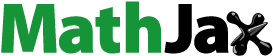
ABSTRACT
In the last 2 decades it has become increasingly evident that a large number of proteins are either fully or partially disordered. Intrinsically disordered proteins lack a stable 3D structure, are ubiquitous and fulfill essential biological functions. Their conformational heterogeneity is encoded in their amino acid sequences, thereby allowing intrinsically disordered proteins or regions to be recognized based on properties of these sequences. The identification of disordered regions facilitates the functional annotation of proteins and is instrumental for delineating boundaries of protein domains amenable to structural determination with X-ray crystallization. This article discusses a comprehensive selection of databases and methods currently employed to disseminate experimental and putative annotations of disorder, predict disorder and identify regions involved in induced folding. It also provides a set of detailed instructions that should be followed to perform computational analysis of disorder.
Introduction
The last 20 years have seen an increasing amount of experimental evidence suggesting an abundance of protein disorder within the protein realm. Intrinsically disordered proteins (IDPs), or hybrid proteins possessing both intrinsically disordered protein regions (IDPRs) and ordered domains, are functional proteins or protein domains that fulfill essential biological functions despite not having a highly populated stable secondary and tertiary structure under physiological conditions.Citation1 In fact, computational studies suggest that all proteomes of organisms in all kingdoms of life and all viral proteomes analyzed so far have considerable quantities of IDPs and IDPRs.Citation2-8 It has also been shown that the length and frequency of disordered regions are both greater in organisms of higher complexity, with at least one third of all eukaryotic proteins containing long IDPRsCitation4 and more than one tenth of these proteins being fully disordered.Citation9 Furthermore, considerably less than 30% of the crystal structures in the Protein Data Bank (PDB) are known to have no disorder.Citation10 Since regions of missing electron density are very frequent in PDB, this raises the question as to which is the minimal length of an IDPR. While previous reports set the limit to 20 residues,Citation1 the minimal length of an IDPR in DisProt 7.0 (i.e. the database of experimentally validated IDPs/IDPRs, see below),Citation11 is 5 residues, and the minimal length of 4 residues was used to annotate disordered regions used in the CASP experiments.Citation12,Citation13
In protein science, the existence of intrinsic disorder in proteins has been known for a long time. This is in spite of the fact that it contradicts the classical protein sequence-structure-function paradigm where the “lock-and-key” model is used to explain how a protein can achieve its biological function via folding into a unique, highly structured state determined by its amino acid sequence.Citation14 IDPs and IDPRs constitute a part of the “dark proteome” that includes entire proteins or protein regions for which the molecular conformation is entirely unknown.Citation15 Traditional ordered proteins have a relatively stable 3-D structure possess Ramachandran angles that vary only slightly around their equilibrium positions with occasional cooperative conformational switches. On the other hand, IDPs/IDPRs, despite being biologically active, fail to form specific 3D structures and exist as highly dynamic structural ensembles, either at the secondary or at the tertiary level.Citation5,Citation6,Citation16-21 Furthermore, intrinsic disorder is characterized by high structural heterogeneity. In fact, it is now recognized that IDPs/IDPRs may contain collapsed disorder (where the intrinsic disorder is present in a molten globular form) and extended disorder (where intrinsic disorder is present in a form of random coil or pre-molten globule) under physiological conditions in vitro.Citation5,Citation20,Citation22 It has also been shown that, in addition to completely ordered and disordered regions, proteins may contain regions of semi-disorder; i.e., fragments that have ∼50% predicted probability to be ordered or disordered.Citation23 Such semi-disordered regions have been shown to play key roles in protein aggregation, and to participate in protein-protein interactions involving induced folding.Citation23 The currently available structural data has been used to suggest that the heterogeneous spatiotemporal structure of IDPs/IDPRs can be described as a set of foldons, inducible foldons, semi-foldons, non-foldons, and unfoldons.Citation21,Citation24 The discovery of IDPs and IDPRs, which would not have been possible without bioinformatics, has drastically expanded the understanding of protein functionality, and exposed new and unexpected roles of dynamics, plasticity, and flexibility in the context of protein functions.
Experimentally, IDPs/IDPRs can be identified by the variety of physicochemical methods elaborated to characterize protein structure and self-organization.Citation20,Citation25-29 These methods include NMR spectroscopy;Citation20,Citation26,Citation30-32 missing electron density in X-ray crystallography maps;Citation33 optical rotatory dispersion spectroscopy (ORD);Citation18,Citation34 circular dichroism spectroscopy in the near-UVCitation35 and far-UV regions;Citation18,Citation34,Citation36,Citation37 Raman spectroscopy and Raman optical activity;Citation38 Fourier transform infrared spectroscopy (FTIR);Citation18 gel-filtration, viscometry, small angle neutron scattering (SANS), small angle X-ray scattering (SAXS), sedimentation, and dynamic and static light scattering;Citation27,Citation39,Citation40 fluorescent spectroscopy;Citation27,Citation40 aberrant mobility in SDS-gel electrophoresis;Citation41,Citation42 limited proteolysis (including conventional limited proteolysisCitation43-47 pulse proteolysis,Citation48 limited proteolysis combined to combined mass spectrometry,Citation49 and rapid and simple thermal proteolysis FASTpp assays;Citation50 H/D exchange;Citation27 abnormal conformational stability;.Citation40,Citation51-54 immunochemical methods;Citation55,Citation56 electron microscopy or atomic force microscopy (AFM),Citation57,Citation58 interaction with molecular chaperones;Citation40 and AFM-based single-molecule force spectroscopy (SMFS)Citation59 and the complementary single-molecule approach based on optical tweezers.Citation60,Citation61 Finally, the spectacular rise of cryo-EM in the last decadeCitation62 presages of an increasing number of examples where protein flexibility will be documented by this powerful, fast-growing structural technique (for 2 such examples see refs.Citation63,Citation64).
While there are IDPs/IDPRs that are able to perform their function while remaining completely disordered (e.g. entropic chains), many such proteins and regions experience a disorder-to-order transition after binding to their physiological partner(s), known as “induced folding.”Citation65 The functional relevance of disorder is the result of increased plasticity which allows for binding numerous and structurally distinct targets. Consequently, intrinsic disorder is a common and distinctive feature of “hub” proteins, with disorder acting as a measure of protein promiscuity.Citation66 As such, the majority of IDPs are involved in functions that involve multiple partner interactions, such as molecular assembly, molecular recognition, signal transduction and transcription, and cell cycle regulation.Citation67
Recognizing the presence of IDPRs in a query protein is becoming increasingly important. For instance, it facilitates functional annotation of proteinsCitation68 and is vital for delineating protein domains amenable to structural determinationCitation69-72 and for protein target selection;Citation73-75 the latter 2 are crucial for the most commonly used X-ray crystallography-based approach to protein structure determination. The field of protein intrinsic disorder has materialized when bioinformatics techniques were used to transform a set of anecdotal examples of structure-less biologically active proteins, originally thought to be interesting outliers of the protein realm, into a quickly growing and vital branch of protein science which has already shown the natural abundance of IDPs/IDPRs. Statistical analyses revealed that amino acid sequences that encode disordered regions are significantly different from those of ordered proteins, which allows IDPs to be predicted accurately from the protein sequence alone. Extended IDPs can be summarized as follows: (i) they have a biased amino acid composition, namely, enriched in G, S, P and depleted in W, F, I, Y, V, and L; (ii) they have a low secondary structure content; (iii) they tend to have a low sequence complexity; (iv) they are, on average, much more variable than ordered proteins, as they are more tolerant of substitutions due to their lack of structural constraints.
Various disorder predictors have been developed using the peculiar sequence features described above, (for detailed reviews of these predictors, see refs. Citation12, Citation69, Citation71, Citation76-80). The availability of different types of predictors allows users to select various aspects of disorder prediction that are suitable to their current studies, and choose an appropriate predictor.Citation12 It has also been shown that, since different disorder predictors are based on different definitions of disorder, combining several predictions from different predictors reinforces the reliability of the overall predictions on a specific position or region.Citation81-84 This reasoning has given rise to the development of metapredictors, which help users deal with the growing number of available disorder predictors and typically improve accuracy by combining the results of several different predictors. Some of these metapredictors also include the prediction of structured regions as a way to improve disorder predictions.
Computational analysis of intrinsic disorder can also be used to find potential functional regions. Since short regions of predicted order embedded within longer regions of predicted disorder have been shown to correspond to binding sites that fold upon complex formation,Citation85,Citation86 several specialized tools that identify short regions that undergo disorder-to-order transitions on binding (known as Molecular Recognition Features, MoRFs) were developed.Citation86-91 Two models complementary to MoRF-like interactions, the Short Linear Motif (SLiM) and the Eukaryotic Linear Motif (ELM), are based on sequence motifs that are recognized by peptide recognition domains.Citation92 A different approach is taken by the ANCHOR model, which identifies segments of disordered regions that are likely to fold in conjunction with a globular binding partner.Citation93,Citation94 Furthermore, a novel computational method DisoRDPbind was recently introduced for high‐throughput prediction of multiple functions of disordered regions that can be used to predict the RNA‐, DNA‐, and protein‐binding residues located in IDRs of the input protein sequences.Citation95,Citation96 One of the most recent methods, DFLpred, predicts disordered regions that serve as either intra-domain or inter-domain linkers.Citation97
Finally, it has been reported that sites of the enzyme-catalyzed posttranslational modifications, such as phosphorylation,Citation98 acetylation, methylation, and ubiquitinationCitation99 are commonly located within the IDRs. Several computational tools utilizing this information have been developed, such as DisPhos (Disorder-enhanced Phosphorylation predictor), which can efficiently find IDR-located phosphorylation sites with 76% accuracy for serine, 81% for threonine, and 83% for tyrosine.Citation98 More recently, another tool has been developed which is a unified sequence-based predictor of 23 types of PTM sites.Citation99
As the understanding of the pivotal importance of disordered regions in proteins (which includes functional interactions, binding, protein conformation, and molecular switch) grows, there is a growing interest in IDPs. Consequently, the number of requests submitted to servers hosting disorder prediction models has shot up exponentially, and due to the demanding resources required for predicting disorder, several research groups have built databases dedicated to storing annotations and predictions related to IDPs. These databases constitute valuable pools of information that can be utilized when seeking data on disordered regions of a protein of interest. They comprise experimentally assessed information and/or predictions from different disorder predictors, thereby fastening the identification of disordered regions. These databases allow fast and easy retrieval of annotated proteins, and allow the end user to search for these annotations using sequence of the query protein, its various identifiers, utilizing a sequence similarity-based search. While additional analyses are necessary to achieve a detailed description of the modular organization of a query protein in most cases, these databases nevertheless provide useful hints on the possible presence of disordered regions and some of their functions in a protein of interest.
In this article, we present a general suggested procedure for disorder prediction based on the combination of various tools for protein disorder prediction.
Materials
Computer with the internet connection.
Amino acid sequence of a query protein in FASTA format.
Methods
Retrieving sequence information from the UniProt database
The first step to using computational tools and to search biological databases is obtaining the sequence of a query protein. Arrive at the UniProt database by entering http://web.expasy.org/docs/swiss-prot_guideline.html in the Internet browser and selecting the “List of UniProtKB/Swiss-Prot (reviewed) entries” link located at the top of the front page. Use the following steps to download sequence information in FASTA format:
In the Search window (located at the top of the page), type the protein name after reviewed:yes and click Search.
On a Search in UniProt Knowledgebase page, choose a protein of interest from the list of hits and click corresponding link (which will be located in the column entitled Entry).
On the left-hand side of the corresponding UniProtKB entry page, look for a blue bar containing a link to Sequence and click this link. In the section entitled Sequence, click the FASTA link located within the light blue box.
Copy the content of the page, which includes a descriptive header related to the protein and a protein sequence. Keep this information as it will be used in the subsequent analysis. This can be done in Notepad or Microsoft Word. A separate document for each protein, which will store all the results of different analyses, is recommended.
Searching databases dedicated to IDPs
As a first step, it is recommended to check whether the protein of interest or a similar protein exists in publicly available databases dedicated to IDPs. The most efficient way to do this is to use the search engines by sequences that are provided by most of their interfaces.
Obviously, the higher the level of similarity between the matching sequences from these databases and the query sequence, the more relevant the information that can be obtained on the query protein.
A search result with more than 90% of sequence identity with a sequence from a database that contains experimental assessed information is the ideal case, but will rarely occurs since these databases still have only a few entries.
A similarly high sequence identity with an entry of a database for which annotations are based on predictions will have to be analyzed further: if all the disorder predictions stored are convergent with high confidence (i.e., with high probability) then the results obtained can be considered of sufficiently good quality.
In all other cases, it will be necessary to gather all the information that makes sense about the structured and disordered regions (boundaries) of the matching proteins which displays a reasonable level of similarity, and then to proceed to the next step (3.2) to complement the analysis by further predictions.
In case the search returns distant homologs of the sequence query (note that even an E-value – see below - inferior to 1.e-11 can be of interest), it is possible that the conserved and non-conserved regions can be identified, where the former will correspond to structured regions, and the latter will likely correspond to disordered regions, due to the higher selection pressure exerted on structured regions.Citation100
Obviously, one important question here is how to evaluate the degree of homology. In other words, how can one conclude whether the alignment between the query sequence and a given sequence from the database reflect biological significance? To test whether an alignment score reflects biological relatedness BLAST uses the E-value. The E-value reflects the number of times you expect to see alignment score X by chance: the lower the E-value, the fewer times you expect to see alignment score X by chance. If the alignment is not due to chance, then it may be due to a biological relationship between the 2 sequences. The e-value thus is a measure of how many such alignments you would expect to find in a database of a given size by chance. When BLAST is run to search for distantly related sequences, a relatively high e-value, typically 1.e-10, is used. Setting a threshold to 1.e-11 will therefore ensure retrieval of distant homologs.
DisProt (http://www.disprot.org) is historically the first database on disorderCitation101 and is also the largest publicly available database of disordered proteins whose disorder has been experimentally assessed. It has been recently upgraded and updated.Citation11 The current release contains information on more than 800 entries and has been curated to remove conflicting cases. As such, the information stored therein is highly valuable since it is experimentally assessed.
Paste the sequence in the “Search by sequence” field (raw format).
Select the search program: Smith waterman (default), or PSI-Blast for a more sensitive search and submit.
Check the score of the best blast hit on the result page (note that an E-value superior to 1.e-11 is probably not worth considering).
If the score is consistent, analyze the alignment of the corresponding matching sequence and note the boundaries of matching/mismatching regions.
Click on the reference of the entry of interest on top of the result page to display the details of the corresponding entries.
Compare the annotations of the selected entry with the boundaries obtained in step 4.
The Database of Disordered Protein Prediction (D2P2) (http://d2p2.pro/search)Citation102 contains disorder predictions for protein sequences from 1,765 complete proteomes and their variants obtained via the following 6 predictors: PONDR® VSL2b, PONDR® VLXT, PV2, PrDOS, IUPred, and ESpritz. D2P2 is also linked with the DisProt and IDEAL databases which include experimentally confirmed information about disordered regions. It is worth noting that D2P2 does not include results for viral proteomes, and does not cover all proteins from the currently covered organisms.
D2P2 uses a “Meta” approach by combining the results from several predictors and databases dedicated to disordered regions in proteins. An example of D2P2 output is provided in . Using D2P2 as a preliminary tool to search for disordered regions can help improve analysis of a query protein.
Figure 1. Output provided by the D2P2 database for human prothymosin α (UniProt ID: P06454), a well-known IDP. This output well illustrates the amount of information that can be obtained on both structural organization and post-translational modifications (PTM). Regions predicted as disordered by the various predictors are shown along with a predicted disorder agreement (with a color code ranging from clear to deep blue with increasing agreement). The majority of predictors predict the C-terminal region as disordered. The latter also contains predicted MoRFs.

Paste the sequence(s) (FASTA format as default) of interest in the “Sequences” field of the “Match Amino Sequence” section of the search page and click on the “Find proteins” button.
The result page displays the corresponding entries that are a 100% match for the query sequence(s). On the graphical part of the output, the matching entries from the IDEAL and DisProt databases, as well as the predictions of disordered regions from the panel of predictors, are aligned. Hovering the cursor over the shape will display complementary information such as the boundaries. If IDEAL or DisProt entries are found, clicking on their representation shapes will lead the user to the corresponding entries in these databases. The bottom part of the graphic displays agreement regarding the predicted disorder (corresponding to regions predicted to be disordered by more than 75% of the predictors) and show additional data such as phosphorylation sites or ANCHOR binding sites.
Below the graphical output, click on the tab titled “Disorder regions” to see a summary of the predicted disordered regions in the corresponding matching sequence. The left side of the page will display the predicted regions for which at least 75% of the predictors agreed, and the right part of the page will list all predictions per predictor.
