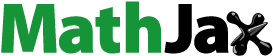
ABSTRACT
Earth observation techniques represent a reliable and faster alternative to in-situ measurements by providing spatio-temporal information on crop status. In this framework, a study was conducted to assess the performance of hybrid approaches, either standard (HYB) or exploiting an active learning optimisation strategy (HYB-AL), to estimate leaf area index (LAI) and canopy nitrogen content (CNC) from Sentinel–2 (S2) data, in rice crops. To achieve this, the PROSAIL-PRO Radiative Transfer Model (RTM) was tested. Results demonstrate that a wide range of rice spectra, simulated according to realistic crop parameters, are reliable when appropriate field background conditions are considered. Simulations were used to train a Gaussian Process Regression (GPR) algorithm. Both cross-validation and validation results showed that HYB-AL approach resulted the best performing retrieval schema. LAI estimation achieved good performance (R2=0.86; RMSE=0.54) and resulted very promising for model application in operational monitoring systems. CNC estimations showed moderate performance (R2=0.63; RMSE=0.89) due to a saturation behaviour limiting the retrieval accuracy for moderate/high CNC values, approximately above 4 [g m−2]. S2 maps of LAI and CNC provided spatio-temporal information in agreement with crop growth, nutritional status and agro-practices applied to the study area, resulting in an important contribution to precision farming applications.
Introduction
In the pre-industrial period, worldwide production and consumption of food happened parallel to each other. Nowadays, the global megatrends (climate change, population growth, technological change) gradually caused the supply-demand balance to shift towards a not sufficient and unsustainable food production, with a potentially dramatic consequence for environmental and humanitarian aspects (Hank et al., Citation2019). Considering this scenario, farmers are forced to increase yields while protecting their most important production factors: soil from degradation, water and air from pollution and atmosphere from emissions of greenhouse gases (FAO Citation2016).
Remote sensing (RS) is recognised as an essential source of data that can contribute to improve agricultural activities and farm management (Weiss et al., Citation2020). In particular to support agro practices RS data can provide: (i) Continuous traits monitoring during all development plant stages related to crop development and growth (e.g. Leaf Area Index (LAI)); (ii) Information on photosynthetic efficiency and early crop stress detection (e.g. leaf chlorophyll content and canopy chlorophyll content); (iii) Indication of plant nutrition status to highlight nitrogen deficiency (e.g. leaf nitrogen content (LNC) and canopy nitrogen content (CNC)).
In developed and industrialised countries, geoinformation products can be used to provide farmers with decision-supporting spatial information (crop traits maps), able to highlight within-field crop variability, as a fundamental tool to support site-specific management (i.e. precision farming; Hank et al., Citation2019; Zarco-Tejada et al., Citation2014). Among the agro-practices supported by precision farming, Nitrogen (N) fertilisations are fundamental, being nitrogen the most critical macro-nutrient for vegetation growth and crop productivity. Due to its overall positive effect on crop production, the use of nitrogen fertilisers increased worldwide in the last decades. However, a general decreasing trend in nitrogen use efficiency is evident, particularly in Europe and North America. Overall, the nitrogen use efficiency in agriculture is estimated at 60%, with a negative effect on the sustainability of crop production from an economic and ecological point of view (Lassaletta et al., Citation2016). For this reason, fertilisation must be supported by rational approaches able to consider the real plant needs. That minimises both under-fertilisation, which can decrease crop yield, and over-fertilisation, leading to plant stress (diseases or lodging) and environmental pollution (groundwater leaching or gas emission; Paleari et al., Citation2019; Zhang et al., Citation2020).
In this context, sustainable agriculture and smart fertilisation strategy should be achieved by exploiting decision support systems based on spatial explicit information on crop nutritional status. The dilution curve approach provides a helpful theoretical framework to assess actual crop nitrogen needs by considering crop growth conditions (i.e. accumulated biomass or reached LAI values) and the corresponding Plant Nitrogen Uptake (PNU) with respect to a reference critical curve (Lemaire et al., Citation2021). Such approach allows the spatial and temporal assessment of actual plant nutritional condition (i.e. N deficiency or luxury consumption), required to estimate actual crop needs (i.e. indicator of fertilisation amount). In particular, the plant nutritional status can be estimated using LAI and PNU (or the organ specific part related to leaves, Canopy Nitrogen Content – CNC) retrieved by remote sensing data (Baret et al., Citation2007; Delloye et al., Citation2018).
