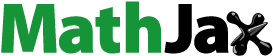
ABSTRACT
Wetlands account for up to 70% of the natural source of methane (CH4) in terrestrial ecosystems on a global scale. Soil microbes are the ultimate producers and biological consumers of CH4 in wetlands. Therefore, simulating microbial mechanisms of CH4 production and consumptionwould improve the predictability of CH4 flux in wetland ecosystems. In this study, we applied a microbial-explicit model, the CLM-Microbe, to simulate CH4 flux in three major natural wetlands in northeastern China. The CLM-Microbe model was able to capture the seasonal variation of gross primary productivity (GPP), dissolved organic carbon (DOC), and CH4 flux. The CLM-Microbe model explained more than 40% of the variation in GPP and CH4 flux across sites. Marsh wetlands had higher CH4 flux than mountain peatlands. Ebullition dominated the CH4 transport pathway in all three wetlands. The methanogenesis dominates while methanotroph makes a minor contribution to the CH4 flux, making all wetlands a CH4 source. Sensitivity analysis indicated that microbial growth and death rates are the key factors governing CH4 emission and vegetation physiological properties (flnr) and maintenance respiration predominate GPP variation. Explicitly simulating microbial processes allows genomic information to be incorporated, laying a foundation for better predicting CH4 dynamics under the changing environment.
KEYWORDS:
Introduction
Natural wetlands store approximately 30% of soil carbon (C) on the land (Melton et al. Citation2013); those C will be released into the atmosphere either as methane (CH4) or CO2, depending on the soil redox potential (Le and Jean Citation2001). Wetlands roughly account for one-third of the increasing atmospheric CH4 concentration (Bhullar et al. Citation2014; Bridgham et al. Citation2013; Saunois et al. Citation2020). CH4, a potent greenhouse gas, has a global warming potential 28 times larger than carbon dioxide (CO2) (Bridgham et al. Citation2013; Harmsen et al. Citation2020; Schaefer Citation2019). The rising CH4 concentration contributed to 22% of climate warming caused by anthropogenic activities since the Industrial Revolution (IPCC Citation2017). However, the estimates of CH4 emissions remain highly uncertain (Saunois et al., Citation2020), particularly in natural wetlands (Jackson et al., Citation2020). From a global perspective, natural wetlands are the largest and most uncertain source of atmospheric CH4 (Bousquet et al. Citation2006a; Kirschke et al. Citation2013a; Zhang et al. Citation2017a).
The land surface CH4 flux depends on the balance of microbial methanogenesis and methanotrophy (Fazli, Man, and Shah Citation2013). When there is a lack of electron acceptors (oxygen, nitrate, sulfate, iron, and manganese), anaerobic fermentation will occur, and methanogens will use fermentation products (hydrogen and CO2, acetic acid, and methyl compounds) to produce CH4 (Cordruwisch, Seitz, and Conrad Citation1988; McGlynn Citation2017; Sieber, McInerney, and Gunsalus Citation2012; Thauer et al. Citation2008; Timmers et al. Citation2017). Under aerobic conditions, CH4 is oxidized to CO2 by methanotrophs. Both processes occur and determine the direction of CH4 flux. Although these processes have been well-understood, microbial models with explicit representation of methanogenesis and methanotrophy are still in their infancy (Xiaofeng et al. Citation2015; Xu et al., Citation2016). In addition, the ecosystem research on modeling wetland CH4 processes in China is rare (Sun et al. Citation2018; Wang et al., Citation2019; Xiaofeng and Tian Citation2012).
Wetland ecosystem models can be categorized as empirical models and process-based models. The empirical models are primarily based on empirical equations obtained from a large amount of data to make predictions. The observed CO2 and CH4 fluxes are directly related to environmental factors such as groundwater level, soil temperature, and net primary productivity (NPP) (Zhang et al. Citation2017a). However, due to the lack of mechanistic representation of the CH4 cycle in these empirical models, there are usually large discrepancies between the measured and simulated flux when the models are used in different times and regions (Huang, Sass, and Fisher Citation1998; Meng et al. Citation2012; Morin et al. Citation2014; Wania et al. Citation2013), which has restricted the popularization and application of these models (Xu et al., 2016). The process-based models consider the major biogeochemical processes of CH4 cycling to achieve accurate predictability of CH4 cycling within a specific ecosystem (Kirschke et al. Citation2013; Hanqin et al. Citation2016). In recent years, some CH4 models have been developed and applied to simulate CH4 cycling in natural wetlands. For example, Lipson et al. (Citation2012) used the DLEM model to explore the CH4 exchange between the atmosphere and marshland over China. Tingting et al. (Citation2020) verified the application of the CH4MOD model in the natural wetlands of the Sanjiang Plain. Zhang et al. (Citation2020a) used the TRIPLEX-GHG model to simulate the spatial pattern of CH4 emissions in the Qinghai-Tibet Plateau. Additionally, Lund-Potsdam-Jena Wetland Hydrology and Methane (LPJ-WHyMe), Terrestrial Ecosystem Model (TEM), Denitrification-Decomposition model (DNDC), and other models have been applied to simulate wetland CH4 fluxes in the Arctic, Europe, and the world (Chadburn et al. Citation2020; Tingting et al. Citation2020; Melton et al. Citation2013; Nzotungicimpaye et al. Citation2020; Youmi et al. Citation2020; Wania, Ross, and Prentice Citation2010).
The CLM-Microbe is a microbial-explicit model that simulates substrates, production, oxidation, transport of CH4 and their environmental controls, along with microbial mechanisms for soil C mineralization (Xiaofeng et al. Citation2014, Citation2015; Yihui et al. Citation2019). A key advantage is that it represents multiple microbial functional groups. In this study, we used the CLM-Microbe model to simulate GPP and CH4 flux in three wetlands. There are three objectives of this study: (1) to evaluate the performance of the CLM-Microbe model in simulating CH4 flux in wetlands of northeastern China; (2) to investigate the differences in CH4 cycling among three major wetlands; (3) to identify the key factors controlling on GPP and CH4 flux among the wetland types.
Methodology
Site description
This study applied the model to three wetland ecosystems in northeast China, including marshland in the Sanjiang Plain (47.58°N, 133.52°E), peatland in the Changbai Mountain (42.35°N, 126.38°E), and the swamp in the Lesser Khingan Mountain (48.16°N, 128.5°E). These three sites represent two major wetland types in northeastern China, freshwater marshes on the lowland<apos;>s plains (Sanjiang Plain) and mountain peatlands (Changbai Mountain and Lesser Khingan Mountain).
The Sanjiang Plain features the largest freshwater wetland in China. It has a continental monsoon climate, with a mean annual temperature of 2.52°C and a mean annual precipitation of 558 mm. The wetland in the Changbai Mountain has a continental monsoon climate, with a mean annual temperature of 3.3°C and mean annual precipitation of 1054 mm. The precipitation is primarily occurring during July-August. The early spring snowfall and precipitation lead to a water table of 10 cm. The dominant plant community in the wetland is Carex sphagnum. There is huge Holocene peat stored in the wetland, with a thickness of 4–5 m. The upper part is mainly moss peat, and a frozen layer is formed in winter. The Lesser Khingan Mountain is located between Greater Khingan Mountain and Changbai Mountain and is one of the major areas with swamps in China. This site has a continental humid monsoon climate, with a mean annual temperature of 0.4°C and mean annual precipitation of 630 mm (primarily occurring during July-August). It primarily consists of forest swamps, shrub swamps, grass swamps, and moss swamps.