In case the search returns no result, the user can go back to the search page and use the second form in the “CS-BLAST Amino Sequence” and enter a sequence of interest in the “Single sequence” field (FASTA format as default) and click on CS-BLAST Proteins to proceed to the result page that will have the same format as described above.
D2P2 (http://d2p2.pro/), much like MobiDB (see below), is a database of protein disorder predictions. Therefore, the result page contains a very useful picture containing the results of the multi-tool analysis of the disorder status of the query protein, as well as some disorder consensus bars, and multiple functional annotations. Note: this page also has useful information on the location of disordered and functional regions and PTM sites, which can be accessed by placing the cursor over the corresponding part of the plot. It is recommended to save the resulting figure since it serves as a useful illustration (see ).
Interpreting D2P2 data are very simple. This database provides an easily interpretable visual output of pre-computed disorder predictionsCitation102 which use the outputs of PONDR® VLXT,Citation103 2 versions of IUPred (IUPred-S and IUPred-L),Citation104 PrDOS,Citation105 PONDR® VSL2B,Citation106,Citation107 3 versions of ESpritz (ESpritz-D, ESpritz-N, and ESpritz-X),Citation108 and PV2.Citation102 The visual console of D2P2 displays 9 colored bars representing the location of disordered regions as predicted by the different disorder predictors. It also provides information on the curated sites of various posttranslational modifications. The next 2 lines with colored and numbered bars show the positions of predicted domains. The green-and-white bar in the middle of the plot shows the predicted disorder agreement between these 9 predictors, with green parts corresponding to disordered regions by consensus. The yellow bar shows the location of the predicted disorder-based binding site (MoRF region), whereas red, yellow, orange, blue, and violet circles at the bottom of the plots show the locations of phosphorylation, acetylation, glycosylation, methylation, and ubuquitylation sites, respectively.
IDEAL (http://www.ideal.force.cs.is.nagoya-u.ac.jp/IDEAL/blast.html) is the second oldest database, dedicated to proteins with experimentally assessed disorder.Citation109 The current release of this database (as of June 2016) contains 713 proteins with 464,962 total residues and 23,207 disordered residues. The IDEAL interface offers a blast engine that enables efficient retrieval of existing annotations pertaining to potential disordered regions within a query sequence.
Paste the sequence (raw format) in the “Blast Search” field.
Check the score of the best blast hit on the result page (note that an E-value superior to 1.e-11 is probably not worth considering).
If the score is consistent, analyze the alignment of the corresponding matching sequence and note the boundaries of matching/mismatching regions.
Click on the reference of the entry of interest on top of the result page to display the details of the corresponding entries. The disordered regions of the current entry are displayed in red. Detailed information can be accessed by clicking on the colored shapes.
Compare the annotations of the selected entry with the boundaries determined in step 3.
MobiDB (http://mobidb.bio.unipd.it/) contains intrinsic disorder annotations for more than 80 million entries (covering the entire PDB and DisProt) and predictions from 6 disorder predictors: IUPred, ESpritz, GlobPlot, DisEMBL, JRONN, and PONDR® VSL2B.Citation110
Although MobiDB is devoid of a blast/sequence search engine, it is cross linked with UniProt, which means that search executed in UniProt will lead to the corresponding entry in MobiDB. In addition, MobiDB has a search engine which uses keywords that can also use UniProt search syntax to retrieve an entry.
Enter the name of the protein of interest, or a more specific UniProt search syntax (e.g., name:“α-synuclein” AND organism:“human”).
On the result page, click on the protein that most corresponds to the query (the column titled “% LD” shows the percentage of residues involved in long disordered regions).
Alternatively, an access to MobiDB can be obtained directly from the UniProt page corresponding to the protein of interest. At the left-hand side of the corresponding UniProtKB entry page, look for a blue bar containing a link to Structure and click this link. In the section titled Structure, locate the MobiDB pointer and click Search link next to it. This will redirect to the same results section as describe in step 2.
The page displaying the protein annotations shows the regions of experimental and of predicted disorder in red and in orange, respectively. Hover the cursor over the colored shapes to get the boundaries and click on them or on the external databases references to get further details from the websites where annotations were picked up. The area entitled “predictors” lists all predictor results and displays a consensus of the predictions on the top of this list. For each prediction, the zoom icon enables retrieving the amino acid sequence in which the ordered and disordered regions are colored differently, thereby making it easy to copy/paste regions of interest.
MobiDB contains outputs from 6 disorder predictors (IUPred, ESpritz, GlobPlot, DisEMBL, JRONN, and PONDR® VSL2B includes information on the consensus disorder prediction, and provides the long IDPR annotation.Citation110 In fact, the MobiDB result page includes a plethora of very useful information about the query protein that includes the results of the multi-tool analysis of the disorder status of the query protein, structural information with corresponding PDB IDs (if available), as well as some functional annotations (such as STRING-based protein-protein interactions). Therefore, it is recommended to keep the content of the entire results page.
Interpretation of the MobiDB data is rather intuitive. The page starts with the general Sequence Annotations, where locations of long disordered regions and structure/disorder information from all available sources (e.g., structural data from the PDB in form of NMR and X-ray structures (if available), and results of multi-tool disorder prediction) are shown. If several NMR (or X-ray) structures are available for a query protein, then data shown in this section will correspond to the consensus of all NMR (or X-ray) data. Numeric disorder scores are shown next to the corresponding lines. The next line shows the location of Pfam domains, followed by the Detailed Disorder Annotations section, which contains multiple sub-sections showing results extracted from the individual PDB entries in a form of distribution of ordered and disordered regions. A consensus for all NMR or all X-ray structures is also shown. Each line ends with the corresponding numeric score. MobiDB also generates consensus disorder scores based on the outputs of 10 disorder predictors, including the 3 varieties of ESpritz(ESpritz-Xray, ESpritz-DisProt, and ESpritz-NMR),108 2 versions of IUPred (IUPred-S and IUPred-L),104 2 versions of DisEMBL (DisEMBL-HL and DisEMBL-465),Citation111 PONDR® VSL2,Citation106,Citation107 GlobPlot,112 and JRONN,Citation113 in addition to displaying the results of these individual predictors. This is followed by the Protein-Protein Interactions section that contains Known Structural Interactors (from PDB) and Known Experimental and Database Interactors (from STRING) subsections. Here, known and predicted binding partners are listed together with their corresponding disorder scores. The page is concluded with the Detailed Sequence Annotations section, where the Consensus Table and the Prediction Table shown numerically locations of disordered regions are located.
PED (Proteins Ensemble Database) (http://pedb.vib.be) is a database for the deposition of structural ensembles of IDPs and of denatured proteins based on small- angle X-ray scattering, nuclear magnetic resonance spectroscopy, and other data measured in solution.Citation114 Each entry consists of (i) primary experimental data with descriptions of the acquisition methods and algorithms used for the ensemble calculations, and (ii) the structural ensembles consistent with these data, provided as a set of models in a Protein Data Bank format. As of September 2016, PED contained 25,473 protein structures of 60 ensembles in 22 entries. Although PED does not possess a blast/sequence search engine, one can search it by using various criteria, such as gene name, protein name, UniProt ID, function, DisProt ID, GenBank ID, ensemble ID, and PDB code. If the PED stores data for the query protein or a related protein, it is likely that the protein possesses disorder (unless the structural ensemble has been obtained under denaturing conditions).
Enter the name of the protein of interest or a more specific UniProt search syntax and then click on “submit.”
Download experimental data and the structural ensemble from the result page.
Although the PDB (Proteins Data Bank) (http://www.rcsb.org/pdb/home/home.do# Subcategory-search_sequences.) is a database dedicated to structured proteins and protein regions, it indirectly provides information on disordered regions. It allows delineating disordered regions and alleviating ambiguity (i.e., structured regions will be readily recognized). The PDB also provides some information on disorder under the mention “REMARK465,” where regions of missing electron density are listed. It should be noted, however, that these regions are generally short, as long regions usually prevent crystallization which is the main route to structurally solve proteins.
Paste the sequence (raw format) in the “Option B: Paste Sequence” field and click on the “Run sequence search” button.
On the result page, select the PDB entries that match the query (check the E-values) and display the corresponding alignments by clicking on the “display full alignment” statement on the “Alignment row.”
Note the boundaries of the matching regions in the selected alignments.
Display the PDB entry pages of interest.
Report the boundaries of matching regions in the alignments to the secondary structure annotation of the PDB entry page selected. The regions for which a secondary structure element has been reported cannot be considered as disordered. Regions of missing electron density can be considered as disordered.
Analysis of protein amino acid composition
One of the specific features of an IDP or an IDR is the characteristic amino-acid compositional bias with low content of order-promoting residues (C, W, V, F, Y, L, I, and M) compensated by high content of disorder-promoting residues (Q, S, P, E, K, G, and A).Citation115-117 Consequently, the ordered or intrinsically disordered nature of a given protein can be estimated based on a simple analysis of its amino acid composition biases using the fractional difference in the amino acid approach.Citation115 Here, the fractional difference is calculated as where r ∈{A, C, D, E, F, G, H, I, K, L, M, N, P, Q, R, S, T, V, W, Y},
f(r) is the count of residues r in a given protein setand forder(r) is the count of residues r in the reference set of globular proteins, plotted for each amino acid using the Composition profiler.Citation118 In the resulting graph, negative bars correspond to amino acids that are underrepresented in a given protein when compared against the set of ordered proteins, whereas positive bars reflect the relative increase in the particular amino acid content in a query protein. A step-by-step protocol for the use of the Composition profiler is provided below:
Start the Composition profiler by entering http://www.cprofiler.org/ in the Internet browser and click the Run Profiler link located at the top right corner of the front page.
Paste the sequence of the query protein in the Query Sample window located on the left side of the window. In the Background Sample window (also located on the left side of the window) choose Dataset and select “PDB select 25” from the drop-down list. Find Output Options on the right side of the window, choose Ordering, and select Flexibility (Vihinen) from the drop-down list. Click the Draw Profile link located in a gray bar at the bottom of the Output Options section. The resulting page will contain a plot showing the fractional amino acid composition of the query protein and a table listing statistical parameters of this analysis.
If numerical values instead of a plot are needed, step 2 should be modified as follows. Paste the sequence of the protein in the Query Sample window located on the left side of the window. In the Background Sample window (also located on the left side of the window) choose data set and select “PDB select 25” from the drop-down list. Find Output Options on the right side of the window and choose Output format, where TXT (raw data) should be selected from the drop-down list. Then, choose Ordering and select Flexibility (Vihinen) from the drop-down list. Click the Draw Profile link located in a gray bar at the bottom of the Output Options section. The resulting page will now contain raw data in tabulated form, where the first column represents a single character residue name, the second column shows the calculated values of the fractional difference, and the third column gives errors.
To obtain a compositional profile of typical IDPs (which is a recommended step to obtain a reference plot), step 2 should be modified as follows. In the Query Sample window located on the left side of the window choose data set and select DisProt 3.4 from the drop-down list. In the Background Sample window (also located on the left side of the window) choose data set and select “PDB select 25” from the drop-down list. Find Output Options on the right side of the window, choose Ordering and select Flexibility (Vihinen) from the drop-down list. Click the Draw Profile link located in a gray bar at the bottom of the Output Options section. The resulting page will contain a plot showing the fractional amino acid composition of typical disordered proteins and a table listing the statistical parameters of this analysis.
If numerical values instead of a plot are needed, step 3 should be modified as follows. In the Query Sample window located on the left side of the window choose data set and select DisProt 3.4 from the drop-down list. In the Background Sample window (also located on the left side of the window) chose data set and select “PDB select 25” from the drop-down list. Find Output Options on the right side of the window and choose Output format where TXT (raw data) should be selected from the drop-down list. Then, choose Ordering and select Flexibility (Vihinen) from the drop-down list. Click the Draw Profile link located in a gray bar at the bottom of the Output Options section. The resulting page will now contain raw data in tabulated form, where the first column represents a single character residue name, the second column shows the calculated values of the fractional difference, and the third column gives errors.
To plot the compositional profile of a query protein versus the corresponding profile of typical IDPs, use numerical data from steps 3 and 5. Although the order of residues retrieved from Compositional profiler follows the Vihinen's flexibility scale, residues should be ranged as follows for better visual representation: C, W, I, Y, F, L, H, V, N, M, R, T, D, G, A, K, Q, S, E, and P; i.e., from the most order-promoting on the left to the most disorder-promoting on the right (see ).
Figure 2. Compositional profiling of an illustrative IDP, human prothymosin α (UniProt ID: P06454, A) in comparison with the compositional profile of typical ordered proteins. The compositional profile of typical intrinsically disordered proteins from the DisProt database is shown for comparison (B).
illustrates this approach by representing the relative amino acid composition of human prothymosin α (UniProt ID: P06454, open bars) vs. the compositional profile of a set of typical IDPs available in the DisProt databaseCitation119 (displayed as black bars). This analysis clearly shows that prothymosin α is enriched in major disorder-promoting residues and depleted in major order-promoting residues, thereby having an amino acid composition very similar to typical IDPs.
Running disorder predictions
Over the last 10 years a number of disorder predictors have been developed which exploit the sequence bias of disordered proteins. Different types of protein disorder exist,Citation120 separated by the extent (i.e., the amount of residual secondary and/or tertiary structure) and the length of disorder. Since different predictors rely on different physico-chemical parameters, a given predictor can be more efficient in detecting a given feature of a disordered protein. Hence, predictors utilizing different aspects of disorder have to be combined in order to attain predictions good enough to decipher the modular organization of a protein.Citation69,Citation70,Citation76,Citation121-124
Disorder predictors can be broken down into 3 categories: those that have been trained on data sets of disordered proteins, those that have not been trained on any data set, and metapredictors that blend the results of different predictors. Some predictors use multiple alignments in the computation of their predictions, and the most advanced ones include structural information from the PDB when available. As previously mentioned, alignments with homologous proteins can further help identify potentially disordered regions since the pressure of selection in disordered regions is not as vital as in structured regions. Accordingly, alignments will typically display a lack of conservation for disordered regions.
While predictors trained on data sets of disordered regions identify disordered regions on the basis of the peculiar sequence properties that characterize them, the other predictors identify disorder as lack of ordered 3D structure. The second group of predictors avoid the shortcomings and biases associated with disordered data sets. Therefore, they are expected to perform better than the former on disordered proteins presently under-represented in training data sets (i.e., fully or mostly disordered proteins).
The performance of predictors depends on both the type of disorder they predict and the type of disorder against which they were trained and tested. This is evident when comparing the results of several recent comparative assessments.Citation12,Citation79,Citation80 In spite of the fact these studies point to certain methods are being more accurate than other methods, they do not agree on which specific method is the most accurate. In addition, many of these predictors achieve similar goals. It is therefore not meaningful to try to define the “best” predictor. Rather, it is advised to combine multiple prediction methods, and in particular predictors relying on different principles, to improve predictive performance of the disorder predictions.Citation69,Citation71,Citation76,Citation83,Citation84,Citation125
Metapredictors are particularly well-suited to improve the analysis of disorder since they combine the results of several predictors and provide a unified view of the core predictors used. However, since disorder-related databases already return consensus predictions from multiple predictors, the added value of running metapredictors is primarily derived from the possibility of retrieving additional information from non-redundant predictors (i.e., predictors not already included in the above described-databases) which complements the already gathered information.
CASP (Critical Assessment of Techniques for Protein Structure Prediction; http://www.predictioncenter.org/) is a biannual, worldwide effort to evaluate methods for prediction of protein structure, which also includes empirical assessment of predictors of disorder. This assessment involves comparison of the putative disorder predicted by a large set of predictive tools against experimental data for proteins for which this experimental data were not yet released. In CASP10 (2012), the last CASP that included assessment of predictions of disorder, the top 3 predictors were PrDOS, DISOPRED, and MFDp.Citation12 Their results were shown to be statistically better than the remaining 23 predictors.Citation12 Their results were also shown to improve with the increase of the disorder region length cutoff from 4 to 20 to 30 residue-long segments.
Individual disorder predictors
Since the metapredictors make use of previously developed individual disorder predictors, we provide a short description of the architectures of several individual disorder predictors, along with guidelines on how to run them.
Predictors trained on data sets of disordered proteins
CSpritz (http://protein.bio.unipd.it/cspritz/) utilizes sequence profiles obtained from PSI-BLAST and structure predictions. It is a disorder predictor for high-throughput applications, including NMR mobility. CSpritz uses 2 separate predictors based on vector machines trained on different data sets.Citation126 The training data set of short disordered regions (less than 45 residues) was derived from a subset of PDB sequences with short regions of missing density, while the training data set of long regions was derived from both DisProt and from a subset of the PDB (i.e., PDBselect25). This server allows the submission of several sequences at one time, and offers the possibility of choosing between predictions of short or of long disordered regions.
