ABSTRACT
Objective
Next generation sequencing is commonly used to characterize the microbiome structure. MiSeq is most commonly used to analyze the microbiome due to its relatively long read length. Illumina also introduced the 250 × 2 chip for NovaSeq. The purpose of this study was to compare the performance of MiSeq and NovaSeq in the context of oral microbiome study.
Methods
Total read count, read quality score, relative bacterial abundance, community diversity, and correlation between two platforms were analyzed. Phylogenetic trees were analyzed for Streptococcus and periodontopathogens.
Results
NovaSeq produced significantly more read counts and assigned more operational taxonomic units (OTUs) compared to MiSeq. Community diversity was similar between MiSeq and NovaSeq. NovaSeq were able to detect more unique OTUs compared to MiSeq. When phylogenetic trees were constructed for Streptococcus and periodontopathogens, both platforms detected OTUs for most of the clades.
Conclusion
Taken together, while both MiSeq and NovaSeq platforms effectively characterize the oral microbiome, NovaSeq outperformed MiSeq in terms of read counts and detection of unique OTUs, highlighting its potential as a valuable tool for large scale oral microbiome studies.
Introduction
Microbiota inhabiting the oral cavity play a significant role in overall host health, and dysbiosis within the oral microbiota is often implicated in the pathogenesis of both oral and systemic diseases [Citation1]. The accessibility of the oral microbiota allows for the direct examination of specific sites of interest [Citation2]. Since the mid-2000s, advances in sequencing technology have brought about a revolution in various research fields, providing a powerful tool for the biodiversity analysis of microbial communities [Citation3,Citation4]. Next-generation sequencing (NGS) has found extensive application in dentistry, particularly in numerous studies focusing association of the oral microbiome with diseases such as periodontitis and peri-implantitis [Citation5–7]. The declining cost of sequencing and the increased computing power, coupled with the development of new bioinformatics tools, have made it feasible to conduct large-scale studies, encompassing diverse populations and subgroups within the cohort with unprecedented precision and scale. This scalability enhances the statistical robustness of findings and supports the identification of subtle microbiome variations linked to factors such as age, lifestyle, or health status.
In microbiome studies, DNA sequencing platforms, such as 454 pyrosequencing [Citation8], Illumina [Citation9], and Ion Torrent [Citation10], have been utilized. Recently, Illumina sequencing instruments (e.g. MiSeq, HiSeq and NovaSeq) have become the most widely used instruments for meta-analysis due to their low cost per sequence and high sequencing accuracy [Citation11]. Although MiSeq and NovaSeq are both sequencing platforms developed by Illumina, there are some differences between two platforms. MiSeq uses reversible terminator sequencing chemistry. It is based on sequencing-by-synthesis, where 4-colour fluorescently labeled nucleotides are added one at a time to the growing DNA strand, and the incorporated nucleotide is detected by imaging [Citation12]. NovaSeq also employs reversible terminator chemistry, but it uses 2-colour fluorescently labeled nucleotides and a flow cell that has pre-defined binding spots for target DNA instead of the random lawn used by MiSeq platform [Citation13]. The maximum length and number of reads produced differ between two platforms. MiSeq produces paired 2 × 300bp reads with a high sequencing capacity (7.5–8.5 Gb), equivalent to maximum 50 million paired-end reads. NovaSeq 6000 can generate 2 × 250bp reads with over 20 billion paired-end reads (2400–3000 Gb) per instrument run. Additionally, NovaSeq 6000 System produces high-quality data comparable to MiSeq, using more efficient storage of base calls and quality scores. Due to these advantages, research using Novaseq in microbiome analysis, which requires large amounts of data are gradually increasing [Citation14,Citation15].
In this study, we compared used oral samples from patients with periodontitis simultaneously sequenced by MiSeq and NovaSeq platforms to determine the similarity and difference between two platforms.
