOP, CAL and PPD were statistically different for HS and PP. Nevertheless, differences between subjects of different countries but with the same clinical condition were scarcer ().
Table 1. Demographic and clinical characteristics of the subjects. One site was sampled from each quadrant, calculating the average CAL and PPD per patient, and recording BOP as positive in the presence of at least one bleeding site. Comparisons: periodontitis patients (PP) vs. healthy subjects (HS) overall and within each country, HS between countries, and PP between countries. BOP: Bleeding on Probing, CAL: Clinical Attachment Loss, PPD: Probing Pocket Depth.
Bacterial differences by diagnosis
In most countries, all species, except for T. socranskii, were significantly more prevalent in PP than in HS. E. brachy, F. alocis, P. endodontalis and P. gingivalis in Peruvian samples, and S. sputigena in Chilean samples showed no differences in prevalence related to diagnosis (Figure S1, Table S2). Concerning bacterial load, the total number of eubacteria was significantly higher in PP than in HS (10.72, 13.49, 7.24 and 10.23-fold in Belgium, Chile, Peru and Spain, respectively); thus, the quantity of each species was adjusted by the number of eubacteria. The resulting relative load was significantly higher in PP than in HS, except for T. socranskii, whose load was significantly higher in HS in all countries. Furthermore, S. sputigena showed no differences in Belgium, Chile and Spain; T. denticola showed no differences in Spain and E. brachy, F. alocis, F. fastidiosum and T. forsythia showed no differences in Peru (, Table S2).
Figure 1. Relative bacterial load (ratio between the number of each bacterial species and the number of eubacteria in log10 scale) in subgingival samples from Belgium, Chile, Peru and Spain. Bar height and whiskers represent the mean and the confidence interval at 95%, respectively. A lower value means a lower relative proportion of that species. Only cases with no significant differences between healthy subjects (light grey) and periodontitis patients (dark grey) are shown in striped bars.

Bacterial differences by country
In HS, Peruvian samples showed a significantly higher prevalence of most species than the other countries. Conversely, Belgium displayed the lowest prevalence in nearly all species although not always significantly. However, in PP, the prevalence of all species except for S. sputigena and T. socranskii (Figure S2, Table S3) was similar between countries. Regarding relative bacterial load, the quantity of most of the species was low in Belgian samples from HS and high in Peruvian samples from HS. The relative load of most species was low in Peruvian samples from PP compared to the other countries (, Table S3).
Figure 2. Differences in relative bacterial load (ratio between the load of each species and the number of eubacteria in log10 scale) between countries. B: Belgium; C: Chile; P: Peru; S: Spain. Bar height and whiskers represent the mean and the confidence interval at 95%, respectively. A lower value means a lower relative proportion of that species. Within each species and diagnosis, the countries with a significantly different bacterial load are represented in different solid tones of grey. Countries with striped bars show no significant differences in bacterial load compared to the countries with the solid bars that share the colours of the stripes (within the same species and diagnosis). Moreover, the striped bars with a different pattern of greys are significantly different from one another.

Differences between species
In HS, T. socranskii was among the least prevalent species in all countries. On the other hand, E. brachy was among the most prevalent species. Depending on the country, P. endodontalis, P. gingivalis, F. alocis and S. sputigena also showed a high prevalence. In PP, T. socranskii exhibited the lowest prevalence, while all other species showed high prevalence (Figure S3, Table S4). In terms of bacterial load, F. fastidiosum, S. sputigena and T. forsythia were mostly present in low numbers in HS, while E. brachy, T. denticola and T. socranskii were usually found in high numbers. In PP, F. fastidiosum, S. sputigena and T. socranskii were found in lower numbers than the other species (, Table S4).
