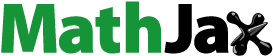
ABSTRACT
The destruction of the ecological system caused by urban expansion has led to the environmental deterioration, cities have become increasingly vulnerable. In this study, six districts and counties along the Yellow River in Zhengzhou were selected as the study area. First, green infrastructure elements were extracted by morphological spatial pattern analysis. Then, outside the urban areas, we used connectivity analysis to evaluate the importance of core areas, adopted minimum cumulative resistance model to extract potential corridors, and identified the important corridors by using the gravity model. Finally, in the urban areas, we set up an evaluation system to assess the demands for ecosystem services. The results showed that: (1) Seven landscape types of green infrastructure be identified in study area. (2) There are 17 vital cores, 136 potential corridors, and 24 vital corridors outside the urban areas. (3) The blocks with high demand for ecosystem services are mostly concentrated in the old blocks with dense populations and poor infrastructure, and there are 5 blocks with comprehensive high-demand. Based on identified importance for green infrastructure land space, and high-demand level for ecosystem services areas in this study, a green infrastructure net plan was proposed based on spatial conservation prioritisation.
Introduction
Amid rapid urbanization, ecosystems within and beyond cities are experiencing diminishing resilience to maintain their functions as biodiversity and ecosystem services (ES) are destroyed and the ecological network becomes seriously fragmented (Dawson et al. Citation2011; Grim et al. Citation2008; Fanhua Kong et al. Citation2012). It is estimated that 68% of the world’s growing population will live in urbanized areas by 2050; by the end of this century, 90% of the world’s population will live in cities (UN-DESA.Citation2019). Indeed, the capacity of cities to cope with natural disasters must be urgently strengthened. Therefore, apart from global sustainable development from an urban ecological perspective, China has also put forward high-quality development strategies at a national level (Roppongi Citation2017; Jin Fengjun Citation2019). Accordingly, protecting and extending green infrastructure (GI) is a sustainable strategy that can enhance the resilience of cities and their surrounding areas while improving ecological and landscape functions (Panagopoulos, Duque, and Dan Citation2016). Cities with ideal ES that are services from GI can attain ecosystem stability and human well-being (Biggs et al. Citation2012; Morley Citation1988).
A green infrastructure network (GIN) is a type of nature-based solution (Cohen- Shacham et al. Citation2016), able to serve multiple purposes, such as protecting biodiversity, combating climate change, creating recreational green spaces, and providing food and cultural services (European Environment Agency Citation2014). Therefore, in order to build a multidimensional and functional GIN, three main aspects must be integrated as “pillars” – biodiversity, landscape connectivity, and ES (European Environment Agency Citation2014; Wang and Banzhaf Citation2018). Furthermore, planners must determine cities’ most pressing needs and how the best results can be achieved. Visualizing areas that require priority maintenance enables decision-makers to achieve an optimal resource allocation for ecosystem maintenance (Firehock Citation2015). However, individual GIN research projects have a limited research focus, e.g, either climate modification, or food and water supply, or air quality (Demuzere et al. Citation2014; Lennon Citation2015; Pugh et al. Citation2012; Haines and Potschin Citation2010), food and water supply, (Artmann and Sartison Citation2018; Bennett, Peterson, and Gordon Citation2009) aesthetics and recreation, (Carlier and Moran Citation2019) soil and habitat support, (CitationBloomberg and Holloway) and production, living and ecological aspects as related to human wellbeing. (Patz and Norris Citation2004; Tzoulas et al. Citation2007).
When identifying and constructing GINs, relatively few cases take all three pillars into account; meanwhile, there is almost no research that prioritizes the conservation of urban nature in terms of GIN construction (Honeck et al. Citation2020). To take action, planners need to know where the most urgent needs are and where actions will deliver optimal results. Visualizing priority conservation areas can support decision-makers to optimally allocate limited resources for ecosystem preservation, and having such priority areas mapped out in advance saves time by avoiding conflicts when a key resource or environmental concern arises once a development project has been initiated (Firehock Citation2015). Compared to the traditional method of GI planning (that simply relies on one or two of the three pillars), based on the extraction of existing GI elements, it will provide new planning ideas for the construction of regional GIN that evaluate important ecological areas in the outskirts and areas with high demand for ES within the urban as approaches to prioritizing site selection for GI implementation.
There has been abundant research regarding the identification and extraction of GIN elements. Since 2010, some scholars have introduced morphological spatial pattern analysis (MSPA) into GIN research to determine and analyze existing GIN elements based on this method, and to conduct a dynamic evaluation or network planning for the elements (Wickham et al. Citation2010; Zhe Tao, Wan Ying, and Li Jun Citation2022; Yao and Qing Citation2013; Xu et al. Citation2015). As an image processing method, MSPA measures, identifies and classifies raster images based on morphology, and determines network elements in a landscape pattern. Based on the Euclidean distance threshold between raster cells, binary raster images can be divided into seven elements: core, islet, bridge, loop, edge, perforation, and branch (Soille and Vogt Citation2009). In previous applications of MSPA, most have stopped at identifying existing GI elements in cities, and in classifying and planning the network, but have not gone further to building new GINs. In addition, the landscape types identified by MSPA affect the functional flow, information flow and logistics of the GIN, and have important ecological implications for local green infrastructure or ecosystems (Rogan et al. Citation2016). These landscape types should also be considered as important factors in the process of constructing GI corridors, which are neglected in current related research. Therefore, this research not only used the MSPA method to identify the GI core areas, but also combined the MSPA landscape type with the construction of the ecological resistance surface in terms of corridor extraction, which can make the planning of GIN more scientific and reasonable. The ecological resistance surface is generated by the least-cost path method. The movement of organisms between different patches needs to overcome the landscape resistance. The landscape resistance reflects the difficulty of species migrating between different landscape units. The least-cost path method is a method to simulate the movement of species between patches and calculate the optimal path for species dispersal (Walker and Craighead Citation2002).
In GIN planning, in addition to the extraction of GI core area and corridor of ecological land outside the construction land, the quantity and spatial balance between the supply and demand of ecosystem services in built-up areas should also be considered. Most current ecosystem services supply and demand estimates are obtained by directly superimposing the normalized aggregate supply and aggregate demand (Burkhard et al. Citation2012; Kroll et al. Citation2012; Uthes and Matzdorf Citation2002; Rodriguez et al. Citation2016; Syrbe and Grunewald Citation2017). Because different types of evaluation indicators or methods are often mixed, the absolute range of supply and demand is likely to be ignored, resulting in inaccurate evaluation results. Therefore, there insufficient research on non-supply and demand superposition calculation of ecosystem services’ additional demand on an urban block scale. In studies of the additional requirements for ecosystem services, urban blocks are taken as the object of focus, which are calculated using a single index. The evaluation results are then used to construct the spatial priority selection of the GIN.