Material and methods
Study population and plaque sample collection
Six periodontal pockets per tooth were probed in participants visiting the Department of Periodontology, Section of Dentistry, Seoul National University Bundang Hospital, Seongnam-si, South Korea. Patients with at least one periodontal pocket with a probing pocket depth of 5 mm or more were classified as periodontitis patients, and plaque and buccal swab samples from these patients were used for analysis. Supragingival plaque samples were collected with a curette from the supragingival area with the most plaque, and subgingival plaque was collected with a curette from the area with the deepest pocket depth. Buccal swab samples were collected from mucosa of both cheeks with a sterile micro brush. Participants were requested to refrain from food and oral hygiene (brushing or flossing the teeth) for 2 h before sampling. All subjects gave written consent to participate in this study, and the protocol was approved by the Institutional Review Board of Seoul National University Bundang Hospital (IRB no. B-2004-604-301). Samples from all subjects were collected and stored at − 80°C for subsequent processing.
Extraction of genomic DNA and next generation sequencing
Total DNA was extracted from the buccal and supragingival plaque using a Gram positive DNA purification kit (Lucigen, Biosearch Technology, Novato, CA) following the manufacturer’s instructions. Each sequenced sample was prepared according to the Illumina 16S Metagenomic Sequencing Library protocols to amplify the V1 and V2 region (27F-338 R). The barcoded fusion primer sequences used for amplifications were as follows: 27F:5’- AGA GTT TGA TYM TGG CTC AG −3’, 338 R: 5’- TGC TGC CTC CCG TAG RAG T −3’. The DNA quality was measured by PicoGreen and NanoDrop ND-1000 spectrophotometer (Thermo Fisher Scientific, USA) and stored at − 80°C until use. The purified amplicons were combined in equimolar amounts and subjected to paired-end sequencing using MiSeq (Illumina, San Diego, CA, USA) and NovaSeq (Illumina, San Diego, CA, USA).
Bioinformatic analysis, statistical analysis, and visualization
A total number of 98 corresponding samples were compared in this study. In practice, a total of 98 and 1600 samples were loaded onto the MiSeq and NovaSeq platform, respectively. Basic microbiome analyses have been performed using the QIIME2 (version 2020.6) [Citation16] and associated plugins. To measure alpha diversities, Choa1 index and Shannon’s index method were used. Principal coordinate analysis (PCoA) of the Bray-Curtis distance was performed to determine the community structure using the vegan package v2.3–0 in R software v3.2.1. The species of each OTU was determined by pre-trained Naive Bayes classifier, using Human Oral Microbiome Database (eHOMD) 16S rRNA Extended RefSeq sequences database (version 15.1) [Citation17]. Phylogenetic tree construction by using align-to-tree-mafft-fasttree implemented in QIIME2 and visualized using iTOL [Citation18].
Results
Patient characterization
The demographic characteristics of the 33 subjects are shown in . The median age was 51 years, and 72% were male. The proportion without diabetes and without dental caries was significantly higher.
Table 1. Characterization of clinical samples.
Read counts during preprocessing
The total read counts obtained from NovaSeq sequencing (193,081 ± 91,268) were significantly higher than those obtained from MiSeq sequencing (71,406 ± 35,095). For MiSeq sequencing, the final non-chimeric read count was 29,584 ± 14,078, and the percentage of input to non-chimeric reads was 41.78 ± 3.22%. On the NovaSeq platform, the final non-chimeric read count was 86,593 ± 39,687, and the percentage of input to non-chimeric reads was 45.25 ± 3.30% (). Thus, NovaSeq produced a significantly higher number of input read counts as well as a significantly higher percentage of input to non-chimeric reads. When the correlation between input read counts and non-chimeric read counts for each corresponding sample was plotted, a significant correlation was observed between MiSeq and NovaSeq (). Taken together, NovaSeq outperformed MiSeq in terms of both total read counts and the production of high-quality non-chimeric reads. The observed correlation further suggests that the two platforms are related in their sequencing outputs, providing a basis for potential comparisons and validations between MiSeq and NovaSeq data.
