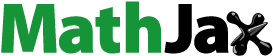
ABSTRACT
The spaceborne imaging spectroscopy mission PRecursore IperSpettrale della Missione Applicativa (PRISMA), launched on 22 March 2019 by the Italian Space Agency, opens new opportunities in many scientific domains, including precision farming and sustainable agriculture. This new Earth Observation (EO) data stream requires new-generation approaches for the estimation of important biophysical crop variables (BVs). In this framework, this study evaluated a hybrid approach, combining the radiative transfer model PROSAIL-PRO and several machine learning (ML) regression algorithms, for the retrieval of canopy chlorophyll content (CCC) and canopy nitrogen content (CNC) from synthetic PRISMA data. PRISMA-like data were simulated from two images acquired by the airborne sensor HyPlant, during a campaign performed in Grosseto (Italy) in 2018. CCC and CNC estimations, assessed from the best performing ML algorithms, were used to define two relations with plant nitrogen uptake (PNU). CNC proved to be slightly more correlated to PNU than CCC (R2 = 0.82 and R2 = 0.80, respectively). The CNC-PNU model was then applied to actual PRISMA images acquired in 2020. The results showed that the estimated PNU values are within the expected ranges, and the temporal trends are compatible with plant phenology stages.
Introduction
Nitrogen (N), naturally present in soil, is the most important macro-nutrient for vegetation growth and productivity (Leghari et al., Citation2016), and for this reason, it is commonly provided in agriculture through fertilisation, in order to maximise biomass production and yield. If nitrogen deficiency can lead to crop production shortage, fertilisation excess can produce negative effects such as plant diseases or lodging, as well as environmental threats such as groundwater leaching or atmospheric pollution (greenhouse gas emission). A recent study estimated that worldwide nitrogen use efficiency in the agro-sector is about 60%, meaning that 40% of N, provided through fertilisation, is wasted in the environment, with negative economic and environmental impacts (Lassaletta et al., Citation2016). Indeed, spatio-temporal information about important crop variables, such as plant nitrogen uptake (PNU – kg ha−1), is fundamental to assess actual plant needs and to develop smart agriculture applications devoted to improve N use efficiency, guarantee sustainable crop production and reduce environmental impacts (Basso et al., Citation2016; Zarco-Tejada & Loudjani, Citation2017).
In the last decades, remote sensing was successfully exploited to map crop nitrogen at farm level, in order to identify the variability of nutritional conditions among and within fields. Most studies derived information on crop nutritional status by estimating leaf/canopy chlorophyll content (Baret et al., Citation2007; Delloye et al., Citation2018; Guerif et al., Citation2007). This workflow had a certain advantage since chlorophyll spectral absorption features are strong in the visible domain and easy to detect through multispectral data as a consequence of light harvest in the photosynthesis process (Berger, Verrelst, Féret, Wang et al., Citation2020). Although a significant amount of literature presents good relationships between nitrogen and chlorophyll (Hansen & Schjoerring, Citation2003; He et al., Citation2016; Tian et al., Citation2011), it was also reported that this linkage is dependent on species and phenological status (Berger, Verrelst, Féret, Wang et al., Citation2020; Homolová et al., Citation2013). Additionally, it is important to remind that chlorophyll contains only a fraction of total leaf (plant) nitrogen (~19%), while the main sink consists of proteins such as rubisco (~30%; Berger, Verrelst, Féret, Wang et al., Citation2020; Kokaly et al., Citation2009). This condition does not guarantee the direct linkage between chlorophyll content and total nitrogen in plant tissue; hence, retrieval schemes based only on chlorophyll estimation may not be transferable in time and space or across species. Consequently, the direct estimation of nitrogen from proteins is foreseen as the best way to exploit remote-sensing information for crop nutritional status monitored across agricultural sites and seasons (Berger, Verrelst, Féret, Wang et al., Citation2020).
Among the different methods presented in the literature for the retrieval of biophysical variables (BVs), data-driven approaches, such as parametric regressions based on vegetation indices calculation (Cilia et al., Citation2014; Clevers & Gitelson, Citation2013; Nutini et al., Citation2018; Stroppiana et al., Citation2009) or machine learning regression algorithm (MLRA) techniques (Verrelst et al., Citation2015, Citation2019), are the most commonly used (Liu et al., Citation2021). Despite the good results, the intrinsic empirical nature of these approaches bounds their use to the specific experimental conditions presented in each study, limiting the transferability to different contexts (including space, time and plant type/crop species). To overcome this issue, physically based methods were proposed for BV estimation through the inversion of radiative transfer models (RTMs). RTMs are based on equations devoted to reconstruct the vegetation reflectance spectrum, as measured by an Earth Observation sensor, considering target features such as vegetation parameters at both leaf and canopy level, background characteristics and illumination/viewing geometry conditions. The estimation of BVs from RTMs can be performed by means of iterative numerical optimization or inversion based on look-up-tables of vegetation spectra (Verrelst et al., Citation2019). Nonetheless, BV retrieval from RTMs remains challenging due to its expensive computational requirements. The hybrid approach, recently introduced by the scientific community for BV retrieval (Berger, Verrelst, Féret, Hank et al., Citation2020; Verrelst et al., Citation2020, Citation2019), represents a possible solution to this problem. This approach consists in the combination of RTM and MLRA: RTM generates a database of simulated vegetation spectra (input), related to the vegetation BVs (output), and MLRA identifies a non-linear model between these input–output pairs. Thus, hybrid methods inherit the generalisation of physically based methods as well as the flexibility and computational efficiency provided by MLRAs. A complete and exhaustive review on this topic is covered in Berger et al. (Citation2020).
Recently, Féret et al. (Citation2021) provided a modification of the well-known leaf-level RTM PROSPECT (Jacquemoud & Baret, Citation1990) by separating the contribution of proteins (Cp) and carbon-based constituents (CBCs) on leaf reflectance, thus allowing to simulate the effect of protein content on leaf spectra. This innovation opens new perspective for the retrieval of leaf protein content as a proxy of plant nitrogen uptake directly from spectroradiometric data. To perform N retrieval from Cp, high spectral resolution data in the full spectral range are needed: besides the mentioned chlorophyll related features in the visible and near infrared (VNIR) spectral region, the main protein absorptions are in the shortwave infrared (SWIR) domain (Berger, Verrelst, Féret, Wang et al., Citation2020; Kokaly et al., Citation2009; Z. Wang, Skidmore, Wang, Skidmore, Wang et al., Citation2015). Hyperspectral data are therefore the candidate data source for protein estimation (Rast et al., Citation2019), and hybrid approaches are the state-of-the-art solution for such retrieval scheme. This approach for canopy nitrogen content (CNC) retrieval was recently tested using spectroradiometric field data (Berger, Verrelst, Féret, Hank et al., Citation2020), simulated hyperspectral satellite imagery (Candiani et al., Citation2022) and actual hyperspectral satellite data, providing the first landscape-level map (Verrelst et al., Citation2021) as well as multitemporal and multi-crop analyses (Tagliabue et al., Citation2022). The foreseen hyperspectral data stream from space, provided by already launched satellites, such as PRISMA (Loizzo et al., Citation2016, Citation2019) from the Italian Space Agency (ASI) or EnMap (Chabrillat et al., Citation2020) from the German Space Agency (GFZ-DLR), or future satellite mission planned in the upcoming years, such as CHIME (Nieke & Rast, Citation2019) from the European Space Agency (ESA) or SBG (Thompson et al., Citation2020) from the National Aeronautics and Space Administration (NASA), will open new opportunities in crop monitoring, sustainable agriculture and precision farming (Hank et al., Citation2019). In this framework, it is important to study and develop new efficient methods for the operational mapping of important crop traits, such as chlorophyll and nitrogen content, exploiting the already available hyperspectral satellite data (i.e. PRISMA).
Consequently, it is necessary to further test the potentiality of hybrid approach to assess canopy chlorophyll and nitrogen content, to evaluate the performance of different MLRAs and the impact of hyperspectral feature selection on BV retrieval. Moreover, it is necessary to set up operational workflows for PNU estimation and to analyse the advantage of the canopy nitrogen content retrieved from proteins with respect to traditional approaches based on chlorophyll estimation. Finally, generation and analysis of multitemporal maps are required to fully assess the capability of imaging spectroscopy to estimate PNU, as an added value for sustainable agriculture.
Therefore, this work develops a hybrid approach for the estimation of maize canopy chlorophyll content (CCC) and canopy nitrogen content (CNC) from actual PRISMA data with the final goal to (i) evaluate the retrieval performance of hybrid models for CCC and CNC, (ii) compare the relations of these BVs with PNU and (iii) demonstrate PRISMA capabilities to map PNU.