The purpose of this research is to try how to incorporate the idea of space prioritization and the idea of balancing the supply and demand of ecosystem services into the planning of GI. We use the natural and semi-natural blue-green spaces inside and outside the urban to build landscape-connected GIN outside the city and establish an ecosystem service demand assessment system within the urban to identify blocks with high demand for GI. The results of this research are used to provide a basis for formulating plans to enhance the function of urban ecological barriers, protect species diversity, and optimize the supply and demand configuration of GI within the urban.
Materials and methods
Study area
In this paper, the study area comprises six districts and counties on the south bank of the Yellow River in the north of Zhengzhou, the capital city of Henan Province in central China. These districts and counties include – from west to east – Gongyi City, Xingyang City, Shangjie District, Huiji District, Jinshui District and Zhongmou County (). The area is situated between 34°58’ N 112°42’ E and 34°16’ N 114° 14’ E and covers approximately 3868.47 km2. It has a temperate continental climate, with hot and rainy summers and cold and hazy winters and lies adjacent to the middle and lower reaches of the Yellow River. The area’s ecosystem is simple and fragile. Presently, the Yellow River has not only supported human civilization for millennia but also continues to sustain multiple ecosystems that benefit the human population (Jin Fengjun Citation2019). However, rapid urbanization has threatened ecological security, and the northern ecological barrier of Zhengzhou – mainly the Yellow River – has been subject to significant damage.
The existing GI in the study area lacks a comprehensive framework in terms of biodiversity, connectivity and ecosystem services. In addition to undertaking the strategic task of ecological protection and high-quality development in the Yellow River Basin at the national government level, the six districts and counties included in this area will undertake different functional orientations for the future urban development of Zhengzhou: Gongyi City, Xingyang City and Zhongmou County are developing into modern small cities that are attractive for living and working. As enclaves of Zhengzhou City, Shangjie District and Xingyang City have jointly undertaken industrial upgrading and functional transformation. Meanwhile, Huiji District and Jinshui District are vital to the economic development and revitalization of the old city (The people’s Government of Henan Province Citation2017). Considering the varied stages of development and land use characteristics for each district and county (Except Huiji District and Jinshui District, the other four cities and counties are outside the central area of Zhengzhou, and have a vast area of non-urban uses), spatial prioritization should be implemented in GIN planning based on three spatial levels: urban, suburban and Yellow River ecology.
Data preparation
Data for building the preliminary GIN were sourced from Landsat-8 OLI_TIRS remote sensing images and 30 m digital elevation model (DEM) data (Source: Geospatial Data Cloud, http://www.gscloud.cn.) in the study area on 7 July 2019, supplemented by Google map and field survey data. Interpretation results were revised to obtain the land use status map with a resolution of 30 m (). Land use types used as GI included woodland, grassland, farmland and water bodies.
The data obtained to evaluate ecosystem services included the concentration of PM2.5 in the study area, the relevant population structure, the number of days of urban waterlogging, the summer temperature, the accessibility of urban regional parks, and water quality (). These data were derived from the following sources: the daily air quality report produced by the Zhengzhou Ecological Environment Bureau in 2019 (http://sthjj.zhengzhou.gov.cn, accessed on March 2020), the Statistical Yearbook of Zhengzhou in 2019 (https://navi.cnki.net/knavi/yearbooks, accessed on January 2020), the remote sensing data of Zhengzhou in 2019 (http://www.gscloud.cn, accessed on 7 July 2019), the statistical data of Zhengzhou Meteorological Observation Station in 2019 (http://ha.cma.gov.cn/index.html, http://www.cma.gov.cn, accessed on January 2020), the vector data of Zhengzhou’s parks, green spaces and roads in 2019 (https://google-earth.gosur.com/cn/, accessed on November 2020, and provided by Zhengzhou Bureau of Gardens and Forestry), the report on the ranking of river water quality in Zhengzhou by the Zhengzhou Ecological Environment Bureau in 2019 (slt.henan.gov.cn/bmzl/szygl/szygb/, accessed on March 2020), and Supplementary data from field research.
It should be noted that the data selected is most representative of the current state of the city’s development. This is because first, the purpose of evaluating the demand for ecosystem services within the urban is to find out the supply and demand problems of urban GI in terms of ecosystem services. The GIN planning and ES evaluation this research is going to carry out are based on the current development status of the city; second, Zhengzhou is a developing city, its urban expansion and transformation occur concurrently, and there is no authoritative conclusion on whether there will be any escalation of the urban environmental problems it currently faces. Accordingly, most recent data better reflects urban problems and enables us to identify problems of supply and demand allocation in GI construction. In addition, rainfall data for 2021 increased because Zhengzhou experienced a rare rainstorm disaster, which paralyzed the whole city. This had not happened in 100 years as a dry inland city. Therefore, it is necessary to highlight and increase floodwater data in academic research for the city to adjust the weight of extra demand.
Construction of integrated GIN
GI pattern analysis
Based on the ArcGIS software platform, the Spatial Analyst module was utilized to reclassify the map according to land use type. Forest lands, grasslands, arable lands and waters with ecosystem services in the study area in were extracted as the foreground for MSPA analysis, while construction land and bare land were taken as the background. After, the GI binary raster image was acquired, and the raster data size was set as 30 m × 30 m. After analyzing the data through the Guidos analysis software, seven non-overlapping ecological landscape types were obtained. These are the study area’s GI spatial elements.
GI connectivity analysis
The selection of the GI core area was based on both patch areas and their connectivity. By considering the basic functions of GI and the distribution of green patches, the core area identified in MSPA (˃1 km2) was taken as the fundamental data for landscape connectivity analysis. Using Conefor2.6 software, the integral index of connectivity (IIC), probability of connectivity (PC), and patch importance (dPC) were selected as indicators to evaluate patch connectivity. The connection distance threshold was set at 1000 m, the connection probability was set at 0.5, and the significance of each patch to the connectivity of the landscape was analyzed. Finally, patches with areas ˃5 km2 and with dPC≥1 were extracted as significant core areas of GI. The respective definitions of IIC, PC and dPC are shown in EquationEquations 1(1)
(1) , Equation2
(2)
(2) and Equation3
(3)
(3) , respectively:
where n is the number of habitat patches existing in the landscape, and
are the areas of the habitat patches i and j, and AL is the total landscape area. Term
is the number of links in the shortest path between patches i and j. Term pij is the probability of a species moving directly from patch i to j. Term
is the metric value after the removal of the plaque.
