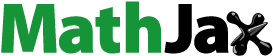
ABSTRACT
Exploring spatial and temporal land-use changes is valuable for local governments to address issues of sustainability and planning policy where urbanization and industrialization are taking place. Besides anthropogenic effects, natural driving forces like climate change may also affect sustainability. However, such relationships have not been studied minutely. Hence, this study first investigates the land-use changes and their relationship with land surface temperature (LST) for the Shazand Watershed, Iran, in 1986, 1998, 2008, and 2016 coincided with supplementary industrialization stages. Furthermore, the relations among LST and other biophysical parameters, including Normalized Difference Vegetation Index (NDVI), Normalized Difference Buildup Index (NDBI), and Normalized Difference Water Index (NDWI), were analyzed, and corresponding variations were explored. The results indicated that the mean LST of the study watershed has an increasing trend from 1986 to 2008 due to land-use change and drought intensification. Later, LST decreased in 2016. Lower LST was associated with irrigation farming and orchard, and higher LST was related to sparse oak forest areas. There was also a negative correlation between LST and NDVI. As a result, it was inferred that greenery declined LST. Conversely, a positive correlation was found between LST and NDBI resulting from the built-up areas. Since LST could influence biological, physical, chemical processes, it can therefore be supported as an effective index for environmental sustainability assessment.
Introduction
The global ecosystem has been changed by increasing industrialization and urbanization (Peng et al. Citation2017), leading to land degradation. Facing the effects of land degradation has increased the attention on monitoring, evaluating, and regulating the state and sources of risk and the sustainability degree of ecosystem health (Dernbach and Mintz Citation2011).
Watershed sustainability refers to a condition in which the use of an ecological system in a manner that satisfies current needs without compromising the needs or options of the future generation (Mirchooli et al. Citation2021; Teklitz et al. Citation2021). To achieve the long-term sustainability of human-induced watersheds, interdisciplinary approaches are necessary to assess the long-term social and economic pressure (Fathizad et al. Citation2017). Different watersheds with various spatial scales must have the same particular framework of sustainability, with their own set of indicators. Different methods for assessing ecosystem sustainability, individual indicator or compound indices, product-related assessment, and integrated assessment tools (Ness et al. Citation2007). Among these, sustainability assessment using an indicator and index method is a helpful tool to track sustainability trends. Understanding sustainability trends helps prepare short-term projections for the future (Ness et al. Citation2007). In this regard, the selection of the proper indicator is vital in sustainability assessment. In the same vein, satellite remote sensing has the potential to provide accurate, timely, and lower-cost information about phenomena and natural resources (Zareie et al. Citation2016). Since land-use maps can explain human interferences such as urbanization and industrial development on the environment, the information on spatiotemporal land-use/cover change is therefore essential, which can help policy/decision-makers apprehend the sustainability-related problems (Ibrahim, Samah, and Fauzi Citation2012). August and implement revitalization measures especially in degraded areas (Spalevic et al. Citation2017). Land-use/cover results from climate change and human exploitation (Zhao et al. Citation2020). They are essential driving forces of socio-economic impact on watershed ecosystems (Asadolahi et al. Citation2018) that can affect soil erosion (Spalevic et al. Citation2016), ecosystem services (Peng et al. Citation2020), water quality (Karcher, VanBriesen, and Nietch Citation2013), and even soil quality (Hinge, Surampalli, and Goyal Citation2019). The important biophysical indices such as Normalized Difference Vegetation Index (NDVI), Normalized Difference Built-Up Index (NDBI), and Normalized Difference Water Index (NDWI) are mutated owing to land-use/cover changes (Pal and Ziaul Citation2017), leading to an alteration in sustainability. It also influences the energy swapping between the land surface and atmosphere (Li et al. Citation2017; Wang et al. Citation2016). Accordingly, Land Surface Temperature (LST) is one of the most critical factors excessively controlled land-use/cover change (Wang et al. Citation2016; Fathizad et al. Citation2017).
LST is an important factor that controls and affects biological, physical, and chemical processes on the earth’s surface (Shi and Zhang Citation2017). It affects the biophysical factors such as vegetation, impervious surface, albedo, and, accordingly, the net radiation and water balance (El-Zeiny and Effat Citation2017). It can also provide important information about the physical characteristic of surface and climate change. Therefore, studying the spatiotemporal dynamic of LST can be of great use as an indicator for sustainability evaluation and land-use planning (Rajendran and Mani Citation2015).
Remote Sensing (RS) and Geographic Information System (GIS) are also extensively used in LST analysis and land surface (Bokaie et al. Citation2016). Remote-sensing satellite data, for instance, Landsat (Grover and Singh Citation2016), ASTER (Wang and Liang Citation2009), MODIS (Duan et al. Citation2014), and NOAA AVHRR (Pu et al. Citation2006), have been used to study thermal environment and to derive biophysical parameters such as vegetation and built up indices. Landsat data have the highest resolution among these images and are easy to obtain (Zhang et al. Citation2017).
Various facets of LST have been studied to analyze the relationship between land-use/cover and LST by Grover and Singh (Citation2016) in India, Zhang et al. (Citation2017) in China, Mushore et al. (Citation2017) in Zimbabwe, Jafari and Hasheminasab (Citation2017) in Iran and Gohain, Mohammad, and Goswami (Citation2021). The relation of some particular land-use/cover such as industrial land (Rao et al. Citation2018), urbanized land (Sheng et al. Citation2017), and type of vegetation cover (Adams and Smith Citation2014) with LST was also studied. Some researchers (e.g., Son et al. Citation2012; Sruthi and Aslam Citation2015) used LST to monitor drought in different areas. The relations among LST and some indices were further investigated by AAli, Marsh, and Smith (Citation2017), Roşca et al. (Citation2017), and Fathizad et al. (Citation2017). LST, Urban Heat Island (UHI), and their relations with land-use/cover changes were analyzed in different urban areas (Uysal and Polat Citation2015; Li et al. Citation2016; Zhang et al. Citation2017; Tarawally et al. Citation2018). The impacts of landscape composition and pattern on LST and UHI were examined by Zhou, Huang, and Cadenasso (Citation2011), Song et al. (Citation2014), and Estoque, Murayama, and Myint (Citation2017). These studies help better understand the concepts and foundations of LST and its impact across time and space. However, the relations among different biophysical parameters and LST are known, and the spatiotemporal interaction of LST and biophysical parameters (Tayyebi, Shafizadeh-Moghadam, and Tayyebi Citation2018; Zhang et al. Citation2017) have rarely been addressed. In contrast, it is vital to manage nexus among different segments of the earth system adaptively.