Materials and methods
General workflow of the study
provides a synthetic representation of the general workflow of this study (top panel – A) and the method and data specifically used for each step (bottom panel – B). The first step consists in the set-up of hybrid models for PRISMA (database generation and training and validation of different MLRA and dimensionality reduction – DR combination) for the estimation of CCC and CNC. The best hybrid models for CCC and CNC from cross-validation were applied to PRISMA-like data, obtained by HyPlant-DUAL hyperspectral airborne images, to compare BV maps with ground data (Step 1). Specific regressive models between CCC and CNC and PNU were defined using 2018 ground data and PRISMA-like estimations (Step 2). Finally, the best hybrid model for BV retrieval was applied to actual PRISMA data acquired on the same study area on 2020 (Step 3). The best BV–PNU linear model was then applied to BV maps to generate PNU maps. PNU crop dynamics at parcel level was evaluated comparing ranges of parameter estimation to crop phenological status and development as derived from time series of Sentinel-2 data (Step 4). Further details will be given in the following sections.
Study area and field measurements
The study area is located in Tuscany (42°49ʹ47.02” N 11°04ʹ10.27” E; elev. 2 m a.m.s.l.), Central Italy, North of Grosseto, at 20 km far from the coastline (). The site consists of a large flat irrigated area where different crops are cultivated. Within the study area, two maize crops of approximately 76 ha (F1) and 33 ha (F2) were selected as test sites. These two fields feature different irrigation systems and different sowing dates. In particular, because of its extension, F1 was divided into subzones, each sown at different dates.
Figure 2. Study area and field measurements. Panel (a) shows the study area, field boundaries and maize cultivation (yellow-meshed colour) for 2020, 1 August 2020 PRISMA false colour image on the background. Panel (b) indicates the test fields showing the ESU of the first (light blue points) and second (yellow points) field campaigns, 31 July 2018 HyPlant-DUAL false colour image on the background.

During June and July 2018, in the framework of the FLuorescence EXplorer mission of the European Space Agency (ESA-FLEX) project, two field campaigns were carried out on the two fields, in order to collect a comprehensive dataset of biochemical and biophysical parameters. Independent measurements were conducted on 87 Elementary Sampling Units (ESU) of 10 × 10 m2, following international protocols and guidelines, as proposed by the CEOS LPV group (Morisette et al., Citation2006) and the VALERI project (Weiss, Citation2006).
Leaf biochemical variables, such as chlorophyll content (LCC), nitrogen concentration (N%) and leaf mass per area (LMA), were measured from the last fully developed leaf from three plants collected on a subset of 31 ESUs. Laboratory extractions of LCC, N% and LMA were performed on a set of three disks with 2.2 cm diameter (total area 11.40 cm2) sampled from each leaf. In addition, for all the 87 ESUs, indirect measurements of leaf chlorophyll were acquired using a SPAD-502 chlorophyll meter (Konica Minolta, Japan). LCC values from laboratory extractions (71 samples) and the corresponding SPAD measurements were used to identify the SPAD–LCC relationship (R2 = 0.93):
Leaf nitrogen content (LNC) was calculated from N% and LMA according to the following equation:
In addition, leaf area index (LAI) was measured in all ESUs using either LAI2200 plant analyser (LI-COR Biosciences, USA) or digital hemispherical photography (Jonckheere et al., Citation2004; Weiss et al., Citation2004), depending on the plant development stage. LAI values were then used to derive CCC and CNC according to the following equations:
Finally, plant density was measured in 27 ESUs. In each of these ESUs, two plants were randomly sampled for subsequent leaf and stalk dry weight measurement. Biomass was calculated by multiplying plant density by average plant dry weight (leaf plus stalks). On the same leaf and stalk samples, nitrogen concentration was also measured in laboratory using CN elemental analyser (Flash EA 1112 NC-Soil, Thermo Fisher Scientific, Pittsburgh, PA, USA), and PNC [N%] was obtained as a weighted average relative to the weight of these specific plant organs. Plant nitrogen uptake was then derived following the equation:
More details of the field and laboratory measurement protocols can be found in Candiani et al. (Citation2022). provides a summary of the available ground measurements.
Table 1. Crop traits at leaf (L), canopy (C) and plant (P) level.
EO dataset
The main EO dataset is represented by PRISMA imagery. PRISMA is a medium-resolution hyperspectral imaging mission of ASI launched in 2019. The PRISMA project is conceived as a pre-operational and technology demonstrator mission, focused on the development and delivery of hyperspectral products and the qualification of the hyperspectral payload in space. The mission provides hyperspectral images at 231 bands, with a swath of 30 km and a GSD of 30 m. The spectral resolution is finer than 12 nm all over the sensor spectral range (400–2500 nm). The satellite includes a panchromatic sensor providing images in the visible spectral range, with a GSD of 5 m.
Since PRISMA was not available during the field campaign carried out in 2018, PRISMA-like data were simulated from two hyperspectral images acquired by the airborne sensor HyPlant-DUAL at the same time of the ground measurements. These images feature 480 bands with a spectral resolution (full width half maximum [FWHM]) of 3–10 nm in the spectral range 380–2510 nm and a GSD value of 1 m and 4.5 m for the scenes acquired on 7 and 30 July, respectively. The atmospheric correction was performed through an empirical line method using spectral signatures of artificial targets, vegetation, water and soil, acquired during the field campaigns (Siegmann et al., Citation2019). To simulate PRISMA-like data, HyPlant-Dual dataset was spectrally resampled to PRISMA wavelengths using Gaussian spectral response functions with band centres and FWHM values computed from actual PRISMA data.
The 2020 dataset includes two L2D PRISMA images acquired on 21 June and 1 August. In order to obtain smooth spectra, PRISMA images were pre-processed using RStudio® following the procedure described in Tagliabue et al. (Citation2022) and Verrelst et al. (Citation2021). This procedure included several steps: (i) random spikes at specific wavelengths were removed using findpeaks function included in the “pracma” package (Borchers, Citation2013), (ii) noisy wavelengths were excluded comparing PRISMA reflectances to ground spectra (i.e. vegetation, asphalt, and crop residues) collected with a field spectroradiometer (SR-4500; Spectral Evolution, USA); and (iii) a spline smoothing interpolation was then applied using the SplineSmoothGapfilling function implemented in the “FieldSpectroscopyCC” package (Julitta et al., Citation2016).
Moreover, for both simulated (Berger et al., Citation2018) and actual (Berger, Verrelst, Féret, Wang et al., Citation2020) PRISMA data, water absorption regions were excluded as well as other bands in the blue region based on considerations in Upreti et al. (Citation2019) and Weiss, Baret et al. (Citation2020). The final set included 150 bands in the spectral ranges 456.2–759.84 nm, 780.66–918.92 nm, 969.3–1109.71 nm, 1163.45–1338.91 nm, 1501.74–1784.39 nm and 2019–2320.59 nm.
In addition to the PRISMA dataset, 31 Sentinel-2 (S2) level 2A images acquired from March to November 2020 were downloaded and pre-processed by means of “sen2r” R package (L. Ranghetti et al., Citation2021; Team, Citation2021) with the goal to reconstruct temporal crop dynamics to evaluate 2020 PRISMA PNU maps. The “sen2r” package was used to automatically download all the S2 images available on the study area between March and November 2020 and process them in order to resample at 10 m all the bands and to extract quality metadata (Scene Classification Map – SCL). shows all the EO datasets described before and used in this study.
Table 2. Details of EO datasets used in this study: year of the dataset, sensor, number of available images, bands, ground sampling distance and acquisition dates (original values are reported in round brackets).
Hybrid model set-up
The hybrid approach involves two steps: (i) the generation of a reflectance spectra database and (ii) the training of MLRAs. The RTM is used to generate a database of thousands of crop reflectance spectra according to a wide range of crop leaf and canopy parameters as well as background reflectance, viewing and illumination geometry. The advantage of using RTMs is the possibility to simulate different conditions which can be found in crop fields, hence widening the range of situations found in limited ground measurements. This database together with the associated BVs of interest is then used as input to train MLRAs. Compared to a traditional ML data-driven approach, the combination of physically based RTMs with the flexibility and computational efficiency of MLRAs should guarantee the identification of generic and robust models, hence their exportability in different contexts, enabling the operational monitoring of BVs. shows the advantages of hybrid approach and some critical aspects to be considered when setting and using the model for crop trait retrieval and analysing the results.
Table 3. Advantages and limitations/critical aspects of hybrid approach for crop traits retrieval.
Database generation phase
The RTM used for the database generation was the PROSAIL, one of the most exploited RTMs (Jacquemoud et al., Citation2009) which includes the leaf model PROSPECT (Jacquemoud & Baret, Citation1990) and the canopy model SAIL (Verhoef, Citation1984). In particular, an ad-hoc MATLAB® script was developed for this study to combine the latest version of the leaf model PROSPECT (PROSPECT-PRO, Féret et al., Citation2021) with the canopy model 4SAIL (Verhoef, Citation1984; Verhoef et al., Citation2007).
