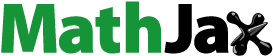
ABSTRACT
This study aims to examine the development trend of COVID-19 in China and propose a model to assess the impacts of various prevention and control measures in combating the COVID-19 pandemic. Using COVID-19 cases reported by the National Health Commission of China from January 2, 2020, to January 2, 2022, we established a Susceptible-Exposed-Infected-Asymptomatic-Quarantined-Vaccinated-Hospitalized-Removed (SEIAQVHR) model to calculate the COVID-19 transmission rate and Rt effective reproduction number, and assess prevention and control measures. Additionally, we built a stochastic model to explore the development of the COVID-19 epidemic. We modeled the incidence trends in five outbreaks between 2020 and 2022. Some important features of the COVID-19 epidemic are mirrored in the estimates based on our SEIAQVHR model. Our model indicates that an infected index case entering the community has a 50%–60% chance to cause a COVID-19 outbreak. Wearing masks and getting vaccinated were the most effective measures among all the prevention and control measures. Specifically targeting asymptomatic individuals had no significant impact on the spread of COVID-19. By adjusting prevention and control parameters, we suggest that increasing the rates of effective vaccination and mask-wearing can significantly reduce COVID-19 cases in China. Our stochastic model analysis provides a useful tool for understanding the COVID-19 epidemic in China.
Background
The first case of COVID-19 was reported on December 1, 2019 in Wuhan city of Hubei province, China, after which the virus quickly spread to other parts. The novel coronavirus spread rapidly worldwide, leading to a pandemic. China implemented strict measures, including wearing masks and quarantining patients, resulting in successful control of the COVID-19 outbreak within its borders. However, since March 2020, China has experienced multiple COVID-19 outbreaks on several occasions.
Since the emergence of COVID-19, researchers from various fields have studied and analyzed its data. Infectious disease models, such as SIR, SEIR, and improved SEIR models, have been widely established, with the SEIR model being the most commonly used.Citation1–5 These models have been used to estimate the basic reproduction number of the virus and predict the epidemic trend in different regions.Citation6–9 However, deterministic models can no longer fully capture the complex dynamics of diseases like COVID-19 due to the constantly changing and unpredictable environment. Therefore, researchers have increasingly turned to stochastic infectious disease models, which take into account random interference in the transmission of the disease.Citation10–14 These studies have shown that COVID-19 has a high probability of spreading to most people, making it crucial to implement rapid and efficient contact detection, management, and timely case isolation to control outbreaks.
In the following section, we constructed a deterministic model and a stochastic model, which were fitted with actual data to analyze the development trend of COVID-19 in China. We evaluated the effects of various prevention and control measures and compared the results of the stochastic model with the deterministic model.
Methods
This study focuses on COVID-19 cases reported by the National Health Commission of the People’s Republic of China between March 4, 2020, and January 21, 2022. We will analyze the severe COVID-19 period between 2020 and 2022, calculate the effective reproduction number of different time periods, compare the COVID-19 outbreaks and their development trend in China, and evaluate the effectiveness of prevention and control measures. Furthermore, we will compare the differences between the stochastic and deterministic models.
The deterministic mode
A typical approach when modeling the spread of an infectious disease in a community is to formulate a compartmental model. We divide the total human population N, into nine compartments: susceptible individuals S, exposed individuals E, symptomatic individuals I, asymptomatic individuals A, quarantined susceptible Qs, quarantined exposed Qe, vaccinated individuals V, hospitalized individuals H and recovered individuals R. The dynamics explained in this subsection are displayed in , also see for the meaning of the remaining parameters.
Figure 1. Flow diagram of the models. Each box represents an individual compartment and the arrows represent transitions between the compartments. Nine individual compartments are susceptible individuals S, exposed individuals E, symptomatic individuals I, asymptomatic individuals A, quarantined susceptible Qs, quarantined exposed Qe, vaccinated individuals V, hospitalized individuals H and recovered individuals R, respectively. The transition rates are shown next to the arrow.

Table 1. Description of variables in the model (1.1).
Table 2. The meaning and value of each parameter in the model.
In order to build this model (1.1), we made the following assumptions:
Susceptible persons can be infected by exposed individuals, symptomatic individuals and asymptomatic individuals, and become exposed after the incubation period. The transmission rate of exposed individuals is
, symptomatic individuals is
and asymptomatic individuals is
.
The individuals may become symptomatic or asymptomatic after being infected by SARS-CoV-2, and the probability of becoming symptomatic is p.