Figure 1. Correlation of read count for each sample between MiSeq and NovaSeq during preprocessing. (a) Number of input read counts for each sample. (b) Number of non-chimeric read counts for each sample.
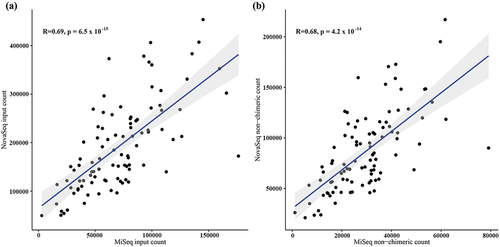
Table 2. Summary of read counts during pre-processing.
Read sequence quality
To evaluate and control the quality of the sequencing data, plots representing the parametric seven-number summary of the quality scores were generated for both forward and reverse reads (). The read length produced by MiSeq and NovaSeq was 301 bases and 251 bases, respectively. For MiSeq, the forward reads produced high quality reads while the reverse reads displayed lower sequencing quality. For NovaSeq, both forward and reverse reads demonstrated consistently high sequencing quality across the entire length. A Q-score of 30 (Q30) corresponds to a 0.1% error rate in base calling and is widely considered a benchmark for high-quality data [Citation19,Citation20]. In MiSeq, the bottom of the boxplot, which represents the 25th percentile of the Q-score, reached Q30 around 260 bps for forward reads and 180 bps for reverse reads. In NovaSeq, the bottom of the boxplot was not detected for both forward and reverse reads (). Taken together, NovaSeq appears to offer more consistently high sequencing quality across both forward and reverse reads compared to MiSeq.
Diversity and taxa assignment
Alpha diversity was measured by the number of operational taxonomic units (OTUs) per sample. The Chao1 index, reflecting richness, and Shannon index, reflecting evenness, were significantly higher (p < 0.001) in the NovaSeq compared to MiSeq (). To compare bacterial community structure, beta-diversity analyses were performed on the corresponding samples. In the Bray Curtis-based principal coordinates analysis (PCoA), most of the samples were closely positioned, suggesting that both platforms produced a similar bacterial composition ().
Figure 3. Bacterial community comparisons between MiSeq and NovaSeq. (a) Alpha diversity was used to describe the microbial richness, evenness and diversity within samples using the Chao1 and Shannon index. Paired t-test was used to identify the group difference. (b) Beta diversity of each sample connected with line. Circle represents MiSeq and triangle represents NovaSeq. Principal coordinate analysis (PCoA) of the Bray-Curtis distance was performed to determine the microbial community structure. ***p < 0.001.
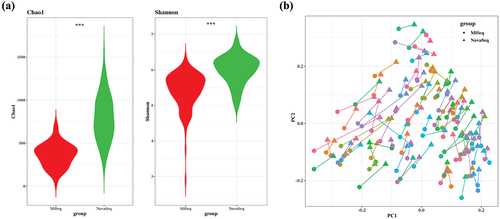
Each OTUs was taxonomically assigned by aligning it to sequences in the HOMD database. A total of 11 phyla were detected in both the MiSeq and NovaSeq platform. At the phylum level, the relative abundance of the seven most abundant phyla (Firmicutes, Bacteroidetes, Proteobacteria, Fusobacteria, Actinobacteria, Saccharibacteria_(TM7), and Spirochaetes) was same in MiSeq and NovaSeq (). At the genus level, NovaSeq detected 132 genera, while MiSeq detected 125 genera. There were several genera that showed some difference in relative abundance between MiSeq and NovaSeq. The relative abundance of Fusobacterium (MiSeq vs NovaSeq: 6.58% vs 4.98%) was lower, whereas the relative abundance of Actinomyces (2.58% vs 3.71%) was higher in NovaSeq compared to MiSeq samples (). At the species level, NovaSeq detected 422 species, while MiSeq detected 397 species. The relative abundance of Capnocytophaga leadbetteri (1.60% vs 1.26%) was lower, whereas the relative abundance of Lautropia mirabilis (2.39% vs 2.96%) and Treponema socranskii (0.77% vs 1.04%) was higher in NovaSeq compared to MiSeq samples (). However, when the overall correlation between relative abundance at the genus level () and species level () were determined, the relative abundance between MiSeq and NovaSeq was significant (p < 0.001). Taken together, despite some taxonomic variations, the strong and statistically significant concordance in relative abundance profiles between the two sequencing platforms underscores the reliability and consistency of MiSeq and NovaSeq in characterizing microbial communities.