In order to simulate leaf reflectance, the PROSPECT model requires several input parameters, describing the leaf structure (N) as well as the content of chlorophyll (LCC), carotenoid (Ccx), anthocyanin (Canth), brown pigment (Cbp) and water (Cw). Moreover, PROSPECT-PRO introduced two additional variables to differentiate the absorption behaviour of protein content (Cp) and carbon-based constituents (CBCs), which includes cellulose, lignin, hemicellulose, starch and sugar. Leaf reflectance obtained from PROSPECT-PRO can be passed to 4SAIL to simulate the canopy reflectance in a simple way, requiring only few parameters, such as leaf area index (LAI) and average leaf angle (ALA). Additionally, the model requires information on illumination conditions and viewing geometry, such as Solar Zenith Angle (SZA), Observer Zenith Angle (OZA), relative Azimuth Angle (rAA) and the hot-spot effect (Hot parameter), computed as the ratio of leaf size over canopy height (Kuusk, Citation1991) and the reflectance of the background below vegetation canopy (BG).
The simulations from PROSAIL-PRO were used to create a training database of 2000 maize reflectance spectra, based on different combinations of input variables, randomly sampled from either normal or uniform probability density functions, defining the crop (leaf and canopy) parameters, the soil type and the sun-sensor geometry. According to preliminary studies (M. Ranghetti et al., Citation2021), the size of 2000 samples was chosen as the best trade-off between accuracy and computation cost. shows the input parameters with their symbols, units of measurement, probability distribution functions and ranges of the sampled data. The ranges of the input parameters were set according to the available field measurements or literature values (Berger et al., Citation2018) when field measurements were not available.
Table 4. Parametrization of the leaf (PROSPECT-PRO) and canopy (4SAIL) model with unit of measurements, ranges and distributions coming from the field campaign.
From each set of input variables, the canopy reflectance was simulated at the spectral resolution of 1 nm, ranging from 400 to 2500 nm. These spectra were then resampled at PRISMA spectral configuration (150 bands) according to the procedure described in Section “EO Dataset”. Starting from the input RTM variables, CCC and CNC were computed according to EquationEquations (3)(3)
(3) and (Equation4
(4)
(4) ). The database with 2000 reflectance spectra and the corresponding CCC and CNC values was used as input in the following MLRA training phase.
MLRA training phase
During the training phase of CCC and CNC models, different combinations of MLRAs and dimensionality reduction (DR) methods were tested. The ML algorithms used in this study include Gaussian Process Regression (GPR), Artificial Neural Networks (NN), Partial Least Square Regression (PLSR), Random Forests (RF) and Support Vector Regression (SVR). gives a brief overview of the adopted ML algorithms.
Table 5. Brief description and references of the algorithms tested in this study.
Full spectral signature has been previously adopted in retrieval approaches based on look-up table inversion (Wang et al., Citation2018; Zheng et al., Citation2018; Zhou et al., Citation2020) or numerical optimization strategies (Wang, Skidmore, Darvishzadeh et al., Citation2015; Wang, Skidmore, Wang et al., Citation2015). However, despite the high information content provided by hyperspectral sensors like PRISMA, thanks to hundreds of narrow bands, such datacube is affected by the so-called curse of dimensionality (Hughes phenomenon; Hughes, Citation1968) and is characterised by spectral redundancy and noise, which may lead to suboptimal regression performances (Verrelst et al., Citation2019). For such reason, DR strategies are proposed in the literature to mitigate this problem and improve model efficiency (Bruce et al., Citation2002; Mojaradi et al., Citation2009; Rivera-Caicedo et al., Citation2017). Different approaches are available based on band selection, either expert based (Wang et al., Citation2015) or automatic (Verrelst, Rivera et al., Citation2016) and feature transformation (Candiani et al., Citation2022; Pepe et al., Citation2020). A recent paper by Pascual-Venteo et al. (Citation2022) performed an analysis of different DR methods, comparing band selected by statistical ranking against principal component analysis (PCA) in a hybrid retrieval approach. Results show that PCA with 20 components, explaining about 99.95% cumulative variance of the full spectral data, slightly outperformed band ranking in the retrieval of all the considered variables. On the basis of these results, PCA was considered as the most promising DR strategy to be exploited in the hybrid approach. This technique transforms a large set of variables (hyperspectral bands) into a new one where the first components contain most of the information of the original set. Reducing the number of variables in a dataset naturally comes at the expense of accuracy, but smaller datasets are easier to explore and visualise and make ML computation faster. Therefore, different numbers of components (PCA-5, PCA-10, PCA-15 and PCA-20) were tested as input to ML to assess their impact on model performance.
In addition, the database is simulated without considering different environmental and instrumental uncertainties, such as sensor or instrument noise, RTM assumption, radiometric calibration, atmospheric and geometric correction (Baret et al., Citation2007); therefore, the addition of noise before MLRA training phase helps to generalise the pure RTM model outputs. As such, a Gaussian white noise of 5% was added to the parameters and canopy reflectances.
At the end of this process, the performance of all 40 model combinations (5 MLRAs × 4 DRs × 2 BVs) was assessed through a k-fold cross-validation technique, with k = 10. In particular, the analysed metrics are the following: Mean Absolute Error (MAE), Root Mean Squared Error (RMSE), RMSE relative to mean observed values (rRMSE), RMSE normalised with respect to observed range values (nRMSE) and the Coefficient of Determination (R2).
BV validation and their relationship with PNU
The best performing trained models for CCC and CNC were then applied to the 2018 datasets reported in . The independent validation of estimated CCC and CNC data was performed against ground data measured during the ESA-FLEX campaign carried out in 2018 according the same error metrics computed in cross-validation phase. Among these error metrics, MAE was chosen as an indicator to assess the best-performing algorithm for CCC and CNC, respectively. All the steps involving model training, validation and generation of maps were performed through ARTMO (Verrelst et al., Citation2021). Orthogonal regression analysis (reduced major axis [RMA]) was adopted to analyse performance and identify a predictive relation between PRISMA-derived CCC or CNC and field measurements of PNU. The MATLAB® gmregress function was used for this analysis (Trujillo-Ortiz & Hernandez-Walls, Citation2010). RMA regression is specifically formulated to handle errors in both the x (independent – BVs) and y (dependent – PNU) variables. The method was proposed in the literature for estimation of biophysical variables by taking into account uncertainty in both ground and remote measures (Berterretche et al., Citation2005; Cohen et al., Citation2003).
PNU map demonstration
In order to demonstrate hybrid approach and PRISMA capability to map PNU, the best hybrid model for BV estimation, identified in the previous step, was applied to the actual 2020 PRISMA dataset. The BV–PNU relation was then used to convert the BV maps into PNU maps, over the agricultural study area.
In order to investigate the temporal behaviour of the considered crops and to extract remarkable crop dates, Sentinel-2 dataset acquired in 2020 over the same area of interest was also exploited to extract and analyse time series of the Modified Soil Adjusted Vegetation Index 2 (MSAVI2, hereinafter referred as MSAVI; Qi et al., Citation1994).
In particular, the R package “sen2rts” (L. Ranghetti et al., Citation2021) was exploited to extract MSAVI time series over the boundaries of 39 maize fields according to official regional information (http://dati.toscana.it/dataset/artea-piani-colturali-grafici-annualita-2020, accessed 5 November 2021). Raw values were smoothed using a Savitzky-Golay lowpass filter, using the SCL layer to weight them based on their associated quality. Maize cropping cycles were identified and interpolated with a double logistic function, from which the following relevant dates were computed:
Start of season (SOS), as the date in which the first derivative of the growing curve reached the 10% of its maximum value;
Peak of production (POP), as the date of occurrence of the maximum interpolated MSAVI value;
End of season (EOS), as the date in which the descending curve reached the 50% of its relative value.
These phenological dates were used to interpret PNU values, estimated at the two PRISMA acquisition dates, according to crop development. Besides the assessment of absolute estimation values (ranges in relation to expected values) and interpretation of consistency of produced maps in terms of identified spatial patterns (within field variability), phenological analysis was devoted to assess reliability of estimated PNU temporal variation.
Results
Hybrid model performance for CCC and CNC
Generally, good results were obtained in cross-validation for all algorithms with their tested dimensionality reduction (PCA) with R2 values ranges between 0.90–0.97 and 0.56–0.68 and MAE ranges between 0.05–0.11 g m−2 and 0.80–0.91 g m−2 for CCC and CNC, respectively. GPR resulted in the most suitable algorithm, followed by NN, RF and finally SVR and PLSR. Moreover, for all the tested algorithms, except for PLSR, PCA-15 and PCA-20 led to slightly superior results than using PCA with less components (PCA-5 and PCA-10). The performances of the hybrid ML algorithms in cross-validation configuration are resumed in . According to cross-validation metrics (MAE and R2), the best algorithm configuration was represented by GPR (as ML) with PCA20 (as DR), for both BVs.