The probability that a susceptible individual will be quarantined is
, and that an exposed individual will be quarantined is
.
,
,
are the recovery rates of symptomatic, asymptomatic, and hospitalized individuals, respectively.
Effective mask wearing rate in the population is
.
Susceptible people have
probability of vaccination and become immune in a short time after vaccination.
The recovered individuals do not acquire the infection again and are not going to infect others.
Based on these assumptions, we proposed a schematic diagram () and provided the corresponding model Equations (1.1):
Effective reproduction number
According to next-generation matrix method, we determine the expression for the effective reproduction rate Rt. In order to this derivation, we add birth rate() and death rate(c) into the model (1.1), get model (1.2):
By adding multiple equations in the model (1.2), we get:
So, there is the following set:
is the positively invariant set of the model (1.2).
According to next-generation matrix method, let .
So, there is the following set:
The new infectious terms and transition terms of the model (1.2) are given by
and
are the Jacobi matrices of
and
. It follows that
Where
Because the effective reproduction number is the same as the FV−1 spectrum radius:
In this study:
The stochastic model
We have extended our deterministic model (1.1) to a corresponding stochastic model, recognizing that the spread of infectious diseases is subject to stochastic perturbations. The study of the stochastic model is motivated by the observation that dynamics can be significantly impacted by small variations in the parameters. In many instances, infections are confined to small localities or geographic regions, making the setup of a stochastic model appropriate. As a result, important and relevant information can be derived from the stochastic model.
Assuming that noise directly disturbs each population segments in proportion, the stochastic model (1.3) is described as follows:
is an independent standard Wiener process with
. And for any
is a continuous bounded function, where
is the strength of the Wiener process.
Estimation
We use Matlab software to estimate the parameters of the model. The fourth-order Runge-Kutta-Fehlberg Method, with tolerance set at 0.001, was used to solve the differential equation, and curve fitting. The parameter estimates were obtained by fitting the data with Matlab software. The routines applied for estimation is as flows: First, the time period data were selected for analysis. Then we used Matlab software to write the program of the ordinary differential equation, and finally the parameters are estimated with the actual data to get the corresponding results. The corresponding stochastic model adds random terms in the deterministic model. For estimation of stochastic models, we write the program of the corresponding stochastic differential equation, and finally estimates the parameters with the actual data to get the corresponding results.
We selected five periods for analyzes and estimation. The periods selected are the severe COVID-19 periods between 2020 and 2022, which are January 2, 2021 to February 14, 2021, July 4, 2021 to September 8, 2021, September 8, 2021 to October 14, 2021, October 14, 2021 to November 24, 2021 and November 24, 2021 to January 21, 2022.
Sensitivity analysis
The forward sensitivity index of normalization of function f with variable x is . According to the formula Rt, Rt is affected by four parameters
,
,
and
, among which parameters
,
and
are uncontrollable variables with fixed parameter values in the process of disease transmission, so we only need to analyze the sensitivity changes of Rt with
.
We find
Result
Since March 4, 2020, there has been a significant decrease in the number of active COVID-19 cases in China, with no significant new outbreaks reported until December 2020. Subsequently, there has been a gradual emergence of COVID-19 outbreaks in China. Serious outbreaks of COVID-19 were observed in January, July, September, November, and December of 2021 (refer to ).
Deterministic model
The output of the fitted model and the number of active COVID-19 cases in five different major epidemics are shown in . shows the parameter estimates and effective reproduction number of the five time periods.
Figure 3. Plots of the output of the fitted model and the observed active COVID-19 case. (a) January 2, 2021 to February 14, 2021; (b) July 4, 2021 to September 8, 2021; (c) September 8, 2021 to October 14, 2021; (d) October 14, 2021 to November 24, 2021; e: November 24, 2021 to January 21, 2022.

Table 3. Mean values of estimated parameters ,
,
,
,
,
and
. These parameters are explained in :
,
, and
refer to transmission rate of susceptible with exposed, symptomatic and asymptomatic human, respectively.
refers to rate of effective vaccination.
and
refer to quarantine rate of susceptible and exposed individuals, respectively.
refers to rate of hospitalized of asymptomatic individual.
Table 4. Mean values of estimated effective reproduction number.