Streptococcus and periodontopathogens detected by MiSeq and NovaSeq
As Streptococcus is one of the predominant species in the oral microbiome, we compared the relative abundance of Streptococcus species between MiSeq and NovaSeq. Among the 37 species in the eHOMD reference database, 28 Streptococcus species were detected in the clinical samples. Interestingly, S. sinensis was exclusively detected by MiSeq, while Streptococcus sp. HMT 061 was exclusively detected by NovaSeq. The overall relative abundance for each species was consistent between the two platforms. However, some species showed differences in relative abundance between MiSeq and NovaSeq. Specifically, the relative abundances of S. australis (0.106% vs. 0.0716%) and S. sobrinus (0.004% vs. 0.028%) were higher in NovaSeq than in MiSeq (). When a phylogenetic tree for Streptococcus was constructed based on OTU sequences, most of the trees included OTUs from both platforms, suggesting that both platforms could detect similar OTUs (). When the number of OTUs detected was determined, 60% of OTUs were detected by both platforms, while 32% of OTUs were uniquely detected by NovaSeq and 8% of OTUs were detected by MiSeq ().
Figure 5. Comparison of various Streptococcus species detected by MiSeq and NovaSeq. (a) Relative abundance of various Streptococcus species, (b) Phylogenetic trees based on OTU sequences assigned as Streptococcus species. The tree was reconstructed using the neighbor-joining method from a distance matrix constructed from aligned sequences. (c) Number of OTUs that were detected by either MiSeq or NovaSeq.

Finally, the relative abundance of periodontopathogens and related species was compared, involving a total of 29 selected species. Most of these species were detected in both MiSeq and NovaSeq platforms, indicating a similar overall detection capability. However, variations in relative abundance were observed. MiSeq samples exhibited higher relative abundances of F. nucleatum subsp. animalis (MiSeq vs. NovaSeq: 1.655% vs. 1.153%) and F. nucleatum subsp. vincentii (1.549% vs. 1.236%), while NovaSeq samples showed higher relative abundances of Actinomyces israelii (0.022% vs. 0.041%), A. naeslundii (0.195% vs. 0.300%), Prevotella dentalis (0.035% vs. 0.048%), P. intermedia (0.326% vs. 0.420%), and T. socraskii (0.368% vs. 1.035%) (). Constructing a phylogenetic tree for periodontopathogens based on OTU sequences revealed that most trees included OTUs from both platforms, suggesting that both platforms could detect similar OTUs (). When determining the number of OTUs detected, 58% of OTUs were found by both platforms, while 31% were uniquely detected by NovaSeq, and 10% were detected by MiSeq (). Taken together, the comparison of detecting Streptococcus species and periodontopathogens between MiSeq and NovaSeq platforms revealed overall consistency, with notable detection of more OTUs by NovaSeq.
Figure 6. Comparison of various periodontopathogens detected by MiSeq and NovaSeq. (a) Relative abundance of various periodontopathogens at species level, (b) Phylogenetic trees based on OTU sequences assigned as periodontopathogens. The tree was reconstructed using the neighbor-joining method from a distance matrix constructed from aligned sequences. (c) Number of OTUs that were detected by either MiSeq or NovaSeq.

Discussion
NGS technologies have demonstrated significant advantages in providing cost-effectiveness, accuracy, and high-resolution insights in microbiome studies. However, because there are many technical variables, careful comparison is needed to provide recommendations on the appropriate methodological approach [Citation21]. In this study, we conducted a comparative analysis of the taxonomic composition of 96 oral samples from patients with periodontitis using Miseq and Novaseq to determine their compatibility and suitability for large-scale surveys of oral microbial communities.