Therefore, the current study has been formulated to investigate the dynamic of spatiotemporal variation of LST variation in connection with land-use change, using NDVI, NDBI, and NDWI over the last 30 years. The results would be helpful for local managers to quantify human-induced effects on the biophysical condition of the study watershed. Besides that, a general and applicable approach is also developed, which can be applied for drawing adaptable strategies for managing watersheds in developing countries.
Materials and methods
Study area
Geographically, the Shazand Watershed, as one of the sub-watershed of the Namak Lake, is located in Markazi Province, Iran, elongated from 33◦ 44’ to 34◦ 12’ N latitude and 49◦ 4’ to 49◦ 52’ E longitude with a total area of 1740 km2. The climate of the watershed is moderate semi-arid to cold semi-arid according to the Embereger classification, with an average annual rainfall of 420 mm and an average temperature of 12 ◦C (Davudirad, Sadeghi, and Sadoddin Citation2016; Sadeghi and Hazbavi Citation2017; Mirchooli et al. Citation2021).
The only forest area of the watershed (ca. 0.01 km2) with a very sparse cover of Quercus persica L., a light and symmetry canopy, is located in the southwest. Moreover, some of the primary vegetation covers of the watershed rangeland include Astragalus verus, Stipa barbata, Acanthophyllum microcephalum, and Artemisia sieberi (Talab et al. Citation2010).
The important industrial areas of Imam Khomeini Refinery, petrochemicals, and thermal power plant and their developmental and supplementary stages were performed in the study watershed over the last 30 years. The study was dissected into four-node years of 1986, 1998, 2008, and 2016 due to data availability and synchronization with industrial development in the area. A general view of the study watershed with supplementary information has been depicted in .
Figure 1. General view, elevation distribution (m), and location of the Shazand Watershed in Markazi Province, Iran.

The rapid urban and industrial expansion has been observed in the Shazand Watershed led to a declining land capability (Davudirad et al. Citation2016; Mirchooli et al. Citation2021). The exerted problems and pressure by urbanization and industrialization motivated researchers to evaluate the land-use change and other related problems in the watershed (Davudirad, Sadeghi, and Sadoddin Citation2016; Hazbavi and Sadeghi Citation2017).
Satellite data processing
In this study, Landsat 5 Thematic Mapper (TM), Landsat 7 Enhanced Thematic Mapper Plus (ETM+), and Landsat 8 Operational Land Imager (OLI) were collected and used for 1986, 1998, 2008, and 2016 to investigate the spatiotemporal variation of LST and to calculate the remote sensing-based indices as shown in . These values have been calculated in ENVI 5.3, ArcGIS 10.3, and Excel 2013.
Table 1. The characteristics of the Landsat image used in the present study
Research method
Land-use classification
The land-use map of the Shazand Watershed for 1986, 1998, and 2008 was obtained from existing records (e.g., Davudirad, Sadeghi, and Sadoddin Citation2016), and the land-use map of 2016 was generated by supervised classification and maximum likelihood algorithm (Davudirad, Sadeghi, and Sadoddin Citation2016). Some eight land-uses/covers viz. bareland, dry farming, forest, irrigation farming, orchard, rangeland, residential, and outcrops were identified. Nearly 1340 samples were collected during the field survey and divided at 30% and 70% for validation and training. Afterward, necessary geo-referenced, radiometric and atmospheric corrections were performed. The error matrix assessed the overall accuracy of land-use, and the producer’s and user’s accuracy were also calculated.
LST retrieval
The TM, ETM+, and OLI images for July (1986, 1998, 2008, and 2016) were used to generate the LST maps using temperature–emissivity separation algorithm through four following main steps (Sahani, Citation2021). Considering the Sahzand watershed located in the center of Iran with an arid and semi-arid climate and the date of image in summer (July), it experiences the lowest value of rainfall and wind and consequent ill-effect on image analysis.
Step 1 – Conversion of digital number into spectral radiance
To estimate the LST in Landsat 5 and 7, digital number (DN) was converted to spectral radiance using EquationEquation (1)(1)
(1) :
where Lλ is spectral at-sensor radiance (Wm−2 sr−1 μm−1), LMin,λ, and LMax,λ are spectral radiance scaled to QCal.Min and QCal, Max, respectively. QCal, Min is the minimum quantized calibrated pixel value (corresponding to LMin), QCal, Max is the maximum quantized calibrated pixel value (corresponding to LMax), and QCal is the quantized calibrated pixel value in digital number (Zareie et al. Citation2016). The “Spectral Subset” in the “Radiometric Correction” tools was used to select the band. All calculations were performed in the ENVI environment.
EquationEquation (2)(2)
(2) was also used for calculation of spectral radiance (Lλ):
where ML represents the band-specific multiplicative rescaling factor, Qcal is the Band 10 image, AL is the band-specific additive rescaling factor, and Oi is the correction value for band 10 (El-Zeiny and Effat Citation2017).
Step 2 – Conversion of spectral radiance to brightness temperature
Spectral radiance was then converted to brightness temperature (TB, in Kelvin) using a thermal constant in metadata with the help of EquationEquation (3)(3)
(3) (Artis and Carnahan Citation1982; Pal and Ziaul Citation2017):
where K1 and K2 are the constant coefficients obtained from metadata (Wm−2 sr−1 μm−1).