GIN construction
To extract and prioritize GIN corridors, potential and important corridors were extracted based on the minimum cumulative resistance model and the gravity model, respectively.
Data on land use classification, elevation, slope and the seven landscape types were used as the resistance layers, and the scores and weights of each resistance layer were obtained through the expert scoring method to construct a resistance system (). The grid calculator in ArcGIS was used to superimpose the weight of landscape resistance data and obtain the comprehensive resistance surface of the study area. Then, the minimal cumulative resistance (MCR) model was used to generate the cost distance and direction using the key core area and the comprehensive resistance surface. Then, the minimum cost path from the significant core area to the target patch was calculated to generate the potential corridor of the study area.
Table 1. Resistance value and weight of each resistance factor.
Finally, the interaction matrix of the GI core interval was constructed based on the gravity model. Based on the evaluation results of the matrix, corridors with an interaction ˃30 were extracted as the important corridors, and the rest as general corridors. The extracted corridors were combined with the GI core area to construct the preliminary GIN of the study area.
Urban ES additional needs assessment
ES selection
Apart from the extraction of significant core areas and vital corridors in the study area (mostly located on the outskirts of urban areas), an ecosystem service additional demand assessment for urban districts was performed based on spatial prioritization to obtain the high additional demand areas, with urban blocks as the priority areas for GIN construction. Three aspects were considered when selecting ES service types: (1) GIN’s service supply capability and spatial flow characteristics: for some regulatory services (such as rainfall, stormwater, temperature and hydrological adjustment) and cultural services (such as recreation, spiritual services and aesthetic enjoyment) the implementation area of GI determines whether such services can be effectively used; (2) concerns for stakeholders: in recent years, problems like haze, water pollution, urban waterlogging and heat island during summer have adversely affected the city’s sustainable development; and, (3) availability of data.
After considering these aspects, four regulating services (air purification, storm water adjustment, temperature adjustment, and hydrological adjustment) and one cultural service (recreational service) were determined as the assessment types for the additional demands of ecosystem services.
Selection and calculation of quantitative indicators
To quantify disaster risks as a result of environmental disasters caused by urban expansion, the Intergovernmental Panel on Climate Change (IPCC) has proposed a disaster Risk (R) assessment framework based on “Hazard (H), Exposure (E) and Vulnerability (V)” (Field et al. Citation2012). The risk assessment framework formula is expressed as follows:
In the formula, (H) means when a hazard risk exceeds a critical threshold there is a corresponding hazard risk; (E) is the direct bearer of the disaster; and (V) reflects the ability of victims to withstand or cope with the disaster. The selected indicators were calculated based on the disaster assessment framework when evaluating the following five ecosystem services:
(1) Air purification
In 2019, the number of days that exceeded standard air quality in the study area was 188; the number of days with light, moderate, heavy and severe pollution were 109, 54, 14 and 11, respectively (mostly during winter and the transitional season of winter). There is a total of 46 PM2.5 monitoring sites in the study area (including the monitoring sites set up by Zhengzhou Ecological Environment Bureau and our supplementary monitoring sites). Researchers have set up monitoring equipment in the blocks of the site, and monitoring points are generally set up in the most polluted places such as densely populated areas and heavy traffic flow in each block. According to the monitoring data, the highest concentration reached 58 μg /m3. Smog is a significant problem affecting air quality in the region’s cities. The PM2.5 pollution risk index is taken as the primary index for evaluating the additional demand for air purification, with PM2.5 concentration, population density and social vulnerability as the secondary indices (). First, the average annual concentration limit of PM2.5 (35 μg/m3) as established by the Ambient Air Quality Standard (GB 3095–2012) was set as the threshold for additional demand. Then, the reclassification tool was used to delimit the areas with an average annual concentration of less than 35 μg/m3 as 0. The other areas were classified into five grades from 1 to 5 (low-to-high concentration values) according to the natural breakpoint method, where “1” represents the lowest degree of stress and “5” represents the highest. These five grades were used as stress evaluation indicators. Population density and the proportion of elderly people and children in blocks in urban areas were also reclassified and assigned with values from 1 to 5. They were used as the exposure evaluation index and the social vulnerability assessment index, respectively.
Table 2. ES additional requirements evaluation indicators and requirements thresholds.
After, the index was normalized with the following formula:
where is the original value,
is the normalized value,
is the minimum value, and
is the maximum value.
Finally, from Formula (5), the PM2.5 risk index of the study area was obtained, and reclassified and assigned to six levels ranging from 0 (no additional demand) to 5 (maximum additional demand).
(2) Stormwater adjustment
From actual survey data in 2019, there were 60 days of rainfall and 37 days of waterlogging in the urban parts of study area, and a maximum daily precipitation of 70 mm. It is worth mentioning that in normal years, urban waterlogging in Zhengzhou occurs frequently in the rainfall season. However, From July 17 to 23, 2021, Henan Province was hit by a rainstorm rarely seen in history. In particular, Zhengzhou city suffered heavy casualties and property losses on July 20, namely the “Zhengzhou 7.20 Rainstorm Disaster Event.” During this period, Zhengzhou accumulated rainfall reached 534 mm, process point rainfall at Baizhai weather station of the Xinmi City of Zhengzhou reached 993.1 mm, the strongest hourly rainfall at Zhengzhou National weather station reached 201.9 mm. The accumulative rainfall of more than 400 mm in Zhengzhou reached 5590 km2, and that of more than 600 mm reached 2068 km2. The accumulative rainfall in Jinshui District was close to 700 mm, and that of Gongyi and Xingyang exceeded 600 mm. On the 20th, the maximum daily rainfall of Zhengzhou National Meteorological Station was 624.1 mm, which was close to the average annual rainfall of Zhengzhou (640.8 mm) and 3.4 times the maximum rainfall since the establishment of Zhengzhou National Meteorological Station (189.4 mm, 2 July 1978). The extreme hourly rainfall intensity of 201.9 mm occurred from 16:00 to 17:00 on the 20th, breaking the historical extreme value of 198.5 mm recorded in Linzhuang, Henan province on 5 August 1975. Of the 38 drainage zones in the main urban area of Zhengzhou, only 1 reached the planned drainage standard, and the actual coping capacity of some zones was less than the standard once in five years (24-hour precipitation 127 mm). During the torrential rain, the maximum waterlogging depth reached 2.6 m (the above data are from the Investigation Report of the “7.20” Heavy Rain Disaster in Zhengzhou, Henan Province, issued by the Disaster Investigation Team of the State Council in January 2022). This extreme value will be used to calculate the weight of the additional services required by ecosystem services. Therefore, the flood risk index was used as a first-level quantitative indicator to assess the additional demand for rainwater adjustment service in the urban regions of the study area; water depth, affected infrastructure and population, and social vulnerability were simulated as the second-level indicators (). Satellite remote-sensing monitoring technology was used to obtain the inundation range, while the ArcGIS spatial analysis technology and data management function were utilized to obtain inundation depth combined with the ground elevation data of the study area to obtain the area’s flood risk index. Based on the evaluation standard of the waterlogging level in the Prevention and Control of Urban Waterlogging Planning Standard as issued by the Ministry of Housing and Urban-Rural Development 15 cm of surface gathered water was taken as the threshold for the additional demand for storm-flood regulation services. Road network density, building area density and population density were selected as the evaluation indices of the exposure index by comprehensively considering the disaster-bearing body of waterlogging disaster. Each index weight was determined based on the analytic hierarchy process.