Paste the sequence in fasta format, enter the name of the query sequence (optional), and enter the e-mail address (optional).
Choose the data set for disorder prediction (i.e., X-ray, “short,” or DisProt “long”) and click on ”Submit.”
Prediction results are returned online. Residues predicted to be disordered or ordered are indicated by a red “D” or a black “O,” respectively. Statistics (i.e., percentage of disorder, length distribution of segments, number of disordered regions of > 30 or of > 50 residues in length) are also displayed.
DICHOT (http://idp1.force.cs.is.nagoya-u.ac.jp/dichot/index.html) was developed by the same research group that established the IDEAL database.Citation127 DICHOT's process of disorder prediction includes the assignment of structural domains (SDs). It divides the entire amino acid sequence of a query protein into SDs and IDRs, and also introduces sequence conservation as a third aspect, which based on the common observation that IDRs are less conserved than structured regions.
Enter the e-mail address, paste the protein sequence (plain text), and click on the “Submit” button.
The results are sent by e-mail. Regions predicted to be disordered are highlighted by red bars. Prediction results from PDB (3D structures), SEG (low complexity region), SCOP domains (classified structures), and sequence motifs (PFAM domain)are shown with colored boxes. A graph showing the probability of the prediction of disorder at each position is also shown. The bottom of the page displays the boundaries of the various regions.
DisEMBL (http://dis.embl.de) is based on a neural network and consists of 3 separate predictors, trained on separate data sets, that comprise residues within “loops/coils,” “hot loops” (loops with high B-factors – i.e., very mobile from X-ray crystal structure), or loops that are missing from the PDB X-ray structures (called “Remark 465”).Citation128 Among these, the only true disorder predictor is Remark 465, since the others only predict regions lacking regular secondary structure. DisEMBL also provides prediction of low sequence complexity (CAST predictor) and aggregation propensity (TANGO predictor).
1. Enter the SwissProt ID (or AC) or paste the sequence in raw format in the foreseen field, enter Title (optional), click on “DisEMBL protein.”
2. The result page provides a postcript (ps) file that can be downloaded. The amino acid sequence of the protein is given below the graph, with residues in loops and hot loops colored blue and red, respectively. Disordered residues, as predicted by Remark 465, are shown in green.
DISOPRED (http://bioinf.cs.ucl.ac.uk/psipred/?disopred=1) is based on support vector machine classifiers trained on PSI-BLAST profiles.Citation129 It incorporates information from multiple sequence alignments since its inputs are derived from sequence profiles generated by PSI-BLAST. Hence, prediction accuracy is lower if there are few homologues. In general, implementing sequence alignments in disorder predictions allows a more reliable identification of disordered regions and a better definition of their boundaries. Sequence alignment also enables identifying regions that are enriched in the same amino acids (i.e., regions that have a similar compositional bias), which not only contributes to a better localization of disordered regions within a query protein, but also allows highlighting conserved sites that may correspond to functionally important regions.
DISOPRED secured the second best finish at the CASP10.
Paste the sequence in raw format, the e-mail address (optional), and provide a short identifier for the query sequence (compulsory). Additional predictions methods can be run to complement the DISOPRED prediction by ticking the corresponding checkboxes (e.g: PSIPRED for secondary structure, MEMPACK for support vector machine prediction of transmembrane topology and helix packing).
Click on “Predict.”
Prediction results are displayed on the web page, but jobs typically take at least 30 minutes. Upon completion, an e-mail is sent with a link to access the results page. The summary page displays the disordered predictions, which are represented by red and green boxes over the sequence of the query. Links to disorder profile plots (png formats) are available from the DISOPRED tab on the result page.
DISpro is based on a neural networkCitation130 and is available from the SCRATCH server (http://scratch.proteomics.ics.uci.edu/).It combines sequence profiles obtained by PSI-BLAST, solvent accessibility, and secondary structure predictions,. This predictor was trained on disordered sequences (i.e., regions of missing atomic coordinates) derived from the PDB.
Enter the e-mail address (required), the sequence name (optional), paste the sequence in raw format, select the disorder predictor (i.e:DISpro) and predictions to be run by ticking the appropriate box (eg: SSpro for Secondary Structure or ABTMpro for α β Transmembrane), and click on “Validate.”
Prediction results are sent by e-mail. Residues predicted to be disordered or ordered are indicated by a “D” or an “O,” respectively. Per residue disorder probabilities are also provided.
DisProt PONDR® VL2, PONDR® VL3, PONDR® VSL2 and derivatives. The DisProt server (http://www.dabi.temple.edu/disprot/predictor.php) provides access to several predictors. Among them are 2 variants of the PONDR® VSL2 predictor: PONDR® VSL2B is the baseline model that uses only 26 features calculated from the amino acid sequence, while the more accurate PONDR® VSL2P uses 22 additional features derived from PSI-BLAST profiles. The PONDR® VSL2 predictor package, which integrates the full set of different features (which include PSI-BLAST profiles, residue features, and secondary structure PHD and PSIPRED predictions), can be downloaded from http://www.dabi.temple.edu/disprot/predictorVSL2.php.
PONDR® VL3 uses several features from a previously introduced PONDR® VL2 predictor,Citation120 but benefits from optimized predictor models and a slightly larger (152 vs. 145) set of disordered proteins that was corrected for mislabeling errors found in the smaller set. The PONDR® VL3 predictor is based on an ensemble of feed-forward neural networks trained on a data set obtained from both DisProt and PDB. PONDR® VL3H uses the same method as VL3, but uses homologues of the disordered proteins in the training stage, while PONDR® VL3P uses attributes derived from sequence profiles obtained by PSI-BLAST searches.Citation131,Citation132 Requests are limited to 100 per IP address per day, and the maximum length of a query sequence is limited to 5,000 residues. For the PONDR® VL3E predictor, which combines predictions from PONDR® VL3P and PONDR® VL3H, up to 10 queries no longer than 500 residues can be processed per IP address per day. Predictions for PONDR® VL3E are sent by e-mail upon completion.
Choose the predictor to be run: PONDR® VL2, PONDR® VL3, PONDR® VL3E, PONDR® VL3H, PONDR® VSL2P, and PONDR® VLS2B.
Paste the sequence in raw format, enter the e-mail address, and click on “submit.”
Prediction results are returned online and the plot can be saved (png format) by clicking on it with the right mouse button. The output also provides a table with disorder probabilities per residue. Values over the significance threshold of 0.5 suggest disordered residues.
DNDisorder (http://iris.rnet.missouri.edu/dndisorder/)Citation133 make uses of deep networks (DNs). DNs are similar to neural networks but contain more layers and are trained in a slightly different manner. The server uses CUDA and several graphical processing units to reduce the runtime of the computation of the results.
Paste the sequence in plain text or fasta format and enter the e-mail address in the corresponding required field. Enter a title for the job (optional), then click on the “Submit job” button.
Results are returned in CASP format (PFRMAT DR) via e-mail.
ESpritz (http://protein.bio.unipd.it/espritz/) is based on a machine learning method which does not require sliding windows or any complex sources of information (Bi-directional Recursive Neural Networks (BRNN)).Citation108 It includes 3 version that predict disorder based on the annotations from X-ray crystal structures, NMR-derived structures and the DisProt database.
Enter the e-mail address (optional), the name of the query sequence (optional), and paste the sequence in raw format.
Choose the type of disorder (i.e., X-ray, NMR, or Disprot) and click on “Predict.”
Prediction results are sent by e-mail. Residues predicted to be disordered are tagged with a “D” character. It is also possible to get disorder predictions (with disorder probability) in text format by using the corresponding link on the top of the result page.
Globplot 2 (http://globplot.embl.de) uses the “Russell/Linding” scale that displays the propensity for a given amino acid to be in “random coil” or in “regular secondary structure.”Citation134 It also provides an easy overview of modular organization of large proteins due to user-friendly, built-in SMART, PFAM, and low complexity predictions. Note that in Globplot outputs, changes of slope often correspond to domain boundaries.
Enter the SwissProt ID (or AC) or paste the sequence in raw format in the foreseen field, enter Title (optional), and click on “GlobPlot now.”
The result page provides a postscript (ps) file that can be downloaded. The amino acid sequence of the protein is given below the graph, with disordered residues colored in blue.
OnD-CRF (http://babel.ucmp.umu.se/ond-crf/) predicts disorder using conditional random fields (CRF).Citation135
Paste the sequence in raw or fasta format or upload the query sequence from a file, and click on “Submit query” (you can also choose to receive results by e-mail)
Prediction results are returned online. The plot can be saved as an image (png format) by clicking on it with the right mouse button. The threshold above which residues are considered as disordered is dynamic and indicated above the plot. Below the graph, the amino acid sequence and boundaries of disordered regions are both provided, with disordered residues shown in red. Disorder probabilities per residue can be seen by hovering over the amino acid sequence shown below the graph.
POODLE-I (Prediction Of Order and Disorder by machine LEarning) is a predictor that uses machine learning approaches on only amino acid sequences in order to predict disordered regions. There are 3 different versions of this method (S-L-W) that are all specialized in the detection of different categories of disordered regions: POODLE-S is specialized for short disordered regions, POODLE-L for long disordered regions (more than 40 consecutive amino acids), and POODLE-W for proteins that are mostly disordered. POODLE-I constitutes a metapredictor approach of the POODLE series that was made available in 2008. It integrates the 3 POODLE versions (S-L-W) and also offers the option to include structural information predictors based on a work-flow approach.Citation136 All POODLE series can be used from http://mbs.cbrc.jp/poodle/poodle.html. The results are sent by email in CASP format and a link for the html page is also provided, displayed as a graphical plot of the POODLE prediction and a table that indicates the probability to be disordered for each residue in the input sequence.
Paste the sequence in raw format, enter the e-mail address, choose the type of prediction (“missing residues” or “High B-Factor residues”), and click on “submit”
Prediction results are sent by e-mail, with a link to a graphical output. Residues with disorder probabilities higher than 0.5 are considered to be disordered. Probabilities per residue are given upon positioning the pointer on the disorder curve. The plot can be saved by using the “screen capture” option of the user's computer (such as the Print Screen button for Windows users).
PONDR (Predictor of Natural Disordered Regions) (http://www.pondr.com/cgi-bin/PONDR/pondr.cgi), a neural-network-based on local amino acid composition, flexibility, and other sequence features, was the first predictor.Citation116 Although access to PONDR was limited in the past, the predictor is now publicly available in various versions: PONDR® XL1_XT, PONDR® VLXT, PONDR® VL3-BA, PONDR® XAN_XT, and PONDR® VSL2. To overcome the poor accuracy of the first PONDR predictors for short disordered regions (< 30 residues), Dunker's group developed the PONDR® VSL2 predictor. This method is based on a support vector machine and aims at providing accurate predictions that are not affected by the length of the disordered region.Citation137 PONDR® VSL2 was ranked among the best predictors in CASP7,Citation138 and turned out to perform equally well on regions of > 30 and of < 30 residues, and was able to identify short disordered regions that were mispredicted by the previous PONDR predictors. PONDR® VLXT is unique in that it can highlight potential protein-binding regions, indicated by sharp drops in the middle of long disordered regions. On the main page, it is also possible to choose to run a Charge-Hydrophathy (CH plot) and a CDF (Cumulative Distribution Function) analysis.
Enter the protein name and paste the sequence in raw (or fasta) format and click on “submit.”
The result is provided as a plot. Values over the significance threshold of 0.5 suggest disordered residues. Segments composed of more than 40 consecutive disordered residues are highlighted by a thick black line.
PrDOS (http://prdos.hgc.jp/cgi-bin/top.cgi) is composed of 2 predictors: a predictor based on the local amino acid sequence, and one based on template proteins (or homologous proteins for which structural information is available).Citation105 The first predictor is implemented using support vector machines for the position specific score matrix (or profile) of the input sequence. More precisely, a sliding window is used to map individual residues into a feature space, similar to secondary structure prediction used by methods like PSIPRED. The second predictor assumes the conservation of intrinsic disorder in protein families, and is simply implemented using PSI-BLAST and a specific measure of disorder. The final prediction is a combination of the results of the 2 predictors. This method was ranked first at the CASP10.
Paste the sequence in raw format, enter the sequence name and the e-mail address (optional), and click on “predict”
A new page appears where the estimated calculation time is indicated. The user is asked to confirm the submission by clicking the OK button.
On the results page, the plot can be saved as an image (png format) by clicking on it with the right mouse button. Residues with disorder probabilities higher than 0.5 are considered to be disordered. Above the graph, the amino acid sequence is shown, and disordered residues are shown in red. Disorder probabilities per residue can be obtained by clicking on the download button below the graph, which yields an output in the casp or csv format.
PreDisorder (http://sysbio.rnet.missouri.edu/predisorder.html)Citation139 was ranked among the best predictors in disorder prediction during CASP8 under the group name MULTICOM-CMFR.Citation140 The prediction is based on an ab initio neural network method. A PSI-BLAST profile of the sequence, along with the predicted secondary structure and solvent accessibility, is fed into a 1D Recursive Neural Network (1D-RNN) that forms the disorder predictions.
Enter the e-mail address, the protein name, and its sequence in the corresponding field, and click on the “Predict” button.
Results typically take several hours to process and are sent by e-mail in the form of 3 lines: the first line displays the amino acid sequence, the second line shows (dis)order predictions (where residues predicted to be disordered and ordered are tagged with D and O, respectively), and the third line displays the probability of disorder. Residues are considered to be disordered if their disorder probability is above 0.5.
RONN (http://www.strubi.ox.ac.uk/RONN) uses an approach based on a bio-basis function neural network. It relies on the calculation of “distances,” as determined by sequence alignment, from well-characterized prototype sequences (ordered, disordered, or a mixture of both). Its key feature is that amino acid side chain properties are not considered at any stage.Citation113 The present version of the predictor is no longer maintained and is expected to be superseded by a brand-new predictor in the near future.
Paste the sequence in fasta format (note that aminoacids have to be in upper case) and click on “Send sequence”
Prediction results are returned online and the plot can be saved as an image (png, jpg, pdf, svg) format from the right tab on top of the graph. The amino acid sequence of the protein is given below the graph. Disordered residues correspond to locations where the graph goes over the “Order/Disorder” boundary, marked in red. The per residue disorder probabilities can also be found above the graph.
SPINE-D (http://sparks-lab.org/SPINE-D/) uses a single neural-network based technique that makes a 3-state prediction reduced to 2 states (ordered - disordered).Citation141 The predictions made by SPINE-D are dependent on the balance in the relative populations of ordered and disordered residues in short and long disordered regions in the test set. The program is also available as a standalone version that is recommended for analysis of large data sets (e.g., genomics projects).
Paste the sequence in fasta format and (optionally) enter the e-mail address and a target ID in the corresponding field, then click on the submit button.
Results are provided in CASP format for disorder predictions (4 columns: position, sequence, Disordered or Ordered status, Probability of the prediction).
Predictors that have not been trained on disordered proteins
DRIP-PRED (Disordered Regions In Proteins PREDiction) (http://www.sbc.su.se/∼maccallr/disorder/cgi-bin/submit.cgi) is based on a search of sequence patterns obtained by PSI-BLAST that are not typically found in the PDB (http://www.forcasp.org/paper2127.html). If a sequence profile is not well represented in the PDB, then it is expected to have no ordered 3D structure. For a query sequence, sequence profile windows are extracted and compare with the reference sequence profile windows, and then an estimation of disorder is performed for each position. As a last step, the results of this comparison are weighed by PSIPRED predictions. Since predictions can take up to 8 hours, it is preferred to choose that they are sent by e-mail. In this latter case, the user is sent an e-mail with a link to the result page.
Enter the e-mail address (optional), paste the sequence in raw format, click on “Submit,” and provide a job name (optional).
Prediction results are shown in the amino acid sequence format with disordered residues underlined, and color coded as a function of disorder probabilities. Per residue disorder probabilities are given below the amino acid sequence in the casp format.
FoldUnfold (http://bioinfo.protres.ru/ogu/) calculates the expected average number of contacts per residue from the amino acid sequence alone.Citation142 The average number of contacts per residue was computed from a data set of globular proteins. A region is considered as natively unfolded when the expected number of close residues is less than 20.4 for its amino acids and the region is greater or equal in size to the averaging window.
Paste the sequence in fasta format, and click on the “Predict” button.
Prediction results are returned online. Boundaries of disordered regions (unfolded) are given at the bottom of the page. In the profile, disordered residues are shown in red.