Step 3 – Estimation of land surface temperature (LST)
The brightness temperature was ultimately converted to LST using EquationEquation 4(4)
(4) (Artis and Carnahan Citation1982):
where λ is the wavelength of emitted radiance (11.45 μm for Landsat 4, 5 and 7; 10.895 μm for Landsat 8 band 10; 12 μm for Landsat 8 band 11), ɛ is land surface emissivity, ρ = h.c/σ, in which σ is Stefan Boltzmann’s constant (5.67 × 10−8Wm−2K−4), h is Plank’s constant (6.626 × 10−34J S), and c is the light velocity (m s−1) ɛ is land surface emissivity; if NDVI <0.2 then the pixel is considered as bare soil, a mean ɛ is 0.97 if NDVI >0.5; the pixels correspond to dense vegetation areas and ɛ is 0.99 and if 0.2 ≤ NDVI ≤0.5. Each pixel corresponds to the mixing of bare soil and vegetation. ɛ was then computed using EquationEquation (5)(5)
(5) (Sobrino, Raissouni, and Li Citation2001):
which ɛv is the emissivity of vegetation and ɛs is bare soil emissivity. Pv and dɛ were also calculated using EquationEquations (6)(6)
(6) and (Equation7
(7)
(7) ) (Carlson and Ripley Citation1997):
where F is the form factor averaging 0.55 according to the different geometric distribution.
EquationEquation (5)(5)
(5) was eventually briefed to EquationEquation (8)
(8)
(8) (Sobrino, Jiménez-Muñoz, and Paolini Citation2004):
Step 4 – Unit conversion of LST
The unit of calculated LST was converted from Kelvin (◦K) to degree Celsius (◦C) by subtracting a constant value of 273.15.
Validation of LST
To validate estimated LST, the recorded data for two synoptic stations of Arak and Shazand located in and at the vicinity of the study Watershed () were used. Although the Shazand station was established in 2007, the only available and synchronized data of 2016 could be applied for the study. The ground-based data of LST were received from the Markazi Meteorological Bureau.
Development of NDVI, NDBI, and NDWI maps
Landsat 5, 7, and 8 images were employed to calculate NDVI, NDBI, and NDWI. NDVI values vary between −1 and 1, in which negative values were linked to water, low positive values demonstrated bareland and soil, and high positive values pertained to vegetation and greenness (Zhang et al. Citation2017; Lloyd and Dennison Citation2018).
The NDBI was sensitive to built-up (residential) areas. As a result, positive values represented built-up area, and negative values exhibited other land-uses. NDBI was expressed using EquationEquation (9)(9)
(9) (Zhang et al. Citation2017):
where MIR is Band 5 (for Landsat TM), and Band 6 (for Landsat 8), and NIR is Band 4 (for Landsat TM), and Band 5 (for Landsat 8).
The NDWI was also used to distinguish the water content of the vegetation canopy, calculated using EquationEquation (10)(10)
(10) (Liu, Yao, and Wang Citation2016):
where NIR is Band 4 (for Landsat TM), and Band 5 (for Landsat 8), and MIR is Band 5 (for Landsat TM), and Band 6 (for Landsat 8).
To examine the relationship between LST and study indices (i.e., NDVI, NDBI, NDWI), some 10,000 random points were designated across the study area, and appropriate modeling was made using a scatter plot. Furthermore, to better understand land-use influences on biophysical parameters and LST, three transect lines were made across the study area to investigate the fluctuations of NDVI, NDBI, NDWI, and LST in different land-uses/covers. These transects were selected to have the most land-use/cover changes along the lines.
Results and discussion
Land-use/cover maps
In the current study, land-uses were monitored from 1986 to 2016. Eight land-use classes (including bareland, dry farming, forest, irrigation farming, orchard, rangeland, residential, and outcrops) were detected in Landsat images using maximum likelihood classification in 1986, 1998, 2008 (Davudirad, Sadeghi, and Sadoddin Citation2016) and 2016 and mapped in and corresponding data have been summarized in . In this way, the interpolate accuracy for the land-use map in 2016 was 87.58%. The land-use maps indicated that the largest area of the watershed is covered by rangeland in all study periods.
Table 2. Land-use distribution (km2) from 1986 to 2016 for the Shazand Watershed, Iran
The results () showed that settlement and industrial areas increased from 1.40% in 1986 to 5.1% in 2016, verifying human interference in the environment. Regarding the trend of industrial development, there was a conversion and degradation of rangeland in all periods. According to land-use maps, the area of orchards increased simultaneously with industrial development. At the same time, with the increase in the orchard area, bareland was also expanded. Between 1986 and 1998, a remarkable increase was observed in irrigation farming, i.e., some doubles. Furthermore, in 1998–2008, the establishment and related developmental projects of Mohajeran City led to urban extension and encroachment to other land-uses. Overall, the results further showed that the green spaces and orchard areas increased simultaneously as industrial areas were developed.
The spatial pattern of NDVI, NDBI, NDWI, and LST
Given that these indicators are closely related, the spatial value of LST was validated by NDVI and NDBI (Grover and Singh Citation2016). The NDVI maps of 1986, 1998, 2008, and 2016 are shown in . The mean NDVI values for the whole watershed are also illustrated in . The weighted mean NDVI of the Shazand Watershed was about 0.18. By considering that this index proves the vegetation health, stress, and greenness or biomass (Grover and Singh Citation2016) and the values more than 0.3 illustrated healthy vegetation (Sahana, Ahmed, and Sajjad Citation2016), the situation of vegetation cover, especially rangeland (covering 42.32% of the watershed) was not found satisfactory. It indicated a decreasing trend of mean value from 1986 to 2008, corresponding with declining precipitation and drought occurrence. After that, there was a slight increase in 2016, which inferred little improvement in green cover. According to the results, sustainability based on the NDVI indicator had a decreasing trend by 2008. Results also realized that the central and southwest of the watershed had the highest mean value of NDVI in the studied years associated with orchard and irrigation lands. In contrast, outcrops, bareland, and poor rangelands had the lowest mean value of NDVI in different years. The former land-uses with the highest NDVI and the latter with the least NDVI caused the lowest and highest LST, respectively.