Figure 3. Statistical differences in the number of bacteria (log10(copies/µl) between species (Ff, F. fastidiosum; Ss: S. sputigena; Ts: T. socranskii; Tf: T. forsythia; Eb: E. brachy; Pe: P. endodontalis; Pg: P. gingivalis; Fa: F. alocis; Td: T. denticola) within each country and diagnosis. Bar height and whiskers represent the mean and the confidence interval at 95%, respectively. Within each country and diagnosis, the species with a significantly different number are represented in different solid tones of grey. Species with striped bars are not significantly different than the species with the solid bars that share the colours of the stripes (within the same country and diagnosis). Moreover, the striped bars with a different pattern of greys are significantly different from one another.

Bacterial differences by clinical conditions
Overall, all species, except S. sputigena and T. socranskii, showed a significantly higher relative load and prevalence in the presence of BOP. However, few comparisons were found to be significant by country and/or diagnosis. In HS, E. brachy and F. fastidiosum were found in higher numbers with BOP. Moreover, T. denticola was observed in higher numbers in PP with BOP. Also, in the presence of BOP, F. alocis showed higher numbers in Chilean HS, while E. brachy, F. fastidiosum and P. endodontalis did so in Spanish HS (Table S5).
Regarding CAL, most species presented a significantly higher relative load and prevalence in High and Medium CAL than in Low CAL. Furthermore, F. alocis, F. fastidiosum, P. endodontalis, and T. forsythia were also quantified in higher numbers in High than in Medium CAL. Conversely, T. socranskii was found in higher numbers at lower CAL measures. Within countries, all species, except for T. socranskii and S. sputigena, showed a higher load in High than in Low CAL. Additionally, in Chile and Spain, most species presented a significantly higher load in Medium than in Low CAL (Table S5).
Concerning PPD, whether samples were analysed overall or by country, all species, except for S. sputigena and T. socranskii, showed higher relative loads and/or prevalence at deeper sites. In Belgium, many species showed significant differences in the three possible comparisons, in Chile and Spain the differences were detected mainly in High and Medium PPD vs. Low PPD, while in Peru most differences were observed between Medium and Low PPD (Table S5).
Considering the number of significant comparisons, BOP had a lower influence over the prevalence and load of the nine pathobionts than CAL and PPD. Moreover, the prevalence and the relative load based on the clinical variables of Chilean and Spanish samples showed a more similar trend than the other countries.
Multivariant analysis
A possible relationship between the nine species was explored through PCA. Relative counts were used to compute principal components, showing that most of the variability could be explained by Principal Component 1 (PC1) and, to some extent, PC2. Samples with higher values of PC1 harboured higher relative numbers of all species, except T. socranskii, compared to samples with lower values of PC1. Samples with higher values of PC2 correlated a higher relative quantity of T. socranskii and S. sputigena.
Afterwards, samples were represented in PCA plots grouped by diagnosis or by diagnosis and country. The greatest separation of groups was observed by diagnosis, where PP presented more positive values of PC1 than HS, indicating higher abundances of all species, except T. socranskii in PP. This separation was not observed in PC2, which agreed with the few statistical differences observed in T. socranskii and S. sputigena (Figure S4). Moreover, samples were barely separated by country (not shown). In fact, when clustering by condition and country, Belgium, Chile, and Spain were clearly separated by diagnosis, while healthy Peruvian and diseased Peruvian showed a greater overlap ().
Discussion
This study aimed to determine the association of nine pathobionts with periodontitis in Belgium, Chile, Peru and Spain through qPCR, which is suitable for species-specific identification. Samples were taken from 507 individuals, which, to the best of our knowledge, makes this study one of the largest to date.
The association of each species with periodontitis was measured through its prevalence and quantity. The analysis of the data showed that most of the variability in those measures was explained by diagnosis and to a lesser degree by country of origin. This was apparent in the PCA, where samples were mostly separated according to diagnosis ( and S4). Despite being subordinated to diagnosis, the prevalence and quantity of the studied bacteria were more similar between samples from the same country, which could be imputed to several factors that have been described to shape the oral microbiota [Citation1,Citation33], such as geographical region [Citation34], ethnicity [Citation12], environment [Citation9], lifestyle [Citation33] or diet [Citation33].