Data sources
The CH4 flux was monitored using the close-path eddy covariance technique and static chamber approach. Flux measurement periods were from May to October in 2012 and 2013 at the Sanjiang Plain () (Sun et al. Citation2018). The CH4 flux for the Changbai Mountain and Lesser Khingan Mountain was obtained through periodic sampling by a static chamber approach (Huang Citation2016; Shi Citation2019). We extracted CH4 flux data for these two sites from the published articles by software GetData version 2.26 (http://getdata-graph-digitizer.com/). The observational GPP data of Sanjiang Plain and Changbai Mountain are calculated with net ecosystem C exchange (NEE) and ecosystem respiration (ER) (Cao Citation2015.). The GPP data of Lesser Khingan Mountain is obtained by extracting the GPP data of MODIS (MOD17A) products (Yuan et al. Citation2020; Maosheng et al. Citation2005).
Table 1. Atmospheric forcing data and flux data source
Modeling experiment
Model description and driving forces
The CLM‐Microbe model branches from the framework of default CLM4.5 by developing a new microbial functional group-based module for CH4 production and consumption (Xiaofeng et al. Citation2015), in association with the decomposition subroutines in CLM4.5 (Koven et al. Citation2013; Thornton et al. Citation2007). It incorporates new mechanisms of DOC fermentation, hydrogenotrophic methanogenesis, acetoclastic methanogenesis, aerobic methanotrophy, anaerobic methanotrophs, and H2 production (Xiaofeng et al. Citation2015; Yihui et al. Citation2019). Detailed mathematical expressions for CH4 production and consumption processes were organized in Xiaofeng et al. (Citation2015) and Yihui et al. (Citation2019), Wang et al. (Citation2022)). The processes of microbial assimilation of C can be referred to in Xiaofeng et al. (Citation2014) and Liyuan et al. (Citation2021a), Liyuan et al. (Citation2021b)). The code for the CLM‐Microbe model is archived at Github (https:// github.com/email‐clm/clm‐microbe). The model version used in this study was checked out from GitHub on 18 June 2018.
In our previous study, the CLM‐Microbe model was validated for simulating the dynamics of CO2 and CH4 emissions from incubation experiments on Arctic soils with invariant soil temperature and soil water content (Xiaofeng et al. Citation2015). In addition, the CLM-Microbe model was applied to examine the microtopographic impacts on CO2 and CH4 flux in the Arctic tundra ecosystem (Yihui et al. Citation2019) and microbial seasonality on soil C cycling in terrestrial ecosystems (He et al., Citation2021b). In this study, we focused on the fully incorporated CLM-Microbe model, with model simulations for each wetland type. Eighteen key parameters were chosen for model parameterization, which represents the decomposition of organic C, methanogenesis, microbial growth, and plant photosynthesis and respiration and therefore controls the GPP and CH4 flux ().
Table 2. Key parameters for sensitivity analysis (see Xiaofeng et al. Citation2015)
The model driving forces include meteorological, edaphic, and vegetation datasets. The meteorological data include air temperature, relative humidity, incoming solar radiation, longwave radiation, precipitation rate, surface pressure, and surface winds. Meteorological variables of Changbai Mountain (2003–2018) and Lesser Khingan Mountain (2010–2018) were extracted from China<apos;>s Meteorological Forcing Dataset (https://data.tpdc.ac.cn/en/data/8028b944-daaa-4511-8769-965612652c49/) by the longitude and latitude information. Since the standardized forcing data are in half-hourly time steps, the extracted 3-hourly data for each study site was interpolated to half-hourly step by using linear interpolation in R programming (R for Window version 4.0.2). The forcing data of Sanjiang Plain marsh wetland (2005–2018) was from the Sanjiang Plain Experimental station (). The observed soil and vegetation variables, such as plant functional type (PFT), were used for model parameterization and validation.
Model implementation
In this study, we set up model simulations with the CLM-Microbe model separately for each site. The model implementation was carried out in three stages. First, the accelerated model spin‐up was set up for 2,000 years to allow the system to accumulate C. Then, a final spin‐up was set up for 50 years to allow the ecosystem to transient to a state with the realistic decomposition rates before the transient simulations that cover the period of 1850–2018 (Koven et al. Citation2013; Thornton et al., Citation2005).
The model parameterization was initialized with the default parameters in Xiaofeng et al. (Citation2015) and Yihui et al. (Citation2019); it was performed within their ranges to determine the optimal values of parameters in the microbial module for simulating the observational GPP and CH4 flux for each site. For the marshland in the Sanjiang Plain, the observed data of GPP and CH4 flux during 2012–2013 were used for model validation. For the peatland at the Changbai Mountain, we extracted the GPP data during 2011 (Cao Citation2015.) and CH4 flux data during 2016 (Shi Citation2019) from the publications. For Lesser Khingan Mountain, we extracted the GPP data during 2010–2011 from MODIS and CH4 flux data during 2013–2014 (Huang Citation2016).
Model evaluation
Simple linear regression was conducted to evaluate the model performance in terms of GPP and CH4 flux. The error statistics were used to quantify the difference between the modeled results and observational data. In order to verify the accuracy of the model, we used three accuracy evaluation indicators, coefficient of determination (R2), root mean square error (RMSE), and mean absolute error (MAE), to evaluate model efficacy.
Where is the observed value;
means the simulated value; n is the number of data points. The MAE indicates the mean error of the model simulation, and thus lower MAE values suggest better model performance. The RMSE quantifies the mean error of model simulation with low values indicating high model accuracy. Higher R2 values indicate better performance of the model, while lower R2 values mean worse model performance and a smaller proportion of variation is explained by the model. It is noteworthy that R2 is not suitable for assessing the goodness-of-fit for the dataset with small sample size.
Sensitivity analysis
To identify the most important process and the most sensitive parameters for CH4 and GPP dynamics, a global sensitivity analysis was conducted for each wetland type. It focused on the 18 parameters related to plant and microbial processes that are critical for microbial biogeochemistry (). For each parameter, we set up model simulations with +20% and −20% changes and investigated the responses of the modeled GPP and CH4 flux. The index S, comparing the change in the model output relative to the model response for a nominal set of parameters, was calculated based on the flowing equation (Xiaofeng et al. Citation2015):
where S is the ratio of the standardized change in model response to the standardized change in parameter values. Ra and Rn are model responses for altered and nominal parameters, respectively, and Pa and Pn are the altered and nominal parameters, respectively. S is negative if the direction of model response opposes the direction of parameter change (Xiaofeng et al. Citation2015; Yihui et al. Citation2019; Yuan et al., Citation2021a Citation2021; Yuan et al., Citation2021b).