Table 3. Mean values of study indices of NI, NDWI, NDBI, and LST in node years in the Shazand Watershed, Iran
shows that the NDBI had positive value in built-up and bareland areas (no vegetation). The mean values of NDBI were −0.005, −0.001, −0.002, and −0.03 in 1986, 1998, 2008, and 2016, respectively. Changes in NDBI will help decision-makers for management and sustainable usage of the lands, as suggested by Sinha, Verma, and Ayele (Citation2016).
The spatial distribution of NDWI was also similar to NDVI in the Shazand Watershed (). NDWI and NDBI took similar bands, but the main difference is what pattern they highlighted (Pal and Ziaul Citation2017), so the distributions of the two indices were similar. The mean values of NDWI are given in .
The LST maps of the study area in 1986, 1998, 2008, and 2016 were ultimately developed, as shown in . The mean LST was 38.82, 40.41, 42.21, and 38.41 ◦C in 1986, 1998, 2008, and 2016. The figures show an increasing trend from 1986 to 2008 due to residential and impervious areas. Furthermore, drought as a natural phenomenon was also a vital driving force that exacerbated the positive trend in LST from 1998 to 2008. However, the effect of other climatic variables such as wind speed on LST has been reported by Tayyebi, Shafizadeh-Moghadam, and Tayyebi (Citation2018) for the metropolitan of Tehran, capital of Iran. The highest value of LST occurred in the western part of the watershed in 1998, and the lowest one occurred in the central part of the watershed in 2008.
To validate the LST retrieval from Landsat images, the ground-based measurements of LST for Arak and Shazand meteorological stations of Markazi Province were collected. The difference between retrieval LST and measured data was 2.81 to 3.84, with the corresponding accuracy beyond 90.98%. The detailed comparisons have been summarized in . The comparison should not be materialized for 1986, 1998, and 2008 in the Shazand station due to the unavailability of measured data.
Table 4. Estimated LST (°C) and corresponding ground-based data for study stations in the Shazand Watershed, Iran
Relation of NDVI, NDBI, NDWI, and LST
These remote sensing-based indices were helpful to interpret land cover more accurately. The variations of NDVI, NDBI, and NDWI were regressed with LST variations whose relationships have been shown in . Relations among remote sensing-based indices and mean LSTs were analyzed using linear regression. The results indicated a negative and significant relationship (R2 = 0.25–0.31, P < 0.01) between LST and NDVI.
Table 5. Relationships between LST and NDVI, NDBI, and NDWI in 1986, 1998, 2008, and 2016 based on 10,000 random points
NDBI was a sensitive index to the built-up area. It was, hence, positively correlated to LST. Though, Bouhennache et al. (Citation2016) reported that other indices such as urban index (UI) could distinguish the urban and soil features better than NDBI. It is also exhibited a negative correlation between LST and NDWI (R2 = 0.25–0.31, P < 0.01) in different years. It was therefore inferred that the lower LSTs, the more water or moisture. These negative and positive relations among indices might be due to the difference in remote sensing-based indices’ capability in recognizing land-uses. For instance, there was only 5% of watershed covered by urban and industrial areas, even in 2016, with the largest comparative area of urbanization. NDBI was defined even for other land-use classes. Hence, the correlation between LST and NDBI was low. This result is similar to that reported by Ali, Marsh, and Smith (Citation2017). Chen and Zhang (Citation2017) also reported the highest correlation coefficient between LST, NDVI, and NDBI. They further indicated that NDBI and NDVI-MNDWI were good indicators for LST estimation. Guha et al. (Citation2018) found a strong relationship between LST and NDVI in Florence and Naples, Italy. Guha and Govil (Citation2021) indicated a strong relationship between NDVI and LST, insignificant for low and high LST zones.
Overall, the results reflected significant consistency with other studies. Roşca et al. (Citation2017) concluded a strong correlation between LST and NDVI in areas with a lack of vegetation cover. These results also agreed with Pal and Ziaul (Citation2017), Zhang et al. (Citation2017), and Madanian et al. (Citation2018). Moreover, the results were consistent with Ali, Marsh, and Smith (Citation2017) except for NDWI, which showed a positive relation with LST and NDWI.
Land-use/cover and LST relationship
Landsat images are helpful tools for investigating the land-use changes and understanding their effect on LST values in different categories. This study concluded that LST in high vegetation-cover areas was lower than the other land-uses. The mean LST was calculated in various land-use classes in studied years (). The LST pattern showed the highest value of the mean LST was mainly distributed in the sparse forest and dry farming. Besides, low values of LST were located mainly in irrigation farming and orchard areas. This was due to the influence of vegetation on LST, which was influenced by evapotranspiration and emissivity. These areas had the highest mean value of NDVI. The sparse residential areas with more vegetation and evaporation cooling effect rather than dense built-up areas (Mushore et al. Citation2017) were found in the study watershed.
Table 6. LSTs (◦C) in different land-uses and study years in the Shazand Watershed, Iran
Overall, the mean LST increased from 1986 to 2016 in most land-use classes. The minimum value of LST occurred in the orchard in 1986, and the maximum value was located in the sparse forest in 2008. The industrial and urban areas had a lower value of LST. Similar results had been reported by Zareie et al. (Citation2016) for Yazd Region, Iran. Furthermore, the forest areas had the highest value of LST in the study period. A sparse oak forest reserve is located on the southern slope of the watershed. The mean value of NDVI for the oak forest was 0.14, which is almost equal to dry farming and rangeland. The other land-uses with high values of LST were rangeland and dry farming. The mean value of LST in 1998 was more than the values in 1986. All land-use classes had the maximum values of LST in 2008 compared to other study years. Most land-use changes occurred in 1998–2008 (Davudirad, Sadeghi, and Sadoddin Citation2016). These results agreed with Jafari and Hasheminasab (Citation2017) that approved the impact role of land-use changes on LST. In addition, Tan et al. (Citation2020) showed that the LST values significantly changed across different land-use types. Mukherjee and Singh (Citation2020) indicated an increase in LST of the study areas, as they experienced an increase in built-up areas. Recently, Mustafa et al. (Citation2021) concluded an increasing trend for LST due to land-use change and population density in Beijing, China.