(3) Temperature adjustment
The most prominent environmental problem in the study area in summer is the urban high temperature leading to the heat island phenomenon. In 2019, the highest daily temperature in urban areas reached 39°C, and the temperature in many places was more than 6°C higher than that in the suburbs. The urban livability climate index was selected as the first level (or primary) quantitative indicator to evaluate the additional demand for urban temperature regulation services; daily maximum temperature, population density and social vulnerability were considered the second level (or secondary) indicators (). Temperature represented the stress degree of disaster factors; meanwhile, population density represented the exposure index and the proportion of elderly and children was used for the vulnerability index. From this, the index system of urban regional liveable climate evaluation was constructed. According to the association standard of the Evaluation of Climate Suitability for Health and Wellness Destinations released by the country’s Meteorological Service Association, 35°C is the demand threshold for temperature regulation services. Meanwhile, the average daily maximum temperature recorded in summer 2019 (recorded by the Zhengzhou Meteorological Observation Station) was used as the stress degree data for disaster factors.
(4) Hydrological adjustment
The surface water quality security index was selected as the primary quantitative index to assess the additional demand for urban hydrological adjustment service. Because the water quality in the city is relatively stable, average annual water quality, population density and social vulnerability were selected as the secondary indicators (). The annual average water quality represents the degree of stress of the disaster factor, population density represents the exposure index, and the proportion of elderly people and children represents the vulnerability index. Thus, the urban water quality safety evaluation index system was constructed to obtain the demand value of hydrology adjustment. According to the report on ranking river water quality in Zhengzhou by the Zhengzhou Ecological Environment Bureau in 2019, surface water in urban areas of the study area that did not meet the “excellent” standard was graded from 1 to 5. The index calculation of the report adopts the Class 3 Standard of Environmental Quality standard for Surface Water (GB3838-2002) and the Class A standard of Urban Sewage Treatment Plant Pollutant Discharge Standard (GB18918-2002). To avoid local river water quality not being detected, we carried out field investigations where river water bodies without monitoring stations and local landscape rivers had stopped flowing in individual months and supplemented the missing data.
(5) Recreational service
Recreational opportunities were taken as the primary quantitative indicator of additional demand, while the number of people with low recreational opportunities was the secondary indicator. The point of interest (POI) method was selected to obtain the data for park green spaces within block range, and ArcGIS was used to obtain park service ability combined with the total area of the block. According to the classification requirements of urban park green spaces by service radius, the extra demand threshold of walking 15 min is considered the standard of good accessibility. By overlaying population density, the population within the low accessibility range of each block was obtained. Finally, the additional demand for recreational service was represented using a 0–5 classification.
ES demand weight calculation
From an overall fairness perspective, based on the discrete degree of each demand index, the entropy value method in the objective weighting method was used to determine each demand weight. The specific calculation steps were as follows:
Normalization of indicators:
Calculating the proportion of ecosystem service demand for item j in the ith block:
Calculating the information entropy of various requirements:
Calculating information entropy redundancy:
Calculating the weight of each demand:
According to the calculated weights, the comprehensive ecosystem service demand value of each block was obtained:
where is the value of the demand of item “j” ecosystem service in block “i;”
and
are the maximum and minimum values of the matrix column, respectively, from which the jth ecosystem service demand is located; “m” is the number of blocks; and “n” is the total number of evaluated ecosystem service demands.
Prioritization of GIN planning
For GIN planning, a spatial prioritized GIN framework was established, consisting of four dimensions: 1) seven types of ecological landscape were extracted on the basis of the MSPA while potential ecological corridors were extracted based on the least resistance model; 2) significant core areas were extracted based on patch area and connectivity analysis and important corridors were extracted based on the gravity model; 3) single-index ecosystem service extra-high demand block was extracted based on ecosystem service extra demand assessment; and 4) an integrated ecosystem service block with an extra-high demand was extracted using the entropy method. Of these dimensions, the first two focus on biodiversity and landscape connectivity GINs in the region, while the others emphasize the human settlement environment in urban areas.
Spatial priorities were also considered in the study. By integrating and super-positioning, the significant core areas, important corridors, and urban ecosystem service extra-high demand blocks into the planning phase, a spatial prioritization GIN scheme for the reconstruction of urban ecological barriers was obtained.
Results
Construction of integrated green infrastructure network
GI pattern analysis
display the seven types of ecological landscapes and data generated by MSPA in the study area. The results demonstrate that the core area is approximately 1,259.12 km2, accounting for 56.96% of the total GI area. The core area is mainly distributed on the east and west sides of the study area, with obvious polarization. On the southwest of the Songshan Mountains (spanning Gongyi and Xingyang), woodland is the primary landscape type. On the southeast in Zhongmu County, there is predominantly farmland area. Huiji District and Jinshui District are situated in the central area of Zhengzhou, with few patches and poor connectivity in the core area. Due to the influence of urban expansion and extensive distribution of bare land, patches along the southern bank of the Yellow River in the northern portion of the study area are significantly fragmented, leading to impeded species migration and diffusion among patches on the eastern and western parts. As a channel for energy and material exchange between the core patches, bridging covers a scattered area of approximately 171.78 km2, accounting for 7.76% of the total GI area. The edges and perforations are the external edges and internal edges of the GI patch, respectively, which are the regions that produce edge effect, correspondingly accounting for 17.79% and 4.48% of the woodland area. Due to serious human interference, there are many large perforations in the study area; the larger the pores, the more serious the damage to the core area. Islets act as stepping stones in the GI, accounting for only 3.23% of the GI area. These are scattered throughout the study area in fragments. The loop area is the channel connected within the core area, accounting for 3.31% of the total GI area. The branch line connects the core area and the non-core area; it is an interrupted corridor, accounting for 6.47% of the total GI area. There are several branches, which reflect the poor connectivity of the ecological landscape.