Results
Comparison of simulated GPP and CH4 flux against observational data
The CLM-Microbe model was able to reconstruct the GPP and CH4 flux during the study period for all three sites (; ). For example, the simulated temporal variations in GPP and CH4 flux were consistent with observational data (R2 ≥ 0.41 for all sites). There were slight differences in simulated and observed variables among sites; specifically, modeled GPP was more consistent with the observed data for the Lesser Khingan Mountain (R2 = 0.93, P < 0.001; ) than for the Sanjiang Plain (R2 = 0.49, P < 0.001; ) and Changbai Mountain (R2 = 0.45, P < 0.001; ). For the dynamics of CH4 flux, modeled CH4 flux was more consistent with the observational fluxes for the Changbai Mountain (R2 = 0.91, P = 0.012; ) than for the Sanjiang Plain (R2 = 0.55, P < 0.001; ) and Lesser Khingan Mountain (R2 = 0.41, P = 0.01; ). Furthermore, the molded DOC, the immediate substrate of CH4 production, was consistent with the observational data ().
Figure 1. Site location map of the study area; purples diamonds are the sites for model simulations with the background as wetland distribution in northeastern China.

Table 3. Site level evaluation of the goodness-of-fit criteria computed for the simulated GPP (gC/m2/d), CH4 flux (gC/m2/d) and DOC
The MAE and RMSE values suggested large variations in model performance when simulating GPP and CH4 among sites. The MAE and RMSE varied within a range of 28- and 27- fold, respectively, among sites. Both MAE and RMSE values for CH4 showed 30-fold variations among sites.
Variability of CH4 flux and GPP dynamic among three sites
Modeled GPP exhibited large variabilities among sites (). The marshland wetland in the Sanjiang Plain has an annual modeled GPP of 589.1 g C/m2/year; the annual GPP was simulated to be 1319.0 g C/m2/year for the wetlands in the Changbai Mountain, and modeled 171.3 g C/m2/year for the swamp in the Lesser Khingan Mountain. For all three sites, the GPP reached its highest value in the summers of the year. The daily modeled maximum values of GPP in the Sanjiang Plain, Changbai Mountain, and Lesser Khingan Mountain were 5.8 g C/m2/d, 12.5 g C/m2/d, and 1.9 g C/m2/d, respectively. Compared with the Sanjiang Plain, the simulated GPP of Changbai Mountain and Lesser Khingan Mountain has a much higher fluctuation.
Figure 2. Scatter plots of the observed and simulated GPP (a-c) and CH4 (d-f) for the Sanjiang Plain, Changbai Mountain, and Lesser Khingan Mountain. The Orange dots represent GPP, and the green dots represent CH4; solid lines are the regression, dash lines are the 1:1 line.

Meanwhile, there were large variations in modeled CH4 flux among sites, with a range of 0.34 to 26.9 g C/m2/year. The annual modeled CH4 emissions in the Sanjiang Plain were the largest at 26.9 g C/m2/year, followed by the Lesser Khingan Mountain at 0.66 g C/m2/year, and the Changbai Mountain has the smallest modeled CH4 emissions at only 0.34 g C/m2/year. The Sanjiang Plain freshwater marsh wetlands, as the largest source of CH4 emissions, have released 79 times more annual CH4 flux than the Mountain peatland (Lesser Khingan Mountain). The peak modeled CH4 flux occurred in the summer period, and the maximum modeled daily emissions of Sanjiang Plain, Changbai Mountain, and Lesser Khingan Mountain were 247.9 mg C/m2/year, 4.2 mg C/m2/year, and 5.9 mg C/m2/year, respectively.
Modeled biomass of methanogens
The modeled microbial biomass for methanogenesis showed obvious seasonality at different depths at three sites (). There was almost no fluctuation of microbial biomass in spring and winter. The microbial biomass of Lesser Khingan Mountain began to increase suddenly from mid-July and then dropped to stabilize in early October. But there was almost no seasonal change in acetoclastic methanogens biomass below 40 cm (). Different from Lesser Khingan Mountain, the seasonal changes of methanogenesis in the Sanjiang Plain and the Changbai Mountain were relatively similar, strongly fluctuating between April and October ( a-4b, d-4e).
Figure 3. Observed and the modeled (a-c) GPP and (d-f) CH4. (a), Sanjiang Plain (b), Changbai Mountain (c), Lesser Khingan Mountain. DOY: day of the year.

There are significant differences in biomass of methanogens at the three sites (). The simulation results showed that Changbai Mountain has the largest acetoclastic methanogens biomass, with a mean of 1.37e−4 mol/m3, followed by Lesser Khingan Mountain and Sanjiang Plain, with a mean of 2.32e−6 and 1.57e−9 mol/m3, respectively. The Sanjiang Plain had the largest hydrogenotrophic methanogens biomass, followed by Changbai Mountain and Lesser Khingan Mountain, with a mean value of 2.55e−6, 1.85e−6, and 7.47e−7 mol/m3, respectively.
Modeled CH4 transport pathway among three sites
Modeled CH4 flux showed that wetland types had a profound impact on CH4 transport pathways (). The modeled CH4 flux showed obvious seasonality in plant-mediated transport, diffusion, and ebullition at Sanjiang Plain and Changbai Mountain, while the plant-mediated transport did not show a clear seasonal pattern in Lesser Khingan Mountain. The model estimated that the main CH4 transport pathway in the wetland was ebullition at an annual scale. The contribution of ebullition transmission in the freshwater wetland (Sanjiang Plain) to CH4 emissions is lower than that of mountain peatlands (Changbai Mountain and Lesser Khingan Mountain), which are 58.96% in Sanjiang Plain, 82.41% in Changbai Mountain, and 71.06% in Lesser Khingan Mountain. Plant-mediated transport in freshwater wetland, as the second-largest transport pathway after ebullition, is significantly different from mountain peatlands. Among them, the contribution rate of plant-mediated transport in Lesser Khingan Mountain was only 0.003%, which was almost negligible (). Diffusion, as the second-largest transport pathway of alpine peatlands, has the smallest contribution to CH4 emissions in freshwater wetlands indeed. In addition, the simulation results can clearly foresee the early spring CH4 pulse when the frozen soil melts in the spring ().
Sensitivity analysis
The dynamics of CH4 and GPP are sensitive to some parameters of photosynthesis, plant growth respiration, maintenance respiration, decomposition, CH4 production, growth and death of methanogens, and growth and death of methanotrophs (). The simulated CH4 fluxes were sensitive to most of the 18 key parameters, while GPP was sensitive to the parameters controlling respiration and photosynthesis. Specifically, the CH4 flux was strongly sensitive to the parameters of GrowRmethanotrophs, AceProdAcemax, KAce, DeadACEMethanogens, GrowACEMethanogens for Sanjiang Plain and Changbai Mountain, which means that acetate production and methanotrophs were the key controls on CH4 flux. The changes of GrowRmethanotrophs are significantly different in the CH4 flux response between the two wetland types. In freshwater wetland (S = 0.02 for CH4 flux with −20% change and S = 0.02 for CH4 flux with +20% change in Sanjiang Plain), whether the GrowRmethanotrophs increase or decreases, the CH4 flux shows an upward trend, but the opposite is true in mountain peatlands (S = −1.14 for CH4 flux with −20% change and S = −2.34 for CH4 flux with +20% change in Changbai Mountain). The magnitude of the AceProdAcemax on CH4 flux were the same between Sanjiang Plain (S = 0.18 for CH4 flux with −20% change and S = 0.08 for CH4 flux with +20% change) and Changbai Mountain (S = 0.63 for CH4 flux with −20% change and S = 0.50 for CH4 flux with +20% change). But it showed a positive correlation in Lesser Khingan Mountain (S = −0.03 for CH4 flux with −20% change and S = 0.04 for CH4 flux with +20% change).