It should be noted that the areas with no changes in land-uses also had different LSTs verifying other affecting factors. In other words, climate change was effective on LST changes in the region located in the arid and semi-arid belt in the northern hemisphere. This could be attributed to drought, which occurred in 2008, so the annual rainfall was the lowest in studied years, as Zaree et al. (Citation2016) mentioned. Besides, the annual rainfall in 2008 was lower than in other years in most meteorological stations located in the study watershed, as shown in . The same land-use classes had the lowest and the highest values of LST due to the reasons mentioned above in 2016.
As depicted in , three transects were arbitrarily considered across the watershed to study the effects of land-uses on biophysical parameters and LST. To this aim, the profile graph was created using an interpolated line in the ArcGIS environment. In general, it is inferred from these transects that the values of LST have increased as a result of land-use changes and indices in different parts of the profile. The southern profile line (, Bottom-right, CD) crossed rangeland in the center and east, and then dry farming and forest areas in the west. The LST in irrigation farming in the west of the watershed was the lowest value. Transect AB corresponded to the highest NDVI and NDWI. The northern profile line (AB) passed through dry, industrial, irrigation, and dry farming from east to west. The vertical profile line (EF) crossed through rangeland, industrial, dry farming, and rangeland areas from north to south. The detailed results of LST values along the profile lines in 2016 have been shown in .
Figure 8. Variations of LST, NDVI, NDBI, and NDWI along with the transect AB (, bottom-right) for 2016 in the Shazand Watershed, Iran.

Conclusion
The dynamics of LST was examined in the current study in connection with land-use/cover and some biophysical parameters using multi-temporal Landsat images. The results showed a negative correlation between NDVI and LST and a positive correlation between NDBI, NDWI, and LST. Besides, most changes were related to the conversion of rangeland to irrigation farming due to urbanization and industrialization in the study period of some 30 years. The land-use conversions resulted in a progressing mean LST in the studied node years from 1986 to 2016. The spatiotemporal dynamic of LST showed that hotspot areas with the highest LST were associated with sparse oak forest and rangeland, resulting from the effects of land-use change and climate change. It can be inferred from the results of correlations among LST and biophysical parameters that the protection and maintenance of greenery spaces as an essential variable in moderate climate are necessary. So politicians and decision-makers should adopt greenery strategies and protect the oak forest against degradation as a practical approach for reducing hotspot effects. The present study results on land-use changes will provide information about the urbanization development process and help policymakers and regional managers adoptively manage the watershed ecosystem. Moreover, identifying the areas with the highest LST helps decision-makers for proper environmental monitoring and sustainability studies and could lead to management decisions to protect natural resources. Of course, more insight studies with a more extended period and high-resolution information and data are needed to draw an integrated conclusion.
Data availability
Some or all data, models, or code generated or used during the study are available in a repository or online in accordance with funder data retention policies. (https://earthexplorer.usgs.gov/)
Supplemental Material
Download MS Word (12.9 KB)Supplemental Material
Download MS Word (2 MB)Disclosure statement
No potential conflict of interest was reported by the authors.
Supplementary material
Supplemental data for this article can be accessed here.
Additional information
Funding
References
- Adams, M. P., and P. L. Smith. 2014. “A Systematic Approach to Model the Influence of the Type and Density of Vegetation Cover on Urban Heat Using Remote Sensing.” Landscape Urban Plan 132: 47–15. doi:10.1016/j.landurbplan.2014.08.008.
- Ali, J. M., S. H. Marsh, and M. J. Smith. 2017. “A Comparison between London and Baghdad Surface Urban Heat Islands and Possible Engineering Mitigation Solutions.” Sustainable Cities and Society 29: 159–168. doi:10.1016/j.scs.2016.12.010.
- Artis, D. A., and W. H. Carnahan. 1982. “Survey of Emissivity Variability in Thermography of Urban Areas.” Remote Sensing of Environment 12: 313–329. doi:10.1016/0034-4257(82)90043-8.
- Asadolahi, Z., A. Salmanmahiny, Y. Sakieh, S. H. Mirkarimi, H. Baral, and M. Azimi. 2018. “Dynamic Trade-off Analysis of Multiple Ecosystem Services under Land Use Change Scenarios: Towards Putting Ecosystem Services into Planning in Iran.” Ecological Complexity 36: 250–260. doi:10.1016/j.ecocom.2018.09.003.
- Bokaie, M., M. K. Zarkesh, P. D. Arasteh, and A. Hosseini. 2016. “Assessment of Urban Heat Island Based on the Relationship between Land Surface Temperature and Land Use/Land Cover in Tehran.” Sustainable Cities and Society 23: 94–104. doi:10.1016/j.scs.2016.03.009.
- Bouhennache, R., T. Bouden, A. Taleb, and A. Chaddad. 2016. “Extraction of Urban Land Features from TM Landsat Image Using the Land Features to Index and Tasseled Cap Transformation.” Extraction 1: 33037. doi:10.1999/1307-6892/33037.
- Carlson, T. N., and D. A. Ripley. 1997. “On the Relation between NDVI, Fractional Vegetation Cover, and Leaf Area Index.” Remote Sensing of Environment 62: 241–252. doi:10.1016/S0034-4257(97)00104-1.
- Chen, X., and Y. Zhang. 2017. “Impacts of Urban Surface Characteristics on Spatiotemporal Pattern of Land Surface Temperature in Kunming of China.” Sustainable Cities and Society 32: 87–99. doi:10.1016/j.scs.2017.03.013.
- Davudirad, A. A., S. H. Sadeghi, and A. Sadoddin. 2016. “The Impact of Development Plans on Hydrological Changes in the Shazand Watershed, Iran.” Land Degradation and Development 27: 1236–1244. doi:10.1002/ldr.2523.