IUPred (http://iupred.enzim.hu) uses an algorithm that evaluates the energy resulting from inter-residues interactions.Citation104 Although it was derived from the analysis of only the sequences of globular proteins, it allows the recognition of disordered proteins based on their lower interaction energy. The method offers a new way to examine the lack of a well-defined structure, which can be viewed as a consequence of a significantly lower capacity to form favorable contacts, correlating with studies by Galzitskaya's group.Citation142
Enter the sequence name (optional), paste the sequence in raw format, choose the prediction type (short disorder, long disorder, structured regions), choose “plot” in output type and adjust the plot window size, and click on “Submit.”
Prediction results are promptly returned online and the plot can be saved (png format) by clicking on it with the right mouse button. The output also provides a table with disorder probabilities per residue. Values over the significance threshold of 0.5 suggest disordered residues.
Binary disorder predictors
The charge/hydropathy method and its derivative FoldIndex. The charge/hydropathy analysis, a predictor that has not been trained on disordered proteins, is based on the novel idea that protein folding is governed by a balance between attractive forces (of hydrophobic nature) and repulsive forces (electrostatic, between similarly charged residues).Citation18 Thus, globular proteins can be distinguished from unstructured ones based on the ratio of their net charge vs. their hydropathy. The Mean Net Charge (R) of a protein is determined as the absolute value of the difference between the number of positively and negatively charged residues divided by the total number of amino acid residues. It can be calculated using the program ProtParam at the ExPASy server (http://www.expasy.ch/tools). The Mean Hydrophobicity (H) is the sum of normalized hydrophobicities of individual residues divided by the total number of amino acid residues minus 4 residues (to take into account fringe effects in the calculation of hydrophobicity). Individual hydrophobicities can be determined using the Protscale program at the ExPASy server, using the options “Hphob / Kyte & Doolittle,” a window size of 5, and normalizing the scale from 0 to 1. The values computed for individual residues are then exported to a spreadsheet, summed and divided by the total number of residues minus 4, to yield (H). A protein is predicted as disordered if
Alternatively, charge/hydropathy analysis of a query sequence can be obtained by choosing this option on the main page of the PONDR server.
Unfortunately, this approach acts as a binary predictor, i.e., it only gives a global (and not positional) indication, which is not valid if the protein comprises both ordered and disordered regions. Consequently, it can only be used with protein domains, requiring a prior knowledge of the modular organization of the protein.
A derivative of this method, FoldIndex (http://bip.weizmann.ac.il/fldbin/findex), solves this problem by computing the charge/hydropathy ratio using a sliding window along the protein.Citation143 However, since the default sliding window is set to 51 residues, FoldIndex does not provide reliable predictions for the N- and C-termini, and is therefore not recommended for proteins with less than 100 residues.
Paste the sequence in raw format and click on “process.”
The results page shows a plot that can be saved as an image (png format) by clicking on it with the right mouse button. Disordered regions are shown in red and have a negative “foldability” value, while ordered regions are shown in green and have a positive value. Disorder statistics (longest disordered region, number of disordered regions, number of disordered residues and scores) are given below the plot.
The cumulative distribution function (CDF) is another binary classification method.Citation144,Citation145 The CDF analysis summarizes the per-residue predictions by plotting predicted disorder scores against their cumulative frequency, which allows ordered and disordered proteins to be distinguished based on the distribution of prediction scores.Citation144,Citation145 A CDF curve gives the fraction of the outputs that are less than or equal to a given value. At any given point on the CDF curve, the ordinate gives the proportion of residues with a disorder score less than or equal to the abscissa. The outputs of predictors are unified to produce per-residue disorder scores ranging from 0 (ordered) to 1 (disordered). In this way, CDF curves for various disorder predictors always begin at the point (0, 0) and end at the point (1, 1) since disorder predictions are defined only in the range [0, 1] with values less than 0.5 suggesting a propensity for order and values greater than or equal to 0.5 suggesting a propensity for disorder. Since the majority of fully disordered protein residues possess high predicted disorder scores, they have a very low percentage of residues with low predicted disorder scores. On the contrary, the majority of residues in ordered proteins are predicted to have low disorder scores. Therefore, the CDF curve of a structured protein would increase very quickly in the domain of low disorder scores, and then flatten out in the domain of high disorder scores. For disordered proteins, the CDF curve would go upward in the domain of low disorder scores, then increase quickly in the domain of high disorder scores. Consequently, CDF curves for fully ordered proteins tend to be convex since a high proportion of the prediction outputs are below 0.5, while fully disordered proteins typically yield concave curves since a high proportion of the prediction outputs are above 0.5. It therefore stands to reason that all fully disordered proteins should be located at the lower right half of the CDF plot, whereas all fully ordered proteins should fall in the upper left half of this plot.Citation144,Citation145 A boundary line between fully disordered and fully ordered proteins can be identified by comparing the locations of their CDF curves,. This boundary line can be used to separate ordered and disordered proteins with an acceptable accuracy, with proteins whose CDF curves are located above the boundary line being likely to be structured, and proteins with CDF curves below the boundary being likely to be disordered.Citation144,Citation145 CDF-plots based on various disorder predictors have different accuraciesCitation145 PONDR® VSL2-based CDF was found to be the most accurate, up to 5–10% higher than the second best of the other 5 CDF functions used for the separation of fully disordered proteins from structured proteins also containing disordered loops or tails. When considering the separation of fully structured from fully disordered proteins, the CDF curves derived from the various disorder predictors all were found to exhibit similar accuracies.Citation145 CDF analysis can be run from the PONDR server ([LINK TO SERVER]).
Enter the protein name and paste the sequence in raw (or fasta) format, choose the disorder predictor to be run, select CDF, and click on “submit.”
The result is provided as a plot than can be saved (gif format) by clicking on it with the right mouse button.
The CH-CDF plot is an analytical tool combining the outputs of 2 binary predictors, the Charge-Hydropathy (CH) plot and the CDF plot, both of which predict an entire protein as either ordered or disordered.Citation146 The CH-plot places each protein onto a 2D graph as a single point by taking the mean Kyte-Doolittle hydropathy of a protein as the X coordinate and the mean net charge of the same protein as the Y coordinate. In a CH-plot, structured, globular proteins and fully disordered, and can be separated by a boundary line.Citation18 Proteins located above this boundary are likely to be disordered, while proteins located below this line are likely to be structured. The vertical distance on CH-plot from the location of the protein to the boundary line, referred to as CH-distance, therefore serves as a scale of disorder (or structure) tendency of the protein. In CDF-plots (described above), ordered proteins curves tend to stay on the upper left half, whereas disordered proteins curves tend to be found at the lower right half of the plot. An approximately diagonal boundary line separating the 2 groups can be plotted, and the average distance of the CDF curves from this boundary, known as CDF-distance, can act as a measure of the disorder (order) status of a given protein. A new method called the CH-CDF plot was designed by combining the CH-distance and the CDF-distance,.Citation146 The CH-CDF plot provides very useful information regarding the general disorder status of a given protein. After setting up boundaries at CH = 0 and CDF = 0, the entire CH-CDF plot can be split into 4 quadrants. Starting from the upper right quadrant, by taking the clockwise sequence, the 4 quadrants are named Q1 (upper right), Q2 (lower right), Q3 (lower left), and Q4 (upper left). Proteins in Q1 are structured by CDF but disordered by CH; proteins in Q2 are predicted to be structured by both CDF and CH; proteins in Q3 are disordered by CDF but structured by CH; and proteins in Q4 are predicted to be disordered by both methods. The location of a given protein in this CH-CDF plot gives information about its overall physical and structural characteristics. Unfortunately, there is currently no publicly available automated server for the generation of CH-CDF plots.
Non-conventional disorder predictors
The hydrophobic cluster analysis (HCA) is a non-conventional disorder predictor in that it provides a graphical representation of the sequence that helps identify disordered regions. Although HCA was not originally intended to predict disorder, it is very useful for discovering disordered regions.Citation147 HCA outputs can be obtained from http://mobyle.rpbs.univ-paris-diderot.fr/cgi-bin/portal.py?form = HCA#forms::HCA and from the MeDor metaserver (http://www.vazymolo.org/MeDor/). HCA provides a 2-dimensional helical representation of protein sequences in which hydrophobic clusters are plotted along the sequence ().Citation147 As such, HCA is not strictly speaking a predictor. Disordered regions are recognizable as they are depleted (or devoid) in hydrophobic clusters. HCA is unique since it provides a representation of the short range environment of each amino acid, which provides information not only on order/disorder but also on folding potential. Although HCA does not provide a quantitative prediction of disorder and rather requires human interpretation, it provides additional, qualitative information, unlike automated predictors. In particular, HCA highlights regions with a biased composition, coiled-coils, very short potential globular domains, and regions with potential for induced folding (for examples see refs. Citation69, Citation70, Citation76). Finally, it allows meaningful comparison with related protein sequences and enables a better definition of the boundaries of disordered regions. On the other hand, while HCA very useful for delineating regions devoid of regular secondary structure elements, it is poorly suited to recognize molten and pre-molten globules, i.e., proteins with a substantial amount of secondary structure but devoid of stable tertiary structure.
Paste the sequence (raw format) in the appropriate field using either the Mobyle portal or the MeDor metaserver.
When running HCA from the Mobyle portal, click on the “Run” button, and then type the text displayed in the window in the appropriate field to validate the submission.
The HCA plot is returned online and can be saved (pdf format).
Figure 3. HCA plot of human prothymosin α (UniProt ID: P06454). Hydrophobic amino acids (V, I, L, F, M, Y, W) are shown in green and are encircled and their contours are joined forming clusters. Clusters mainly correspond to regular secondary structures (α-helices and β-strands). The shape of the clusters is often typical of the associated secondary structures. Hence, horizontal and vertical clusters are mainly associated with α-helices and β-strands, respectively. A dictionary of hydrophobic clusters, gathering the main structural features of the most frequent hydrophobic clusters has been published helping the interpretation of HCA plots.Citation175 Sequence segments separating hydrophobic clusters (at least 4 non hydrophobic amino acids) mainly correspond to loops or linker (LNK) regions between globular domains. Long regions devoid of clusters correspond to disordered regions and small clusters within disordered regions correspond to putative MoRFs. Coiled-coil regions have a peculiar and easily recognizable appearance in the form of long horizontal clusters. Symbols are used to represent amino acids with peculiar structural properties (stars for prolines, black diamonds for glycines, squares and dotted squares for threonines and serines, respectively). Basic and acidic residues are shown in blue and red, respectively.

SLIDER (Super-fast predictor of proteins with Long Intrinsically DisordERed regions) predicts proteins with long disordered regions, defined as 30 or more consecutive disordered residues.Citation148 For each input protein, it provides propensity that quantifies likelihood that this protein includes a long disordered region. This method utilizes logistic regression that takes selected physicochemical properties of amino acids, sequence complexity, and amino acid composition as its inputs to generate prediction. SLIDER offers competitive predictive performance combined with low runtime. It was shown to outperform by at least a modest margin a comprehensive set of modern disorder predictors that can indirectly predict LDRs (prediction of disorder at the residue level can be used to find long disordered regions and this way the corresponding proteins can be identified). At the same time its runtime is at least 16 times lower which allows for applications on the whole genome scale using a desktop computer. An average sized proteome can be predicted in several minutes. This predictor is available as a web server at http://biomine.ece.ualberta.ca/SLIDER/.
Enter the protein sequence in fasta format (up to 75000 sequences can be entered at the same time) and provide the e-mail address in the corresponding field. Click on the “Run SLIDER” button.
Results can be accessed from a link shown on the page generated by the web server. An e-mail with a link to this page is also sent. Results are provided in the form of a list of numerical scores (one for each input protein) ranging between 0 and 1 that quantify propensity for inclusion of a long disordered region. They can be also downloaded in the csv format.
RAPID (Regression-based Accurate Predictor of Intrinsic Disorder) predicts an overall amount of disorder in a query protein sequence, defined as the fraction of disordered residues among all residues in that sequence.Citation149 It uses support vector regression to predict a numeric score in the 0 to 1 range that represents the fraction (content) of the disordered residues. This method is geared toward whole-genome analyses and correspondingly its key advantage is low runtime. Prediction of an average-size eukaryotic proteome takes less than one hour. A web server for this predictor can be found at http://biomine.ece.ualberta.ca/RAPID/.
Enter the protein sequence in fasta format (up to 75000 sequences can be input together) and provide the e-mail address in the corresponding field. Click on the “Run RAPID” button.
Results are available at a link shown on the page generated by the web server. An e-mail with these results and a link to the web page that stores these results is also sent. For each input protein the number of disordered residues and the corresponding fraction of disordered residues is provided. Moreover, these results can be downloaded in csv format.
Metapredictors
DisCoP (Disorder based on Consensus of Predictors) uses regression to combine predictions from 7 empirically selected to maximize predictive performance disorder predictors: ESpritz-Disprot, ESpritz-Xray, CSpritz-long, MD, SPINE-D, DISOPRED2, and DISOclust.Citation83 This method was optimized to generate a conservative and high-quality disorder predictions, i.e., predictions characterized by low false positive rate that corresponds to low rate of overprediction of disorder. Empirical evaluations on benchmark test data set has shown that this method improves predictive quality when compared with its input predictors and several other metapredictors that apply as many as twice the number of input disorder predictors. The web server that implements DisCoP is available at http://www.biomine.ece.ualberta.ca/disCoP/
Enter the protein sequence in fasta format and provide the e-mail address in the e-mail field. Click on the “Run DisCoP” button.
Results can be accessed from a link displayed on a webpage generated by the webserver. An e-mail is also sent giving a link to this page. The results are color-coded and provided as a binary prediction (disordered vs structured residue) and real-valued confidence (propensity for disorder). Disordered residues are marked by a red “D” character, structured by green “n” character, and the confidence values are reported below. The results can also be downloaded in csv format.
DisMeta (Disorder Prediction MetaServer, (http://www.wenmr.eu/wenmr/dismeta-disorder-prediction-metaserver) was developed within the WeNMR project framework (European FP7 e-Infrastructure grant, www.wenmr.eu). It runs several well-known disorder predictors, including DISOPRED2, DISEMBL, DRIPPRED, DISpro, FoldUnfold, FoldIndex, IUPred, GlobPlot2, PONDR® VSL2, and RONN. DisMeta also utilizes results provided by several sequence analysis tools, including ANCHOR, coils, TMHMM, SignalP, PROFphd, SEG, and PSIPRED. DisMeta presents the results as an HTML web page with a static graphical overview of each predictor result, and offers the user a consensus summarized as a graphic.
Enter the e-mail address, the protein name, paste the sequence (raw format) in the corresponding field, and click on the “Submit” button.
The system sends an email which includes links to the result page in html or in a raw text. The html version includes a consensus of disorder prediction as a graphics with the number of predictors predicting each position as disordered. The results of all disorder predictors in a box mapping representation are summarized at the bottom of the page.
GeneSilico MetaDisorder MD2 (http://iimcb.genesilico.pl/metadisorder/metadisorder.html) is a method based on 13 disorder predictors and gaps in alignment produced by 8-fold recognition methods, optimized using a genetic algorithm.Citation150 It is an improved version of the first MetaDisorder version released in 2008. It includes 15 distinct disorder predictors and weighs their output according to their individual prediction accuracy. These predictors are DISPROT (PONDR® VSL2), DisEMBL, DISpro, iPDA, GlobPlot, IUPred short (IUPRED-S), IUPred long (IUPRED-L), PDISORDER, PrDOS, POODLE-S, POODLE-L, Spritz short, Spritz long, DISOPRED, and RONN. Since these predictors include several metaservers, MetaDisorderMD2 is an extreme application of the concept that “the combination of different disorder predictors helps in refining the predictions.” In addition to the 15 disorder predictors, MetadisorderMD2 also uses fold recognition such as PSI-Blast (against PDB70 and CULLPDB databases), HHsearch, PCONS, and PHYRE. The end result of this method is a CASP-formatted output of each disorder included predictor and the corresponding alignments for the fold recognition methods, along with a consensus prediction of disorder in the same format. It also provides a plot that allows one to compare the consensus against any other disorder predictor result. MetaDisorder was among the best predictors of protein disorder evaluated during independent tests in CASP8 (2008) and CASP9 (2010).
Enter a title to the query, the e-mail address, paste the sequence (raw format) in the corresponding field, then click on the “Submit” button.
The results are displayed on an HTML page, but can also be viewed in raw text from a link available on the results page. An email is sent giving a link for the result page. On the graphical output, residues whose disorder probability is above 0.5 are considered as disordered.