Figure 5. The CLM-Microbe model simulated CH4 transport pathways and their contributions to the annual CH4 flux at three sites. (a) Sanjiang Plain; (b) Changbai Mountain; (c) Lesser Khingan Mountain; DOY = day of the year.

In addition, photosynthesis and DOC also affect CH4 production. In the freshwater wetland, CH4 flux was sensitive to the fraction of leaf nitrogen in the Rubisco enzyme functioning in photosynthesis (flnr). The high response was in freshwater marshes (S = 1.44 for CH4 flux with −20% change and S = −1.11 for CH4 flux with +20% change in Sanjiang Plain), followed by peatlands (S = 0.14 for CH4 flux with −20% change and S = 0.07 for CH4 flux with +20% change in Changbai Mountain). CH4 flux also responded to changes in autotrophic respiration (grperc and br_mr; ). In the mountain peatlands (Changbai Mountain and Lesser Khingan Mountain), the parameters of the acetic acid methanogenesis pathway are still the main factors affecting CH4 production. But its sensitivity is weaker than that of freshwater marshes ( b and c).
The most important processes of GPP dynamics are related to photosynthesis and respiration, which control C fixation and release from wetlands. The flnr and grperc were identified as the primary factor for GPP, NPP, ER, and NEE (). As increases in the flnr lead to decrease in GPP, NEE, ER and NPP in the Sanjiang Plain (S = −1.71 for GPP, S = −2.49 for NEE, S = −1.61 for ER, and S = −1.47 for NPP with +20% change) but GPP, NEE, ER and NPP raise in the Changbai Mountain (S = 0.32 for GPP, S = 0.37 for NEE, S = 0.32 for ER, and S = 0.35 for NPP with +20% change) and Lesser Khingan Mountain (S = 1.00 for GPP, S = 0.58 for NEE, S = 0.93 for ER and, S = 0.47 for NPP with +20% change). There was a significant increase in GPP, NEE, ER and NPP for all sites with decreased flnr. As decrease in the grperc lead to decrease in GPP, ER and NPP in the Sanjiang Plain and Lesser Khingan Mountain but a NEE rise in the Changbai Mountain. In addition, GPP, NEE, ER and NPP were sensitive to maintenance respiration (br_mr) and froot_leaf in the Sanjiang Plain ().
Discussions
Simulation of CH4 emissions from wetlands in Northeast China
The CLM-Microbe model produced consistent results with the observed CH4 flux and GPP in wetland ecosystems (). However, we also observed poor CH4 flux simulation results at some sites, such as CH4 flux at Changbai Mountain and Lesser Khingan Mountain (, ). There may be several reasons for the relatively poor performance of the CLM-Microbe model at those sites. First, the available observational data were not too large, resulting in small R2 values at those sites. The R2 is not suitable for assessing the goodness-of-fit for a small amount of data due to the large bias in small samples (He et al., Citation2021a). Compared with the Sanjiang Plain, we observed smaller MAE and RMSE values in Changbai Mountain and Lesser Khingan Mountain. Second, the difference in measurements methods for CH4 flux is also an important factor. In the method section, we mentioned that the eddy covariance technique is used to measure CH4 flux in Sanjiang Plain, while the static chamber approach is used in Changbai Mountain and Lesser Khingan Mountain. As we know, the static chamber approach has its inherent shortcomings. It changes the temperature and pressure in the box, destroys the soil environment, and affects CH4 emissions. In addition, this method has large spatial variability and large single-point observation errors, requiring a lot of repetition, and it is difficult to achieve long-term continuous observation (Wang et al. Citation2013). The biggest advantage of the eddy covariance technique is to achieve long-term continuous observation of large-scale ecosystems without damaging the environment (Wang et al. Citation2013). The inter-annual differences in CH4 flux measured by the static chamber approach are obvious. Third, although the microbial processes of CH4 production and oxidation are included in our model, the lack of microbial data makes it difficult to verify the microbial biomass changes of methanogens. This might be one of the reasons for relatively low model performances. Yihui et al. (Citation2019) also pointed out the impact of topography on simulations should be considered when using the CLM-Microbe model.
Figure 6. Sensitivity analysis of the CLM-Microbe model in terms of gross primary productivity (GPP), net ecosystem carbon exchange (NEE), ecosystem respiration (ER), net primary productivity (NPP), and methane (CH4) flux to 18 parameters (KAce, AceProdACmax, GrowAceMethanogens, GrowH2Methanogens, GrowRMethanotrophs, DeadAceMethanogens, DeadH2Methanogens, DeadRMethanotrophs, YAceMethanogens, YH2Methanogens, YMethanotrophs, k_dom, dom_diffus, froot_leaf, flnr, grperc, bdnr, and br_mr) for (a) Sanjiang Plain, (b)Changbai Mountain, and (c) Lesser Khingan Mountain. The symbols “+”and “‐” indicate a 20% increase or 20% decrease of parameter values. Darker red and darker blue indicate a stronger positive or negative model response to a parameter change. S is negative if the direction of model response opposes the direction of a parameter change, and vice versa.

Wetland types impacts on CH4 flux
Our results show that there are significant differences in GPP and CH4 flux between freshwater wetlands and peatlands, which is mainly due to differences in vegetation composition (). The dominant species in the Sanjiang Plain are aerenchyma sedge plants, while the Changbai Mountain is dominated by Carex sphagnum and shrubs, and the Lesser Khingan Mountain is dominated by sphagnum moss. There are obvious differences in plant species and productivity among wetland types, and this difference leads to differences in CH4 flux (Ding et al. Citation2002). Through the simulation results of the model, we found that there is a large difference in plant-mediated transport between the three sites. The plant-mediated transport in the Sanjiang Plain accounted for 23.96%, while in Lesser Khingan Mountain only accounts for 0.003% of CH4 emissions (). Plant species are important factors influencing the ability of plants to transport CH4 (Sun et al. Citation2012; Bhullar et al., Citation2014; Han et al. Citation2017; Lawrence et al. Citation2017). Previous studies have pointed out that the plant-mediated transport pathway of the C. lasiocarpa wetland only accounts for about 30% of CH4 emissions, which is similar to our results (Sun et al. Citation2012; Ding, Cai, and Wang Citation2004). In addition, not only due to the high capacity for freshwater marsh plants to transport CH4 into the atmosphere but due to more plant litter inundated in the standing water, which could provide substrate for CH4 production (Ding et al. Citation2002).
Through sensitivity analysis, we found that the rate of microbial growth and death is a key constraint on CH4 dynamics in wetlands (Xiaofeng et al. Citation2015; Hernández et al. Citation2017). In the summer months, higher microbial biomass was associated with a higher CH4 flux in the wetland ( and ). The results implied that a positive correlation existed between the microbial biomass and CH4 flux. Additionally, the microbial composition also has a significant impact on the methanogenic pathway in wetlands (Xiaofeng et al. Citation2015; Xueyang et al. Citation2020). The acetotrophic and hydrogenotrophic pathways have been reported to be the main methanogenic pathway in most environments (Angle et al. Citation2017; Thauer Citation1998; Zhang et al. Citation2019). Freshwater marshes are dominated by hydrogenotrophic methanogens, while alpine peatlands are dominated by acetoclastic methanogens (), which can indicate that the Changbai Mountain and Lesser Khingan Mountain are mainly produced by aceticlastically (by disproportionation of acetate to CO2 and CH4), and the Sanjiang Plain is dominated by hydrogenotrophic methanogenesis (CO2+ H2) (Hernández et al. Citation2017).