Since the number of samples loaded on each platform is not equal, a direct comparison of read counts between MiSeq and NovaSeq may not be appropriate. Therefore, a comparison of practical read counts was conducted. In this study, a total of 98 and 1600 samples were loaded onto the MiSeq and NovaSeq chips, respectively. Among the 1600 samples for NovaSeq, 98 samples were matched with MiSeq samples. Not only was NovaSeq loaded with a significantly larger number of samples simultaneously but also produced a significantly higher number of read counts compared to MiSeq. Additionally, the percentage of input to non-chimeric reads was also significantly higher in NovaSeq, suggesting that NovaSeq is both suitable for large-scale study and can generate sequences with high quality for efficient pairing.
To further assess sequencing quality, the sequencing data’s quality for both forward and reverse reads was plotted. Q-scores on the NovaSeq are calculated using the streamlined real-time analysis process (RTA) 3, a simpler approach compared to the MiSeq’s RTA2 system. In NovaSeq, the RTA3 method was developed to streamline the quality table, employing only three Q-scores correspond to marginal (< Q15), medium (~Q20), and high-quality (> Q30) base calls [Citation22]. This enhances the speed of data processing, reduces data storage requirements, and simplifies Q-scoring. In MiSeq, RTA2 methods employs 41 Q-scores [Citation23]. Thus, when sequencing quality score was plotted, NovaSeq showed a simplified plot but still provided sufficient information to evaluate the sequencing quality. Taken together, NovaSeq can achieve a much greater sequencing depth and generate significantly more data than MiSeq. Deeper sequencing was shown to have advantageous for detecting rare taxonomic groups [Citation9]. Moreover, it was reported that with sufficient sequencing depth, MiSeq cannot detect new sequences, whereas NovaSeq was still finding new sequences [Citation24]. Thus, NovaSeq maybe suitable for detecting greater biological diversity within samples compared to MiSeq.
To compare microbial complexity, alpha and beta diversity was analyzed. In concordance with read counts, alpha diversity within samples was significantly higher in NovaSeq compared to MiSeq. However, beta diversity between the two platforms remained similar. Previous studies have also shown that microbial diversity is not significantly influenced by platform differences [Citation25,Citation26]. Taken together, NovaSeq produced higher alpha diversity but provided compatible beta diversity to MiSeq.
When the correlation of taxa assigned by each platform was analyzed, the relative abundance of each corresponding taxa showed significant correlations at phylum and genus level (). However, at the species level, substantial differences were observed. Firstly, we compared the relative abundance of Streptococcus, which are found in almost every location in the human body and are the dominant species in the human oral cavity and upper respiratory tract. Streptococci are known as a challenging genus to identify at the species level due to high sequence similarity among its species [Citation27,Citation28]. Especially, the mitis group is the largest of the groups found in the oral cavity and species within the mitis group have been challenging to differentiate based on 16S RNA sequence alone, particularly S. oralis and S. mitis [Citation29,Citation30]. Previous studies were reported that the choice of primer sets can impact 16S rRNA amplicon sequencing [Citation31]. Although primers targeting the V3-V4 region of the 16S rRNA gene are currently the most widely used for human microbiome study [Citation32,Citation33], the V1-V2 region has been reported to be more efficient for oral microbiome study [Citation31,Citation34]. In this study, we employed V1-V2 region primers to identify numerous species of Streptococcus to enable a more in-depth analysis of the sequencing data. Among mitis group, S. mitis, S. oralis subsp. dentisani, S. oralis subsp. oralis, and S. oralis subsp. tigurinus were both detected by MiSeq and NovaSeq. It is also important to test to discriminate S. pneumoniae, which is very closely related to S. mitis and S. oralis [Citation35]. Since our clinical samples were collected from periodontitis patients, S. pneumoniae was not detected. It would be interesting to test the primers are efficient in discriminating S. pneumoniae from other mitis group. Phylogenetic tree of Streptococcus was constructed to test if there were any specific trees that were detected by either platform. Similar OTUs were detected by both platforms and 60% of OTUs were detected by both platforms, while NovaSeq uniquely detected 32% of OTUs. Several hypotheses could explain these results. One involves the sequencing quality and the pairing algorithm: higher sequencing quality can lead to more efficient pairing. Given that NovaSeq demonstrates superior sequencing quality towards the end of reads, it should theoretically enable more efficient pairing compared to MiSeq. Another factor could be the sequencing process itself. In MiSeq, the placement of dots is random, which might introduce errors in identifying paired sequences. Conversely, the more uniform placement of dots in NovaSeq could facilitate more efficient matching of paired ends.