GI connectivity analysis
present the results of the connectivity analysis of the important core areas. Indicatively, there are 17 important core areas, and most ecological sources with greater connectivity are distributed along the east and west of the study area. This reflects a better landscape connectivity, which is conducive to species migration and biodiversity conservation. The poor connectivity of patches in the central region and the lack of core patches along the Yellow River greatly diminish the function of the river and its banks as an urban ecological barrier.
GIN construction
Based on the interaction matrix among the patches generated by the gravity model, the significance of the corridors among such patches can be evaluated. The interaction between ecological source areas 3 and 6 is the strongest, the landscape resistance between the surface patches is the weakest, and the communication and dissemination of the species is the simplest. Therefore, strengthening the construction and protection of the corridors between the two core areas is conducive to the conservation of GI connectivity and biodiversity within the study area. The east and west core areas are far apart with a weak interaction force. For instance, core areas 2 and 7 are generally less correlated and require greater resistance to be overcome if species move and migrate; hence, such movements and migrations are less likely to occur. illustrate the corridor network. Based on the minimum resistance model and gravity model, 136 potential corridors and 24 important corridors are generated. Inadequate GI in the middle of the study area leads to poor connectivity between the east and the west, which is not conducive for species migration and ecological flow diffusion.
Urban ES additional needs assessment
Urban ES individual needs assessment
) present the evaluation results for the additional requirement of each ecosystem service. In the studied urban blocks, the number of blocks corresponding to the extra demand value of air purification from 5 to 0 are 3, 3, 4, 7, 26 and 0, respectively. The part with high additional demand is Xinhua Road block in Gongyi City, Dashiqiao block in Jinshui District, and Duling block in Jinshui District, while the portions with an even higher additional demand are Nanyangxincun block in Jinshui District, Nanyang block in Jinshui District and Renmin Road block in Huiji District.
The numbers of blocks that correspond to the additional demand value of stormwater adjustment from 5 to 0 are 5, 14, 14, 7, 6 and 0, respectively. In terms of spatial distribution, areas with high rainfall and flood adjustment demand are primarily situated in the center or the south of the city. Among these, parts with high additional demand are Duling block in Jinshui District, Liuzhai block in Huiji District, Changxing Road block in Huiji District, Fenghuangtai block in Jinshui District, and Fengchanlu block in Jinshui District.
The number of blocks corresponding to the additional demand value of temperature adjustment from 5 to 0 are 3, 8, 7, 14, 11 and 3, respectively. High-demand areas are typically concentrated in densely populated areas within the urban center, with demand values decreasing from the urban center to the periphery. These areas are Nanyang Road block in Jinshui District, Huayuan Road block in Jinshui District, and Dashiqiao block in Jinshui District.
The numbers of blocks corresponding to the additional demand value of hydrological adjustment from 5 to 0 are 2, 1, 2, 4, 29 and 8, respectively. The generally high-demand areas in hydrological adjustment are Duling block in Jinshui block, Xinhua Road block in Gongyi City, and Laoyachen block in Jinshui block. They are typically distributed in relatively small blocks, close to the city center.
The additional demand value for recreational service ranges between 5 and 0, and the corresponding block numbers are 2, 1, 4, 3, 33, and 3, respectively. In terms of spatial distribution, areas with high or higher additional demand are primarily distributed near the edge of the study area, including Duling block in Jinshui block, Laoyachen block in Huiji block, and Nanyang block in Jinshui block.
Urban ES comprehensive needs assessment
Using MATLAB software and the entropy method, the weight of each additional demand service was computed. The results demonstrate that the weight of air purification demand is the highest (0.531), stormwater adjustment demand is 0.166; recreation service demand is 0.093, hydrological regulation demand is 0.139, and the weight value of temperature regulation demand is the smallest, at 0.071. Using ArcGIS software, the weight values as obtained by comprehensive superposition were calculated and the additional demand value of integrated ecosystem service was acquired after recalculating and classifying them (). The results show that the respective numbers of blocks corresponding to comprehensive ecosystem service demand value from 5 to 0 are 2, 3, 4, 11, 26 and 0, respectively, among which there are three blocks with high demand, all of which are in old urban areas (). From a spatial structure perspective, the areas with high demand are usually distributed in the central part with small areas, while those with low demand are typically distributed in portions near the edge of the city.
Table 3. Blocks of integrated ES high excess demand.
Prioritization of GIN
illustrates the GIN, which was derived by integrating the significant ecological core areas and corridors, as well as the additional ecosystem service requirements for urban blocks considering spatial priorities. To enhance the function of GIN in Zhengzhou during various disasters and to reconstruct the ecological barrier within the north of the city requires more than merely connecting the green space. To benefit from the resilience of GIN, multidimensional research approaches must be simultaneously adopted. In this context, this research discusses how to apply the three pillars of GIN as the starting point and to utilize the concept of space prioritization to establish a highly implementable GIN.
Discussion
Core design for GIN planning
There are two types of core in the GIN area of study: one includes habitat areas for wild animals and plants located in the surrounding mountains, and the other includes farmland, artificial forests, and country parks with high human participation. Because cores provide various functions, it is necessary to design them with different design approaches. In the case of wildlife habitat cores (such as 7, 8, and 14–16), it is significant to provide habitats for native flora and fauna, as well as origins and destinations for them through GIN. In this context, the ecological landscape of the significant core areas should be large and continuous. The source plaques (selected by combining MSPA with connectivity) have quantitative and objective attributes in this study; the significant source patches are extracted by the combination of connectivity and area size, which satisfies the characteristics of good connectivity and large patch areas.
Thus, cores not only provide sites for biodiversity, farmlands can provide food throughout the region (such as 3, 4, 6, 12, and parts of cores 9,11, 17). Artificial forests are used to preserve soil and water, resist sand and wind, and provide certain economic materials, such as part of cores 9, 11 and 17. Apart from providing places for recreation, country parks are also used for the conservation, cultivation and migration of animal and plant resources, such as cores 1, 2, and 13. Therefore, in the study we adopted two strategies for these cores of high human activity: cores design on the basis of supply service, and those for ecological maintenance. The supply service cores include farmlands and a small number of artificial forests. The design strategy is to reduce soil pollution by optimizing agricultural structure and development patterns while maintaining the size and integrity of the existing cores. The ecological conservation cores include country parks and most of the artificial forests. The design strategy is to enrich species resources, regulate the regional climate, and provide a site for animal migration through the protection and restoration of vegetation and bodies of water.
Overall, it is highly necessary to transform a large area of bare land outside the city, especially along the Yellow River, into ecological land to increase the area and number of core areas for maintaining biodiversity.