Model implications
This study has dual implications for model development and understanding CH4 mechanisms in natural wetlands of northeastern China. The successful application of the CLM-Microbe model in simulating CH4 flux in regions beyond of its original domain indicates the feasibility of the model structure. Meanwhile, the simulated dynamics of methanogenesis are consistent with the CH4 production, suggesting the microbial dominance on CH4 production. Many previous studies reported the predominance of substrates or water table on CH4 flux (Whiting and Chanton Citation1993; Lipson et al. Citation2012; Zona et al. Citation2009); the causes might be the microbial responses to water table and ignorance of microbial mechanisms in those studies. Further genomic analysis in association with CH4 processes should provide critical information for model development and validation (Xiaofeng et al. Citation2015).
The implication of CH4 budget is primarily for the wetlands in Northeast China. Northeast China features the largest freshwater wetlands in China, and the natural wetlands can be found in mountains and plains. The large C storage in these ecosystems will likely be decomposed by microorganisms and released in the form of CH4 when the climate continues warming (Song et al. Citation2012; Wang et al. Citation2017). In this study, the CLM-Microbe model performed well in capturing the variabilities in GPP and CH4 flux for primary wetland types in northeastern China, which emphasizes the importance of spatial heterogeneity in simulating CH4 flux in wetland ecosystem CH4 models. The simulated differences in GPP and CH4 emissions between freshwater wetlands and peatlands are consistent with previous studies (Sun et al. Citation2018; Xueyang et al. Citation2020). Furthermore, the sensitivity analysis showed that the photosynthetic (flnr) and respiratory (br_mr) parameters of plants are highly sensitive at all sites. Therefore, plant composition is one of the main reasons for the difference in CH4 emissions. In addition, the peak CH4 emission varies among different wetland types, especially in Sanjiang Plain and Lesser Khingan Mountain. The reason may be that Lesser Khingan Mountain is located in the permafrost region, and the methanogens reach their maximum activity at the end of the growing season, thereby increasing the CH4 flux (Sun et al. Citation2018). Given the changing environment, the differences in CH4 flux among wetlands call for accurate estimations of regional CH4 flux at the regional scale (Song et al. Citation2019).
The prospect
With a modeling study, we found that environmental factors affect CH4 emission by influencing microbial processes in the natural wetlands. The important role of microorganisms in the wetland ecosystem was demonstrated (Given and Dickinson Citation1975; He et al., Citation2020a; Villa Citation2020; Wallenius et al. Citation2021; Xiaofeng et al. Citation2015; Xu et al., 2016; Zhang et al. Citation2020a). Although the results of this paper prove that the CLM-Microbe model can simulate the CH4 flux in northern wetlands, follow-up works are still needed. First, we have recognized the importance of microorganisms in the production and oxidation of CH4 in wetlands; genomic data in association with the microbial function should be thus used for model parameterization. This allows the model to more accurately capture the dynamics in CH4 production and oxidation. Second, with a warming climate, the area of permafrost has shrunk, and the active layer has deepened, which has caused a substantial impact on the C budget in wetlands. However, the scarcity of CH4 flux data caused difficulties in model validation and application. We call for the monitoring of specific CH4 processes and the establishment of an open data platform, such as ChinaFLUX and FLUXNET, to further develop the model.
Conclusions
We parameterized the CLM-Microbe model against the field observational data of CO2 and CH4 flux in three major wetland types in northeastern China and then applied the model to understand processes and environmental factors affecting GPP and CH4 flux at different wetland types in northeastern China. The results showed that the CLM-Microbe model was able to reconstruct the observed GPP dynamics and CH4 flux. The modeled results showed that freshwater marsh wetlands (Sanjiang Plain) have higher CH4 emissions than mountain peatlands (Changbai Mountain and Lesser Khingan Mountain). However, Changbai Mountain has the largest GPP, followed by Sanjiang Plain, and Lesser Khingan Mountain has the smallest. The model estimated that the main CH4 flux transport pathway in the wetland was ebullition at an annual time scale. The modeled results showed that the freshwater marsh wetlands mainly use the hydrogenotrophic CH4 production, and the mountain peatlands mainly use the process of acetolactic CH4 production. Model sensitivity analysis determined that the growth and death rate of microorganisms and the availability of substrates are the most important factors in controlling CH4 emissions from wetland ecosystems. Photosynthesis and respiration have a more significant impact on GPP, NEE, ER, and NPP. Our finding highlights the importance of explicit representation of microbial mechanisms on C cycling in northeastern China, which will improve the simulation performance of CH4 cycling under climate change. Our results also provide an important scientific basis for the quantification and prediction of the CH4 budget in wetland areas in northeastern China.
Disclosure statement
No potential conflict of interest was reported by the author(s).
Correction Statement
This article has been republished with minor changes. These changes do not impact the academic content of the article.
Additional information
Funding
References
- Angle, J. C., T. H. Morin, L. M. Solden, A. B. Narrowe, G. J. Smith, M. A. Borton, C. Rey-Sanchez, et al. 2017. “Methanogenesis in Oxygenated Soils Is a Substantial Fraction of Wetland Methane Emissions.” Nature Communications 8 (1): 1567. doi:10.1038/s41467-017-01753-4.
- Bhullar, G. S., P. J. Edwards, H. Olde Venterink, and F.-H. Yu. 2014. “Influence of Different Plant Species on Methane Emissions from Soil in a Restored Swiss Wetland.” Plos One 9 (2): e89588. doi:10.1371/journal.pone.0089588.
- Bousquet, P., P. Ciais, J. B. Miller, E. J. Dlugokencky, D. A. Hauglustaine, C. Prigent, G. R. Van der Werf, et al. 2006. “Contribution of Anthropogenic and Natural Sources to Atmospheric Methane Variability.” Nature 443 (7110): 439–14. doi:10.1038/nature05132.
- Bridgham, S. D., H. Cadillo-Quiroz, J. K. Keller, and Q. Zhuang. 2013. “Methane Emissions from Wetlands: Biogeochemical, Microbial, and Modeling Perspectives from Local to Global Scales.” Global Change Biology 19 (5): 1325–1346. doi:10.1111/gcb.12131.
- Cao, N. 2015. Study on the carbon dioxide flux in Peatland of volcanic cluster in the longgang region at changbai mountain —Take the Jinchuan peatland as an example . Master. Northeast Normal University.
- Chadburn, S. E., T. Aalto, M. Aurela, D. Baldocchi, C. Biasi, J. Boike, E. J. Burke, et al. 2020. “Modeled Microbial Dynamics Explain the Apparent Temperature Sensitivity of Wetland Methane Emissions.” Global Biogeochemical Cycles 34 (11): e2020GB006678. doi:10.1029/2020GB006678.
- Cordruwisch, R., H. J. Seitz, and R. Conrad. 1988. “The Capacity of Hydrogenotrophic Anaerobic-Bacteria to Compete for Traces of Hydrogen Depends on the Redox Potential of the Terminal Electron-Acceptor.” Archives of Microbiology 149 (4): 350–357. doi:10.1007/Bf00411655.