- Dernbach, J. C., and J. A. Mintz. 2011. “Environmental Laws and Sustainability: An Introduction.” Sustainability 3: 531–540. doi:10.3390/su3030531.
- Duan, S. B., Z. L. Li, B. H. Tang, H. Wu, and R. Tang. 2014. “Generation of a Time-consistent Land Surface Temperature Product from MODIS Data.” Remote Sensing of Environment 140: 339–349. doi:10.1016/j.rse.2013.09.003.
- El-Zeiny, A. M., and H. A. Effat. 2017. “Environmental Monitoring of Spatiotemporal Change in Land Use/land Cover and Its Impact on Land Surface Temperature in El-Fayoum Governorate, Egypt.” Remote Sensing Applications: Society and Environment 8: 266–277. doi:10.1016/j.rsase.2017.10.003.
- Estoque, R. C., Y. Murayama, and S. W. Myint. 2017. “Effects of Landscape Composition and Pattern on Land Surface Temperature: An Urban Heat Island Study in the Megacities of Southeast Asia.” Science of the Total Environment 577: 349–359. doi:10.1016/j.scitotenv.2016.10.195.
- Fathizad, H., M. Tazeh, S. Kalantari, and S. Shojaei. 2017. “The Investigation of Spatiotemporal Variations of Land Surface Temperature Based on Land Use Changes Using NDVI in Southwest of Iran.” Journal of African Earth Sciences 134: 249–256. doi:10.1016/j.jafrearsci.2017.06.007.
- Gohain, K. J., P. Mohammad, and A. Goswami. 2021. “Assessing the Impact of Land Use Land Cover Changes on Land Surface Temperature over Pune City, India.” Quaternary International 575: 259–269. doi:10.1016/j.quaint.2020.04.052.
- Grover, A., and R. B. Singh. 2016. “Monitoring Spatial Patterns of Land Surface Temperature and Urban Heat Island for Sustainable Megacity: A Case Study of Mumbai, India, Using Landsat TM Data.” Environment and Urbanization ASIA 7: 38–54. doi:10.1177/0975425315619722.
- Guha, S., and H. Govil. 2021. “An Assessment on the Relationship between Land Surface Temperature and Normalized Difference Vegetation Index.” Environment, Development and Sustainability 23 (2): 1944–1963. doi:10.1007/s10668-020-00657-6.
- Guha, S., H. Govil, A. Dey, and N. Gill. 2018. “Analytical Study of Land Surface Temperature with NDVI and NDBI Using Landsat 8 OLI and TIRS Data in Florence and Naples City, Italy.” European Journal of Remote Sensing 51 (1): 667–678. doi:10.1080/22797254.2018.1474494.
- Hazbavi, Z., and S. H. R. Sadeghi. 2017. “Watershed Health Characterization Using Reliability–Resilience–Vulnerability Conceptual Framework Based on Hydrological Responses.” Land Degradation and Development 28: 1528–1537. doi:10.1002/ldr.2680.
- Hinge, G., R. Y. Surampalli, and M. K. Goyal. 2019. “Effects of Land Use and Soil Management on Soil Quality in India’s Northeastern Himalayas.” Journal of Environmental Engineering 145 (4): 04019007. doi:10.1061/(ASCE)EE.1943-7870.0001507.
- Ibrahim, I., A. A. Samah, and R. Fauzi. 2012 August. “Land Surface Temperature and Biophysical Factors in Urban Planning.” International Conference on Ecosystem, Environment, and Sustainable Development. Kuala Lumpur, Malaysia, 1792–1797.
- Jafari, R., and S. Hasheminasab. 2017. “Assessing the Effects of Dam Building on Land Degradation in Central Iran with Landsat LST and LULC Time Series.” Environmental Monitoring and Assessment 189: 74. doi:10.1007/s10661-017-5792-y.
- Karcher, S. C., J. M. VanBriesen, and C. T. Nietch. 2013. “Alternative Land-use Method for Spatially Informed Watershed Management Decision Making Using SWAT.” Journal of Environmental Engineering 139 (12): 1413–1423. doi:10.1061/(ASCE)EE.1943-7870.0000770.
- Li, X., W. Li, A. Middel, S. L. Harlan, A. J. Brazel, and B. L. Turner. 2016. “Remote Sensing of the Surface Urban Heat Island and Land Architecture in Phoenix, Arizona: Combined Effects of Land Composition and Configuration and Cadastral–demographic-economic Factors.” Remote Sensing of Environment 174: 233–243. doi:10.1016/j.rse.2015.12.022.
- Li, Z., W. Wu, X. Liu, B. D. Fath, H. Sun, X. Liu, X. Xia, and J. Cao. 2017. “Land Use/cover Change and Regional Climate Change in an Arid Grassland Ecosystem of Inner Mongolia, China.” Ecological Modelling 353: 86–94. doi:10.1016/j.ecolmodel.2016.07.019.
- Liu, Z., Z. Yao, and R. Wang. 2016. “Assessing Methods of Identifying Open Water Bodies Using Landsat 8 OLI Imagery.” Environmental Earth Sciences 75: 1–13. doi:10.1007/s12665-016-5686-2.
- Lloyd, B. J., and P. E. Dennison. 2018. “Evaluating the Response of Conventional and Water Harvesting Farms to Environmental Variables Using Remote Sensing.” Agriculture, Ecosystems & Environment 262: 11–17. doi:10.1016/j.agee.2018.04.009.
- Madanian, M., A. R. Soffianian, S. S. Koupai, S. Pourmanafi, and M. Momeni. 2018. “The Study of Thermal Pattern Changes Using Landsat-derived Land Surface Temperature in the Central Part of Isfahan Province.” Sustainable Cities and Society 39: 650–661. doi:10.1016/j.scs.2018.03.018.