MeDor (MEtaserver of DisORder) (http://www.vazymolo.org/MeDor/) is unique from other metapredictors in that (i) it provides an output in a specific format that can be annotated, saved, and further modified, and (ii) is not intended to provide a consensus of disorder prediction, and was designed to speed up the disorder prediction step by itself and provide a global overview of predictions.Citation82 It allows fast, simultaneous analysis of a query sequence by multiple predictors, and offers easy comparison of the prediction results. It also enables a standardized access to disorder predictors and allows meaningful comparisons among various query sequences, and provides a graphical interface with a unified view of the output of multiple disorder predictors. Furthermore, MeDor is also conceived to serve as a tool which allows the user to highlight specific regions of interest and retrieve their sequence. In addition, MeDor outputs can be saved, modified, and printed. Presently, the following programs are run by MeDor: a secondary structure prediction (SSP) based on the StrBioLib library of the Pred2ary program,Citation151 IUPred, HCA, FoldUnfold, RONN, FoldIndex, DisEMBL, DISPROT (PONDR® VSL2B, PONDR® VL3, and PONDR® VL3H), GlobPlot2, and Phobius. Phobius (http://phobius.sbc.su.se/index.html) predicts transmembrane regions. While SSP and HCA do not require a web connection, the other predictors are remotely launched through connection to the public web servers.
MeDor provides a graphical output, in which the sequence query and the results of the various predictors are featured horizontally, with a scroll bar allowing progression from the N-terminus to the C-terminus. All predictions are drawn along the sequence that is represented as a single, continuous horizontal line. MeDor also allows highlighting specific regions of interest and retrieving their sequence. Output files are in the specific (.med) format that is made of XML and can thus provide a graphical output for any program that return such a format. As XML is quite simple to access, it is also possible to edit the .“med” file manually to get a fully customized output that could even integrate additional predictions not initially provided. The (.med) file format can also be opened by any XML reader and the format is well described by the “xsd” file provided with the program. It is also possible to customize the output (highlight regions of interest, change colors, add and edit comments.) and to retrieve the predictor statistics values at each position, as well as the amino acid sequence of specific regions of interest.
Go to the MeDor home page (http://www.vazymolo.org/MeDor/)
Paste the sequence in either raw or fasta format and (optionally) enter the sequence name
Click on “Start MeDor”
Alternatively, MeDor can be downloaded (choose the version suitable to your operating system). Using the downloaded version of MeDor instead of the applet version enables the user to (i) run DISPROT (PONDR® VL3, PONDR® VL3H and PONDR® VSL2B) predictions (in the limit of 100 requests per IP number), (ii) print the results, (iii) save the output as an image, (iv) save (and load) files in the MeDor format, (v) access the comment panel, and (vi) import a sequence by providing the SwissProt accession number.
MetaPrDOS uses support vector machines on the prediction results of 7 independent predictors (DISOPRED, PrDOS, DISPROT (PONDR® VSL2P), DisEMBL, IUPred DISpro, and POODLE-S).Citation81 This method attained a higher prediction accuracy than all methods participating in CASP7 (2006).Citation138
Paste the sequence in raw format, enter the sequence name and the e-mail address, then click on “Predict.”
A new page appears where the user is asked to confirm the submission by clicking the OK button.
The link for the results page is sent by email. On the results page, the plot can be saved as an image (png format) by clicking on it with the right mouse button. Residues with disorder probabilities higher than 0.5 are considered as disordered. Above the graph, the amino acid sequence is shown and disordered residues are shown in red. Disorder probabilities per residue can be obtained by clicking on the download button (below the graph), which yields an output in the casp or csv format.
MFDp (Multilayered Fusion-based Disorder predictor) is a metapredictor that consists of 3 support vector machines specialized for the prediction of short, long and all disordered regions. It combines these results with multiple complementary disorder predictors, namely DISOPRED, DISOclust, IUPRED–S, and IUPRED-L. In addition, MFDp also utilizes solvent accessibility, secondary structure predictions, B-factors, and backbone dihedral torsion angles in order to generate its consensus.Citation152 This predictor secured the top-3 finish at the CASP10. The web server can be found at http://biomine.ece.ualberta.ca/MFDp/
3. Enter the protein sequence in fasta format and provide the e-mail address in the corresponding field. Tick the predictors used by the metapredictor for which you'd like to see the results in the output in addition to the MFDp prediction, and then click on the “start” button.
4. Results can be accessed from a link displayed on the MFDp processing page. An e-mail is also sent giving a link for the result page. Results are provided in the form of an alignment of the different predictor results and the consensus prediction built by MFDp. Disordered residues are marked by a red “D” character and the confidence values are reported below. In addition, results can also be downloaded in csv format.
MFDp2 (http://biomine.ece.ualberta.ca/MFDp2/) combines per-residue disorder probabilities predicted by MFDp with per-sequence disorder content predicted by DisCon method,Citation153 and applies post-processing filters to provide disorder predictions.Citation154
Enter the protein sequence in fasta format and provide the e-mail address in the corresponding field.
The output shows optimized per-residue disorder probability profiles, per-sequence disorder content, list (with analysis) of disordered segments, and several profiles that help in the interpretation of the results. The results are available online in a graphical format and can be also downloaded in a text-based (parsable) format.
MULTICOM is a simple averaging approach that is different from other meta methods utilizing consensus voting.Citation139 MULTICOM makes predictions based on a consensus formed from other CASP8 disorder predictors including the PreDisorder predictor that is the authors' developed ab initio method. It also includes most of the predictors that participated in CASP8 and it works by averaging their output. It was ranked among the top disorder predictors in CASP8.Citation140 The server can be reached from http://sysbio.rnet.missouri.edu/multicom_cluster/ and returns results by e-mail in a CASP format.
Enter a target name, the protein sequence in raw format, and provide the email address in the corresponding field. Then click on the “Predict” button.
Open the result e-mail that contains model evaluation, model combination, and model refinement data in the CASP / PDB format.
PONDR-FIT uses a consensus artificial neural network (ANN) prediction method that combines PONDR® VSL2, PONDR® VLXT, FoldIndex, PONDR® VL3, TopIDP, andIUPred.Citation155 It was made available in 2010 and the predictor can be run online for academic use only, from http://www.disprot.org/pondr-fit.php.
Enter the sequence file in fasta (or EMBL) format and then click on the “Submit” button.
The server returns a graphical plot of disorder probabilities for each amino acid position, along with a raw output file of the results.
PredictProtein (www.predictprotein.org) is a server based on a system of neural networks that combines the outputs from several original prediction methods, using evolutionary profiles and sequence features that correlate with protein disorder such as protein flexibility and predicted solvent accessibility. In addition to providing predictions of trans-membrane regions, secondary structure, and disulphide bridges, the server also returns predictions of disorder. In particular, the UCON, NORSnet, and MetaDisorder (MD) programs can be run from the PredictProtein server.
MD (Meta Disorder)Citation156 runs a panel of 4 predictors, namely PROFbval,Citation157 DISOPRED2, Ucon, and NORSnet. Once it receives results from these predictors it calculates the arithmetic average over the 4 raw outputs. The results of MD that are included within the PredictProtein output come in a raw format providing the computed probability for the MD consensus associated with each distinct disorder predictor results. Like Ucon and NORSp, MD can be also downloaded as a Debian package from http://rostlab.org/debian/pool/non-free/m/metadisorder/.
NORSnet is a neural-network-based method for the identification of unstructured loops.Citation158 NORSnet was trained to distinguish between very long contiguous segments with non-regular secondary structure (NORS regions) and well-folded proteins. Since NORSnet was trained on predicted information rather than on experimental data, it was optimized on a large data set, thus overcoming the biases related to the small size of experimental data sets. NORSnet covers regions in sequence space that are not covered by other disorder predictors. The program is also provided as a Debian package that can be found at https://rostlab.org/owiki/index.php/Norsnet.
Ucon (http://www.predictprotein.org/submit_ucon.html) is a method that combines predictions for protein-specific contacts with a generic pairwise potential. This predictor was trained using the annotations of disorder from DisProt and PDB. It performs well in predicting proteins with long disordered regions.Citation159 Ucon can also be downloaded as a Debian package from https://rostlab.org/owiki/index.php/Ucon.
From the PredictProtein page:
Enter the amino acid sequence (raw data) and click on the “PredictProtein” button.
Either enter the e-mail address without creating an account (in which case you will run Open PredictProtein) or create an account that will allow you subsequently to login with a password. Note that Open PredictProtein does not store jobs.
Upon completion of prediction, the user is sent an e-mail with a link to the result page. Boundaries of NORS regions are indicated above the annotated sequence in which solvent exposure, secondary structure elements, coils and trans-membrane regions are also indicated. On the left side of the result page, different layout options can be chosen. Clicking on “Protein Disorder and Flexibility” will give access to prediction results as provided by Profbval, Ucon, NORSnet and MD in the form of colored boxes. Mouse over the different colored boxes to learn more about the annotations.
Combining predictors and experimental data
An extreme extension of the combined use of different predictors is combining in silico and experimental approaches maximize the mount of discovered structural information while limiting the experimental characterization to relatively low-demanding experiments. An illustration of such an approach can be found in ref. Citation160 where a computational and spectroscopic analyses were combined. The authors plotted the ratio between the Θ222 and Θ200 (Θ222/Θ200) of a set of IDPs under study, along with the Θ222/Θ200 ratio of a set of well-characterized random coil-like and pre-molten globule-like proteins.Citation161 The authors then set an arbitrary threshold of the Θ222/Θ200 ratio that allows discrimination between random coil-like IDPs and IDPs adopting a pre-molten-like conformation. Then, they generated a plot in which the distance of each IDP under study from this threshold was plotted as a function of its CH-distance in the CH plot. This analysis was intended to combine, and hence extend, the 2 methods previously introduced by UverskyCitation18,Citation161 to allow random coil-like forms to be readily and easily distinguished from pre-molten globule-like forms among proteins predicted to be intrinsically disordered by the hydropathy/charge method. In the resulting plot, increasingly negative CH distances indicate proteins with increasing disorder, while increasingly positive Θ222/Θ200 distances indicate IDPs becoming progressively more collapsed due to an increased content in regular secondary structure. Thus, the bottom left quadrant is expected to correspond to IDPs adopting a random coil-like conformation, while the bottom right quadrant is meant to designate IDPs adopting a pre-molten globule-like conformation.
Identifying regions of induced folding
IDPs bind to their target(s) through interaction-prone short segments that become ordered after binding to partner(s). These regions are referred to as “Molecular Recognition Elements” (MoREs) or “Molecular Recognition Features” (MoRFs)Citation86,Citation88,Citation162 or “Intrinsically Disordered Binding” (IDB) sites.Citation163
Even before predictors designed specifically for identifying these regions were publicly available, they could be successfully discovered using tools that were originally designed for other purposes, such as PONDR® VLXT and HCA. Due to its high sensitivity to local sequence peculiarities, PONDR® VLXT was discovered to be able to identify disorder-based interaction sitesCitation86 (for examples see refs. Citation164, Citation165). HCA is similarly instrumental for the identification of regions undergoing induced folding, as buried hydrophobic residues at the protein-partner interface are often the major driving force in protein folding.Citation163,Citation166 In some cases, hydrophobic clusters are found within secondary structure elements that are unstable in the native protein, but can stably fold upon binding to a partner. Therefore, HCA can be very useful to highlight potential induced folding regions (for examples see refs. Citation124, Citation160, Citation167).
Perform HCA on the query sequence using either the Mobyle portal or the MeDor metaserver, and look for short hydrophobic clusters occurring within disordered regions.
Perform prediction using PONDR® VLXT and look for sharp (and short) drops in the middle of disorder predictions.
More recently, a few specific predictors aimed at identifying disorder-based regions have become publicly available. A short description of their architectures and details on how to run them are provided below.
ANCHOR (http://anchor.enzim.hu/) is aimed at identifying segments that reside in disordered regions which cannot form enough favorable intrachain interactions to fold on their own, and are likely to gain stabilizing energy by interacting with a globular protein partner. The reasoning used to develop ANCHOR relies on the pairwise energy estimation approach developed for IUPred.Citation93
Enter the SWISS-PROT/TrEMBL ID or accession number of the query sequence, or paste the sequence in fasta or raw format. Optionally, ELM and other motifs can also be searched for by entering the motif names in proper format in the appropriate field.
Click on “Submit.”
Results are returned online in the form of a plot that contains the per residue IUPred and ANCHOR probabilities as a function of residue positions. Predicted binding regions are shown as blue boxes along the sequence below the plots. The plot (see ) can be saved (png format) by clicking on it with the right mouse button. The output also provides a summary of the predicted binding sites (in the form of a Table) along with a Table with position specific scores.
Figure 4. Prediction of potential disorder-based interaction sites human prothymosin α (UniProt ID: P06454) by ANCHOR. The plot provides the distribution of disorder propensity (evaluated by IUPred, red line) and distribution of ANCHOR scores (blue line). In IUPred plot, residues/regions with scores >0.5 are predicted to be disordered. In ANCHOR plot, residues/regions with scores >0.5 are predicted to correspond to the potential disorder-based binding sites. Bottom of plot represents binding regions as bars with different shades of blue, with darker color corresponding to higher ANCHOR scores. This bottom graph shows regions possessing ANCHOR scores >0.5.
MoRFpred (http://biomine.ece.ualberta.ca/MoRFpred/) identifies all main types of MoRF that include α, β, coil and complex (defined based on their secondary structure conformation upon binding).Citation89 It combines annotations generated by sequence alignment with predictions obtained via a support vector machine. The latter utilizes a custom designed set of sequence-derived features that quantify information regarding selected physiochemical properties of amino acids, evolutionary profiles, solvent accessibility, predicted disorder, and B-factors. Empirical evaluation on several data sets shows that MoRFpred outperforms both α-MoRF-Pred (which predicts α-MoRFs)Citation88 and ANCHOR.
Paste the sequence in FASTA format, provide the e-mail address (required), and click on “Run MoRFpred.”
Results are returned online by clicking on a link to the results page (an e-mail is also sent as soon as results are available), and can also be downloaded in csv format. The first line displays the query sequence, while the second and third lines show the predictions. The second row annotates Molecular Recognition Feature (MoRF) (marked as “M”, in red) and non-MoRF (marked as “n”, in green) residues, and the third row gives prediction scores (the higher the score the more likely it is that a given residue is MoRF). A horizontal scroll bar allows moving along the sequence.
Predicting potential sites of posttranslational modifications
It has been shown that intrinsic disorder prediction might help increase the prediction accuracy of several protein post-translational modification (PTM) sites, including protein methylation,Citation168 phosphorylation,Citation98 and many other mostly enzymatically catalyzed PTMs.
DisPhos is a tool for finding phosphorylation sites. DEPP (or DisPhos) uses disorder information to improve the discrimination between phosphorylation and non-phosphorylation sites. The retrieved prediction score approximates the probability that the residue is phosphorylated. Only residues with a prediction score >0.5 (which) are considered to be phosphorylated.
1) Go to the PONDR® working page (http://www.pondr.com/) and click the DEPP Prediction button. This redirects to the DEPP working page (http://www.pondr.com/cgi-bin/depp.cgi). While on this page, type Protein name in the space provided (optional) and enter NCBI Accession Code or Protein Sequence (FASTA format or sequence only) in the corresponding boxes. Scroll down the page and check the box Raw Output at the Output Options section. Click the Submit Query button to be taken to the DEPP results page.
2) The top of DEPP results page displays a plot which provides the distribution of DEPP scores over the amino acid sequence. There are 3 types of symbols corresponding to the Ser (blue squares), Thr (green triangles), and Tyr residues (red circles) predicted to be phosphorylated. Only residues with DEPP scores >0.5 will be shown.
3) Raw data related to this analysis are provided at the end of the page in the PREDICTOR VALUES section. The DEPP NNP STATISTICS section provides useful information on the number of phosphorylated threonines, serines, and tyrosines, as well as the total number of these residues in a given protein and the relative phosphorylation efficiency. It is suggested to keep the content of the entire DEPP results page for the future use.
ModPred is a unified sequence-based predictor of 23 types of PTM sites.Citation99 This tool represents a very useful instrument for guiding biological experiments and data interpretation.Citation99
1) Go to the official ModPred page (www.modpred.org). Enter the query protein sequence in the Paste the protein sequence box, one at a time. Click the Check all link, and then the Predict link. When calculations are finished, the result page will be displayed.
2) The results page has an INPUT section that provides sequence ID of the query protein, its length, and lists the predicted PTMs. The OUTPUT section provides prediction results, where sequence is color coded to show residues predicted to be modified with low confidence (red), medium confidence (yellow), and high confidence (green), as well as residues corresponding to multiple PTM sites (blue). A table that lists all prediction results is included below, which can be downloaded as a tab-delimited file.
General procedure for disorder prediction
As the performance of predictors is dependent on both the type of disorder they predict and on the type of disorder against which they were trained, multiple prediction methods need to be combined to improve the accuracy and specificity of disorder predictions. illustrates a general sequence analysis procedure that integrates the peculiarities of each method to predict disordered regions.
Retrieve the amino acid sequence and the description file of the protein of interest by entering the protein name at the UniProt (http://www.uniprot.org) in the “Search” field.