- Ding, W., Z. Cai, H. Tsuruta, and L. Xiaoping. 2002. “Effect of Standing Water Depth on Methane Emissions from Freshwater Marshes in Northeast China.” Atmospheric Environment 36 (33): 5149–5157. doi:10.1016/S1352-2310(02)00647-7.
- Ding, W., Z. Cai, and D. Wang. 2004. “Preliminary Budget of Methane Emissions from Natural Wetlands in China.” Atmospheric Environment 38 (5): 751–759. doi:10.1016/j.atmosenv.2003.10.016.
- Fazli, H. C., U. K. M. Man, and A., . I. Shah. 2013. “Characteristics of Methanogens and Methanotrophs in Rice Fields: A Review.” Asia Pac J Mol Biol Biotechnol 21 (1): 3–17.
- Given, P. H., and C. H. Dickinson. 1975. “Biochemistry and Microbiology of Peats.” Soil Biochemistry, 3:123–212 .
- Han, W., M. Shi, J. Chang, Y. Ren, R. Xu, C. Zhang, and Y. Ge. 2017. “Plant Species Diversity Reduces N2O but Not CH4 Emissions from Constructed Wetlands under High Nitrogen Levels.” Environmental Science and Pollution Research 24 (6): 5938–5948. doi:10.1007/s11356-016-8288-3.
- Han, Y., M. Wang, S. Wang, Y. Dong, S. Liu, and X. Zhiwei. 2018. “Characteristics of Soil Enzyme Activity of Peat Bog in Jinchuan, Changbai Mountain.” Wetland Science 16 (5): 671–678. doi:10.13248/j.cnki.wetlandsci.2018.05.014.
- Hanqin, T., L. Chaoqun, C. Philippe, A. M. Michalak, J. G. Canadell, S. Eri, D. N. Huntzinger, et al. 2016. “The Terrestrial Biosphere as a Net Source of Greenhouse Gases to the Atmosphere.” Nature 531 (7593): 225–+. doi:10.1038/nature16946.
- Harmsen, M., D. P. van Vuuren, B. L. Bodirsky, J. Chateau, O. Durand-Lasserve, L. Drouet, O. Fricko, S. Fujimori, D. E. H. J. Gernaat, and T. Hanaoka. 2020. “The Role of Methane in Future Climate Strategies: Mitigation Potentials and Climate Impacts.” Climatic Change 163 (3): 1409–1425. doi:10.1007/s10584-019-02437-2.
- He, L., Chun-Ta. L, Melanie A. M, Shohei. M, Xiaofeng. X. 2021a. “Microbial seasonality promotes soil respiratory carbon emission in natural ecosystems: A modeling study”. Global Change Biology, 27(13): 3035-3051. https://doi.org/10.1111/gcb.15627.
- He, L., David, A. L, Jorge L. M. R, Melanie. M, Robert G. B, Bruno. G, Peter. T, Xiaofeng. X. 2021b. “Dynamics of Fungal and Bacterial Biomass Carbon in Natural Ecosystems: Site-Level Applications of the CLM-Microbe Model”. Journal of Advances in Modeling Earth Systems 13: (2) e2020MS002283. https://doi.org/10.1029/2020MS002283.
- Hernández, M., R. Conrad, M. Klose, K. Ma, and L. Yahai. 2017. “Structure and Function of Methanogenic Microbial Communities in Soils from Flooded Rice and Upland Soybean Fields from Sanjiang Plain, NE China.” Soil Biology and Biochemistry 105: 81–91. doi:10.1016/j.soilbio.2016.11.010.
- Huang, Y., R. L. Sass, and F. M. Fisher Jr. 1998. “A semi-empirical Model of Methane Emission from Flooded Rice Paddy Soils.” Global Change Biology 4 (3): 247–268. doi:10.1046/j.1365-2486.1998.00129.x.
- Huang, Shizhu 2016 Response of CH4 and N2O emission from peatlands to changed environments in Little Xing'an Mountains, northeat China . . Doctor. Northeast Forestry University.
- IPCC, 2017. “IPCC Fifth Assessment Report (AR5) Observed Climate Change Impacts Database, Version 2.01.” NASA Socioeconomic Data and Applications Center (SEDAC), Palisades, NY.
- Jackson, R. B., M. Saunois, P. Bousquet, J. G. Canadell, B. Poulter, A. R. Stavert, P. Bergamaschi, Y. Niwa, A. Segers, and A. Tsuruta. 2020. “Increasing Anthropogenic Methane Emissions Arise Equally from Agricultural and Fossil Fuel Sources.” Environmental Research Letters 15 (7): 071002. doi:10.1088/1748-9326/ab9ed2.
- Kirschke, S., P. Bousquet, P. Ciais, M. Saunois, J. G. Canade ll, E. J. Dlugokencky, P. Bergamaschi, et al. 2013. “Three Decades of Global Methane Sources and Sinks.” Nature Geoscience 6 (10): 813–823. doi:10.1038/ngeo1955.
- Koven, C. D., W. J. Riley, Z. M. Subin, J. Y. Tang, M. S. Torn, W. D. Collins, G. B. Bonan, D. M. Lawrence, and S. C. Swenson. 2013. “The Effect of Vertically Resolved Soil Biogeochemistry and Alternate Soil C and N Models on C Dynamics of CLM4.” Biogeosciences 10 (11): 7109–7131. doi:10.5194/bg-10-7109-2013.
- Lawrence, B. A., S. C. Lishawa, N. Hurst, B. T. Castillo, and N. C. Tuchman. 2017. “Wetland Invasion by Typha× Glauca Increases Soil Methane Emissions.” Aquatic Botany 137: 80–87. doi:10.1016/j.aquabot.2016.11.012.
- Le, M., and R. P. Jean. 2001. “Production, Oxidation, Emission and Consumption of Methane by Soils: A Review.” European Journal of Soil Biology 37 (1): 25–50. doi:10.1016/S1164-5563(01)01067-6.
- Lipson, D. A., D. Zona, T. K. Raab, F. Bozzolo, M. Mauritz, and W. C. Oechel. 2012. “Water-table Height and Microtopography Control Biogeochemical Cycling in an Arctic Coastal Tundra Ecosystem.” Biogeosciences 9 (1): 577–591. doi:10.5194/bg-9-577-2012.
- Liyuan, H., C.-T. Lai, M. A. Mayes, S. Murayama, and X. Xu. 2021a. “Microbial Seasonality Promotes Soil Respiratory Carbon Emission in Natural Ecosystems: A Modeling Study.” Global Change Biology 27 (13): 3035–3051. doi:10.1111/gcb.15627.
- Liyuan, H., D. A. Lipson, M. Rodrigues, L. Jorge, M. Melanie, R. G. Björk, G. Bruno, T. Peter, and X. Xiaofeng. 2021b. “Dynamics of Fungal and Bacterial Biomass Carbon in Natural Ecosystems: Site-Level Applications of the CLM-Microbe Model.” Journal of Advances in Modeling Earth Systems 13 (2): e2020MS002283. doi:10.1029/2020MS002283.