- Mirchooli, F., S. H. R. Sadeghi, A. Khaledi Darvishan, and J. Strobl. 2021. “Multi-dimensional Assessment of Watershed Condition Using a Newly Developed Barometer of Sustainability.” Science of the Total Environment 791: 148389. doi:10.1016/j.scitotenv.2021.148389.
- Mukherjee, F., and D. Singh. 2020. “Assessing Land Use–land Cover Change and Its Impact on Land Surface Temperature Using LANDSAT Data: A Comparison of Two Urban Areas in India.” Earth System Environment 4 (2): 385–407. doi:10.1007/s41748-020-00155-9.
- Mushore, T. D., O. Mutanga, J. Odindi, and T. Dube. 2017. “Linking Major Shifts in Land Surface Temperatures to Long Term Land Use and Land Cover Changes: A Case of Harare, Zimbabwe.” Urban Climate 20: 120–134. doi:10.1016/j.uclim.2017.04.005.
- Mustafa, E. K., G. Liu, A. El-Hamid, T. Hazem, and M. R. Kaloop. 2021. “Simulation of Land Use Dynamics and Impact on Land Surface Temperature Using Satellite Data.” GeoJournal 86 (3): 1089–1107. doi:10.1007/s10708-019-10115-0.
- Ness, B., E. Urbel-Piirsalu, S. Anderberg, and L. Olsson. 2007. “Categorising Tools for Sustainability Assessment.” Ecological Economics 60: 498–508. doi:10.1016/j.ecolecon.2006.07.023.
- Pal, S., and S. Ziaul. 2017. “Detection of Land Use and Land Cover Change and Land Surface Temperature in English Bazar Urban Centre.” The Egyptian Journal of Remote Sensing and Space Sciences 20: 125–145. doi:10.1016/j.ejrs.2016.11.003.
- Peng, J., L. Tian, Z. Zhang, Y. Zhao, S. M. Green, T. A. Quine, and J. Meersmans. 2020. “Distinguishing the Impacts of Land Use and Climate Change on Ecosystem Services in a Karst Landscape in China.” Environmental Earth Sciences 46: 101199. doi:10.1016/j.ecoser.2020.101199.
- Peng, J., Y. Liu, T. Li, and J. Wu. 2017. “Regional Ecosystem Health Response to Rural Land Use Change: A Case Study in Lijiang City, China.” Ecological Indicators 72: 399–410. doi:10.1016/j.ecolind.2016.08.024.
- Pu, R. M., P. Gong, R. Michishita, and T. Sasagawa. 2006. “Assessment of Multi-resolution and Multi-sensor Data for Urban Surface Temperature Retrieval.” Remote Sensing of Environment 104: 211–225. doi:10.1016/j.rse.2005.09.022.
- Rajendran, P., and K. Mani. 2015. “Estimation of Spatial Variability of Land Surface Temperature Using Landsat 8 Imagery.” International Journal of Engineering Science Technologies 11: 19–23.
- Rao, Y., Y. Xu, J. Zhang, Y. Guo, and M. Fu. 2018. “Does Subclassified Industrial Land Have a Characteristic Impact on Land Surface Temperatures? Evidence for and Implications of Coal and Steel Processing Industries in a Chinese Mining City.” Ecological Indicators 89: 22–34. doi:10.1016/j.ecolind.2018.01.058.
- Roşca, C. F., G. V. Harpa, A. E. Croitoru, I. Herbel, A. M. Imbroane, and D. C. Burada. 2017. “The Impact of Climatic and Non-climatic Factors on Land Surface Temperature in Southwestern Romania.” Theoretical and Applied Climatology 130: 775–790. doi:10.1007/s00704-016-1923-6.
- Sadeghi, S. H., and Z. Hazbavi. 2017. “Spatiotemporal Variation of Watershed Health Propensity through Reliability-resilience-vulnerability Based Drought Index (Case Study: Shazand Watershed in Iran).” Science of the Total Environment 587: 168–176. doi:10.1016/j.scitotenv.2017.02.098.
- Sahana, M., R. Ahmed, and H. Sajjad. 2016. “Analyzing Land Surface Temperature Distribution in Response to Land Use/land Cover Change Using Split Window Algorithm and Spectral Radiance Model in Sundarban Biosphere Reserve, India.” Modeling Earth Systems and Environment 2: 81. doi:10.1007/s40808-016-0135-5.
- Sahani, N., 2021. “Assessment of spatio-temporal changes of land surface temperature (LST) in Kanchenjunga Biosphere Reserve (KBR), India using Landsat satellite image and single channel algorithm”. Remote Sens. Appl.: Soci. Environ., 24, 100659. DOI: 10.1016/j.rsase.2021.100659
- Sheng, L., X. Tang, H. You, Q. Gu, and H. Hu. 2017. “Comparison of the Urban Heat Island Intensity Quantified by Using Air Temperature and Landsat Land Surface Temperature in Hangzhou, China.” Ecological Indicators 72: 738–746. doi:10.1016/j.ecolind.2016.09.009.
- Shi, Y., and Y. Zhang. 2017. “Remote Sensing Retrieval of Urban Land Surface Temperature in Hot-humid Region.” Urban Climate 1–12. doi:10.1016/J.Uclim.2017.01.001.
- Sinha, P., N. K. Verma, and E. Ayele. 2016. “Urban Built-up Area Extraction and Change Detection of Adama Municipal Area Using Time-series Landsat Images.” International Journal Of Advancement In Remote Sensing, Gis And Geography 5: 1886–1895. doi:10.23953/cloud.ijarsg.67.
- Sobrino, J. A., J. C. Jiménez-Muñoz, and L. Paolini. 2004. “Land Surface Temperature Retrieval from Landsat TM 5.” Remote Sensing of Environment 90: 434–440. doi:10.1016/j.rse.2004.02.003.
- Sobrino, J. A., N. Raissouni, and Z.-L. Li. 2001. “A Comparative Study of Land Surface Emissivity Retrieval from NOAA Data.” Remote Sensing of Environment 75: 256–266. doi:10.1016/S0034-4257(00)00171-1.