The highest effective reproduction number of the epidemic was observed from January 2, 2021 to February 14, 2021, followed by the period from November 24, 2021 to January 21, 2022, while the lowest was noted from September 8, 2021 to October 14, 2021. This observation may be related to the implementation of prevention and control measures, population mobility, and the scope of the epidemic. Patients in the incubation period are more infectious than asymptomatic patients and symptomatic patients. Furthermore, a higher vaccination rate is observed during severe outbreaks. illustrates the fitting of the model with real COVID-19 data for all datasets. It is important to emphasize that the results of the model are effective only for a short time and depend entirely on the current epidemic situation. Predictions for the long term are neither feasible nor appropriate, given the dynamic and evolving nature of disease and interventions.
Sensitivity analysis
According to the sensitivity index analysis, Rt was most sensitive to parameters ,
, and positively correlated with
,
,
, negatively correlated with
. In theory, in order to control the spread of COVID-19,
can be reduced, and the effect of reducing
may be better. At the same time, increasing the effective vaccination rate of susceptible individuals and improving the quarantine rate of susceptible individuals can better control the spread of COVID-19. The effect of quarantine rates of exposed individuals and hospitalization rates of symptomatic and asymptomatic individuals on the transmission of COVID-19 is not significant ().
Figure 4. Rt sensitivity analysis. (a) January 2, 2021 to February 14, 2021; (b) July 4, 2021 to September 8, 2021; (c) September 8, 2021 to October 14, 2021; (d) October 14, 2021 to November 24, 2021; (e) November 24, 2021 to January 21, 2022. Sensitivity index of Rt with respect to parameters ,
,
,
,
and
respectively. These parameters are explained in :
,
, and
refer to transmission rate of susceptible with exposed, symptomatic and asymptomatic human, respectively.
refers to rate of effective vaccination.
and
refer to quarantine rate of susceptible and exposed individuals, respectively.
refers to rate of hospitalized of symptomatic individuals.
refers to rate of hospitalized of asymptomatic individual.

Stochastic model
We applied the stochastic differential equation (SDE) to simulate each of the five outbreaks 100 times. We set the Wiener process intensity for each equation to 0.00025,
0.025,
0.035. Initially, we compared the average of the 100 random model fits to the actual data and the results of the deterministic model. The figure demonstrates that the mean of the stochastic model is similar to the fitting results of the deterministic model, except for the period from July 4, 2021, to September 8, 2021 (). To further investigate the effect of randomness on the infected individuals, we graphed 100 simulation runs for both symptomatic (I) and asymptomatic (A) infected individuals. We observed that asymptomatic individuals might be more numerous than symptomatic patients due to random interference (Supplementary 1 and Supplementary 2).
Figure 5. Output of the stochastic model and deterministic model and observed active COVID-19 cases. (a) January 2, 2021 to February 14, 2021; (b) July 4, 2021 to September 8, 2021; (c) September 8, 2021 to October 14, 2021; (d) October 14, 2021 to November 24, 2021; (e) November 24, 2021 to January 21, 2022.

In order to enhance the comprehension of the outcomes of the stochastic model, we generated a distribution plot of the total number of individuals affected by 100 simulations of the five outbreaks. The order of the figures is consistent with . Our findings reveal that the likelihood of a relatively minor outbreak is considerably low, leading to only a few individuals getting infected. Conversely, the probability of a significant epidemic is noticeably more than 60%. Furthermore, even two minor outbreaks in September and October can result in significant harm (Supplementary 3).
As an illustration, we examined the preventive and control measures for the epidemic that occurred from January 2, 2021 to February 14, 2021, by utilizing stochastic models. We evaluated the impact of measures such as wearing masks, quarantine, vaccination, and hospitalization, while varying intervention rates from 0 to 0.8. These images from left to right correspond to intervention measures of 0, 0.2, 0.4, 0.6, and 0.8 in size, respectively. Our analysis revealed that as the rates of quarantine for susceptible and exposed individuals, effective vaccination, effective mask-wearing, and hospitalization increase, there is a gradual decrease in the likelihood of a major outbreak. Notably, the hospitalization rate of asymptomatic individuals did not have a significant effect on the epidemic. We further observed that effective mask-wearing, quarantine of exposed individuals, and effective vaccination can shift the distribution of infected individuals toward a positive skewness. (See Supplementary 4–9 for detailed results.)
Discussion
Since the emergence of COVID-19 in Wuhan, China in December 2019, rigorous control measures have been implemented resulting in successful containment of the large-scale spread of the disease by April 2020. Despite this achievement, the intricate global landscape has imposed significant challenges on the efforts to prevent and manage the epidemic, leading to the reemergence of the COVID-19 epidemic in the country.