Finally, we compared the relative abundance of periodontopathogens including P. gingivalis, Treponema denticola, and Tannerella forsythia. The NovaSeq samples exhibited a lower relative abundance of Fusobacterium species compared to MiSeq samples, whereas Treponema socranskii displayed higher abundance in NovaSeq samples than in MiSeq samples. However, most of the tested taxa showed similar abundance between MiSeq and NovaSeq. In phylogenetic tree analysis, most of the trees were detected by both platforms. Taken together, both platforms showed similar detection performance for Streptococcus and periodontopathogens. Moreover, NovaSeq was superior in detecting more diverse OTUs compared to MiSeq.
While MiSeq is relatively inexpensive, the recommended number of samples to be loaded is around 100. On the other hand, NovaSeq is expensive – almost 10 times the cost of the MiSeq – with the recommended number of samples around 4000. For these reasons, MiSeq remains a suitable platform for a relatively small number of samples in NGS analysis, while NovaSeq is more appropriate for large-scale studies. Additionally, data produced by MiSeq and NovaSeq are compatible for comparison between the two platforms. Further studies are required to confirm their compatibility.
Conclusions
In conclusion, while both MiSeq and NovaSeq platforms effectively characterize the oral microbiome, NovaSeq outperformed MiSeq in terms of read counts and detection of unique OTUs. This study highlights potential compatibility of NovaSeq as a valuable tool for large scale oral microbiome studies.
Disclosure statement
No potential conflict of interest was reported by the author(s).
Data availability statement
The raw sequencing data have been deposited in NCBI GenBank under BioProject ID PRJEB73332.
Additional information
Funding
References
- Baker JL, Mark Welch JL, Kauffman KM, et al. The oral microbiome: diversity, biogeography and human health. Nat Rev Microbiol. 2024;22(2):89–9. PubMed PMID: 37700024. doi: 10.1038/s41579-023-00963-6
- Baker JL, Bor B, Agnello M, et al. Ecology of the oral microbiome: beyond bacteria. Trends Microbiol. 2017;25(5):362–374. PubMed PMID: 28089325. doi: 10.1016/j.tim.2016.12.012
- Unno T. Bioinformatic suggestions on MiSeq-based microbial community analysis. J Microb Biotech. 2015;25(6):765–770. PubMed PMID: 25563415. doi: 10.4014/jmb.1409.09057
- Anslan S, Mikryukov V, Armolaitis K, et al. Highly comparable metabarcoding results from MGI-Tech and Illumina sequencing platforms. PeerJ. 2021;9:e12254. PubMed PMID: 34703674. doi: 10.7717/peerj.12254
- Liu G, Chen F, Cai Y, et al. Measuring the subgingival microbiota in periodontitis patients: comparison of the surface layer and the underlying layers. Microbiol Immunol. 2020;64(2):99–112. Epub 2019/12/04 PubMed PMID: 31793046. doi: 10.1111/1348-0421.12759
- Na HS, Kim SY, Han H, et al. Identification of potential oral microbial biomarkers for the diagnosis of periodontitis. J Clin Med. 2020;9(5). Epub 2020/05/24. PubMed PMID: 32443919; PubMed Central PMCID: PMC7290295. doi: 10.3390/jcm9051549
- Kim HJ, Ahn DH, Yu Y, et al. Microbial profiling of peri-implantitis compared to the periodontal microbiota in health and disease using 16S rRNA sequencing. J Periodontal Implant Sci. 2023;53(1):69. PubMed PMID: 36468472. doi: 10.5051/jpis.2202080104
- Park OJ, Yi H, Jeon JH, et al. Pyrosequencing analysis of subgingival microbiota in distinct periodontal conditions. J Dent Res. 2015;94(7):921–927. PubMed PMID: 25904141. doi: 10.1177/0022034515583531.