The clinical variables presented the expected statistical differences according to diagnosis [Citation16,Citation31,Citation32,Citation35] (). Also, the average age of PP was consistently higher, which was not surprising since the risk of periodontitis increases with age [Citation36]. This increase is thought to be related to changes in lifestyle, diet, decrease in salivary production or decline of host immunity, among others [Citation33,Citation36,Citation37], which facilitate the appearance of new periodontitis localisations or the progression of existing ones. The microbiome in saliva has also been seen to be influenced by age [Citation33,Citation38]. On the other hand, when comparing samples from patients of the same diagnosis from different countries, the demographic and clinical variables also presented some significant differences. Both age and the particularities of each country could influence the statistical analyses of bacterial prevalence and load according to diagnosis and population. Thus, age, gender, and BOP were included in the statistical models to account for the confounding effect of these variables upon microbial prevalence and load, thereby increasing the precision of the statistical estimators calculated by the LMs and GLMs [Citation39], while, because they showed high multicollinearity with the diagnosis, CAL and PPD were excluded [Citation40].
Among the clinical variables, BOP showed the lowest influence on the studied microbiota (table S5). BOP is an indicator of local inflammation, which explains why several studies have attempted to relate bleeding to certain bacteria. However, the results are controversial even between the members of the red complex [Citation5,Citation10,Citation41,Citation42]. In our study, overall (n = 507 samples), all species, except T. socranskii and S. sputigena, were significantly associated with bleeding. However, by diagnosis and/or country, where the sample size was lower, few results were significant, which could be due to the elevated interindividual variability in bleeding sites observed elsewhere [Citation42].
Under the concept of predictive medicine, oral microbiota has been proposed as a biomarker of periodontitis. Other factors, like cytokines, have been used to build predictive models of periodontitis [Citation43]. Therefore, microbiota may serve as an indicator of sites of active disease and contribute to an early diagnosis of periodontitis, thereby allowing for the prevention of severe tissue damage [Citation2,Citation44,Citation45]. However, since well-established periodontopathogens like P. gingivalis have been found in periodontally healthy individuals [Citation3], diagnosis should not rely on the detection of a single species [Citation3]. In fact, according to the polymicrobial synergy and dysbiosis hypothesis, diagnosis should be based on the detection of a set of species rather than of a specific pathogen [Citation2,Citation3]. Therefore, the results from verifying the degree of association with periodontitis of the nine studied species could be used to come up with sets of biomarkers. The suitability of these sets to build predictive models that would contribute to prevention and early diagnosis would be evaluated in a future study. All the species, except for T. socranskii and S. sputigena, showed more than 70% prevalence in periodontitis. However, all these bacteria were also found in health, and only in Belgium three species presented a prevalence of lower than 30% in health (F. alocis, F. fastidiosum and P. gingivalis). Thus, the presence of the studied species may not be a suitable marker of disease. By contrast, bacterial load, which is a continuous variable, could correlate better with the presence of periodontitis. In fact, most species showed a significantly higher relative load in PP (), though the numerical difference between health and disease varied depending on the country (Table S2). In Peru, only P. gingivalis seemed to show sufficient differences between PP and HS to be used as a marker of disease. Instead, in the other countries, the significantly higher relative load in periodontitis of F. alocis, P. endodontalis, P. gingivalis and T. forsythia, adding T. denticola in Belgium and Chile, makes them suitable for the study of biomarkers that could be introduced in a predictive model of disease (Table S2). It is worth noting that these five species are associated with periodontitis in most studies [Citation5,Citation6,Citation8,Citation10,Citation15] and include the three recognised periodontopathogens of the red complex [Citation5] along with F. alocis, which has gained recognition as a periodontopathogen in recent years [Citation7]. In case these five species ended up not serving as a reliable prediction model of periodontitis, E. brachy and F. fastidiosum could be included in the model to increase its accuracy, though the difference in quantity thereof between health and periodontitis was less than 10-fold in Chile and Spain (Table S2).