Corridor design for GIN planning
The ecological corridors in this paper refers to the most suitable path for biological migration (Adriaensen et al. Citation2003). The corridors of GIN in the study area are classified into two types: potential corridors and important corridors. By adopting the minimum cumulative resistance model, the optimal elements of the potential corridors can be quantitatively analyzed and extracted; meanwhile, using the gravity model, the relationship strength among the core areas can be quantitatively evaluated and the significance of the corridors can be assessed. The important corridors that are generated by the gravity model represent the larger interaction forces between the patches. They are ecological corridors that can and must be easily maintained. Their role is highlighted in terms of landscape connectivity, which facilitates species migration and the diffusion of ecological flows.
Based on spatial priority, the important corridors design for GIN planning can be categorized into linear corridor planning and stepping-stone corridor planning methods. In this study, a linear corridor is defined as a linear pathway for either humans or wildlife, such as waterfront green spaces, trails, local rivers, and urban roads with planted trees (Jeong, Kim, and Song Citation2020). A stepping-stone corridor is defined as islets based on MSPA results that can be used as steppingstones to form an ecological pathway. According to the function and size, we designed and applied regional greenway and wild greenway concepts for corridor planning.
Regional greenways are landscapes that integrate various civic functions into existing highways, roads with different speeds of movement and railways landscapes, and roads with a vegetated median that separates arterial throughways and provides water treatment services. These comprise corridor midpieces of 2, 4, 5, 7, 9, 12, 15–17, 19 and 23 in this study. The socioecological services that regional greenways need to offer include disaster control, evacuation routes, conservation buffers, and habitat protection. Therefore, the planning of these areas needs to be concerned with the potential for dust, flooding and providing safe zones for the protection and migration of native plants and animals, such as corridors 2, 4, 5, 7, 9, 12, 16, 17, 19 and 23. Scientific planting of tree species can prevent wind, fix sand and reduce dust, and infiltration facilities along the highway and can reduce the harm of floods and waterlogging. Corridors 15, which are close to wildlife preserves, need to use the islets in their adjacent areas as steppingstones to increase ecological land and enhance connectivity with the core area, thus creating new corridors to ensure the survival and migration of species.
Wild greenways are located mainly in complete ecological source areas, including linear landscapes with strong connectivity in mountains, country parks, along rivers, farmlands, and artificial forests, such as corridors 3, 6, 8, 10, 11, 13, 21, 22, 24, the south sections of 12, 14, 15, 18, 19, 20, the western section of 1, and the middle of 2. The ecological services offered by wild greenways include species preservation, migration, and regional ecological maintenance. Therefore, the planning focus of these greenways is to carry out targeted protection and restoration based on the investigation of current species resources and ecological maintenance. For instance, corridors 21, 22, and the south section of 12, 14, 15, 18, 19, and 20 located in Songshan mountain and its peripheral regions have good ecological status, although parts located at the edge of these ecological sources tend to be eroded and fragmented. Therefore, based on ecological restoration, we propose to use the surrounding islets to add steppingstone corridors. Environmental problems in greenways near rivers (such as the western section of corridor 1, the middle of 2, and the north section of 14 and 15) include abandoned land and waste accumulation, disruption of migration pathways due to hard revetments, and sewage discharge. The corresponding solutions we put forward include cleaning up waste materials and replenishing vegetation, building soft revetment, increasing water purification facilities, and cultivating plants, and cultivating plants and microorganisms beneficial to water purification. Greenways that pass across farmland and artificial forest areas (such as corridors 3, 6, 8, 10, 11, 13, and 24) have unstable ecological functions due to the influence of farming activity. Meanwhile, soil pollution caused by extensive agriculture is gradually increasing. Therefore, we recommend the development of multi-layer and ecological agriculture as well as soil remediation.
However, current inadequate corridors connecting the east and the west along the middle of the study area does not mean that corridor construction in this portion is insignificant. The fragmentation and lack of patches makes such construction difficult. Therefore, apart from the use of bare land to increase the core area and other large patches of green spaces, islets, bridges, branches and other points, linear GI should be established in Huiji District and Jinshui District to increase connectivity, in order to enhance the role of the ecological barrier along the Yellow River in the north of the study area. This would eventually form a complete GIN.
ES needs assessment for GIN planning
This research is devoted to planning a GIN that serves the city’s needs. Therefore, both indicator selection and research scope reflect such needs. By undertaking a needs assessment of the city ecosystem services, the different needs of the different blocks for the GI ecosystem service functions have been determined. Based on environmental quality standards, quantitative assessment and the mapping of spatial mismatch between supply and demand of urban ecosystem services, targeted auxiliary information can be provided for decision-making on urban GIN planning. This method can pinpoint areas with high additional ecosystem services demand and make them priority locations for urban GI implementation. This will help to maximize the comprehensive benefits of ecosystem services provided by GI. According to environmental problems faced by different blocks, we designed and applied soft and hard landscape concepts for GI planning.
Soft landscapes include the use of urban open space to add small green areas, the construction of green roofs and rain gardens, and other points of GI. Hard landscapes include the addition of permeable pavements and small activity areas. For air purification, dust pollution in the streets can be improved by greening the exposed scattered spaces, such as the roadside in narrow streets and the corner in front of houses, as well as building green roofs for houses with conditions, such as Xinhua block. For stormwater adjustment, green roofs, rain gardens, and permeable paving can increase infiltration and effectively address vulnerability to waterlogging within blocks, such as Duling block, Liuzhai block, Changxing block, Fenghuangtai block and Fengchanlu Block. For temperature adjustment, planting in bare plots and green roof construction can alleviate the heat-island, and contribute to indoor energy conservation, such as Nanyang block, Huayuan Block and Dashiqiao block. For hydrological adjustment, according to the ecological connectivity of rivers damaged by sewage discharge and hard revetment, it is necessary to carry out ecological restoration of river revetment by adding soft revetment and purifying the water using plants and microorganisms (as in the case of Yiluo River in Xinhua block and Jinshui River in Duling block). For recreational services, the construction of urban street parks and corner parks can change the present situation of bare and idle blocks and large areas of pavement, while at the same time as the added point GIs they can provide steppingstones for ecology, such as for Duling block and Xinhua block.
In this study, use of environmental quality standards as additional demand thresholds is applicable for adjustment and recreation services, while subjective service demands other than the ecological environment should not be considered. In addition, single-scale GIN programming cannot be used to its full effect in practice. Thus, from a spatial planning perspective, a multiscale and multidimensional ecosystem service evaluation has been conducted, providing data to support GIN site selection prioritization.