Generate a multiple sequence alignment. A set of related sequences can be obtained by running HHblits (http://toolkit.tuebingen.mpg.de/hhblits). Click on the “get selected sequences” option and save them to a file in fasta format. Use this file as input for building up a multiple sequence alignment using TCoffee (http://tcoffee.crg.cat/apps/tcoffee/do:regular). Mark variable regions as likely corresponding to flexible linkers or long disordered regions. Use DFLpred method if you want to ascertain disordered linkers.
Search for long (>50 residues) regions devoid of predicted secondary structure using the PSIPRED (http://bioinf.cs.ucl.ac.uk/psipred/psiform.html)Citation169 and PredictProtein (http://www.predictprotein.org/) servers.
Using either the UniProt ID or the amino acid sequence, search the D2P2 and MobiDB databases. Since D2P2 does not cover all organisms, and MobiDB does not include IDEAL entries, it is also recommended to search the IDEAL database.
In the event that no information about disordered regions can be obtained, or the information is incomplete, the following steps should be performed.
Perform an analysis of sequence composition using the ProtParam ExPASy server (http://www.expasy.ch/tools/protparam.html) and compare the results with the average sequence composition of proteins within the UniProtKB/Swiss-Prot database (http://www.expasy.ch/sprot/relnotes/relstat.html).
Perform an analysis of sequence complexity using the SEG program.Citation170 Although the SEG program is implemented in many protein prediction servers (such as PredictProtein for instance), the program can also be downloaded from ftp://ftp.ncbi.nih.gov/pub/seg/seg, while simplified versions with default settings can be run at either http://mendel.imp.univie.ac.at/METHODS/seg.server.html or http://www.ncbi.nlm.nih.gov/BLAST or http://mendel.imp.ac.at/METHODS/seg.server.html. The stringency of the search for low-complexity segments is determined by 3 user-defined parameters: trigger window length [W], trigger complexity [K(1)] and extension complexity [K(2)]. Typical parameters for disorder prediction of long non-globular domains are [W] = 45, [K(1)] = 3.4 and [K(2)] = 3.75, while for short non-globular domains they are [W] = 25, [K(1)] = 3.0 and [K(2)] = 3.3. It is worth noting that low complexity regions can also be found in ordered proteins, such as coiled-coils and other non-globular proteins like collagen.
Search for (i) signal peptides and transmembrane regions using the Phobius server (http://phobius.sbc.su.se/index.html),Citation171 (ii) leucine zippers using the 2ZIP server (http://2zip.molgen.mpg.de/),Citation172 and (iii) coiled-coils using programs such as Coils (http://www.ch.embnet.org/software/COILS_form.html).Citation173 Note that the identification of coiled-coils is vital as they can lead to miss-predictions of disorder (for examples see refs.Citation69,Citation76). It is also recommended to use DIpro (http://contact.ics.uci.edu/bridge.html)Citation174 to identify possible disulfide bridges and to search for possible metal-binding regions by looking for conserved Cys3-His or Cys2-His2 motifs in multiple sequence alignments. The presence of conserved cysteines and/or of metal-binding motifs prevents meaningful local predictions of disorder within these regions, since they may display features typifying disorder while gaining structure upon disulfide formation or upon binding to metal ions.Citation18
Run HCA to highlight regions devoid of hydrophobic clusters and with obvious sequence bias composition.
Run disorder predictions and identify a consensus of disorder. Since running multiple prediction methods is a time-consuming procedure, and since combining several predictors often allows achieving accuracies higher than those of each of the component predictors, it is recommended to perform predictions using metapredictors. It is recommended to use the default parameters of each metapredictor, as they generally perform the best in terms of accuracy, specificity, and sensitivity. Once a gross domain architecture for the protein of interest is established, the case of domains whose structural state is uncertain can be settled using the charge/hydropathy method, which has a quite low error rate. As a last step, boundaries between ordered and disordered regions can be refined using HCA, and regions with a propensity to undergo induced folding can be identified using ANCHOR and MoRFpred.
We recommend using SLIDER and RAPID methods for the whole genome analysis of species that are not included in the D2P2 database.
Disclosure of potential conflicts of interest
No potential conflicts of interest were disclosed.
References
- Dunker AK, Babu MM, Barbar E, Blackledge M, Bondos SE, Dosztányi Z, Dyson HJ, Forman-Kay J, Fuxreiter M, Gsponer J, et al. What's in a name? Why these proteins are intrinsically disordered. Intrinsically Disordered Proteins 2013; 1:e24157; http://dx.doi.org/10.4161/idp.24157
- Dunker AK, Obradovic Z, Romero P, Garner EC, Brown CJ. Intrinsic protein disorder in complete genomes. Genome Inform Ser Workshop Genome Inform 2000; 11:161-71; PMID:11700597
- Uversky VN. The mysterious unfoldome: structureless, underappreciated, yet vital part of any given proteome. J Biomed Biotechnol 2010; 2010:568068; PMID:20011072; http://dx.doi.org/10.1155/2010/568068
- Ward JJ, Sodhi JS, McGuffin LJ, Buxton BF, Jones DT. Prediction and functional analysis of native disorder in proteins from the three kingdoms of life. J Mol Biol 2004; 337:635-45; PMID:15019783; http://dx.doi.org/10.1016/j.jmb.2004.02.002
- Dunker AK, Lawson JD, Brown CJ, Williams RM, Romero P, Oh JS, Oldfield CJ, Campen AM, Ratliff CM, Hipps KW, et al. Intrinsically disordered protein. J Mol Graph Model 2001; 19:26-59; PMID:11381529; http://dx.doi.org/10.1016/S1093-3263(00)00138-8
- Uversky VN, Dunker AK. Understanding protein non-folding. Biochim Biophys Acta 2010; 1804:1231-64; PMID:20117254; http://dx.doi.org/10.1016/j.bbapap.2010.01.017
- Xue B, Dunker AK, Uversky VN. Orderly order in protein intrinsic disorder distribution: disorder in 3500 proteomes from viruses and the three domains of life. J Biomol Struct Dyn 2012; 30:137-49; PMID:22702725; http://dx.doi.org/10.1080/07391102.2012.675145
- Peng Z, Yan J, Fan X, Mizianty MJ, Xue B, Wang K, Hu G, Uversky VN, Kurgan L. Exceptionally abundant exceptions: comprehensive characterization of intrinsic disorder in all domains of life. Cell Mol Life Sci 2015; 72:137-51; PMID:24939692; http://dx.doi.org/10.1007/s00018-014-1661-9
- Bogatyreva NS, Finkelstein AV, Galzitskaya OV. Trend of amino acid composition of proteins of different taxa. J Bioinform Comput Biol 2006; 4:597-608; PMID:16819805; http://dx.doi.org/10.1142/S0219720006002016
- Obradovic Z, Peng K, Vucetic S, Radivojac P, Brown CJ, Dunker AK. Predicting intrinsic disorder from amino acid sequence. Proteins 2003; 53:566-72; PMID:14579347; http://dx.doi.org/10.1002/prot.10532
- Piovesan D, Tabaro F, Micetic I, Necci M, Quaglia F, Oldfield CJ, Aspromonte MC, Davey N, Davidovic R, Dosztanyi Z, et al. DisProt 7.0: A major update of the database of disordered proteins. Nucleic Acids Res; PMID:27899601; http://dx.doi.org/10.1093/nar/gkw1056
- Monastyrskyy B, Kryshtafovych A, Moult J, Tramontano A, Fidelis K. Assessment of protein disorder region predictions in CASP10. Proteins 2014; 82 Suppl 2:127-37; PMID:23946100; http://dx.doi.org/10.1002/prot.24391
- Monastyrskyy B, Fidelis K, Moult J, Tramontano A, Kryshtafovych A. Evaluation of disorder predictions in CASP9. Proteins 2011; 79 Suppl 10:107-18; PMID:21928402; http://dx.doi.org/10.1002/prot.23161
- Fischer E. Einfluss der configuration auf die wirkung der enzyme. Ber Dt Chem Ges 1894; 27:2985-93; http://dx.doi.org/10.1002/cber.18940270364
- Perdigao N, Heinrich J, Stolte C, Sabir KS, Buckley MJ, Tabor B, Signal B, Gloss BS, Hammang CJ, Rost B, et al. Unexpected features of the dark proteome. Proc Natl Acad Sci U S A 2015; 112:15898-903; PMID:26578815; http://dx.doi.org/10.1073/pnas.1508380112
- Dunker AK, Garner E, Guilliot S, Romero P, Albrecht K, Hart J, Obradovic Z, Kissinger C, Villafranca JE. Protein disorder and the evolution of molecular recognition: theory, predictions and observations. Pac Symp Biocomput 1998:473-84; PMID:9697205
- Wright PE, Dyson HJ. Intrinsically unstructured proteins: re-assessing the protein structure-function paradigm. J Mol Biol 1999; 293:321-31; PMID:10550212; http://dx.doi.org/10.1006/jmbi.1999.3110
- Uversky VN, Gillespie JR, Fink AL. Why are “natively unfolded” proteins unstructured under physiologic conditions? Proteins 2000; 41:415-27; PMID:11025552; http://dx.doi.org/10.1002/1097-0134(20001115)41:3%3c415::AID-PROT130%3e3.0.CO;2-7
- Tompa P. Intrinsically unstructured proteins. Trends Biochem Sci 2002; 27:527-33; PMID:12368089; http://dx.doi.org/10.1016/S0968-0004(02)02169-2
- Daughdrill GW, Pielak GJ, Uversky VN, Cortese MS, Dunker AK. Natively disordered proteins. In: Buchner J, Kiefhaber T, eds. Handbook of Protein Folding. Weinheim, Germany: Wiley-VCH, Verlag GmbH & Co. KGaA, 2005, 271-353.
- Uversky VN. Unusual biophysics of intrinsically disordered proteins. Biochim Biophys Acta 2013; 1834:932-51; PMID:23269364; http://dx.doi.org/10.1016/j.bbapap.2012.12.008
- Uversky VN. Protein folding revisited. A polypeptide chain at the folding-misfolding-nonfolding cross-roads: which way to go? Cell Mol Life Sci 2003; 60:1852-71; PMID:14523548; http://dx.doi.org/10.1007/s00018-003-3096-6
- Zhang T, Faraggi E, Li Z, Zhou Y. Intrinsically semi-disordered state and its role in induced folding and protein aggregation. Cell Biochem Biophys 2013; 67:1193-205; PMID:23723000; http://dx.doi.org/10.1007/s12013-013-9638-0
- Uversky VN. A decade and a half of protein intrinsic disorder: biology still waits for physics. Protein Sci 2013; 22:693-724; PMID:23553817; http://dx.doi.org/10.1002/pro.2261
- Uversky VN. Natively unfolded proteins: a point where biology waits for physics. Protein Sci 2002; 11:739-56; PMID:11910019; http://dx.doi.org/10.1110/ps.4210102
- Bracken C, Iakoucheva LM, Romero PR, Dunker AK. Combining prediction, computation and experiment for the characterization of protein disorder. Curr Opin Struct Biol 2004; 14:570-6; PMID:15465317; http://dx.doi.org/10.1016/j.sbi.2004.08.003
- Receveur-Brechot V, Bourhis JM, Uversky VN, Canard B, Longhi S. Assessing protein disorder and induced folding. Proteins 2006; 62:24-45; PMID:16287116; http://dx.doi.org/10.1002/prot.20750
- Uversky VN, Dunker AK. Multiparametric analysis of intrinsically disordered proteins: looking at intrinsic disorder through compound eyes. Anal Chem 2012; 84:2096-104; PMID:22242801; http://dx.doi.org/10.1021/ac203096k
- Uversky VN. Biophysical Methods to Investigate Intrinsically Disordered Proteins: Avoiding an “Elephant and Blind Men” Situation. Adv Exp Med Biol 2015; 870:215-60; PMID:26387104; http://dx.doi.org/10.1007/978-3-319-20164-1_7
- Dyson HJ, Wright PE. Insights into the structure and dynamics of unfolded proteins from nuclear magnetic resonance. Adv Protein Chem 2002; 62:311-40; PMID:12418108; http://dx.doi.org/10.1016/S0065-3233(02)62012-1
- Dyson HJ, Wright PE. Unfolded proteins and protein folding studied by NMR. Chem Rev 2004; 104:3607-22; PMID:15303830; http://dx.doi.org/10.1021/cr030403s
- Dyson HJ, Wright PE. Elucidation of the protein folding landscape by NMR. Methods Enzymol 2005; 394:299-321; PMID:15808225; http://dx.doi.org/10.1016/S0076-6879(05)94011-1
- Ringe D, Petsko GA. Study of protein dynamics by X-ray diffraction. Methods Enzymol 1986; 131:389-433; PMID:3773767; http://dx.doi.org/10.1016/0076-6879(86)31050-4
- Adler AJ, Greenfield NJ, Fasman GD. Circular dichroism and optical rotatory dispersion of proteins and polypeptides. Methods Enzymol 1973; 27:675-735; PMID:4797940; http://dx.doi.org/10.1016/S0076-6879(73)27030-1
- Fasman GD. Circular dichroism and the conformational analysis of biomolecules. New York: Plenem Press, 1996.
- Provencher SW, Glockner J. Estimation of globular protein secondary structure from circular dichroism. Biochemistry 1981; 20:33-7; PMID:7470476; http://dx.doi.org/10.1021/bi00504a006
- Woody RW. Circular dichroism. Methods Enzymol 1995; 246:34-71; PMID:7538625; http://dx.doi.org/10.1016/0076-6879(95)46006-3
- Smyth E, Syme CD, Blanch EW, Hecht L, Vasak M, Barron LD. Solution structure of native proteins with irregular folds from Raman optical activity. Biopolymers 2001; 58:138-51; PMID:11093113; http://dx.doi.org/10.1002/1097-0282(200102)58:2%3c138::AID-BIP30%3e3.0.CO;2-W
- Glatter O, Kratky O. Small angle X-ray scattering. London: Academic Press, 1982.
- Uversky VN. A multiparametric approach to studies of self-organization of globular proteins. Biochemistry (Mosc) 1999; 64:250-66; PMID:10205295
- Iakoucheva LM, Kimzey AL, Masselon CD, Smith RD, Dunker AK, Ackerman EJ. Aberrant mobility phenomena of the DNA repair protein XPA. Protein Sci 2001; 10:1353-62; PMID:11420437; http://dx.doi.org/10.1110/ps.40101
- Tompa P. Intrinsically unstructured proteins. Trends Biochem Sci 2002; 27; PMID:11796221
- Markus G. Protein substrate conformation and proteolysis. Proc Natl Acad Sci U S A 1965; 54:253-8; PMID:5216360; http://dx.doi.org/10.1073/pnas.54.1.253
- Mikhalyi E. Application of proteolytic enzymes to protein structure studies. Boca Raton: CRC Press, 1978.