Our results suggest that some of the species most commonly associated with periodontitis [Citation5,Citation6,Citation8,Citation10,Citation15] (typically shown in studies from the USA and Europe) may not be suitable biomarkers of periodontal disease in countries under-represented in the literature, such as Peru. Despite being located to the south of Peru, Chile is one of the more westernised countries in South America [Citation46], which may explain its similarities with Belgium and Spain, especially with the latter. Peruvian individuals may harbour different microbiota, shaped by ethnicity, diet, lifestyle, etc. [Citation12,Citation33,Citation34]. However, to the best of our knowledge, there are no previous studies into the association of bacteria with periodontitis using Peruvian samples. Furthermore, the first part of this project, which aimed to characterise the subgingival microbiota in health and periodontitis of the four countries through 16S metagenomics, found a higher richness (Chao1 index) in Peruvian periodontitis samples than in those from the other countries, and a higher degree of complexity of the co-occurrence microbiome network of both healthy and periodontitis Peruvian samples (data not shown). Therefore, within the frame of the polymicrobial synergy and dysbiosis hypothesis [Citation3,Citation4,Citation7], certain roles or genes related to periodontitis may be fulfilled by pathobionts not included in this study that might be either more common or only present in Peruvian oral microbiota.
Based on samples from all countries, except for those from Peru, our study has confirmed by qPCR the association with periodontitis of E. brachy, F. alocis, F. fastidiosum, P. endodontalis, P. gingivalis, T. denticola and T. forsythia, which agrees with previous observations [Citation5,Citation7,Citation9,Citation47–49]. Of these species, F. fastidiosum has been recently proposed as a periodontopathogen [Citation7,Citation9,Citation47] although, to our knowledge, only one study [Citation50] has previously used qPCR to confirm its association with periodontitis. Our study also corroborated the association of E. brachy with periodontitis in Belgium, Chile and Spain. This species has been mentioned in only a few studies [Citation10,Citation49], since most refer to the genus Eubacterium or to species such as E. saphenum or E. nodatum [Citation7,Citation35,Citation45]. Moreover, we found a high prevalence of S. sputigena in PP, which agrees with previous studies that relate this species to periodontitis [Citation6,Citation10,Citation51] and the potential proinflammatory role of its lipopolysaccharides [Citation51]. However, to the best of our knowledge, there are no studies quantifying S. sputigena, which in our study did not show differences when comparing HS and PP.
Surprisingly, T. socranskii showed no association with periodontitis, while other authors found it to be more prevalent and/or abundant in periodontitis using 16S metagenomics [Citation10,Citation49], which is not reliable at the species level [Citation13]. In fact, Camelo-Castillo et al. associated T. socranskii with health by 16S metagenomics [Citation35]. Other HTS studies identified Treponema in periodontitis, but this genus also contains other species associated to periodontitis such as T. denticola and T. lecithinolyticum [Citation7,Citation51], hence the importance of confirming the results from exploratory methods like 16S metagenomics.
This study is not without limitations. Although all the examiners followed the same criteria [Citation52], they could not be calibrated, which may have resulted in variability between countries in the measures of the clinical outcomes. The most evident result is the recruitment of a lower number of PP with High CAL and High PPD in Peru, which may explain some of the differences with the other countries. This could be related to the statistically lower average age of the Peruvian periodontitis patients or to an intrinsic characteristic of this population. Furthermore, the inclusion criteria used during recruitment were focused on the diagnosis, resulting in some heterogeneity between the groups in the demographic variables. Nevertheless, demographic and clinical variability was corrected by adding such variables in the statistical linear models.