Conclusion
This study combines the regional ecological network and the urban ecosystem services needs to develop a GIN to reconstruct the ecological barrier in the north of Zhengzhou and reduce disaster risks in the city. The suggested method can help resolve environmental problems in urban development by studying the necessary functions of cities for coping with natural disasters at multiple scales. Previous studies on GINs have focused on only one aspect at a single scale. Comparably, the GIN model in this study utilizes the significant core areas and corridors outside the city as key resources to construct ecological barriers. It then evaluates the ecosystem service demands on a block scale within the city. The GIN modeling method presented in this study can be applied to other developing cities with rapid population and urban expansion rates.
A feasible planning idea has been put forward for decisionmakers and practitioners to use for the design of better cities by integrating a peripheral ecosystem and urban ecosystem service needs. For instance, MSPA and the gravity model can provide grading and classification guidance for GIN planning by analyzing dates, problems of different ecological land areas outside the city are urgent to solve, especially a large area of bare lands on the south bank of the Yellow River has been left unused, which hinders the spread and diffusion of ecological flow. On this basis, the project solutions were given in detail. The selection of the ecosystem service demand evaluation index and the construction of the system should be based on the environmental problems of the studied cities. Through evaluation, we found that the old areas within the city lacked dotted GI to form completed GIN, while the most prominent environmental problems were air pollution and urban waterlogging. We proposed different strategies to solve the problems faced by blocks with high ecosystem service demand. According to the GIN plan in this study, significant core patches and corridors will be protected and built, landscape connectivity along the east and west sides of the Yellow River will be enhanced, different blocks within the city will establish GI based on the evaluation results of ecosystem service additional demands, and a multiscale and multidimensional GIN with spatial priority and strong real-time performance will be generated.
Combined with the research results and the existing policies, we put forward the following suggestions to implement the GIN planning: Based on the delineation of basic farmland and the ecological red line to protect existing GI elements, moreover, delineate more GI elements within the scope of ecological construction and ecological governance policies; corresponding GI land in provincial and national ecological reserves Conservation and planning, such as Zhengzhou Yellow River National Wetland Park located on the south bank of the Yellow River, as an important ecological source and ecological corridor, is beneficial to increase ecological connectivity and species diversity within the study area and improve the entire regional GIN; combined with local policy of the Urban regeneration in the old city for GI layout and design of urban areas with supply and demand balance in mind. Nevertheless, the study has its limitations. Due to insufficient relevant data concerning biological species, the conservation of species diversity is considered only in terms of patch patterns and connectivity intensity. In addition, the study may be difficult to implement, since it involves collaboration between different government departments, and resolution of conflict concerning the division of permanent prime farmland and ecological conservation land. Thus, further research is required to establish a more concrete GIN that considers changes in biological species and land use types.
Acknowledgments
We would like to thank the support of the International Joint Laboratory of Landscape Architecture, Henan Agricultural University, for their infinite help. We also thank Data Center for Zhengzhou Ecological Environment Bureau and Zhengzhou Meteorological Observation Station.
Supplemental Material
Download MS Word (2 MB)Disclosure statement
No potential conflict of interest was reported by the author(s).
Supplementary material
Supplemental data for this article can be accessed online at https://doi.org/10.1080/20964129.2022.2088403
Additional information
Funding
References
- Adriaensen, F., J. P. Chardon, G. D. Blust, E. Swinnen, S. Villalba, H. Gulinck, and E. Matthysen. 2003. “The Application of ‘least-cost’ Modeling as a Functional Landscape Model.” Landscape and Urban Planning 64 (4): 1–16. doi:10.1016/S0169-2046(02)00242-6.
- Artmann, M., and K. Sartison. 2018. “The Role of Urban Agriculture as a nature-based Solution: A Review for Developing a Systemic Assessment Framework.” Sustainability 10 (6): 1937. doi:10.3390/su10061937.
- Bennett, E. M., G. D. Peterson, and L. J. Gordon. 2009. “Understanding Relationships among Multiple Ecosystem Services.” Ecology Letters 12 (12): 1394–1404. doi:10.1111/j.1461-0248.2009.01387.x.
- Biggs, R., M. Schlüter, D. Biggs, P. C. West, S. BurnSilver, G. Cundill, and V. Dakos. 2012. “Toward Principles for Enhancing the Resilience of Ecosystem Services.” Annual Review of Environment and Resources 37 (1): 421–448. doi:10.1146/annurev-environ-051211-123836.
- Bloomberg, M. R., and C. Holloway. “NYC Green Infrastructure Plan: A Sustainable Strategy for Clean Waterways.” EB/OL. http://www.nyc.gov/html/dep/pdf/green_infrastructure/NYCGreenInfrastructurePlan_LowRes.pdf
- Burkhard, B., F. Kroll, S. Nedkov, and F. Müller. 2012. “Mapping Ecosystem Service Supply, Demand and Budgets.” Ecological Indicators 21: 17–29. doi:10.1016/j.ecolind.2011.06.019.
- Carlier, J., and J. Moran. 2019. “Landscape Typology and Ecological Connectivity Assessment to Inform Greenway Design.” The Science of the Total Environment 651 (Pt 2): 3241–3252. doi:10.1016/j.scitotenv.2018.10.077.
- Cohen- Shacham, E., G. Walters, S. Maginnis, and C. Janzen. 2016. Nature-based Solutions to Address Global Societal Challenges. Gland: IUCN.
- Dawson, T. P., S. T. Jackson, J. I. House, I. C. Prentice, and G. M. Mace. 2011. “Beyond Predictions: Biodiversity Conservation in a Changing Climate.” Science 332 (6025): 53–58. doi:10.1126/science.1200303.
- Demuzere, M., K. Orru, O. Heidrich, D. Olazabal, H. Geneletti, H. Orru, A. G. Bhave, N. Mittal, E. Feliu, and M. Faehnle. 2014. “Mitigating and Adapting to Climate Change: Multi-functional and multi-scale Assessment of Green Urban Infrastructure.” Journal of Environmental Management 146: 107–115. doi:10.1016/j.jenvman.2014.07.025.
- European Environment Agency. 2014. “Spatial Analysis of Green Infrastructure in Europe.” Technical report No 2/2014. EEA
- Fengjun, J. 2019. “Coordinated Promotion Strategy of Ecological Protection and High-quality Development in the Yellow River Basin.” Reform 309 (11): 33–39.
- Field, C. B., V. Barros, T. F. Stocker, and Q. Dahe. 2012. “Managing the Risks of Extreme Events and Disasters to Advance Climate Change Adaptation (Special Report of the Intergovernmental Panel on Climate Change).” In Determinants of Risk: Exposure and Vulnerability, 65–108. doi:10.1017/CBO9781139177245(Chapter2).
- Firehock, K. 2015. Strategic Green Infrastructure planning—a multi-scale Approach”. Washington, DC: Island Press.