Modeling is an important method to describe the transmission of COVID-19 in China and assess the effectiveness of prevention and control measures. The SEIAQVHR model we constructed is methodologically robust and is the extensions the classical SEIR model. We proposed deterministic and stochastic models to model the incidence trends during five COVID-19 outbreaks in China. Unlike other models, in our deterministic model we considered the impact of asymptomatic infections, quarantine, vaccination and hospitalization on the spread of the epidemic. Our stochastic model is an extension of the deterministic model, where we considered random interference in the transmission of the disease to make the results more realistic. Our SEIAQVHR model was proposed to assessed the impact of various preventive and control measures on China’s efforts to combat the COVID-19 pandemic and provide a reference for the prevention and control of emerging infectious diseases that may occur in the future.
In this study, we analyzed the course of COVID-19 in China and developed a deterministic compartment model to describe disease transmission in the population. Our model included measures such as mask-wearing, hospitalization of symptomatic and asymptomatic patients, quarantine for susceptible and exposed individuals, and vaccination of susceptible individuals. The result of the fitted model indicate that the phase of the epidemic differed from the actual situation at the beginning. The starting point of the time period we selected is at the point where the previous epidemic tends to end and the next one does not start. At this point, the number of existing patients has a downward trend. Since the model fits the data according to the ordinary differential equation, the model will have a decrease at first, but the number of active cases is obviously increasing. It is inevitable that the model fitting results differ from the actual data. The parameters included in the model, such as recovery rate, isolation rate, etc., are fixed, but the actual situation is constantly changing, and the corresponding parameters are also in the process of changing. Therefore, the initial phase of the epidemic differed from the actual situation and the model wasn’t able to fit it well. After that, the actual situation tends to stabilize and the model fitted the actual data better. We will further improve this in subsequent studies. We will consider setting the parameters as changing parameters, which are changing over time.
Our estimated parameters showed that the transmission rate was highest among exposed individuals, followed by symptomatic individuals, and lowest among asymptomatic individuals. This suggests that exposed individuals, who may not display clinical symptoms, could spread the disease to susceptible individuals for an extended period of time. Therefore, we recommend focusing on this population, as ignoring them could lead to a severe COVID-19 epidemic. Our Rt calculation indicated that the outbreak was most severe between 2 January 2021 and 4 February 2021, similar to the actual situation. During this period, the effective vaccination rate was also found to be higher than other periods, possibly due to the promotion of vaccines during the severe epidemic. These results highlight the importance of controlling disease transmission rates to prevent rapid spread among the population.
The results of the stochastic model indicate that it is a useful tool for analyzing disease spread and providing additional information beyond what is obtained from deterministic models. Our analysis of five outbreaks revealed that most of the outbreaks had a high probability (>60%) of developing into serious outbreaks, with a potential total patient count exceeding 20,000, highlighting the highly infectious nature of COVID-19. This finding warrants attention, as it suggests that even with strict prevention and control measures, large-scale outbreaks are still possible.
We conducted a comprehensive analysis of parameters associated with interventions such as face masks, quarantine, vaccination, and hospitalization, using a stochastic model. Our results suggest that higher intervention efforts are required to control disease outbreaks within a shorter period in China. The reduction in the probability of a major outbreak mainly depends on the rate of effective vaccination, which directly reduces the number of susceptible individuals and thus reduces the spread of the disease in the population. Quarantine of susceptible and exposed individuals is a crucial measure to prevent the spread of the disease. We found that the higher the quarantine rate, the lower the probability of susceptible individuals coming into contact with infectious individuals (exposed, symptomatic, and asymptomatic individuals), thus reducing the spread of the disease. Hospitalization of symptomatic individuals has a significant impact on controlling the spread of the disease, but the hospitalization rate of asymptomatic individuals has little effect. Wearing masks is also an effective measure to control the spread of COVID-19. Our findings suggest that increasing the effective wearing rate of masks can be as effective in reducing the spread of COVID-19 as vaccination. However, no single intervention strategy can completely eliminate COVID-19, as even an increase of 80% in any one intervention would still result in a large number of patients. Nonetheless, a combination of multiple interventions can theoretically eliminate COVID-19.Citation20
While there are signs that COVID-19 may transit into an endemic phase, it is still unclear when this stage will be reached. However, our modeling approach provides some evidence how to manage future outbreaks of COVID-19 or other infectious diseases with similar characteristics. We found vaccination and non-pharmacological interventions like mask-wearing as most effective in curbing an epidemic outbreak. While vaccination may not completely stop transmission it can reduce the probability of transmission and wearing masks adds to this effect thereby stopping further spread of the infection in the community. These findings can not only help governments prepare for future outbreaks, but also provide an important reference for optimizing intervention plans for this and similar diseases.