In conclusion, using methods like qPCR should be considered for confirming at species-level the association with periodontitis of taxa described by 16S metagenomics. Moreover, counts should be adjusted by the total number of bacteria to account for differences between samples. Following this strategy, our study has confirmed the association with periodontitis of most of the bacteria analysed. Furthermore, we have observed some differences between countries, which highlights the importance of using caution when extrapolating results from some populations to others. Besides, prevalence of the species has proven not to be suitable for predicting disease; nonetheless, their quantities allowed us to propose five candidate biomarkers that could be applied in Belgium, Chile and Spain. These results show that a world-wide mapping of the oral microbiota might serve as a tool to find potential biomarkers that might help practitioners to predict periodontitis and provide new targets for future therapies.
Author’s contribution
Conceptualisation: RL and VB; Methodology: GA, RL and VB; Resources: CM, EH, GM, IL, JN, LI, MJC and WT; Investigation: GA and SI; Formal analysis: GA; Visualisation: GA; Writing – original draft preparation: GA; Writing – review and editing: AA, CM, EH, GA, GM, IL, JN, LI, MJC, RL, SI, VB, WT. All authors gave their final approval and agreed to be accountable for all aspects of the work.
The datasets generated during the current study are available from the corresponding author on reasonable request.
Supplemental Material
Download MS Word (5 MB)Acknowledgments
We would like to thank Mrs Ann Bangle for her help in reviewing the language.
Disclosure statement
The authors declared the following potential conflicts of interest: Dr Nart received research grants through the Universitat Internacional de Catalunya from Klockner Implants and Straumann group; lecture fees from Straumann group, Oral-B and KIN Laboratories; and consultant fees from Klockner Implants. All other authors declared no potential conflicts of interest with respect to the authorship and/or publication of this article.
Supplementary material
Supplemental data for this article can be accessed online at https://doi.org/10.1080/20002297.2023.2188630.
Additional information
Funding
References
- Kumar PS, Dabdoub SM, Ganesan SM. Probing periodontal microbial dark matter using metataxonomics and metagenomics. Periodontol 2000. 2021;85(1):12–10.
- Belibasakis GN, Bostanci N, Marsh PD, et al. Applications of the oral microbiome in personalized dentistry. Arch Oral Biol. 2019;104(March):7–12.
- Hajishengallis G. Periodontitis: from microbial immune subversion to systemic inflammation. Nat Rev Immunol. 2015;15(1):30–44.
- Radaic A, Kapila YL. The oralome and its dysbiosis: new insights into oral microbiome-host interactions. Comput Struct Biotechnol J. 2021;19:1335–1360.
- Socransky SS, Haffajee AD, Cugini MA, et al. Microbial complexes in subgingival plaque. J Clin Periodontol. 1998;25(2):134–144.
- Kumar PS, Griffen AL, Barton JA, et al. New bacterial species associated with chronic periodontitis. J Dent Res. 2003;82(5):338–344.
- Feres M, Retamal‐valdes B, Gonçalves C, et al. Did Omics change periodontal therapy? Periodontol 2000. 2021;85(1):182–209.
- Griffen AL, Beall CJ, Campbell JH, et al. Distinct and complex bacterial profiles in human periodontitis and health revealed by 16S pyrosequencing. Isme J. 2012;6(6):1176–1185.
- Teles F, Wang Y, Hajishengallis G, et al. Impact of systemic factors in shaping the periodontal microbiome. Periodontol 2000. 2021;85(1):126–160.
- Abusleme L, Dupuy AK, Dutzan N, et al. The subgingival microbiome in health and periodontitis and its relationship with community biomass and inflammation. Isme J. 2013;7(5):1016–1025.
- Abu Fanas S, Brigi C, Varma SR, et al. The prevalence of novel periodontal pathogens and bacterial complexes in stage II generalized periodontitis based on 16S rRNA next generation sequencing. J Appl Oral Sci. 2021;29:e20200787.