- Hubbard SJ, Eisenmenger F, Thornton JM. Modeling studies of the change in conformation required for cleavage of limited proteolytic sites. Protein Sci 1994; 3:757-68; PMID:7520312; http://dx.doi.org/10.1002/pro.5560030505
- Fontana A, de Laureto PP, de Filippis V, Scaramella E, Zambonin M. Probing the partly folded states of proteins by limited proteolysis. Fold Des 1997; 2:R17-R26; PMID:9135978; http://dx.doi.org/10.1016/S1359-0278(97)00010-2
- Fontana A, de Laureto PP, Spolaore B, Frare E, Picotti P, Zambonin M. Probing protein structure by limited proteolysis. Acta Biochim Pol 2004; 51:299-321; PMID:15218531
- Park C, Marqusee S. Pulse proteolysis: a simple method for quantitative determination of protein stability and ligand binding. Nat Methods 2005; 2:207-12; PMID:15782190; http://dx.doi.org/10.1038/nmeth740
- Feng Y, De Franceschi G, Kahraman A, Soste M, Melnik A, Boersema PJ, de Laureto PP, Nikolaev Y, Oliveira AP, Picotti P. Global analysis of protein structural changes in complex proteomes. Nat Biotechnol 2014; 32:1036-44; PMID:25218519; http://dx.doi.org/10.1038/nbt.2999
- Minde DP, Maurice MM, Rudiger SG. Determining biophysical protein stability in lysates by a fast proteolysis assay, FASTpp. PLoS One 2012; 7:e46147; PMID:23056252; http://dx.doi.org/10.1371/journal.pone.0046147
- Privalov PL. Stability of proteins: small globular proteins. Adv Protein Chem 1979; 33:167-241; PMID:44431; http://dx.doi.org/10.1016/S0065-3233(08)60460-X
- Ptitsyn O. Molten globule and protein folding. Adv Protein Chem 1995; 47:83-229; PMID:8561052; http://dx.doi.org/10.1016/S0065-3233(08)60546-X
- Ptitsyn OB, Uversky VN. The molten globule is a third thermodynamical state of protein molecules. FEBS Lett 1994; 341:15-8; PMID:8137915; http://dx.doi.org/10.1016/0014-5793(94)80231-9
- Uversky VN, Ptitsyn OB. All-or-none solvent-induced transitions between native, molten globule and unfolded states in globular proteins. Fold Des 1996; 1:117-22; PMID:9079371; http://dx.doi.org/10.1016/S1359-0278(96)00020-X
- Westhof E, Altschuh D, Moras D, Bloomer AC, Mondragon A, Klug A, Van Regenmortel MH. Correlation between segmental mobility and the location of antigenic determinants in proteins. Nature 1984; 311:123-6; PMID:6206398; http://dx.doi.org/10.1038/311123a0
- Berzofsky JA. Intrinsic and extrinsic factors in protein antigenic structure. Science 1985; 229:932-40; PMID:2410982; http://dx.doi.org/10.1126/science.2410982
- Miyagi A, Tsunaka Y, Uchihashi T, Mayanagi K, Hirose S, Morikawa K, Ando T. Visualization of intrinsically disordered regions of proteins by high-speed atomic force microscopy. Chemphyschem 2008; 9:1859-66; PMID:18698566; http://dx.doi.org/10.1002/cphc.200800210
- Ishino S, Yamagami T, Kitamura M, Kodera N, Mori T, Sugiyama S, Ando T, Goda N, Tenno T, Hiroaki H, et al. Multiple interactions of the intrinsically disordered region between the helicase and nuclease domains of the archaeal Hef protein. J Biol Chem 2014; 289:21627-39; PMID:24947516; http://dx.doi.org/10.1074/jbc.M114.554998
- Oroz J, Hervas R, Valbuena A, Carrion-Vazquez M. Unequivocal single-molecule force spectroscopy of intrinsically disordered proteins. Methods Mol Biol 2012; 896:71-87; PMID:22821518
- Solanki A, Neupane K, Woodside MT. Single-molecule force spectroscopy of rapidly fluctuating, marginally stable structures in the intrinsically disordered protein alpha-synuclein. Phys Rev Lett 2014; 112:158103; PMID:24785077; http://dx.doi.org/10.1103/PhysRevLett.112.158103
- Neupane K, Solanki A, Sosova I, Belov M, Woodside MT. Diverse metastable structures formed by small oligomers of alpha-synuclein probed by force spectroscopy. PLoS ONE 2014; 9:e86495; PMID:24475132; http://dx.doi.org/10.1371/journal.pone.0086495
- Elmlund D, Elmlund H. Cryogenic electron microscopy and single-particle analysis. Annu Rev Biochem 2015; 84:499-517; PMID:25747402; http://dx.doi.org/10.1146/annurev-biochem-060614-034226
- Keller PW, Huang RK, England MR, Waki K, Cheng N, Heymann JB, Craven RC, Freed EO, Steven AC. A two-pronged structural analysis of retroviral maturation indicates that core formation proceeds by a disassembly-reassembly pathway rather than a displacive transition. J Virol 2013; 87:13655-64; PMID:24109217; http://dx.doi.org/10.1128/JVI.01408-13
- Wu W, Leavitt JC, Cheng N, Gilcrease EB, Motwani T, Teschke CM, Casjens SR, Steven AC. Localization of the Houdinisome (Ejection Proteins) inside the Bacteriophage P22 Virion by Bubblegram Imaging. MBio 2016; 7:e01152-16; PMID:27507825; http://dx.doi.org/10.1128/mBio.01152-16
- Uversky VN. The multifaceted roles of intrinsic disorder in protein complexes. FEBS letters 2015; 589:2498-2506; PMID:26073257; http://dx.doi.org/10.1016/j.febslet.2015.06.004
- Haynes C, Oldfield CJ, Ji F, Klitgord N, Cusick ME, Radivojac P, Uversky VN, Vidal M, Iakoucheva LM. Intrinsic disorder is a common feature of hub proteins from four eukaryotic interactomes. PLoS Comput Biol 2006; 2:e100; PMID:16884331; http://dx.doi.org/10.1371/journal.pcbi.0020100
- Habchi J, Tompa P, Longhi S, Uversky VN. Introducing Protein Intrinsic Disorder. Chem Rev 2014; 114:6561-88; PMID:24739139; http://dx.doi.org/10.1021/cr400514h
- Lobley A, Swindells MB, Orengo CA, Jones DT. Inferring function using patterns of native disorder in proteins. PLoS Comput Biol 2007; 3:e162; PMID:17722973; http://dx.doi.org/10.1371/journal.pcbi.0030162
- Ferron F, Longhi S, Canard B, Karlin D. A practical overview of protein disorder prediction methods. Proteins 2006; 65:1-14; PMID:16856179; http://dx.doi.org/10.1002/prot.21075
- Ferron F, Rancurel C, Longhi S, Cambillau C, Henrissat B, Canard B. VaZyMolO: a tool to define and classify modularity in viral proteins. J Gen Virol 2005; 86:743-9; PMID:15722535; http://dx.doi.org/10.1099/vir.0.80590-0
- Lieutaud P, Ferron F, Habchi J, Canard B, Longhi S. Predicting protein disorder and induced folding : a practical approach. In: Dunn B, ed. Advances in Protein and Peptide Sciences. Bentham Science Publishers, 2013:441-92 (52).
- Atkins JD, Boateng SY, Sorensen T, McGuffin LJ. Disorder Prediction Methods, Their Applicability to Different Protein Targets and Their Usefulness for Guiding Experimental Studies. Int J Mol Sci 2015; 16:19040-54; PMID:26287166; http://dx.doi.org/10.3390/ijms160819040
- Mizianty MJ, Kurgan L. Sequence-based prediction of protein crystallization, purification and production propensity. Bioinformatics 2011; 27:i24-33; PMID:21685077; http://dx.doi.org/10.1093/bioinformatics/btr229
- Mizianty MJ, Kurgan LA. CRYSpred: accurate sequence-based protein crystallization propensity prediction using sequence-derived structural characteristics. Protein Peptide Lett 2012; 19:40-9; http://dx.doi.org/10.2174/092986612798472910
- Wang H, Feng L, Zhang Z, Webb GI, Lin D, Song J. Crysalis: an integrated server for computational analysis and design of protein crystallization. Sci Rep 2016; 6:21383; PMID:26906024; http://dx.doi.org/10.1038/srep21383
- Bourhis JM, Canard B, Longhi S. Predicting protein disorder and induced folding: from theoretical principles to practical applications. Curr Protein Pept Sci 2007; 8:135-49; PMID:17430195; http://dx.doi.org/10.2174/138920307780363451
- Uversky VN, Radivojac P, Iakoucheva LM, Obradovic Z, Dunker AK. Prediction of intrinsic disorder and its use in functional proteomics. Methods Mol Biol 2007; 408:69-92; PMID:18314578; http://dx.doi.org/10.1007/978-1-59745-547-3_5
- He B, Wang K, Liu Y, Xue B, Uversky VN, Dunker AK. Predicting intrinsic disorder in proteins: an overview. Cell Res 2009; 19:929-949; PMID:19597536; http://dx.doi.org/10.1038/cr.2009.87
- Peng ZL, Kurgan L. Comprehensive comparative assessment of in-silico predictors of disordered regions. Curr Protein Pept Sci 2012; 13:6-18; PMID:22044149; http://dx.doi.org/10.2174/138920312799277938
- Walsh I, Giollo M, Di Domenico T, Ferrari C, Zimmermann O, Tosatto SC. Comprehensive large-scale assessment of intrinsic protein disorder. Bioinformatics 2015; 31:201-8; PMID:25246432; http://dx.doi.org/10.1093/bioinformatics/btu625
- Ishida T, Kinoshita K. Prediction of disordered regions in proteins based on the meta approach. Bioinformatics 2008; 24:1344-8; PMID:18426805; http://dx.doi.org/10.1093/bioinformatics/btn195
- Lieutaud P, Canard B, Longhi S. MeDor: a metaserver for predicting protein disorder. BMC Genomics 2008; 9:S25; PMID:18831791; http://dx.doi.org/10.1186/1471-2164-9-S2-S25
- Fan X, Kurgan L. Accurate prediction of disorder in protein chains with a comprehensive and empirically designed consensus. J Biomol Struct Dyn 2014; 32:448-64; PMID:23534882; http://dx.doi.org/10.1080/07391102.2013.775969
- Peng Z, Kurgan L. On the complementarity of the consensus-based disorder prediction. Pac Symp Biocomput 2012:176-87; PMID:22174273
- Garner E, Romero P, Dunker AK, Brown C, Obradovic Z. Predicting binding regions within disordered proteins. Genome Informatics 1999; 10:41-50; PMID:11072341
- Oldfield CJ, Cheng Y, Cortese MS, Romero P, Uversky VN, Dunker AK. Coupled Folding and Binding with alpha-Helix-Forming Molecular Recognition Elements. Biochemistry 2005; 44:12454-70; PMID:16156658; http://dx.doi.org/10.1021/bi050736e
- Mohan A, Oldfield CJ, Radivojac P, Vacic V, Cortese MS, Dunker AK, Uversky VN. Analysis of molecular recognition features (MoRFs). J Mol Biol 2006; 362:1043-59; PMID:16935303; http://dx.doi.org/10.1016/j.jmb.2006.07.087
- Cheng Y, Oldfield CJ, Meng J, Romero P, Uversky VN, Dunker AK. Mining alpha-helix-forming molecular recognition features with cross species sequence alignments. Biochemistry 2007; 46:13468-77; PMID:17973494; http://dx.doi.org/10.1021/bi7012273
- Disfani FM, Hsu WL, Mizianty MJ, Oldfield CJ, Xue B, Dunker AK, Uversky VN, Kurgan L. MoRFpred, a computational tool for sequence-based prediction and characterization of short disorder-to-order transitioning binding regions in proteins. Bioinformatics 2012; 28:i75-83; PMID:22689782; http://dx.doi.org/10.1093/bioinformatics/bts209
- Yan J, Dunker AK, Uversky VN, Kurgan L. Molecular Recognition Features (MoRFs) in three domains of life. Mol Biosyst 2015; 12:697-710; PMID:26651072; http://dx.doi.org/10.1039/c5mb00640f
- Malhis N, Gsponer J. Computational identification of MoRFs in protein sequences. Bioinformatics 2015; 31:1738-44; PMID:25637562; http://dx.doi.org/10.1093/bioinformatics/btv060
- Puntervoll P, Linding R, Gemund C, Chabanis-Davidson S, Mattingsdal M, Cameron S, Martin DM, Ausiello G, Brannetti B, Costantini A, et al. ELM server: A new resource for investigating short functional sites in modular eukaryotic proteins. Nucleic Acids Res 2003; 31:3625-30; PMID:12824381; http://dx.doi.org/10.1093/nar/gkg545
- Dosztanyi Z, Meszaros B, Simon I. ANCHOR: web server for predicting protein binding regions in disordered proteins. Bioinformatics 2009; 25:2745-6; PMID:19717576; http://dx.doi.org/10.1093/bioinformatics/btp518
- Dosztanyi Z, Csizmok V, Tompa P, Simon I. The pairwise energy content estimated from amino acid composition discriminates between folded and intrinsically unstructured proteins. J Mol Biol 2005; 347:827-39; PMID:15769473; http://dx.doi.org/10.1016/j.jmb.2005.01.071
- Peng Z, Wang C, Uversky VN, Kurgan L. Prediction of disordered RNA, DNA, and protein binding regions using DisoRDPbind. In: Kloczkowski A, Zhou Y, Faraggi E, Yang Y, eds. Prediction of Protein Secondary Structure and Other One-dimensional Structural Properties. Springer, 2016.
- Peng Z, Wang C, Uversky AV, Kurgan L. Prediction of disordered RNA, DNA, and protein binding regions using DisoRDPbind. Methods Mol Biol 2015: accepted.
- Meng F, Kurgan L. DFLpred: High-throughput prediction of disordered flexible linker regions in protein sequences. Bioinformatics 2016; 32:i341-i50; PMID:27307636; http://dx.doi.org/10.1093/bioinformatics/btw280
- Iakoucheva LM, Radivojac P, Brown CJ, O'Connor TR, Sikes JG, Obradovic Z, Dunker AK. The importance of intrinsic disorder for protein phosphorylation. Nucleic Acids Res 2004; 32:1037-49; PMID:14960716; http://dx.doi.org/10.1093/nar/gkh253
- Pejaver V, Hsu WL, Xin F, Dunker AK, Uversky VN, Radivojac P. The structural and functional signatures of proteins that undergo multiple events of post-translational modification. Protein Sci 2014; 23:1077-93; PMID:24888500; http://dx.doi.org/10.1002/pro.2494
- Brown CJ, Johnson AK, Dunker AK, Daughdrill GW. Evolution and disorder. Curr Opin Struct Biol 2011; 21:441-6; PMID:21482101; http://dx.doi.org/10.1016/j.sbi.2011.02.005
- Sickmeier M, Hamilton JA, LeGall T, Vacic V, Cortese MS, Tantos A, Szabo B, Tompa P, Chen J, Uversky VN, et al. DisProt: the Database of Disordered Proteins. Nucleic Acids Res 2007; 35:D786-93; PMID:17145717; http://dx.doi.org/10.1093/nar/gkl893
- Oates ME, Romero P, Ishida T, Ghalwash M, Mizianty MJ, Xue B, Dosztanyi Z, Uversky VN, Obradovic Z, Kurgan L, et al. D(2)P(2): database of disordered protein predictions. Nucleic Acids Res 2013; 41:D508-16; PMID:23203878; http://dx.doi.org/10.1093/nar/gks1226
- Romero P, Obradovic Z, Li X, Garner EC, Brown CJ, Dunker AK. Sequence complexity of disordered protein. Proteins 2001; 42:38-48; PMID:11093259; http://dx.doi.org/10.1002/1097-0134(20010101)42:1%3c38::AID-PROT50%3e3.0.CO;2-3
- Dosztanyi Z, Csizmok V, Tompa P, Simon I. IUPred: web server for the prediction of intrinsically unstructured regions of proteins based on estimated energy content. Bioinformatics 2005; 21:3433-4; PMID:15955779; http://dx.doi.org/10.1093/bioinformatics/bti541
- Ishida T, Kinoshita K. PrDOS: prediction of disordered protein regions from amino acid sequence. Nucleic Acids Res 2007; 35:W460-4; PMID:17567614; http://dx.doi.org/10.1093/nar/gkm363
- Obradovic Z, Peng K, Vucetic S, Radivojac P, Dunker AK. Exploiting heterogeneous sequence properties improves prediction of protein disorder. Proteins 2005; 61 Suppl 7:176-82; PMID:16187360; http://dx.doi.org/10.1002/prot.20735
- Peng K, Radivojac P, Vucetic S, Dunker AK, Obradovic Z. Length-dependent prediction of protein intrinsic disorder. Bmc Bioinformatics 2006; 7:208; PMID:16618368; http://dx.doi.org/10.1186/1471-2105-7-208
- Walsh I, Martin AJ, Di Domenico T, Tosatto SC. ESpritz: accurate and fast prediction of protein disorder. Bioinformatics 2012; 28:503-9; PMID:22190692; http://dx.doi.org/10.1093/bioinformatics/btr682
- Fukuchi S, Amemiya T, Sakamoto S, Nobe Y, Hosoda K, Kado Y, Murakami SD, Koike R, Hiroaki H, Ota M. IDEAL in 2014 illustrates interaction networks composed of intrinsically disordered proteins and their binding partners. Nucleic Acids Res 2014; 42:D320-5; PMID:24178034; http://dx.doi.org/10.1093/nar/gkt1010
- Potenza E, Di Domenico T, Walsh I, Tosatto SC. MobiDB 2.0: an improved database of intrinsically disordered and mobile proteins. Nucleic Acids Res 2015; 43:D315-20; PMID:25361972; http://dx.doi.org/10.1093/nar/gku982
- Linding R, Jensen LJ, Diella F, Bork P, Gibson TJ, Russell RB. Protein disorder prediction: implications for structural proteomics. Structure 2003; 11:1453-9; PMID:14604535; http://dx.doi.org/10.1016/j.str.2003.10.002
- Linding R, Russell RB, Neduva V, Gibson TJ. GlobPlot: exploring protein sequences for globularity and disorder. Nucleic Acids Res 2003; 31:3701-8; PMID:12824398; http://dx.doi.org/10.1093/nar/gkg519
- Yang ZR, Thomson R, McNeil P, Esnouf RM. RONN: the bio-basis function neural network technique applied to the detection of natively disordered regions in proteins. Bioinformatics 2005; 21:3369-76; PMID:15947016; http://dx.doi.org/10.1093/bioinformatics/bti534
- Varadi M, Kosol S, Lebrun P, Valentini E, Blackledge M, Dunker AK, Felli IC, Forman-Kay JD, Kriwacki RW, Pierattelli R, et al. pE-DB: a database of structural ensembles of intrinsically disordered and of unfolded proteins. Nucleic Acids Res 2014; 42:D326-35; PMID:24174539; http://dx.doi.org/10.1093/nar/gkt960
- Dunker AK, Lawson JD, Brown CJ, Williams RM, Romero P, Oh JS, Oldfield CJ, Campen AM, Ratliff CM, Hipps KW, et al. Intrinsically disordered protein. J Mol Graph Model 2001; 19:26-59; PMID:11381529; http://dx.doi.org/10.1016/S1093-3263(00)00138-8
- Romero P, Obradovic Z, Li X, Garner EC, Brown CJ, Dunker AK. Sequence complexity of disordered proteins. Proteins 2001; 42:38-48; PMID:11093259; http://dx.doi.org/10.1002/1097-0134(20010101)42:1%3c38::AID-PROT50%3e3.0.CO;2-3
- Vucetic S, Brown CJ, Dunker AK, Obradovic Z. Flavors of protein disorder. Proteins 2003; 52:573-84; PMID:12910457; http://dx.doi.org/10.1002/prot.10437
- Vacic V, Uversky VN, Dunker AK, Lonardi S. Composition Profiler: a tool for discovery and visualization of amino acid composition differences. BMC Bioinformatics 2007; 8:211; PMID:17578581; http://dx.doi.org/10.1186/1471-2105-8-211
- Vucetic S, Obradovic Z, Vacic V, Radivojac P, Peng K, Iakoucheva LM, Cortese MS, Lawson JD, Brown CJ, Sikes JG, et al. DisProt: a database of protein disorder. Bioinformatics 2005; 21:137-40; PMID:15310560; http://dx.doi.org/10.1093/bioinformatics/bth476
- Vucetic S, Brown C, Dunker K, Obradovic Z. Flavors of protein disorder. Proteins 2003; 52:573-84; PMID:12910457; http://dx.doi.org/10.1002/prot.10437
- Karlin D, Ferron F, Canard B, Longhi S. Structural disorder and modular organization in Paramyxovirinae N and P. J Gen Virol 2003; 84:3239-52; PMID:14645906; http://dx.doi.org/10.1099/vir.0.19451-0
- Severson W, Xu X, Kuhn M, Senutovitch N, Thokala M, Ferron F, Longhi S, Canard B, Jonsson CB. Essential amino acids of the hantaan virus N protein in its interaction with RNA. J Virol 2005; 79:10032-9; PMID:16014963; http://dx.doi.org/10.1128/JVI.79.15.10032-10039.2005
- Llorente MT, Barreno-Garcia B, Calero M, Camafeita E, Lopez JA, Longhi S, Ferron F, Varela PF, Melero JA. Structural analysis of the human respiratory syncitial virus phosphoprotein: characterization of an a-helical domain involved in oligomerization. J Gen Virol 2006; 87:159-69; PMID:16361428; http://dx.doi.org/10.1099/vir.0.81430-0
- Habchi J, Mamelli L, Darbon H, Longhi S. Structural Disorder within Henipavirus Nucleoprotein and Phosphoprotein: From Predictions to Experimental Assessment. PLoS One 2010; 5:e11684; PMID:20657787; http://dx.doi.org/10.1371/journal.pone.0011684
- Longhi S, Lieutaud P, Canard B. Conformational disorder. Methods Mol Biol 2010; 609:307-25; http://dx.doi.org/10.1007/978-1-60327-241-4_18
- Pollastri G, McLysaght A. Porter: a new, accurate server for protein secondary structure prediction. Bioinformatics 2005; 21:1719-20; PMID:15585524; http://dx.doi.org/10.1093/bioinformatics/bti203
- Fukuchi S, Hosoda K, Homma K, Gojobori T, Nishikawa K. Binary classification of protein molecules into intrinsically disordered and ordered segments. BMC structural biology 2011; 11:29; PMID:21693062; http://dx.doi.org/10.1186/1472-6807-11-29
- Linding R, Jensen LJ, Diella F, Bork P, Gibson TJ, Russell RB. Protein disorder prediction: implications for structural proteomics. Structure (Camb) 2003; 11:1453-9; http://dx.doi.org/10.1016/j.str.2003.10.002
- Ward JJ, McGuffin LJ, Bryson K, Buxton BF, Jones DT. The DISOPRED server for the prediction of protein disorder. Bioinformatics 2004; 20:2138-9; PMID:15044227; http://dx.doi.org/10.1093/bioinformatics/bth195
- Cheng J, Sweredoski M, Baldi P. Accurate prediction of protein disordered regions by mining protein structure data, data mining and knowledge discovery. 2005; 11:213-22.