Authors’ contribution
Conceptualization and Writing – original draft: Wenting Zha and Han Ni; Formal analysis and Software: Wenting Zha, Wentao Kuang and Yuxi He; Investigation and Data curation: Xuewen Yang and Jin Zhao; Validation and Methodology: Nan Ni, Nan Zhou and Yuxi He; Visualization and Project administration: Wentao Kuang, Liuyi Fu and Haoyun Dai; Writing – review & editing: Yuan Lv, Nan Zhou and Xuewen Yang; Funding acquisition: Yuan Lv and Wenting Zha. All authors have read and agreed to the published version of the manuscript.
Code
The research involves a significant amount of computational code, and we will only provide some of the key code snippets here:
s=randn(1,44);
for idx=1:length(T)-1
S(idx+1)=S(idx)-S(idx)*(1-(b+c+ds))*(a1*E(idx)+a2*I(idx)
+a3*A(idx))-b*S(idx)-ds*S(idx)+0.00025*s(idx)*S(idx);
E(idx+1)=E(idx)+S(idx)*(1-(b+c+ds))*(a1*E(idx)+a2*I(idx)
+a3*A(idx))-de*E(idx)-f*E(idx)+0.025*s(idx)*E(idx);
I(idx +1)=I(idx)+f*g*E(idx)-h*I(idx)-j*I(idx)+0.035*s(idx)*I(idx);
A(idx+1)=A(idx)+f*(1-g)*E(idx)-i*A(idx)-k*A(idx)+0.025*s(idx)
*A(idx);
Qs(idx +1)=Qs(idx)+ds*S(idx)+0.025*s(idx)*Qs(idx);
Qe(idx +1)=Qe(idx)+de*E(idx)+0.035*s(idx)*Qe(idx);
V(idx +1)=V(idx)+b*S(idx)+0.025*s(idx)*V(idx);
H(idx +1)=H(idx)+h*I(idx)+i*A(idx)-l*H(idx)+0.025*s(idx)*H(idx);
R(idx +1)=R(idx)+j*I(idx)+k*A(idx)+l*H(idx)+0.025*s(idx)*R(idx);
Q(idx +1)=Qs(idx)+Qe(idx);
Supplementary 2.tif
Download TIFF Image (28.1 MB)Supplementary 6.tif
Download TIFF Image (2.3 MB)Supplementary 9.tif
Download TIFF Image (2.4 MB)Supplementary 1.tif
Download TIFF Image (27.7 MB)Supplementary 3.tif
Download TIFF Image (5.1 MB)Supplementary 5.tif
Download TIFF Image (2.8 MB)Supplementary 8.tif
Download TIFF Image (3 MB)Supplementary 7.tif
Download TIFF Image (2.9 MB)Supplementary 4.tif
Download TIFF Image (2.6 MB)Disclosure statement
No potential conflict of interest was reported by the author(s).
Data availability statement
Data sources for this study: https://ourworldindata.org/; https://coronavirus.jhu.edu/data.
Supplementary material
Supplemental data for this article can be accessed on the publisher’s website at https://doi.org/10.1080/21645515.2024.2338953.
Additional information
Funding
References
- Grabowski A, Kosinski RA. Epidemic spreading in a hierarchical social network. Phys Rev E Stat Nonlin Soft Matter Phys. 2004;70:031908. doi:10.1103/PhysRevE.70.031908.
- Wang Y, Jin Z, Yang Z, Zhang ZK, Zhou T, Sun GQ. Global analysis of an SIS model with an infective vector on complex networks. Nonlinear Anal Real World Appl. 2012;13(2):543–11 doi:10.1016/j.nonrwa.2011.07.033.
- Jia J, Li Q. Qualitative analysis of an SIR epidemic model with stage structure. Appl Math Comput. 2007;193(1):106–15. doi:10.1016/j.amc.2007.03.041.
- Velasco RP. Modelos mixtos para medir la eficiencia de las intervencionessanitarias: el caso de la inmunización frente al influenzavirus en el medio ambiente. España: Universidad de La Rioja; 2006.
- Tracht SM, Del Valle SY, Hyman JM. Mathematical modeling of the effectiveness of facemasks in reducing the spread of novel influenza a (H1N1). PLOS ONE. 2010;5(2):e9018. doi:10.1371/journal.pone.0009018.