- Mason MR, Nagaraja HN, Camerlengo T, et al. Deep sequencing identifies ethnicity-specific bacterial signatures in the oral microbiome. PLoS ONE. 2013;8(10):e77287. Biswas I, ed. doi:10.1371/journal.pone.0077287.
- Johnson JS, Spakowicz DJ, Hong BY, et al. Evaluation of 16S rRNA gene sequencing for species and strain-level microbiome analysis. Nat Commun. 2019;10(1):5029.
- Jeong J, Yun K, Mun S, et al. The effect of taxonomic classification by full-length 16S rRNA sequencing with a synthetic long-read technology. Sci Rep. 2021;11(1):1727.
- Vincent JW, Falkler WA, Suzuki JB. Systemic antibody response of clinically characterized patients with antigens of eubacterium brachy initially and following periodontal therapy. J Periodontol. 1986;57(10):625–631.
- Papapanou PN, Sanz M, Buduneli N, et al. Periodontitis: consensus report of workgroup 2 of the 2017 world workshop on the classification of periodontal and peri-implant diseases and conditions. J Periodontol. 2018;89(March):S173–182.
- Caton JG, Armitage G, Berglundh T, et al. A new classification scheme for periodontal and peri-implant diseases and conditions - Introduction and key changes from the 1999 classification. J Clin Periodontol. 2018;45:S1–8.
- Tonetti MS, Greenwell H, Kornman KS. Staging and grading of periodontitis: framework and proposal of a new classification and case definition. J Periodontol. 2018;89:S159–172.
- Cafiero C, Matarasso S. Predictive, preventive, personalised and participatory periodontology: ‘the 5ps age’ has already started. EPMA Journal. 2013;4(1):16.
- Àlvarez G, González M, Isabal S, et al. Method to quantify live and dead cells in multi-species oral biofilm by real-time PCR with propidium monoazide. AMB Express. 2013;3(1). Published online. DOI:10.1186/2191-0855-3-1.
- Sambrook J, Fritsch EF, Maniatis T. Molecular cloning - a laboratory manual. 2nd ed. New York: Cold Spring Harbor Laboratory Press; 1987.
- R Core Team. R: a language and environment for statistical computing. R Foundation for Statistical Computing; 2022. Published online. Available from: https://www.R-project.org/
- Wickham H, Averick M, Bryan J, et al. Welcome to the Tidyverse. J Open Source Softw. 2019;4(43):1686.
- Cheng M. ggpattern. Available from: https://coolbutuseless.github.io/package/ggpattern/index.html
- Hope RM. Rmisc. R package version 15; 2013. Published online.
- Peña EA, Slate EH. Global validation of linear model assumptions. J Am Stat Assoc. 2006;101(473):341–354.
- Fox J, Weisberg S. An {R} companion to applied regression. 3rd ed. Sage; 2019. Available from: https://socialsciences.mcmaster.ca/jfox/Books/Companion/
- Lenth RV, Buerkner P, Herve M, et al. Emmeans. R package version 155; 2021. Published online.
- Kassambara A, Mundt F Factoextra. R package version 107; 2020. Published online.
- Richardson DB, Ciampi A. Effects of exposure measurement error when an exposure variable is constrained by a lower limit. Am J Epidemiol. 2003;157(4):355–363.
- Sattari M, Taheri RA, ArefNezhad R, et al. The expression levels of MicroRNA-146a, RANKL and OPG after non-surgical periodontal treatment. BMC Oral Health. 2021;21(1):523.
- Chapple ILC, Mealey BL, van Dyke TE, et al. Periodontal health and gingival diseases and conditions on an intact and a reduced periodontium: consensus report of workgroup 1 of the 2017 world workshop on the classification of periodontal and peri-implant diseases and conditions. J Periodontol. 2018;89:S74–84.
- Zaura E, Pappalardo VY, Buijs MJ, et al. Optimizing the quality of clinical studies on oral microbiome: a practical guide for planning, performing, and reporting. Periodontol 2000. 2021;85(1):210–236.