- Son, N. T., C. F. Chen, C. R. Chen, L. Y. Chang, and V. Q. Minh. 2012. “Monitoring Agricultural Drought in the Lower Mekong Basin Using MODIS NDVI and Land Surface Temperature Data.” International Journal of Applied Earth Observation and Geoinformation 18: 417–427. doi:10.1016/j.jag.2012.03.014.
- Song, J., S. Du, X. Feng, and L. Guo. 2014. “The Relationships between Landscape Compositions and Land Surface Temperature: Quantifying Their Resolution Sensitivity with Spatial Regression Models.” Landscape and Urban Planning 123: 145–157. doi:10.1016/j.landurbplan.2013.11.014.
- Spalevic, V., G. Barovic, A. Fikfak, S. Kosanovic, M. Djurovic, and S. Popovic. 2016. “Sediment Yield and Land Use Changes in the Northern Montenegrin Watersheds: Case Study of Seocki Potok of the Polimlje Region.” Journal of Environmental Protection and Ecology 17: 990–1002.
- Spalevic, V., M. Lakicevic, D. Radanovic, P. Billi, G. Barovic, D. Vujacic, and A. K. Khaledi Darvishan. 2017. “Ecological-Economic (Eco-eco) Modelling in the River Basins of Mountainous Regions: Impact of Land Cover Changes on Sediment Yield in the Velicka Rijeka, Montenegro.” Notulae Botanicae Horti Agrobotanici Cluj-Napoca 45: 602–610. doi:10.15835/nbha45210695.
- Sruthi, S., and M. M. Aslam. 2015. “Agricultural Drought Analysis Using the NDVI and Land Surface Temperature Data; a Case Study of Raichur District.” Aquatic Procedia 4: 1258–1264. doi:10.1016/j.aqpro.2015.02.164.
- Talab, S. R. F., A. Siavash, M. Shaban, and E. Panahpour. 2010. “The Study of Ecological Changes and Seriate Structure of Markazi Province Forest (Case Study: Shazand Province, Oak Jungles).” International Journal of Agronomy Plant Product 3: 87–91.
- Tan, J., D. Yu, Q. Li, X. Tan, and W. Zhou. 2020. “Spatial Relationship between Land-use/land-cover Change and Land Surface Temperature in the Dongting Lake Area, China.” Scientific Reports 10 (1): 1–9. doi:10.1038/s41598-019-56847-4.
- Tarawally, M., W. Xu, W. Hou, and T. D. Mushore. 2018. “Comparative Analysis of Responses of Land Surface Temperature to Long-term Land Use/cover Changes between a Coastal and Inland City: A Case of Freetown and Bo Town in Sierra Leone.” International Journal of Remote Sensing 10: 112. doi:10.3390/rs10010112.
- Tayyebi, A., H. Shafizadeh-Moghadam, and A. H. Tayyebi. 2018. “Analyzing Long-term Spatio-temporal Patterns of Land Surface Temperature in Response to Rapid Urbanization in the Mega-city of Tehran.” Land Use Policy 71: 459–469. doi:10.1016/j.landusepol.2017.11.023.
- Teklitz, A., C. Nietch, T. Whiteaker, M. S. Riasi, D. Maidment, and L. Yeghiazarian. 2021. “Stochastic Reliability-based Risk Evaluation and Mapping for Watershed Systems and Sustainability (STREAMS).” Journal of Hydrology 596: 126030. doi:10.1016/j.jhydrol.2021.126030.
- Uysal, M., and N. Polat. 2015. “An Investigation of the Relationship between Land Surface Temperatures and Biophysical Indices Retrieved from Landsat TM in Afyonkarahisar (Turkey).” Teh. Vjesn. - Tech. Gaz 22: 177–181. doi:10.17559/TV-20140514212110.
- Wang, K., and S. Liang. 2009. “Evaluation of ASTER and MODIS Land Surface Temperature and Emissivity Products Using Long-term Longwave Radiation Observations at SURFRAD Sites.” Remote Sensing of Environment 113: 1556–1565. doi:10.1016/j.rse.2009.03.009.
- Wang, S., Q. Ma, H. Ding, and H. Liang. 2016. “Detection of Urban Expansion and Land Surface Temperature Change Using Multi-temporal Landsat Images.” Resources, Conservation and Recycling 128: 526–534. doi:10.1016/j.resconrec.2016.05.011.
- Zareie, S., H. Khosravi, A. Nasiri, and M. Dastorani. 2016. “Using Landsat Thematic Mapper (TM) Sensor to Detect Change in Land Surface Temperature in Relation to Land Use Change in Yazd, Iran.” Solid Earth 7: 1551. doi:10.5194/se-7-1551-2016.
- Zhang, F., H. Kung, V. C. Johnson, B. I. LaGrone, and J. Wang. 2017. “Change Detection of Land Surface Temperature (Lst) and Some Related Parameters Using Landsat Image: A Case Study of the Ebinur Lake Watershed, Xinjiang, China.” Wetlands 1–16. doi:10.1007/s13157-017-0957-6.
- Zhang, X., R. C. Estoque, and Y. Murayama. 2017. “An Urban Heat Island Study in Nanchang City, China Based on Land Surface Temperature and Social-ecological Variables.” Sustainable Cities and Society 32: 557–568. doi:10.1016/j.scs.2017.05.005.
- Zhao, L., W. Huang, J. Chen, Y. Dong, B. Ren, and Y. Geng. 2020. “Land Use/cover Changes in the Oriental Migratory Locust Area of China: Implications for Ecological Control and Monitoring of Locust Area.” Agriculture, Ecosystems & Environment 303: 107110. doi:10.1016/j.agee.2020.107110.
- Zhou, W., G. Huang, and M. L. Cadenasso. 2011. “Does Spatial Configuration Matter? Understanding the Effects of Land Cover Pattern on Land Surface Temperature in Urban Landscapes.” Landscape and Urban Planning 102: 54–63. doi:10.1016/j.landurbplan.2011.03.009.