Table 6. Cross-validation metrics of hybrid MLRAs and DR tested for CCC retrieval: Mean Absolute Error (MAE, g m−2), Root Mean Squared Error (RMSE, g m−2), relative RMSE (rRMSE, %), normalised RMSE (nRMSE, %) and coefficient of determination (R2).
Table 7. Cross-validation metrics of hybrid MLRAs and DR tested for CNC retrieval: Mean Absolute Error (MAE, g m−2), Root Mean Squared Error (RMSE, g m−2), relative RMSE (rRMSE, %), normalised RMSE (nRMSE, %) and coefficient of determination (R2).
BV validation and PNU relation
These models were used to map CNC and CCC from PRISMA-like images. The estimations of CCC and CNC were validated using, respectively, 87 and 31 field measurements, available from the Grosseto 2018 dataset. Good results were obtained for CCC and CNC with R2 around 0.79 and 0.84 and nRMSE around 19% and 15%, respectively. shows the scatterplots between measured and estimated BVs. In general, CCC resulted in somewhat overestimated, whereas CNC retrieval was slightly underestimated. Moreover, the scatterplot highlights higher uncertainties in the CNC retrieval, featuring higher coefficient of variation values than those associated to CCC retrieval.
Both BV estimations resulted in strongly correlated to ground measurements of PNU (), with CNC showing a slightly better correlation with respect to CCC (R2 = 0.82 and R2 = 0.80, respectively). Moreover, remote-sensed CNC is a direct estimation of a fraction of PNU, representing the organ-specific uptake from the leaves. In this view, the underestimation reported in the plot is an expected behaviour (Bender et al., Citation2013; Berger, Verrelst, Féret, Hank et al., Citation2020). Indeed, this characteristic of CNC, together with the slightly better correlation with PNU, supports this BV being potentially more important than traditional CCC to assess maize nutritional status.
PNU maps from PRISMA data
shows PNU maps, obtained from actual PRISMA data acquired on 21 June 2020 ( and 1 August 2020 ( with the proposed retrieval approach. The maps show a typical situation of summer Mediterranean cropland: a majority of land with low PNU values (reddish colours) and few fields with high PNU values (green-bluish colours). Low PNU values in the June image may represent either winter crop fields (in senescence phase or already harvested) or secondary crop fields with late sown (see southern portion of F1 in or alfalfa fields after mowing. High PNU values in June, besides the maize fields, correspond to sunflower and chickpeas, whose patches disappear in the August image as a consequence of harvesting. In August, the only fields in vegetative phase are represented by maize crop.
Figure 5. PNU maps and zoom on fields with different intra-fields sowing dates (c–d) on (a) 21 June 2020 and (b) 1 August 2020.

In order to evaluate the PNU maps generated from the proposed approach, boxplot diagrams of PNU values were produced for each maize field. These boxplots were compared to the temporal MSAVI trend of the same field. reports this comparison for four different fields, presenting different dynamics according to sowing dates, cultivated varieties and agro-practices. MSAVI plots show original (black dots) and Savitzky–Golay smoothed (black line) Sentinel-2 data and the double logistic curve (red line) used to assess crop phenological stages. The MSAVI analyses of the selected fields highlight situation where maize was in vegetative phase (, late sowing) or already in reproductive phase (, early sowing) during PRISMA acquisitions.
Figure 6. Temporal MSAVI and PNU boxplot in four different maize fields. Original and Savitzky–Golay smoothed Sentinel-2 data are reported as black dots and black line, respectively. The red line represents the double logistic curve used to compute the dates of start of season (green), peak of production (blue) and end of season (orange). The red dashed lines are the dates of PRISMA acquisitions for which PNU maps were calculated (21 June 2020 and 1 August 2020).

Discussion
Hybrid workflow configuration to estimate nitrogen uptake
Accuracy and error metrics from the cross-validation analysis demonstrated how GPR resulted in the best-performing algorithm (four times out of five best ranks – see ) among all the tested ML × DR combinations, for both BVs. In particular, the GPR-PCA20 configuration provided the absolute best-performing metrics in cross-validation: R2 = 0.97 and 0.68 and RMSE 0.11 g m−2 and 1.23 g m−2 for CCC and CNC, respectively. These results are in agreement with other studies found in the literature, confirming GPR algorithm to be a useful tool for the operational retrieval of biophysical variables from either multispectral (Upreti et al., Citation2019; Zhou et al., Citation2020) or hyperspectral (Berger, Verrelst, Féret, Hank et al., Citation2020; Candiani et al., Citation2022; Tagliabue et al., Citation2022; Verrelst et al., Citation2021) data. Another high-performing ML algorithm was NN, with 20 and 15 PCA components for CCC and CNC, respectively. Indeed, NN is used in different hybrid approach (Delloye et al., Citation2018), and it was selected as the base for operational products generated with Sentinel-2 data in the official ESA biopar processor SNAP (ATBD, Weiss, Baret et al., Citation2020). Recently, Upreti et al. (Citation2019) tested different ML algorithms for the estimation of LAI, LCC, CCC and fractional cover, in two test sites located in Maccarese (Italy) and Shunyi (China). Regarding CCC, PLSR and RF resulted the best configurations for Maccharese and Shunyi, respectively. In another study, Liu et al. (Citation2021) used RF in a hybrid retrieval schema for CCC but selecting it a priori with no comparison with other algorithm.
In conclusion, hybrid approaches are proposed as efficient inversion schemes, but no common agreement still exists about the best ML to be adopted. From the results achieved in this study, GPR and PCA transformation represented the best solution for CCC and CNC retrieval. This is in accordance with other papers which demonstrated that GPR with applied PCA ensures good estimation accuracy for crop BVs (Candiani et al., Citation2022; Danner et al., Citation2021; De Grave et al., Citation2020; Rivera-Caicedo et al., Citation2017; Tagliabue et al., Citation2022). Besides the good experimental results obtained in this study, performance and efficiency provided by coupling GPR and PCA are also confirmed by their intrinsic characteristic. GPR, being a kernel-based regression method, works well with small dataset and can provide uncertainty errors, in other words, an insight of how the algorithm is performing. Moreover, a spectral reduction based on the PCA approach has the benefit to exploit the full spectral signature and, at the same time, to reduce collinearity and redundancy typical of high-dimensional data like hyperspectral images.
BV retrieval performance and PNU relation
Performances of the best-identified retrieval configurations were validated with independent ground data, collected in real farm conditions, by sampling spatial heterogeneity of two fields with different sowing dates. The obtained results were more than satisfying as demonstrated by accuracy and error metrics: R2 of 0.79 and 0.84 and nRMSE of 19% and 15% for CCC and CNC, respectively. Scatterplots in show that data are well aligned on 1:1-line despite some overestimation for CCC and a slightly underestimation for CNC values greater than 6 g m−2 (60 kg ha−1). Validation results of CCC retrieval are comparable or even better than those reported in the literature, considering both hybrid approach using S2 data for wheat monitoring (R2 = 0.61 and nRMSE = 26%; Delloye et al., Citation2018; R2 = 0.74 and nRMSE = 23%; Upreti et al., Citation2019) and hyperspectral data from field spectroradiometric measurements on heterogeneous grassland (R2 = 0.74 and nRMSE = 33%; Darvishzadeh et al., Citation2008).
For what concerns CNC, the results are very promising achieving a comparable performance when compared with traditional empirical approaches on multispectral data (RedEdge vegetation indices for maize: R2 = 0.79–0.87; Schlemmera et al., Citation2013) or more sophisticated combination of ML (PLSR for leaf nitrogen estimation) and RTM (for LAI) exploiting hyperspectral data (R2 = 0.85; S. Wang et al., Citation2021). Moreover, it is important to note that, with respect to other reported researches on maize (Crema et al., Citation2020; S. S. Wang et al., Citation2021), this study was performed on real field condition with no artificial nitrogen variability generated by different fertilisation levels. Therefore, the range of values is more limited and mainly related to natural spatial diversity within a typical cultivated field in the Mediterranean area. Other studies tested the hybrid approach exploiting the PROSAIL-PRO model. Berger et al. (Citation2020) demonstrated retrieval feasibility using multitemporal field spectroradiometric measurements conducted along the entire crop season. The results showed a good correlation with field data, even though estimates from the developed hybrid nitrogen model resulted in more correlation with leaf and stalk nitrogen (i.e. PNU; R2 = 0.84–0.86 and RMSE = 2.32–2.15 g m−2) than with only leaf nitrogen (i.e. CNC; R2 = 0.69–0.71 and RMSE = 5.8–5.5 g m−2), which was strongly overestimated for medium–high nitrogen values. It is important to remark that, in Berger et al. (Citation2020) study, field CNC was estimated in a different way: organ-specific N% (i.e. plant part) was multiplied by the corresponding dry mass per unit of ground area, whereas, in this study, CNC estimations were obtained from LNC, determined from leaf disk measurements with fixed area, multiplied by corresponding measured LAI. Moreover, our field dataset was limited to the vegetative phase (V2/3 and V11/12; Ritchie et al., Citation1989) when neither senescence nor cobs, hence translocation processes, were present. The same approach was recently adopted by Verrelst et al. (Citation2021) to produce the first landscape canopy nitrogen maps from PRISMA. In that study, a GPR and active learning techniques (Verrelst, Dethier et al., Citation2016) were applied to the same dataset of Berger et al. (Citation2020) in order to define a predictive CNC model to be applied to real spaceborne data. After active learning training (and including non-vegetated spectra), hybrid CNC model shows good performances (R2 = 0.69, RMSE = 3.42 g m−2 and nRMSE = 17%) but slightly worse than those obtained in our study (R2 = 0.84 and nRMSE = 15%). Finally, similar results to this study were obtained in Candiani et al. (Citation2022) and Tagliabue et al. (Citation2022), which share the same field protocol for CNC measurements, even though the latter adopted an active learning technique exploiting the available data to train the model.