- Takeshita T, Matsuo K, Furuta M, et al. Distinct composition of the oral indigenous microbiota in South Korean and Japanese adults. Sci Rep. 2015;4(1):6990.
- Camelo-Castillo AJ, Mira A, Pico A, et al. Subgingival microbiota in health compared to periodontitis and the influence of smoking. Front Microbiol. 2015;6. DOI:10.3389/fmicb.2015.00119.
- Billings M, Holtfreter B, Papapanou PN, et al. Age-dependent distribution of periodontitis in two countries: findings from NHANES 2009 to 2014 and SHIP-TREND 2008 to 2012. J Periodontol. 2018;89:S140–158.
- Xu X, He J, Xue J, et al. Oral cavity contains distinct niches with dynamic microbial communities. Environ Microbiol. 2015;17(3):699–710.
- Schwartz JL, Peña N, Kawar N, et al. Old age and other factors associated with salivary microbiome variation. BMC Oral Health. 2021;21(1):490. DOI:10.1186/s12903-021-01828-1
- Faraway JJ. Linear models with R. 2nd ed. Boca Ratón: CRC Press, Taylor & Francis Group; 2015. (Dominici F, Faraway J, Tanner M, and Zidek J, editors).
- Rawlings JO, Pantula SG, Dickey DA. Collinearity. In: Casella G, Fienberg S, and Olkin I, editors. Applied regression analysis : a research tool. Second ed. Springer. 1998; p. 433–462.
- Savitt ED, Darack AP, Killoy WJ, et al. Site selection criteria for microbiological testing of periodontal microorganisms. J Periodontol. 1991;62(9):558–561.
- Camelo-Castillo A, Novoa L, Balsa-Castro C, et al. Relationship between periodontitis-associated subgingival microbiota and clinical inflammation by 16S pyrosequencing. J Clin Periodontol. 2015;42(12):1074–1082.
- Tomás I, Arias-Bujanda N, Alonso-Sampedro M, et al. Cytokine-based predictive models to estimate the probability of chronic periodontitis: development of diagnostic nomograms. Sci Rep. 2017;7(1):1–15.
- Yost S, Duran-Pinedo AE, Teles R, et al. Functional signatures of oral dysbiosis during periodontitis progression revealed by microbial metatranscriptome analysis. Genome Med. 2011;7(1). DOI:10.1186/s13073-015-0153-3
- He J, Li Y, Cao Y, et al. The oral microbiome diversity and its relation to human diseases. Folia Microbiol (Praha). 2015;60(1):69–80.
- United Nations Development Programme. United nations development programm. Human development report 2020; 2020 [cited 2022 Aug 17]. Available from: https://hdr.undp.org/content/human-development-report-2020
- Pérez-Chaparro PJ, Gonçalves C, Figueiredo LC, et al. Newly identified pathogens associated with periodontitis: a systematic review. J Dent Res. 2014;93(9):846–858.
- Lamont RJ, Hajishengallis G. Polymicrobial synergy and dysbiosis in inflammatory disease. Trends Mol Med. 2015;21(3):172–183. Published online. doi:10.1016/j.molmed.2014.11.004.
- Ikeda E, Shiba T, Ikeda Y, et al. Japanese subgingival microbiota in health vs disease and their roles in predicted functions associated with periodontitis. Odontology. 2019;108(2):280–291.
- Ye C, Xia Z, Tang J, et al. Unculturable and culturable periodontal-related bacteria are associated with periodontal inflammation during pregnancy and with preterm low birth weight delivery. Sci Rep. 2020;10(1):15807.
- Hiranmayi K, Sirisha K, Ramoji Rao M, et al. Novel pathogens in periodontal microbiology. J Pharm Bioallied Sci. 2017;9(3):155.
- Armitage GC. Development of a classification system for periodontal diseases and conditions. Ann Periodontol. 1999;4(1):1–6.