- Grim, N. B., S. H. Faeth, N. E. Golubiewski, C. L. Redman, J. Wu, X. Bai, and J. M. Briggs. 2008. “Global Change and the Ecology of Cities.” SCIENCE 319 (5864): 756–760. doi:10.1126/science.1150195.
- Haines, Y. R., and M. Potschin. 2010. “The Links between Biodiversity, Ecosystem Services and Human well-being.” In Ecosystem Ecology: A New Synthesis, edited by D. G. Raffaelli, C.L.J. Frid . Cambridge: Cambridge University Press 110–139 .
- Honeck, E., A. Sanguet, M. A. Schlaepfer, N. Wyler, and A. Lehmann. 2020. “Methods for Identifying Green Infrastructure.” SN Applied Sciences 2 (11). doi:10.1007/s42452-020-03575-4.
- Jeong, D., M. Kim, and K. Song. 2020. “Junga Lee.” Planning a Green Infrastructure Network to Integrate Potential Evacuation Routes and the Urban Green Space in a Coastal City: The Case Study of Haeundae.” Science of the Total Environment 761.5737. doi:10.1016/j.scitotenv.2020.143179.
- Kong, F., H. Yin, N. Nakagoshi, and P. James. 2012. “Simulating Urban Growth Processes Incorporating a Potential Model with Spatial Metrics.” Ecological Indicators 20: 82–91. doi:10.1016/j.ecolind.2012.02.003.
- Kroll, F., F. Mueller, D. Haase, and N. Fohrer. 2012. “Rural-urban Gradient Analysis of Ecosystem Services Supply and Demand Dynamics.” Land Use Policy 29 (3): 521–535. doi:10.1016/j.landusepol.2011.07.008.
- Lennon, M. 2015. “Green Infrastructure and Planning Policy: A Critical Assessment.” Local Environment 20 (8): 957–980. doi:10.1080/13549839.2014.880411.
- Morley, P. M. 1988. “Urban Forestry in North York. For.” The Forestry Chronicle 64 (4): 360–364. doi:10.5558/tfc64360-4.
- Panagopoulos, T., J. A. G. Duque, and M. B. Dan. 2016. “Urban Planning with respect to Environmental Quality and Human well-being.” Environmental Pollution 208: 137–144. doi:10.1016/j.envpol.2015.07.038.
- Patz, J. A., and D. E. Norris. 2004. “Land Use Change and Human Health.” Ecosystems and Land Use Change 153: 159–167.
- The people’s Government of Henan Province. 2017. “Spatial Planning of Zhengzhou Metropolitan Area (2018-2035).” https://www.henan.gov.cn/
- Pugh, T. A. M., A. R. Mackenzie, J. D. Whyatt, and C. N. Hewitt. 2012. “Effectiveness of Green Infrastructure for Improvement of Air Quality in Urban Street Canyons.” Environmental Science& Technology 46 (14). doi:10.1021/es300826w.
- Rodriguez, R. D., J. H. Kain, D. Haase, A. B. Kaczorowska, and A. Kaczorowska. 2015. “Urban self-sufficiency through Optimised Ecosystem Service Demand. A Utopian Perspective from European Cities.” Futures 70: 13–23. doi:10.1016/j.futures.2015.03.007.
- Rogan, J., T.M. Wright, J. Cardille, and H. Pearsall, Ogneva-Himmelberger, Y., Riemann, R., Riitters, K., Partington, K. 2016. “Forest Fragmentation in Massachusetts, USA: A town-level Assessment Using Morphological Spatial Pattern Analysis and Affinity Propagation GIScience & Remote Sensing.” 53(4). doi:10.1080/15481603.2016.1141448.
- Roppongi, H. 2017. In Urbanization and Climate Co-Benefits: Implementation of Win-Win Interventions in Cities. CRC Press: Boca Raton, FL, USA. 125–129.
- Soille, P., and P. Vogt. 2009. “Morphological Segmentation of Binary Patterns.” Pattern Recognition Letters 30 (4): 456–459. doi:10.1016/j.patrec.2008.10.015.
- Syrbe, R., and K. Grunewald. 2017. ““Ecosystem Service Supply and Demand - the Challenge to Balance Spatial Mismatches.” International Journal of Biodiversity Science Ecosystem Services & Management 13. doi:10.1080/21513732.2017.1407362.
- Tzoulas, K., K. Korpela, S. Venn, V. Yli-Pelkonen, A. Kaźmierczak, J. Niemela, and P. James. 2007. “Promoting Ecosystem and Human Health in Urban Areas Using Green Infrastructure: A Literature Review.” Landscape and Urban Planning 81 (3): 167–178. doi:10.1016/j.landurbplan.2007.02.001.
- UN-DESA (United Nations, Department of Economic and Social Affairs, Population Division) (2019). “World Urbanization Prospects: The 2018 Revision” (ST/ESA/SER.A/420). New York: United Nations.
- Uthes, S., and B. Matzdorf. 2016. “Budgeting for government-financed PES: Does Ecosystem Service Demand Equal Ecosystem Service Supply?” Ecosystem Services 17: 255–264. doi:10.1016/j.ecoser.2016.01.001.
- Walker, R., and L. Craighead. “Analyzing Wildlife Movement Corridors in Montana Using GIS” Environmental Sciences Research Institute.” Proceedings of the 1997 International ArcInfo Users’ Conference, San Diego CA, (2002)
- Wang, J., and E. Banzhaf. 2018. “Towards a Better Understanding of Green Infrastructure: A Critical Review.” Ecological Indicators 85: 758–772. doi:10.1016/j.ecolind.2017.09.018.
- Wickham, J. D., K. H. Riitters, T. G. Wade, and P. Vogt. 2010. “A National Assessment of Green Infrastructure and Change for the Conterminous United States Using Morphological Image Processing.” Landscape and Urban Planning 94 (3–4): 186–195. doi:10.1016/j.landurbplan.2009.10.003.
- Xu, F., H. W. Yin, F. H. Kong, and J. G. Xu. 2015. “Developing Ecological Networks Based on Mspa and the least-cost Path Method: A Case Study in Bazhong Western New District.” Journal of Ecology. doi:10.5846/stxb201402130248.
- Yao, Q., and C. Qing. 2013. “Wang Jing.” A MSPA-based Planning of Urban Green Infrastructure Network———A Case of Shenzhen.” China Garden 29 (5): 104–108.
- Zhe Tao, X., Z. Wan Ying, and H. A. O. Li Jun. 2022. “Retraction Note: The Evolution of Spatial and Temporal Patterns of Zhengzhou Ecological Network Based on MSPA.” Arabian Journal of Geosciences 15 (8). doi:10.1007/s12517-022-10068-8.