- Caporaso JG, Lauber CL, Walters WA, et al. Ultra-high-throughput microbial community analysis on the Illumina HiSeq and MiSeq platforms. Isme J. 2012;6(8):1621–1624. Epub 2012/03/10. PubMed PMID: 22402401; PubMed Central PMCID: PMC3400413. doi: 10.1038/ismej.2012.8
- Junemann S, Prior K, Szczepanowski R, et al. Bacterial community shift in treated periodontitis patients revealed by ion torrent 16S rRNA gene amplicon sequencing. PLOS ONE. 2012;7(8):e41606. Epub 2012/08/08. PubMed PMID: 22870235; PubMed Central PMCID: PMC3411582. doi: 10.1371/journal.pone.0041606
- Dominy SS, Lynch C, Ermini F, et al. Porphyromonas gingivalis in Alzheimer’s disease brains: Evidence for disease causation and treatment with small-molecule inhibitors. Sci Adv. 2019;5(1):eaau3333. epub 2019/02/13. PubMed PMID: 30746447; PubMed Central PMCID: PMC6357742. doi: 10.1126/sciadv.aau3333
- Ravi RK, Walton K, Khosroheidari M. MiSeq: a next generation sequencing platform for genomic analysis. Methods Mol Biol. 2018;1706. PubMed PMID: 29423801. doi: 10.1007/978-1-4939-7471-9_12.
- Zhong Y, Xu F, Wu J. Application of next generation sequencing in laboratory medicine. Ann Lab Med. 2021;41(1):25–43. PubMed PMID: 32829577. doi: 10.3343/alm.2021.41.1.25
- Valles-Colomer M, Blanco-Míguez A, Manghi P, et al. The person-to-person transmission landscape of the gut and oral microbiomes. Nature. 2023;614(7946):125–135. PubMed PMID: 36653448. doi: 10.1038/s41586-022-05620-1
- De Filippis F, Paparo L, Nocerino R, et al. Specific gut microbiome signatures and the associated pro-inflamatory functions are linked to pediatric allergy and acquisition of immune tolerance. Nat Commun. 2021;12(1). PubMed PMID: 34645820. doi: 10.1038/s41467-021-26266-z
- Hall M, Beiko RG. 16S rRNA gene analysis with QIIME2. Methods Mol Biol. 2018;1849:113–129. Epub 2018/10/10. PubMed PMID: 30298251. doi: 10.1007/978-1-4939-8728-3_8
- Wade WG. The oral microbiome in health and disease. Pharmacol Res. 2013;69(1):137–143. Epub 2012/12/04. PubMed PMID: 23201354. doi: 10.1016/j.phrs.2012.11.006
- Letunic I, Bork P. Interactive Tree of Life (iTOL) v5: an online tool for phylogenetic tree display and annotation. Nucleic Acids Research. 2021;49(W1):W293–W296. PubMed PMID: 33885785. doi: 10.1093/nar/gkab301
- Ewing B, Green P. Base-calling of automated sequencer traces using phred. II. Error probabilities. Genome Res. 1998;8(3):186–194. PubMed PMID: 9521922. doi: 10.1101/gr.8.3.186
- Ewing B, Hillier L, Wendl MC. Base-calling of automated sequencer traces using phred. I. Accuracy assessment. Genome Res. 1998;8(3):175–185. PubMed PMID: 9521921. doi: 10.1101/gr.8.3.175
- Clooney AG, Fouhy F, Sleator RD, et al. Comparing apples and oranges?: next generation sequencing and its impact on microbiome analysis. PLOS ONE. 2016;11(2):e0148028. PubMed PMID: 26849217. doi: 10.1371/journal.pone.0148028
- Illumina. NovaSeqTM 6000 System Quality Scores and RTA3 Software. Application Note. 2017.