- Zhou T, Liu Q, Yang Z, Liao J, Yang K, Bai W, Lu X, Zhang W. Preliminary prediction of the basic reproduction number of the Wuhan novel coronavirus 2019‐nCoV. Wiley-Blackwell Online Open. 2020;13(1):3–7. doi:10.1111/jebm.12376.
- Wu JT, Leung K, Leung GM. Nowcasting and forecasting the poten- tial domestic and international spread of the 2019–nCoV outbreak o- riginating in Wuhan, China: a modelling study. Lancet. 2020;395(10225):689–97. doi:10.1016/S0140-6736(20)30260-9.
- Wan H, Cui JA, Yang GJ. Risk estimation and prediction of the transmission of coronavirus disease-2019 (COVID-19) in the mainland of china excluding Hubei province. Infect Dis Poverty. 2020;9(1):116. doi:10.1186/s40249-020-00683-6.
- Kissler SM, Tedijanto C, Goldstein E, Grad YH, Lipsitch M. Projecting the transmission dynamics of SARS-CoV-2 through the postpandemic period. Science. 2020;368(6493):860–8. doi:10.1126/science.abb5793.
- Hellewell J, Abbott S, Gimma A, Bosse NI, Jarvis CI, Russell TW, Munday JD, Kucharski AJ, Edmunds WJ, Funk S, et al. Feasibility of controlling COVID-19 outbreaks by isolation of cases and contacts. Lancet Glob Health. 2020;8(4):e488–96. doi:10.1016/S2214-109X(20)30074-7.
- Boukanjime B, Caraballo T, El Fatini M, El Khalifi M. Dynamics of a stochastic coronavirus (COVID-19) epidemic model with Markovian switching. Chaos, Solitons & Fractals. 2020;141:110361. doi:10.1016/j.chaos.2020.110361.
- Wintachai Phitchayapak PK. Stability analysis of SEIR model related to efficiency of vaccines for COVID-19 situation. Heliyon. 2021;7(4):e6812. doi:10.1016/j.heliyon.2021.e06812.
- Srivastav AK, Tiwari PK, Srivastava PK, Ghosh M, Kang Y. A mathematical model for the impacts of face mask, hospitalization and quarantine on the dynamics of COVID-19 in India: deterministic vs. stochastic. MBE. 2021;18(1):182–213. doi:10.3934/mbe.2021010.
- Liu P, Huang L, Din A, Huang X. Impact of information and Lévy noise on stochastic COVID-19 epidemic model under real statistical data. J Biol Dyn. 2022;16(1):236–53. doi:10.1080/17513758.2022.2055172.
- Lauer SA, Grantz KH, Bi Q, Jones FK, Zheng Q, Meredith HR, Azman AS, Reich NG, Lessler J. The incubation period of coronavirus disease 2019 (COVID-19) from publicly reported confirmed cases: estimation and application. Ann Intern Med. 2020;172(9):577–82. doi:10.7326/M20-0504.
- Li R, Pei S, Chen B, Song Y, Zhang T, Yang W, Shaman J. Substantial undocumented infection facilitates the rapid dissemination of novel coronavirus (SARS-CoV-2). Science. 2020;368(6490):489–93. doi:10.1126/science.abb3221.
- Chen TM, Rui J, Wang QP, Zhao Z-Y, Cui J-A, Yin L. A mathematical model for simulating the phase-based transmissibility of a novel coronavirus. Infect Dis Poverty. 2020;9(1):24. doi:10.1186/s40249-020-00640-3.
- Zhou F, Yu T, Du R, Fan G, Liu Y, Liu Z, Xiang J, Wang Y, Song B, Gu X, et al. Clinical course and risk factors for mortality of adult inpatients with COVID-19 in Wuhan, China: a retrospective cohort study. Lancet. 2020;395(10229):1054–62. doi:10.1016/S0140-6736(20)30566-3.
- Tang B, Wang X, Li Q, Bragazzi NL, Tang S, Xiao Y, Wu J. Estimation of the transmission risk of the 2019-nCoV and its implication for public health interventions. J Clin Med. 2020;9(2):462. doi:10.3390/jcm9020462.
- Balsa C, Lopes I, Guarda T, Rufino J. Computational simulation of the COVID-19 epidemic with the SEIR stochastic model. Comput Math Organ Th. 2021:1–19. doi:10.1007/s10588-021-09327-y.