Since PNU is the biophysical parameter of interest, used to perform “plant-based diagnosis” of crop N nutritional status using the concept of “critical N dilution curve” (Lemaire, Citation2021), CCC and CNC estimations were then compared to this variable to find the best BV-PNU model. CCC was strongly correlated with PNU (R2 = 0.80). This can also be explained considering that only a specific crop (maize) was investigated in limited vegetative phase. The result confirms the validity of the approach proposed in the literature for wheat (Baret et al., Citation2007; Delloye et al., Citation2018; Guerif et al., Citation2007) and maize (Crema et al., Citation2020; Schlemmera et al., Citation2013). However, the comparison with reported examples is not straightforward: the studies show different CCC–PNU performances and regression parameters and confounding terminology on which part of plant nitrogen uptake is considered (e.g. entire plant, only leaves/canopy, etc.). These aspects highlight the empirical nature of CCC–PNU relation. This is also reported in other studies that underline how the chlorophyll-based relation with nitrogen is moderately strong across different species and dependent on crop status (Berger, Verrelst, Féret, Hank et al., Citation2020; Berger, Verrelst, Féret, Wang et al., Citation2020; Homolová et al., Citation2013). Under this perspective, PNU estimated from CCC may not be transferable in different contexts.
On the other hand, the identified CNC–PNU relation, besides slightly better (R2 = 0.82), appears to be physiologically based, being leaf-level nitrogen uptake a part of the entire plant nitrogen sink. As a matter of fact, plant nitrogen is strictly bound to proteins, while chlorophyll pigments represent only a part of it as reported in the work by Kokaly et al. (Citation2009) and Berger et al. (Citation2020). In our study, estimated CNC resulted, on average, about 30% of PNU in different analysed conditions (e.g. F1 and F2) which is compatible to what reported in the literature for maize hybrids (Bender et al., Citation2013; Berger, Verrelst, Féret, Hank et al., Citation2020). In this context, traditional agronomic experiment (available or to be specifically conducted) could be used to develop allometric relations between organ-specific (e.g. canopy level) and total plant N uptake. Assimilation of CNC in crop models can be considered a powerful alternative to estimate nitrogen partitioning in the plant (Weiss, Jacob et al., Citation2020). These considerations candidate CNC as a more direct way to estimate PNU from spectral data without using empirical relation with another variable, such as chlorophyll. From an operational point of view, PNU estimated from CNC should be more robust and transferable across seasons and crops.
Nitrogen uptake mapping and implication for precision agriculture and crop monitoring
The best CNC hybrid model (GPR algorithm with PCA-20) was applied to 2020 PRISMA acquisitions for the generation of CNC maps on the study area. The relation between CNC and PNU was then used to map the spatial distribution of PNU in all the maize fields identified from the official common agricultural policy declarations (). PNU maps for maize fields showed values within expected ranges, except for the peak, when PNU reaches values of about 180–200 kg ha−1 (Bender et al., Citation2013; Berger, Verrelst, Féret, Hank et al., Citation2020).
Intra-field variability from PNU maps provides useful information related to crop development in early phenological stages. This is more evident from the first PRISMA image acquired in late June, in which some maize fields with late sowing date show plants not yet in full development (). It is also interesting to discuss the temporal evolution of PNU estimation in relation to crop conditions and phenological development. Crop dynamics for each investigated field were reconstructed using the time-series analysis provided by “sen2rts” R package (L. Ranghetti et al., Citation2021). Analysis of MSAVI trend of each field, derived from S2 imagery, allowed estimating dates of important crop phases such as “start of season”, “peak of production” and “end of season”. PNU estimation for the two dates resulted in agreement with the expected nitrogen uptake trend from the plant, showing an increase before the reproductive stage up to the “peak of production” and a reduction afterwards due to senescence and translocation to reproductive organs (see boxplot diagrams in ).
PNU maps produced from spaceborne hyperspectral data (e.g. PRISMA) can be exploited for several applications; in this context, user needs would determine what would be the most useful maps to consider. In case of precision farming workflows devoted to provide information to end-user for agro-management, an active interaction with farmers is expected. Based on sowing dates, farmers will exploit geo-products provided at time suitable to support fertilisation. Indeed, mapping the plant nitrogen spatial variability information within fields during leaf development stages (V3 – V13, Ritchie et al., Citation1989) is crucial for the generation of prescription maps; these data can be directly exploited by variable rate technology machinery for the application of site-specific fertilisation (Crema et al., Citation2020).
In case of system devoted to monitor district (regional) crop condition or to estimate yield and grain quality (Busetto et al., Citation2017), the most usable proxies for final yield estimation are remote-sensing products related to plant nitrogen acquired close to the peak of season (about flowering; Wang et al., Citation2021). Crema et al. (Citation2020) demonstrated that nitrogen nutritional index maps derived by remote-sensing products can be a good proxy to identify statistical difference in maize production among fields. This study showed that, for an operational nitrogen monitoring system, PNU information obtained from EO data at field level needs to be integrated by Sentinel-2 decametric data to identify specific crop stages.
Conclusions
In this study, an operational workflow for the mapping of the plant nitrogen uptake from space imaging spectroscopy was developed. The work proposed a hybrid method, which combines the radiative transfer model PROSAIL-PRO and different machine learning regression algorithms (GPR, NN, PLSR, SVR and RF), for the estimation of CCC and CNC as a future proxy for PNU mapping. The use of a hybrid method ensures the exportability of the procedures and a fast processing. Dimensionality reduction methods (PCA) were used to condense the spectral data into components and to analyse their influence on the models. GPR algorithms trained with PCA-20 resulted as the most suitable models for both CCC and CNC. The independent validation against ground data showed good performances: R2 = 0.79, nRMSE = 19% for CCC and R2 = 0.84, nRMSE = 15% for CNC. These models were subsequently applied to PRISMA-like images, acquired in 2018 over the study area, and the relationship between estimated values of CCC and CNC with measured values of PNU was investigated. PNU was slightly more correlated to CNC (R2 = 0.82) than CCC (R2 = 0.80). Therefore, the hybrid model of CNC and the CNC–PNU relation was applied to real PRISMA images, acquired in 2020 over the study area, for the generation of PNU maps.
Result obtained from this study show how crop traits, in particular maize traits, can be estimated from space using data from new-generation hyperspectral sensors. The hybrid approach, which is fully independent from field measurements and datasets, led to good performance and accuracy during the validation phase against the test site data. Moreover, PNU was estimated within the expected ranges, and its dynamics resulted in agreement with the usual plant nitrogen uptake.
Disclosure statement
No potential conflict of interest was reported by the authors.
Additional information
Funding
References
- Baret, F., Houlès, V., & Guérif, M. (2007). Quantification of plant stress using remote sensing observations and crop models: The case of nitrogen management. Journal of Experimental Botany, 58(4), 869–17. https://doi.org/10.1093/jxb/erl231
- Basso, B., Dumont, B., Cammarano, D., Pezzuolo, A., Marinello, F., & Sartori, L. (2016). Environmental and economic benefits of variable rate nitrogen fertilization in a nitrate vulnerable zone. Science of the Total Environment, 545-546, 227–235. https://doi.org/10.1016/j.scitotenv.2015.12.104
- Bender, R. R., Haegele, J. W., Ruffo, M. L., & Below, F. E. (2013). Nutrient uptake, partitioning, and remobilization in modern, transgenic insect-protected maize hybrids. Agronomy Journal, 105(1), 161–170. https://doi.org/10.2134/agronj2012.0352
- Berger, K., Atzberger, C., Danner, M., D’Urso, G., Mauser, W., Vuolo, F., & Hank, T. (2018). Evaluation of the PROSAIL model capabilities for future hyperspectral model environments: A review study. Remote Sensing, 10(1), 85. https://doi.org/10.3390/rs10010085
- Berger, K., Verrelst, J., Féret, J.-B., Hank, T., Wocher, M., Mauser, W., & Camps-Valls, G. (2020). Retrieval of aboveground crop nitrogen content with a hybrid machine learning method. International Journal of Applied Earth Observation and Geoinformation, 92, 102174. https://doi.org/10.1016/j.jag.2020.102174
- Berger, K., Verrelst, J., Féret, J. B., Wang, Z., Wocher, M., Strathmann, M., Danner, M., Mauser, W., & Hank, T. (2020). Crop nitrogen monitoring: Recent progress and principal developments in the context of imaging spectroscopy missions. Remote Sensing of Environment, 242, 111758. https://doi.org/10.1016/j.rse.2020.111758
- Berterretche, M., Hudak, A. T., Cohen, W. B., Maiersperger, T. K., Gower, S. T., & Dungan, J. (2005). Comparison of regression and geostatistical methods for mapping leaf area index (LAI) with Landsat ETM+ data over a boreal forest. Remote Sensing of Environment, 96(1), 49–61. https://doi.org/10.1016/j.rse.2005.01.014
- Borchers, H. W. (2013). pracma: Practical numerical math functions. R package version 1.4.5. Cran.