- Obradovic Z, Peng K, Vucetic S, Radivojac P, Brown CJ, Dunker AK. Predicting intrinsic disorder from amino acid sequence. Proteins 2003; 53 Suppl 6:566-72; PMID:14579347; http://dx.doi.org/10.1002/prot.10532
- Peng K, Vucetic S, Radivojac P, Brown CJ, Dunker AK, Obradovic Z. Optimizing long intrinsic disorder predictors with protein evolutionary information. J Bioinform Comput Biol 2005; 3:35-60; PMID:15751111; http://dx.doi.org/10.1142/S0219720005000886
- Eickholt J, Cheng J. DNdisorder: predicting protein disorder using boosting and deep networks. BMC Bioinformatics 2013; 14:88; PMID:23497251; http://dx.doi.org/10.1186/1471-2105-14-88
- Linding R, Russell RB, Neduva V, Gibson TJ. GlobPlot: Exploring protein sequences for globularity and disorder. Nucleic Acids Res 2003; 31:3701-8; PMID:12824398; http://dx.doi.org/10.1093/nar/gkg519
- Wang L, Sauer UH. OnD-CRF: predicting order and disorder in proteins using [corrected] conditional random fields. Bioinformatics 2008; 24:1401-2; PMID:18430742; http://dx.doi.org/10.1093/bioinformatics/btn132
- Hirose S, Shimizu K, Noguchi T. POODLE-I: Disordered Region Prediction by Integrating POODLE Series and Structural Information Predictors Based on a Workflow Approach. In Silico Biol 2010; 10:185-91; PMID:22430291
- Obradovic Z, Peng K, Vucetic S, Radivojac P, Dunker AK. Exploiting heterogeneous sequence properties improves prediction of protein disorder. Proteins 2005; 61:176-82; PMID:16187360; http://dx.doi.org/10.1002/prot.20735
- Bordoli L, Kiefer F, Schwede T. Assessment of disorder predictions in CASP7. Proteins 2007; 69 Suppl 8:129-36; PMID:17680688; http://dx.doi.org/10.1002/prot.21671
- Deng X, Eickholt J, Cheng J. PreDisorder: ab initio sequence-based prediction of protein disordered regions. BMC Bioinformatics 2009; 10:436; PMID:20025768; http://dx.doi.org/10.1186/1471-2105-10-436
- Noivirt-Brik O, Prilusky J, Sussman JL. Assessment of disorder predictions in CASP8. Proteins 2009; 77 Suppl 9:210-6; PMID:19774619; http://dx.doi.org/10.1002/prot.22586
- Zhang T, Faraggi E, Xue B, Dunker AK, Uversky VN, Zhou Y. SPINE-D: accurate prediction of short and long disordered regions by a single neural-network based method. J Biomol Struct Dyn 2012; 29:799-813; PMID:22208280; http://dx.doi.org/10.1080/073911012010525022
- Galzitskaya OV, Garbuzynskiy SO, Lobanov MY. FoldUnfold: web server for the prediction of disordered regions in protein chain. Bioinformatics 2006; 22:2948-9; PMID:17021161; http://dx.doi.org/10.1093/bioinformatics/btl504
- Zeev-Ben-Mordehai T, Rydberg EH, Solomon A, Toker L, Auld VJ, Silman I, Botti S, Sussman JL. The intracellular domain of the Drosophila cholinesterase-like neural adhesion protein, gliotactin, is natively unfolded. Proteins 2003; 53:758-67; PMID:14579366; http://dx.doi.org/10.1002/prot.10471
- Oldfield CJ, Cheng Y, Cortese MS, Brown CJ, Uversky VN, Dunker AK. Comparing and combining predictors of mostly disordered proteins. Biochemistry 2005; 44:1989-2000; PMID:15697224; http://dx.doi.org/10.1021/bi047993o
- Xue B, Oldfield CJ, Dunker AK, Uversky VN. CDF it all: consensus prediction of intrinsically disordered proteins based on various cumulative distribution functions. FEBS letters 2009; 583:1469-74; PMID:19351533; http://dx.doi.org/10.1016/j.febslet.2009.03.070
- Mohan A, Sullivan WJ, Jr., Radivojac P, Dunker AK, Uversky VN. Intrinsic disorder in pathogenic and non-pathogenic microbes: discovering and analyzing the unfoldomes of early-branching eukaryotes. Mol Biosyst 2008; 4:328-40; PMID:18354786; http://dx.doi.org/10.1039/b719168e
- Callebaut I, Labesse G, Durand P, Poupon A, Canard L, Chomilier J, Henrissat B, Mornon JP. Deciphering protein sequence information through hydrophobic cluster analysis (HCA): current status and perspectives. Cell Mol Life Sci 1997; 53:621-45; PMID:9351466; http://dx.doi.org/10.1007/s000180050082
- Peng Z, Mizianty MJ, Kurgan L. Genome-scale prediction of proteins with long intrinsically disordered regions. Proteins 2014; 82:145-58; PMID:23798504; http://dx.doi.org/10.1002/prot.24348
- Yan J, Mizianty MJ, Filipow PL, Uversky VN, Kurgan L. RAPID: fast and accurate sequence-based prediction of intrinsic disorder content on proteomic scale. Biochim Biophys Acta 2013; 1834:1671-80; PMID:23732563; http://dx.doi.org/10.1016/j.bbapap.2013.05.022
- Kozlowski LP, Bujnicki JM. MetaDisorder: a meta-server for the prediction of intrinsic disorder in proteins. BMC Bioinformatics 2012; 13:111; PMID:22624656; http://dx.doi.org/10.1186/1471-2105-13-111
- Chandonia JM. StrBioLib: a Java library for development of custom computational structural biology applications. Bioinformatics 2007; 23:2018-20; PMID:17537750; http://dx.doi.org/10.1093/bioinformatics/btm269
- Mizianty MJ, Stach W, Chen K, Kedarisetti KD, Disfani FM, Kurgan L. Improved sequence-based prediction of disordered regions with multilayer fusion of multiple information sources. Bioinformatics 2010; 26:i489-96; PMID:20823312; http://dx.doi.org/10.1093/bioinformatics/btq373
- Mizianty MJ, Zhang T, Xue B, Zhou Y, Dunker AK, Uversky VN, Kurgan L. In-silico prediction of disorder content using hybrid sequence representation. BMC Bioinformatics 2011; 12:245; PMID:21682902; http://dx.doi.org/10.1186/1471-2105-12-245
- Mizianty MJ, Uversky V, Kurgan L. Prediction of intrinsic disorder in proteins using MFDp2. Methods Mol Biol 2014; 1137:147-62; PMID:24573480; http://dx.doi.org/10.1007/978-1-4939-0366-5_11
- Xue B, Dunbrack RL, Williams RW, Dunker AK, Uversky VN. PONDR-FIT: a meta-predictor of intrinsically disordered amino acids. Biochim Biophys Acta 2010; 1804:996-1010; PMID:20100603; http://dx.doi.org/10.1016/j.bbapap.2010.01.011
- Schlessinger A, Punta M, Yachdav G, Kajan L, Rost B. Improved disorder prediction by combination of orthogonal approaches. PLoS One 2009; 4:e4433; PMID:19209228; http://dx.doi.org/10.1371/journal.pone.0004433
- Schlessinger A, Yachdav G, Rost B. PROFbval: predict flexible and rigid residues in proteins. Bioinformatics 2006; 22:891-3; PMID:16455751; http://dx.doi.org/10.1093/bioinformatics/btl032
- Schlessinger A, Liu J, Rost B. Natively unstructured loops differ from other loops. PLoS Comput Biol 2007; 3:e140; PMID:17658943; http://dx.doi.org/10.1371/journal.pcbi.0030140
- Schlessinger A, Punta M, Rost B. Natively unstructured regions in proteins identified from contact predictions. Bioinformatics 2007; 23:2376-84; PMID:17709338; http://dx.doi.org/10.1093/bioinformatics/btm349
- Blocquel D, Habchi J, Gruet A, Blangy S, Longhi S. Compaction and binding properties of the intrinsically disordered C-terminal domain of Henipavirus nucleoprotein as unveiled by deletion studies. Mol Biosyst 2012; 8:392-410; PMID:22108848; http://dx.doi.org/10.1039/C1MB05401E
- Uversky VN. Natively unfolded proteins: a point where biology waits for physics. Protein Sci 2002; 11:739-56; PMID:11910019; http://dx.doi.org/10.1110/ps.4210102
- Vacic V, Oldfield CJ, Mohan A, Radivojac P, Cortese MS, Uversky VN, Dunker AK. Characterization of molecular recognition features, MoRFs, and their binding partners. J Proteome Res 2007; 6:2351-66; PMID:17488107; http://dx.doi.org/10.1021/pr0701411
- Meszaros B, Simon I, Dosztanyi Z. Prediction of protein binding regions in disordered proteins. PLoS Comput Biol 2009; 5:e1000376; PMID:19412530; http://dx.doi.org/10.1371/journal.pcbi.1000376
- Bourhis J, Johansson K, Receveur-Bréchot V, Oldfield CJ, Dunker AK, Canard B, Longhi S. The C-terminal domain of measles virus nucleoprotein belongs to the class of intrinsically disordered proteins that fold upon binding to their physiological partner. Virus Res 2004; 99:157-67; PMID:14749181; http://dx.doi.org/10.1016/j.virusres.2003.11.007
- John SP, Wang T, Steffen S, Longhi S, Schmaljohn CS, Jonsson CB. Ebola virus VP30 is an RNA binding protein. J Virol 2007; 81:8967-76; PMID:17567691; http://dx.doi.org/10.1128/JVI.02523-06
- Meszaros B, Tompa P, Simon I, Dosztanyi Z. Molecular principles of the interactions of disordered proteins. J Mol Biol 2007; 372:549-61; PMID:17681540; http://dx.doi.org/10.1016/j.jmb.2007.07.004
- Habchi J, Blangy S, Mamelli L, Ringkjobing Jensen M, Blackledge M, Darbon H, Oglesbee M, Shu Y, Longhi S. Characterization of the interactions between the nucleoprotein and the phosphoprotein of Henipaviruses. J Biol Chem 2011; 286:13583-602; PMID:21317293; http://dx.doi.org/10.1074/jbc.M111.219857
- Daily KM, Radivojac P, Dunker AK. Intrinsic disorder and protein modifications: building an SVM predictor for methylation. IEEE Symposium on Computational Intelligence in Bioinformatics and Computational Biology, CIBCB 2005. San Diego, California, U.S.A., 2005:475-81.
- McGuffin LJ, Bryson K, Jones DT. The PSIPRED protein structure prediction server. Bioinformatics 2000; 16:404-5; PMID:10869041; http://dx.doi.org/10.1093/bioinformatics/16.4.404
- Wootton JC. Non-globular domains in protein sequences: automated segmentation using complexity measures. Comput Chem 1994; 18:269-85; PMID:7952898; http://dx.doi.org/10.1016/0097-8485(94)85023-2
- Kall L, Krogh A, Sonnhammer EL. Advantages of combined transmembrane topology and signal peptide prediction–the Phobius web server. Nucleic Acids Res 2007; 35:W429-32; PMID:17483518; http://dx.doi.org/10.1093/nar/gkm256
- Bornberg-Bauer E, Rivals E, Vingron M. Computational approaches to identify leucine zippers. Nucleic Acids Res 1998; 26:2740-6; PMID:9592163; http://dx.doi.org/10.1093/nar/26.11.2740
- Lupas A, Van Dyke M, Stock J. Predicting coiled coils from protein sequences. Science 1991; 252:1162-4; PMID:2031185; http://dx.doi.org/10.1126/science.252.5009.1162
- Baldi P, Cheng J, Vullo A. Large-scale prediction of disulphide bond connectivity. Adv Neural Inf Process Syst 2004; 17:97-104.
- Eudes R, Le Tuan K, Delettre J, Mornon JP, Callebaut I. A generalized analysis of hydrophobic and loop clusters within globular protein sequences. BMC Struct Biology 2007; 7:2; PMID:17210072; http://dx.doi.org/10.1186/1472-6807-7-2