- Maosheng, Z., F. A. Heinsch, R. R. Nemani, and S. W. Running. 2005. “Improvements of the MODIS Terrestrial Gross and Net Primary Production Global Data Set.” Remote Sensing of Environment 95 (2): 164–176. doi:10.1016/j.rse.2004.12.011.
- McGlynn, S. E. 2017. “Energy Metabolism during Anaerobic Methane Oxidation in ANME Archaea.” Microbes and Environments 32 (1): 5–13. doi:10.1264/jsme2.ME16166.
- Melton, J. R., R. Wania, E. L. Hodson, B. Poulter, B. Ringeval, R. Spahni, T. Bohn, et al. 2013. “Present State of Global Wetland Extent and Wetland Methane Modelling: Conclusions from a Model inter-comparison Project (WETCHIMP).” Biogeosciences 10 (2): 753–788. doi:10.5194/bg-10-753-2013.
- Meng, L., P. G. M. Hess, N. M. Mahowald, J. B. Yavitt, W. J. Riley, Z. M. Subin, D. M. Lawrence, S. C. Swenson, J. Jauhiainen, and D. R. Fuka. 2012. “Sensitivity of Wetland Methane Emissions to Model Assumptions: Application and Model Testing against Site Observations.” Biogeosciences 9 (7): 2793–2819. doi:10.5194/bg-9-2793-2012.
- Morin, T. H., G. Bohrer, R. P. D. M. Frasson, L. Naor-Azreli, S. Mesi, K. C. Stefanik, and K. V. R. Schäfer. 2014. “Environmental Drivers of Methane Fluxes from an Urban Temperate Wetland Park.” Journal of Geophysical Research: Biogeosciences 119 (11): 2188–2208. doi:10.1002/2014JG002750.
- Nzotungicimpaye, C.-M., A. H. MacDougall, J. R. Melton, C. C. Treat, E. Michael, L. F. W. Lesack, and K. Zickfeld. 2020. “WETMETH 1.0: A New Wetland Methane Model for Implementation in Earth System Models.” Geoscientific Model Development Discussions 1–40. doi:10.5194/gmd-2020-176.
- Saunois, M., A. R. Stavert, B. Poulter, P. Bousquet, J. G. Canadell, R. B. Jackson, P. A. Raymond, et al. 2020. “The Global Methane Budget 2000-2017”.” Earth System Science Data 12 (3): 1561–1623. doi:10.5194/essd-12-1561-2020.
- Schaefer, H. 2019. “On the Causes and Consequences of Recent Trends in Atmospheric Methane.” Current Climate Change Reports 5 (4): 259–274. doi:10.1007/s40641-019-00140-z.
- Shi, Yao 2019 Effects of nitrogen input on carbon and nitrogen transformations in peatlands . Doctor, Northeast Normal University.
- Sieber, J. R., M. J. McInerney, and R. P. Gunsalus. 2012. “Genomic Insights into Syntrophy: The Paradigm for Anaerobic Metabolic Cooperation.” Annual Review of Microbiology 66 (1): 66(429–452. doi:10.1146/annurev-micro-090110-102844.
- Song, C., X. Xiaofeng, X. Sun, H. Tian, L. Sun, Y. Miao, X. Wang, and Y. Guo. 2012. “Large Methane Emission upon Spring Thaw from Natural Wetlands in the Northern Permafrost Region.” Environmental Research Letters 7 (3). doi:10.1088/1748-9326/7/3/034009.
- Song, Y., C. Song, J. Ren, M. Xiuyan, W. Tan, X. Wang, J. Gao, and A. Hou. 2019. “Short-Term Response of the Soil Microbial Abundances and Enzyme Activities to Experimental Warming in a Boreal Peatland in Northeast China.” Sustainability 11 (3): 590. doi:10.3390/su11030590.
- Sun, X., C. Song, Y. Guo, X. Wang, G. Yang, L. Yingchen, R. Mao, and L. Yongzheng. 2012. “Effect of Plants on Methane Emissions from a Temperate Marsh in Different Seasons.” Atmospheric Environment 60 (277): 277–282. doi:10.1016/j.atmosenv.2012.06.051.
- Sun, L., S. Changchun, P. M. Lafleur, M. Yuqing, W. Xianwei, G. Chao, Q. Tianhua, Y. Xueyang, and W. Tan. 2018. “Wetland-atmosphere Methane Exchange in Northeast China: A Comparison of Permafrost Peatland and Freshwater Wetlands.” Agricultural and Forest Meteorology 249 (239): 239–249. doi:10.1016/j.agrformet.2017.11.009.
- Thauer, R. K. 1998. “Biochemistry of Methanogenesis: A Tribute to Marjory Stephenson: 1998 Marjory Stephenson Prize Lecture.” Microbiology 144 (9): 2377–2406. doi:10.1099/00221287-144-9-2377.
- Thauer, R. K., A. K. Kaster, H. Seedorf, W. Buckel, and R. Hedderich. 2008. “Methanogenic Archaea: Ecologically Relevant Differences in Energy Conservation.” Nature Reviews Microbiology 6 (8): 579–591. doi:10.1038/nrmicro1931.
- Thornton P E and Rosenbloom N A. (2005). Ecosystem model spin-up: Estimating steady state conditions in a coupled terrestrial carbon and nitrogen cycle model. Ecological Modelling, 189(1–2), 25–48. 10.1016/j.ecolmodel.2005.04.008
- Thornton, P. E., J.-F. Lamarque, N. A. Rosenbloom, and N. M. Mahowald. 2007. “Influence of carbon-nitrogen Cycle Coupling on Land Model Response to CO2 Fertilization and Climate Variability.” Global Biogeochemical Cycles 21 (4). doi:10.1029/2006GB002868.
- Timmers, P. H. A., C. U. Welte, J. J. Koehorst, C. M. Plugge, M. S. M. Jetten, and A. J. M. Stams. 2017. “Reverse Methanogenesis and Respiration in Methanotrophic Archaea.” Archaea-an International Microbiological Journal 2017. doi:10.1155/2017/1654237.
- Tingting, L., L. Yanyu, Y. Lingfei, W. Sun, Q. Zhang, W. Zhang, G. Wang, et al. 2020. “Evaluation of CH4MOD(wetland) and Terrestrial Ecosystem Model (TEM) Used to Estimate Global CH4 Emissions from Natural Wetlands.” Geoscientific Model Development 13 (8): 3769–3788. doi:10.5194/gmd-13-3769-2020.
- Villa, J. A. 2020. “Functional Representation of Biological Components in Methane-Cycling Processes in Wetlands Improves Modeling Predictions.” Journal of Geophysical Research: Biogeosciences 125 (10): e2020JG005794. doi:10.1029/2020JG005794.
- Wallenius, A. J., D. Martins, S. Paula, P. Caroline, and M. S. M. Jetten. 2021. “Anthropogenic and Environmental Constraints on the Microbial Methane Cycle in Coastal Sediments.” Frontiers in Microbiology 12 (293). doi:10.3389/fmicb.2021.631621.
- Wang, J. M., J. G. Murphy, J. A. Geddes, C. L. Winsborough, N. Basiliko, and S. C. Thomas. 2013. “Methane Fluxes Measured by Eddy Covariance and Static Chamber Techniques at a Temperate Forest in Central Ontario, Canada.” Biogeosciences 10 (6): 4371–4382. doi:10.5194/bg-10-4371-2013.