- Breiman, L. (1996). Bagging predictors. Machine Learning, 24(2), 123–140. https://doi.org/10.1007/bf00058655
- Breiman, L. (2001). Random forests. Machine Learning, 45(1), 5–32. https://doi.org/10.1023/A:1010933404324
- Bruce, L. M., Koger, C. H., & Li, J. (2002). Dimensionality reduction of hyperspectral data using discrete wavelet transform feature extraction. IEEE Transactions on Geoscience and Remote Sensing, 40(10), 2331–2338. https://doi.org/10.1109/TGRS.2002.804721
- Busetto, L., Casteleyn, S., Granell, C., Pepe, M., Barbieri, M., Campos-Taberner, M., Casa, R., Collivignarelli, F., Confalonieri, R., Crema, A., Garcia-Haro, F. J., Gatti, L., Gitas, I. Z., Gonzalez-Perez, A., Grau-Muedra, G., Guarneri, T., Holecz, F., Katsantonis, D., Minakou, C., … Boschetti, M. (2017). Downstream services for rice crop monitoring in Europe: From regional to local scale. IEEE Journal of Selected Topics in Applied Earth Observations and Remote Sensing, 10(12), 5423–5441. https://doi.org/10.1109/JSTARS.2017.2679159
- Candiani, G., Tagliabue, G., Panigada, C., Verrelst, J., Picchi, V., Rivera Caicedo, J. P., & Boschetti, M. (2022). Evaluation of hybrid modeling to estimate chlorophyll and nitrogen content of maize crops in the framework of the future CHIME mission. Remote Sensing, Submitted, 14(8), 1792. https://doi.org/10.3390/rs14081792
- Chabrillat, S., Guanter, L., Segl, K., Foerster, S., Fischer, S., Rossner, G., Schickling, A., Laporta, L., Honold, H. P., & Storch, T. (2020). The enmap German spaceborne imaging spectroscopy mission: Update and highlights of recent preparatory activities. International Geoscience and Remote Sensing Symposium (IGARSS). https://doi.org/10.1109/IGARSS39084.2020.9324006
- Cilia, C., Panigada, C., Rossini, M., Meroni, M., Busetto, L., Amaducci, S., Boschetti, M., Picchi, V., & Colombo, R. (2014). Nitrogen status assessment for variable rate fertilization in maize through hyperspectral imagery. Remote Sensing, 6 (7), 6549–6565. https://doi.org/10.3390/rs6076549
- Clevers, J. G. P. W., & Gitelson, A. A. (2013). Remote estimation of crop and grass chlorophyll and nitrogen content using red-edge bands on sentinel-2 and-3. International Journal of Applied Earth Observation and Geoinformation, 23(1), 344–351. https://doi.org/10.1016/j.jag.2012.10.008
- Cohen, W. B., Maiersperger, T. K., Gower, S. T., & Turner, D. P. (2003). An improved strategy for regression of biophysical variables and Landsat ETM+ data. Remote Sensing of Environment, 84(4), 561–71. https://doi.org/10.1016/S0034-4257(02)00173-6
- Crema, A., Boschetti, M., Nutini, F., Cillis, D., & Casa, R. (2020). Influence of soil properties on maize and wheat nitrogen status assessment from Sentinel-2 data. Remote Sensing, 12 (14), 2175. https://doi.org/10.3390/rs12142175
- Danner, M., Berger, K., Wocher, M., Mauser, W., & Hank, T. (2021). Efficient RTM-based training of machine learning regression algorithms to quantify biophysical & biochemical traits of agricultural crops. ISPRS Journal of Photogrammetry and Remote Sensing, 173, 278–96. https://doi.org/10.1016/j.isprsjprs.2021.01.017
- Darvishzadeh, R., Skidmore, A., Schlerf, M., Atzberger, C., Corsi, F., & Cho, M. (2008). LAI and chlorophyll estimation for a heterogeneous grassland using hyperspectral measurements. ISPRS Journal of Photogrammetry and Remote Sensing, 63(4), 409–426. https://doi.org/10.1016/j.isprsjprs.2008.01.001
- de Grave, C., Verrelst, J., Morcillo-Pallarés, P., Pipia, L., Rivera-Caicedo, J. P., Amin, E., Belda, S., & Moreno, J. (2020). Quantifying vegetation biophysical variables from the Sentinel-3/FLEX tandem mission: Evaluation of the synergy of OLCI and FLORIS data sources. Remote Sensing of Environment, 251, 112101. https://doi.org/10.1016/j.rse.2020.112101
- Delloye, C., Weiss, M., & Defourny, P. (2018). Retrieval of the canopy chlorophyll content from Sentinel-2 spectral bands to estimate nitrogen uptake in intensive winter wheat cropping systems. Remote Sensing of Environment, 216, 245–61. https://doi.org/10.1016/j.rse.2018.06.037
- Féret, J. B., Berger, K., de Boissieu, F., & Malenovský, Z. (2021). PROSPECT-PRO for estimating content of nitrogen-containing leaf proteins and other carbon-based constituents. Remote Sensing of Environment 252 , . https://doi.org/10.1016/j.rse.2020.112173
- Geladi, P., & Kowalski, B. R. (1986). Partial least-squares regression: A tutorial. Analytica Chimica Acta, 185(C), 1–17. https://doi.org/10.1016/0003-2670(86)80028-9
- Guerif, M., Houles, V., & Baret, F. (2007). Remote sensing and detection of nitrogen status in crops. Application to precise nitrogen fertilization. 4th International Symposium on Intelligent Information Technology in Agriculture, ISIITA 2007.
- Hank, T. B., Berger, K., Bach, H., Clevers, J. G. P. W., Gitelson, A., Zarco-Tejada, P., & Mauser, W. (2019). Spaceborne imaging spectroscopy for sustainable agriculture: Contributions and challenges. Surveys in Geophysics, 40(3), 515–551. https://doi.org/10.1007/s10712-018-9492-0
- Hansen, P. M., & Schjoerring, J. K. (2003). Reflectance measurement of canopy biomass and nitrogen status in wheat crops using normalized difference vegetation indices and partial least squares regression. Remote Sensing of Environment, 86(4), 542–53. https://doi.org/10.1016/S0034-4257(03)00131-7
- He, L., Song, X., Feng, W., Guo, B. B., Zhang, Y. S., Wang, Y. H., Wang, C. Y., & Guo, T. C. (2016). Improved remote sensing of leaf nitrogen concentration in winter wheat using multi-angular hyperspectral data. Remote Sensing of Environment, 174, 122–133. https://doi.org/10.1016/j.rse.2015.12.007
- Homolová, L., Malenovský, Z., Clevers, J. G. P. W., García-Santos, G., & Schaepman, M. E. (2013). Review of optical-based remote sensing for plant trait mapping. Ecological Complexity, 15, 1–16. https://doi.org/10.1016/J.ECOCOM.2013.06.003
- Hughes, G. F. (1968). On the mean accuracy of statistical pattern recognizers. IEEE Transactions on Information Theory, 14(1), 55–63. https://doi.org/10.1109/TIT.1968.1054102
- Jacquemoud, S., & Baret, F. (1990). PROSPECT: A model of leaf optical properties spectra. Remote Sensing of Environment, 34(2), 75–91. https://doi.org/10.1016/0034-4257(90)90100-Z
- Jacquemoud, S., Verhoef, W., Baret, F., Bacour, C., Zarco-Tejada, P. J., Asner, G. P., François, C., & Ustin, S. L. (2009). PROSPECT + SAIL models: A review of use for vegetation characterization. Remote Sensing of Environment, 113(SUPPL. 1), S56–S66. https://doi.org/10.1016/j.rse.2008.01.026
- Jonckheere, I., Fleck, S., Nackaerts, K., Muys, B., Coppin, P., Weiss, M., & Baret, F. (2004). Review of methods for in situ leaf area index determination part I. Theories, sensors and hemispherical photography. Agricultural and Forest Meteorology, 121(1–2), 19–35. https://doi.org/10.1016/j.agrformet.2003.08.027
- Julitta, T., Migliavacca, M., & Wutzler, T. (2016). FieldSpectroscopyCC: R package for characterization and calibration of spectrometers; R Package Version 0.5.227; 2016. Download https://rdrr.io/github/tommasojulitta/FieldSpectroscopyCC/.https://rdrr.io/github/tommasojulitta/FieldSpectroscopyCC/
- Kokaly, R. F., Asner, G. P., Ollinger, S. V., Martin, M. E., & Wessman, C. A. (2009). Characterizing canopy biochemistry from imaging spectroscopy and its application to ecosystem studies. Remote Sensing of Environment, 113(SUPPL. 1), S78–S91. https://doi.org/10.1016/j.rse.2008.10.018
- Kuusk, A. (1991). The hot spot effect in plant canopy reflectance. Photon-Vegetation Interactions 139–159 . https://doi.org/10.1007/978-3-642-75389-3_5
- Lassaletta, L., Billen, G., Garnier, J., Bouwman, L., Velazquez, E., Mueller, N. D., & Gerber, J. S. (2016). Nitrogen use in the global food system: Past trends and future trajectories of agronomic performance, pollution, trade, and dietary demand. Environmental Research Letters, 11(9), 095007. https://doi.org/10.1088/1748-9326/11/9/095007
- Leghari, S. J., Wahocho, N. A., Laghari, G. M., HafeezLaghari, A., MustafaBhabhan, G., HussainTalpur, K., Bhutto, T. A., Wahocho, S. A., & Lashari, A. A. (2016, September). Role of nitrogen for plant growth and development : A review. Advan Ces InEnvironmental Biology, 10(9), 209–19.