- Xia J, Gill EE, Hancock RE. NetworkAnalyst for statistical, visual and network-based meta-analysis of gene expression data. Nat Protoc. 2015;10(6):823–844. PubMed PMID: 25950236. doi: 10.1038/nprot.2015.052
- Singer GA, Fahner NA, Barnes JG, et al. Comprehensive biodiversity analysis via ultra-deep patterned flow cell technology: a case study of eDNA metabarcoding seawater. Sci Rep. 2019;9(1). PubMed PMID: 30979963. doi: 10.1038/s41598-019-42455-9
- Kuczynski J, Liu Z, Lozupone C, et al. Microbial community resemblance methods differ in their ability to detect biologically relevant patterns. Nat Methods. 2010;7(10):813–819. Epub 2010/09/08. PubMed PMID: 20818378; PubMed Central PMCID: PMC2948603. doi: 10.1038/nmeth.1499
- Allali I, Arnold JW, Roach J, et al. A comparison of sequencing platforms and bioinformatics pipelines for compositional analysis of the gut microbiome. BMC Microbiol. 2017;17(1):194. Epub 2017/09/15. PubMed PMID: 28903732; PubMed Central PMCID: PMC5598039. doi: 10.1186/s12866-017-1101-8
- Tapp J, Thollesson M, Herrmann B. Phylogenetic relationships and genotyping of the genus Streptococcus by sequence determination of the RNase P RNA gene, rnpB. Int J Syst Evol Microbiol. 2003;53(6):1861–1871. PubMed PMID: 14657115. doi: 10.1099/ijs.0.02639-0
- Kosecka-Strojek M, Sabat AJ, Akkerboom V. Development of a reference data set for assigning Streptococcus and Enterococcus species based on next generation sequencing of the 16S–23S rRNA region. Antimicrob Resist Infect Control. 2019;8(1). PubMed PMID: 31788235. doi: 10.1186/s13756-019-0622-3
- Menon T, Naveenkumar V. Use of 16s rRNA Gene sequencing for the identification of viridans group Streptococci. Cureus. 2023;15(10). PubMed PMID: 38022358. doi: 10.7759/cureus.47125
- Kawamura Y, Hou XG, Sultana F. Determination of 16S rRNA sequences of Streptococcus mitis and Streptococcus gordonii and phylogenetic relationships among members of the genus Streptococcus. Int J Bacteriol. 1995;45(4):882–882. PubMed PMID: 7537076. doi: 10.1099/00207713-45-4-882a.
- Na HS, Song Y, Yu Y, et al. Comparative analysis of primers used for 16S rRNA gene sequencing in oral microbiome studies. Methods Protoc. 2023;6(4):71. PubMed PMID: 37623922. doi: 10.3390/mps6040071
- Lim MY, Hong S, Bang SJ, et al. Gut microbiome structure and association with host factors in a Korean population. mSystems. 2021;6(4). PubMed PMID: 34342532. doi: 10.1128/mSystems.00179-21
- Lee SH, Lee H, You HS, et al. Metabolic pathway prediction of core microbiome based on enterotype and orotype. Front Cell Infect Microbiol. 2023;13. PubMed PMID: 37424791. doi: 10.3389/fcimb.2023.1173085
- Wade WG, Prosdocimi EM. Profiling of oral bacterial communities. J Dent Res. 2020;99(6):621–629. PubMed PMID: 32286907. doi: 10.1177/0022034520914594
- Do T, Jolley KA, Maiden MC, et al. Population structure of Streptococcus oralis. Microbiol. 2009;155(8):2593–2602. PubMed PMID: 19423627. doi: 10.1099/mic.0.027284-0