- Wang, J., C. Song, A. Hou, and X. Fengming. 2017. “Methane Emission Potential from Freshwater Marsh Soils of Northeast China: Response to Simulated Freezing-Thawing Cycles.” Wetlands 37 (3): 437–445. doi:10.1007/s13157-017-0879-3.
- Wang, Y., F. Yuan, K. A. Arndt, J. Liu, L. He, Y. Zuo, D. Zona, et al. 2022. “Upscaling Methane Flux from plot-level to Eddy Covariance Tower Domains by Combining the CLM-Microbe Model with Three Footprint Models.” Atmospheric Environment Under review
- Wania, R., I. Ross, and I. C. Prentice. 2010. “Implementation and Evaluation of a New Methane Model within a Dynamic Global Vegetation Model: LPJ-WHyMe v1.3.1.” Geoscientific Model Development 3 (2): 565–584. doi:10.5194/gmd-3-565-2010.
- Wania, R., J. R. Melton, E. L. Hodson, B. Poulter, B. Ringeval, R. Spahni, T. Bohn, et al. 2013. “Present State of Global Wetland Extent and Wetland Methane Modelling Methodology of a Model inter-comparison Project (WETCHIMP).” Geosci. Model Dev 6 (3): 617–641. doi:10.5194/gmd-6-617-2013.
- Whiting, G. J., and J. P. Chanton. 1993. “Primary Production Control of Methane Emission from Wetlands.” Nature 364 (6440): 794–795. doi:10.1038/364794a0.
- Xiaofeng, X., and H. Tian. 2012. “Methane Exchange between Marshland and the Atmosphere over China during 1949-2008.” Global Biogeochemical Cycles 26 (2). doi:10.1029/2010gb003946.
- Xiaofeng, X., J. P. Schimel, P. E. Thornton, S. Xia, Y. Fengming, and S. Goswami. 2014. “Substrate and Environmental Controls on Microbial Assimilation of Soil Organic Carbon: A Framework for Earth System Models.” Ecology Letters 17 (5): 547–555. doi:10.1111/ele.12254.
- Xiaofeng, X., D. A. Elias, D. E. Graham, T. J. Phelps, S. L. Carroll, S. D. Wullschleger, and P. E. Thornton. 2015. “A Microbial Functional group-based Module for Simulating Methane Production and Consumption: Application to an Incubated Permafrost Soil.” Journal of Geophysical Research Biogeosciences 120 (7): 1315–1333. doi:10.1002/2015JG002935.
- Xiaofeng, X., Y. Fengming, J. H. Paul, D. W. Stan, E. T. Peter, J. R. William, S. Xia, E. G. David, S. Changchun, and T. Hanqin. 2016. “Reviews and Syntheses: Four Decades of Modeling Methane Cycling in Terrestrial Ecosystems.” Biogeosciences, 1–56. doi:10.5194/bg-2016-37.
- Xueyang, Y., C. Song, L. Sun, X. Wang, and W. Tan. 2020. “Towards an Improved Utilization of Eddy Covariance Data: Growing Season CO2 Exchange from a Permafrost Peatland in the Great Hing’an Mountains, Northeast China.” Ecological Indicators 115: 106427. doi:10.1016/j.ecolind.2020.106427.
- Yihui, W., Fengming. Y., F. Yuan., Baohua. G., Melanie S. H., MS. Torn., Daniel M. R., Jitendra. K., Liyuan. H., Donatella. Z., David A. L., Robert. W. Walter C. O., Stan D. W., Peter E. T., Xiaofeng. X. 2019. “Mechanistic Modeling of Microtopographic Impacts on CO2 and CH4 Fluxes in an Alaskan Tundra Ecosystem Using the CLM-Microbe Mode.” Journal of Advances in Modeling Earth Systems 11 (12): 4288–4304 https://doi.org/10.1029/2019MS001771.
- Yihui, W., F. Yuan, F. Yuan, B. Gu, M. S. Hahn, M. S. Torn, D. M. Ricciuto, et al. 2019. “Mechanistic Modeling of Microtopographic Impacts on CO2 and CH4 Fluxes in an Alaskan Tundra Ecosystem Using the CLM-Microbe Model.” Journal of Advances in Modeling Earth Systems 11 (12): 4288–4304. doi:10.1029/2019MS001771.
- Youmi, O., Z. Qianlai, L. Licheng, L. R. Welp, M. C. Y. Lau, T. C. Onstott, M. David, et al. 2020. “Reduced Net Methane Emissions Due to Microbial Methane Oxidation in a Warmer Arctic.” Nature Climate Change 10 (4): 317–321. doi:10.1038/s41558-020-0734-z.
- Yuan, F., J. Liu, Y. Zuo, Z. Guo, N. Wang, C. Song, Z. Wang, et al. 2020. “Rising Vegetation Activity Dominates Growing Water Use Efficiency in the Asian Permafrost Region from 1900 to 2100.” Science of the Total Environment 736 (139587): 139587. doi:10.1016/j.scitotenv.2020.139587.
- Yuan, F., Y. Wang, D. Ricciuto, X. Shi, F. Yuan, T. Brehme, S. Bridgham, et al. 2021a. “Hydrological Feedbacks on Peatland CH4 Emission under Warming and Elevated CO2: A Modeling Study.” Journal of Hydrology 603: 127137. doi:10.1016/j.jhydrol.2021.127137.
- Yuan, F., Y. Wang, D. Ricciuto, X. Shi, F. Yuan, P. Hanson, S. Bridgham, J. Keller, P. Thornton, and X. Xiaofeng. 2021b. “An Integrative Model for Soil Biogeochemistry and Methane Processes: II. Warming and Elevated CO2 Effects on Peatland CH4 Emissions.” Journal of Geophysical Research: Biogeosciences 126. doi:10.1029/2020JG005963.
- Zhang, B., H. Tian, L. Chaoqun, G. Chen, S. Pan, C. Anderson, and B. Poulter. 2017. “Methane Emissions from Global Wetlands: An Assessment of the Uncertainty Associated with Various Wetland Extent Data Sets.” Atmospheric Environment 165: 310–321. doi:10.1016/j.atmosenv.2017.07.001.
- Zhang, Y., M. Anzhou, G. Zhuang, and X. Zhuang. 2019. “The Acetotrophic Pathway Dominates Methane Production in Zoige Alpine Wetland Coexisting with Hydrogenotrophic Pathway.” Scientific Reports 9 (1): 9141. doi:10.1038/s41598-019-45590-5.
- Zhang, H., D. Goll, Y. Wang, P. Ciais, W. Wieder, R. Abramoff, Y. Huang, et al. 2020a. “Microbial Dynamics and Soil Physicochemical Properties Explain Large Scale Variations in Soil Organic Carbon.” Global Change Biology 26 (4): 2668–2685. doi:10.1111/gcb.14994.
- Zona, D., W. C. Oechel, J. Kochendorfer, U. Paw, K. T. Salyuk, P. C. A. N., Olivas, S. F. Oberbauer, and D. A. Lipson. 2009. “Methane Fluxes during the Initiation of a large-scale Water Table Manipulation Experiment in the Alaskan Arctic Tundra.” Global Biogeochemical Cycles 23 (2). doi:10.1029/2009GB003487.