- Lemaire, G. (2021). Ecophysiology of grasslands: Dynamic aspects of forage plant populations in grazed swards. International Grassland Congress Proceedings. https://uknowledge.uky.edu/igc/19/1/35
- Liu, N., Townsend, P. A., Naber, M. R., Bethke, P. C., Hills, W. B., & Wang, Y. (2021). Hyperspectral imagery to monitor crop nutrient status within and across growing seasons. Remote Sensing of Environment, 255, 112303. https://doi.org/10.1016/j.rse.2021.112303
- Loizzo, R., Ananasso, C., Guarini, R., Lopinto, E., Candela, L., & Pisani, A. R. (2016). The PRISMA hyperspectral mission. European Space Agency, (Special Publication) ESA SP, SP–740.
- Loizzo, R., Daraio, M., Guarini, R., Longo, F., Lorusso, R., DIni, L., & Lopinto, E. (2019). Prisma mission status and perspective. International Geoscience and Remote Sensing Symposium (IGARSS). https://doi.org/10.1109/IGARSS.2019.8899272
- Mojaradi, B., Abrishami-Moghaddam, H., Valadan Zoej, M. J., & Duin, R. P. W. (2009). Dimensionality reduction of hyperspectral data via spectral feature extraction. IEEE Transactions on Geoscience and Remote Sensing, 47(7), 2091–2105. https://doi.org/10.1109/TGRS.2008.2010346
- Morisette, J. T., Baret, F., Privette, J. L., Myneni, R. B., Nickeson, J. E., Garrigues, S., Shabanov, N. V., Weiss, M., Fernandes, R. A., Leblanc, S. G., Kalacska, M., Sánchez-Azofeifa, G. A., Chubey, M., Rivard, B., Stenberg, P., Rautiainen, M., Voipio, P., Manninen, T., Pilant, A. N., & Cook, R. (2006). Validation of global moderate-resolution LAI products: A framework proposed within the CEOS land product validation subgroup. IEEE Transactions on Geoscience and Remote Sensing, 44(7), 1804–17. https://doi.org/10.1109/TGRS.2006.872529
- Nieke, J., & Rast, M. (2019). Status: Copernicus hyperspectral imaging mission for the environment (CHIME). International Geoscience and Remote Sensing Symposium (IGARSS). https://doi.org/10.1109/IGARSS.2019.8899807
- Nutini, F., Confalonieri, R., Crema, A., Movedi, E., Paleari, L., Stavrakoudis, D., & Boschetti, M. (2018). An operational workflow to assess rice nutritional status based on satellite imagery and smartphone apps. Computers and Electronics in Agriculture, 154, 80–92. https://doi.org/10.1016/j.compag.2018.08.008
- Pascual-Venteo, A. B., Portalés, E., Berger, K., Tagliabue, G., Garcia, J. L., Pérez-Suay, A., Rivera-Caicedo, J. P., & Verrelst, J. (2022). Prototyping crop traits retrieval models for CHIME: Dimensionality reduction strategies applied to PRISMA data. Remote Sensing, 14(10), 2448. https://doi.org/10.3390/RS14102448
- Pepe, M., Pompilio, L., Gioli, B., Busetto, L., & Boschetti, M. (2020). Detection and classification of non-photosynthetic vegetation from PRISMA hyperspectral data in croplands. Remote Sensing 2020, 12(23), 3903. https://doi.org/10.3390/RS12233903
- Qi, J., Chehbouni, A., Huete, A. R., Kerr, Y. H., & Sorooshian, S. (1994). A modified soil adjusted vegetation index. Remote Sensing of Environment, 48(2), 119–26. https://doi.org/10.1016/0034-4257(94)90134-1
- Ranghetti, M., Boschetti, M., Gianinetto, M., Tagliabue, G., Panigada, C., & Candiani, G. (2021). Retrieval of maize biophysical variables from multispectral and hyperspectral EO data using a hybrid approach. Planet Care from Space, AIT Series: Trends in Earth Observation, 2, 129–132 DOI: 10.978.88944687/00.
- Ranghetti, L., Boschetti, M., Nutini, F., & Busetto, L. (2020). “sen2r”: An R toolbox for automatically downloading and preprocessing Sentinel-2 satellite data. Computers and Geosciences 139 , . https://doi.org/10.1016/j.cageo.2020.104473
- Ranghetti, L., Nutini, F., Cillis, D., & Boschetti, M. (2021). A reproducible workflow to derive crop phenology and agro-practice information from Sentinel-2 time series: A case study for Sardinia cropping systems. Planet Care from Space, AIT Series: Trends in Earth Observation, 2, 133–136 dDOI: 10.978.88944687/00.
- Rasmussen, C. E., & Williams, C. K. I. (2006). Gaussian processes for machine learning . In The MIT press. (Vols. 2 (MIT press Cambridge, MA) 3).
- Rast, M., Ananasso, C., Bach, H., Ben-Dor, E., Chabrillat, S., Colombo, R., Del Bello, U., Feret, J. B., Giardino, C., Green, R. O., Guanter, L., Marsh, S., Nieke, J., Ong, C. C. H., Rum, G., Schaepman, M. E., Schlerf, M., Skidmore, A. K., & Strobl, P. (2019). Copernicus hyperspectral imaging mission for the environment: Mission requirements document (2.1, Issue ESA-EOPSM-CHIM-MRD-3216). European Space Agency (ESA).
- Ritchie, S., Hanway, J., & Benson, G. (1989). How a corn plant develops. Ames: Iowa State University of Science and Technology Cooperative Extension Service, 48.
- Rivera-Caicedo, J. P., Verrelst, J., Muñoz-Marí, J., Camps-Valls, G., & Moreno, J. (2017). Hyperspectral dimensionality reduction for biophysical variable statistical retrieval. ISPRS Journal of Photogrammetry and Remote Sensing, 132, 88–101. https://doi.org/10.1016/J.ISPRSJPRS.2017.08.012
- Schlemmera, M., Gitelson, A., Schepersa, J., Fergusona, R., Peng, Y., Shanahana, J., & Rundquist, D. (2013). Remote estimation of nitrogen and chlorophyll contents in maize at leaf and canopy levels. International Journal of Applied Earth Observation and Geoinformation, 25(1), 47–54. https://doi.org/10.1016/j.jag.2013.04.003
- Schmidhuber, J. (2015). Deep learning in neural networks: An overview. Neural Networks, 61, 85–117. https://doi.org/10.1016/j.neunet.2014.09.003
- Siegmann, B., Alonso, L., Celesti, M., Cogliati, S., Colombo, R., Damm, A., Douglas, S., Guanter, L., Hanuš, J., Kataja, K., Kraska, T., Matveeva, M., Moreno, J., Muller, O., Pikl, M., Pinto, F., Vargas, J. Q., Rademske, P., Rodriguez-Morene, F. and Rascher, U. (2019). The high-performance airborne imaging spectrometer HyPlant—from raw images to top-of-canopy reflectance and fluorescence products: Introduction of an automatized processing chain. Remote Sensing 2019, 11(23), 2760. https://doi.org/10.3390/RS11232760
- Stroppiana, D., Boschetti, M., Brivio, P. A., & Bocchi, S. (2009). Plant nitrogen concentration in paddy rice from field canopy hyperspectral radiometry. Field Crops Research, 111(1–2), 119–29. https://doi.org/10.1016/j.fcr.2008.11.004
- Tagliabue, G., Boschetti, M., Bramati, G., Candiani, G., Colombo, R., Nutini, F., Pompilio, L., Rivera-Caicedo, J. P., Rossi, M., Rossini, M., Verrelst, J., & Panigada, C. (2022). Hybrid retrieval of crop traits from multi-temporal PRISMA hyperspectral imagery. ISPRS Journal of Photogrammetry and Remote Sensing 187 , 236–377. https://doi.org/10.1016/j.isprsjprs.2022.03.014
- Team, R. C. (2021). R: A language and environment for statistical computing v. 3.6. 1. Scientific Reports, (R Foundation for Statistical Computing, Vienna, Austria, 2019). 11.
- Thompson, D. R., Schimel, D. S., Poulter, B., Brosnan, I., Hook, S. J., Green, R. O., Glenn, N., Guild, L., Henn, C., Cawse-Nicholson, K., Kokaly, R., Lee, C., Luvall, J., Miller, C. E., Nastal, J., Pavlick, R., Phillips, B., Schneider, F., Uz, S. S., & Wang, W. (2020). NASA’s surface biology and geology concept study: Status and next steps. International Geoscience and Remote Sensing Symposium (IGARSS). https://doi.org/10.1109/IGARSS39084.2020.9323295
- Tian, Y. C., Yao, X., Yang, J., Cao, W. X., Hannaway, D. B., & Zhu, Y. (2011). Assessing newly developed and published vegetation indices for estimating rice leaf nitrogen concentration with ground- and space-based hyperspectral reflectance. Field Crops Research, 120(2), 299–310. https://doi.org/10.1016/j.fcr.2010.11.002
- Trujillo-Ortiz, A., & Hernandez-Walls, R. (2010). gmregress: Geometric Mean Regression (Reduced Major Axis Regression). http://www.mathworks.com/matlabcentral/fileexchange/27918-gmregress
- Upreti, D., Huang, W., Kong, W., Pascucci, S., Pignatti, S., Zhou, X., Ye, H., & Casa, R. (2019). A comparison of hybrid machine learning algorithms for the retrieval of wheat biophysical variables from Sentinel-2. Remote Sensing, 11 (5), 481. https://doi.org/10.3390/rs11050481
- Verhoef, W. (1984). Light scattering by leaf layers with application to canopy reflectance modeling: The SAIL model. Remote Sensing of Environment, 16(2), 125–41. https://doi.org/10.1016/0034-4257(84)90057-9
- Verhoef, W., Jia, L., Xiao, Q., & Su, Z. (2007). Unified optical-thermal four-stream radiative transfer theory for homogeneous vegetation canopies. IEEE Transactions on Geoscience and Remote Sensing, 45(6), 1808–1822. https://doi.org/10.1109/TGRS.2007.895844
- Verrelst, J., Berger, K., & Rivera-Caicedo, J. P. (2020). Intelligent sampling for vegetation nitrogen mapping based on hybrid machine learning algorithms. IEEE Geoscience and Remote Sensing Letters. https://doi.org/10.1109/lgrs.2020.3014676
- Verrelst, J., Camps-Valls, G., Muñoz-Marí, J., Rivera, J. P., Veroustraete, F., Clevers, J. G. P. W., & Moreno, J. (2015). Optical remote sensing and the retrieval of terrestrial vegetation bio-geophysical properties – A review. ISPRS Journal of Photogrammetry and Remote Sensing, 108, 273–290. https://doi.org/10.1016/j.isprsjprs.2015.05.005
- Verrelst, J., Dethier, S., Rivera, J. P., Munoz-Mari, J., Camps-Valls, G., & Moreno, J. (2016). Active learning methods for efficient hybrid biophysical variable retrieval. IEEE Geoscience and Remote Sensing Letters, 13(7), 1012–1016. https://doi.org/10.1109/LGRS.2016.2560799
- Verrelst, J., Malenovský, Z., van der Tol, C., Camps-Valls, G., Gastellu-Etchegorry, J. P., Lewis, P., North, P., & Moreno, J. (2019). Quantifying vegetation biophysical variables from imaging spectroscopy data: A review on retrieval methods. Surveys in Geophysics, 40(3), 589–629. https://doi.org/10.1007/s10712-018-9478-y
- Verrelst, J., Rivera-Caicedo, J. P., Reyes-Muñoz, P., Morata, M., Amin, E., Tagliabue, G., Panigada, C., Hank, T., & Berger, K. (2021). Mapping landscape canopy nitrogen content from space using PRISMA data. ISPRS Journal of Photogrammetry and Remote Sensing 178 , 382–395. https://doi.org/10.1016/j.isprsjprs.2021.06.017
- Verrelst, J., Rivera, J. P., Alonso, L., & Moreno, J. (2011). ARTMO: An automated radiative transfer models operator toolbox for automated retrieval of biophysical parameters through model inversion. Proceedings of EARSeL 7th SIG-Imaging Spectroscopy Workshop, Edinburgh, UK.
- Verrelst, J., Rivera, J. P., Gitelson, A., Delegido, J., Moreno, J., & Camps-Valls, G. (2016). Spectral band selection for vegetation properties retrieval using Gaussian processes regression. International Journal of Applied Earth Observation and Geoinformation, 52, 554–567. https://doi.org/10.1016/J.JAG.2016.07.016
- Wang, S., Guan, K., Wang, Z., Ainsworth, E. A., Zheng, T., Townsend, P. A., Liu, N., Nafziger, E., Masters, M. D., Li, K., Wu, G., & Jiang, C. (2021). Airborne hyperspectral imaging of nitrogen deficiency on crop traits and yield of maize by machine learning and radiative transfer modeling. International Journal of Applied Earth Observation and Geoinformation 105 , 102617. https://doi.org/10.1016/j.jag.2021.102617
- Wang, Z., Skidmore, A. K., Darvishzadeh, R., Heiden, U., Heurich, M., & Wang, T. (2015). Leaf nitrogen content indirectly estimated by leaf traits derived from the PROSPECT model. IEEE Journal of Selected Topics in Applied Earth Observations and Remote Sensing, 8(6), 3172–3182. https://doi.org/10.1109/JSTARS.2015.2422734
- Wang, Z., Skidmore, A. K., Darvishzadeh, R., & Wang, T. (2018). Mapping forest canopy nitrogen content by inversion of coupled leaf-canopy radiative transfer models from airborne hyperspectral imagery. Agricultural and Forest Meteorology 253–254 , 247–260. https://doi.org/10.1016/j.agrformet.2018.02.010
- Wang, Z., Skidmore, A. K., Wang, T., Darvishzadeh, R., & Hearne, J. (2015). Applicability of the PROSPECT model for estimating protein and cellulose + lignin in fresh leaves. Remote Sensing of Environment, 168, 205–218. https://doi.org/10.1016/J.RSE.2015.07.007
- Weiss, M. (2006). Valeri LAI-2000 data processing. INRA, Avignon.
- Weiss, M., Baret, F., & Jay, S. (2020). S2ToolBox level 2 products: LAI, FAPAR, FCOVER.(Version 2.0). Institut National de La Recherche Agronomique (INRA).
- Weiss, M., Baret, F., Smith, G. J., Jonckheere, I., & Coppin, P. (2004). Review of methods for in situ leaf area index (LAI) determination part II. Estimation of LAI, errors and sampling. Agricultural and Forest Meteorology, 121(1–2), 19–35. https://doi.org/10.1016/j.agrformet.2003.08.001
- Weiss, M., Jacob, F., & Duveiller, G. (2020). Remote sensing for agricultural applications: A meta-review. Remote Sensing of Environment, 236, 111402. https://doi.org/10.1016/j.rse.2019.111402
- Zarco-Tejada, N., & Loudjani, P. (2017). Precision agriculture: An opportunity for EU farmers. Potential Support with the Cap 2014–2020 (EPRS: European Parliamentary Research Service).
- Zhang, F., & O’Donnell, L. J. (2020). Support vector regression. Machine Learning: Methods and Applications to Brain Disorders, 123–140. https://doi.org/10.1016/B978-0-12-815739-8.00007-9
- Zheng, H., Li, W., Jiang, J., Liu, Y., Cheng, T., Tian, Y., Zhu, Y., Cao, W., Zhang, Y., & Yao, X. (2018). A comparative assessment of different modeling algorithms for estimating leaf nitrogen content in winter wheat using multispectral images from an unmanned aerial vehicle. Remote Sensing, 10(12), 2026. https://doi.org/10.3390/rs10122026
- Zhou, X., Zhang, J., Chen, D., Huang, Y., Kong, W., Yuan, L., Ye, H., & Huang, W. (2020). Assessment of leaf chlorophyll content models for winter wheat using Landsat-8 multispectral remote sensing data. Remote Sensing, 12 (16), 2574. https://doi.org/10.3390/RS12162574