Several methods to estimate LAI and CNC from spectral data were proposed in the literature. They can be grouped into the following main categories:
Data-driven methods exploit the full spectral information, or data transformation (such as principal component analysis, derivative analysis, or vegetation indices computation), and ground data through parametric or non-parametric Machine Learning Regression Algorithms (MLRAs);
Physically based methods, using a minimisation procedure or a hybrid approach, invert radiative transfer models (RTMs) to simulate plant spectral response according to crop parameters variability and soil background properties.
The hybrid approach (HYB) is proposed in scientific literature as the state-of-art. It is an innovative solution able to combine RTMs, able to generate a database of crop parameters and spectra for a wide range of conditions, with flexible and computationally efficient MLRAs, for an efficient solution to the inversion problem. For a detailed description of available methods, see Verrelst et al. (Citation2019). These approaches have been already demonstrated to be efficient to estimate LAI from multispectral data, in different contexts and cropping systems (M. Campos-Taberner et al., Citation2016b; Delloye et al., Citation2018).
Concerning plant nitrogen, the most popular approaches are data-driven methods using parametric regressions and narrowband hyperspectral vegetation indices (Clevers & Gitelson, Citation2013; Fava et al., Citation2009; Hansen & Schjoerring, Citation2003; Schlemmer et al., Citation2013; Daniela Stroppiana et al., Citation2009), or more advanced approaches based on machine learning regression algorithms, such as Partial Least Squares Regression – PLSR (Hansen & Schjoerring, Citation2003; Liu et al., Citation2021; Wang et al., Citation2021). Recently, the new PROSPECT-PRO model (Féret et al., Citation2021) allowed researchers to assess the effect of protein content and carbon-based constituents on leaf spectra, providing a physically-based theoretical framework for crop nitrogen retrieval by means of modelling, overcoming the traditional empirical data-driven approaches. Once coupled with a canopy model (i.e. SAIL4, Verhoef, Citation1984), it is possible to assess the plant-level effect of nitrogen content on crop spectra. Through the inversion of such leaf-canopy radiative transfer model (PROSAIL-PRO), it is theoretically possible to estimate CNC on a fully physical base using a hybrid approach, as recently demonstrated with field data of wheat and corn (Berger et al., Citation2020a) and PRISMA satellite images (Tagliabue et al., Citation2022; Verrelst et al., Citation2021a).
In this context, the objective of this study is to test an hybrid approach (HYB) to estimate LAI and CNC in rice cropping systems from Sentinel-2 data. This goal is achieved through the following steps:
Evaluation of the PROSAIL-PRO model to reproduce rice spectra, in relation to different background conditions by comparing model simulations with field spectra;
Development of hybrid models for Sentinel −2, exploiting field data from a controlled experiment, by testing (i) a standard approach based on a Look-Up-Table (LUT) generated from the RTM forward simulation, and (ii) optimisation procedures through Active Learning (AL) to select the most significative spectra in the LUT;
Assessment of LAI and CNC maps, generated by the best retrieval model using Sentinel-2 and ground data, acquired in real farming conditions, for precision farming activities.
Materials
Overall study workflow
provides a schematic representation of the methodological study phases.
In Phase #1, the potentiality of the PROSAIL-PRO model to simulate paddy rice spectra, according to crop parameters variability and considering the background influence, was assessed. Data from controlled field experiments were used, including spectroradiometric and crop traits measurements acquired along the crop season.
In Phase #2, hybrid models were developed to retrieve the biophysical parameters from Sentinel-2 spectral configuration. An AL procedure was also used to optimise the spectra selection from the simulated database. The results were validated using independent datasets to evaluate the model exportability in real operational conditions.
In Phase #3, multitemporal maps of LAI and CNC were generated from Sentinel-2 data, on the study area (North of Italy rice district). This phase aimed to assess the added value of produced data in providing spatial and temporal agronomic information on crop growth and status. The assessment of traits’ maps capability in highlighting within-field variability investigation was based on available information on (i) ground data collected in different farms for a district-level analysis and (ii) prescription maps used for variable-rate nitrogen fertilisation for a field level analysis. Trait maps were analysed in terms of spatial and temporal information provided for rice crop monitoring and discussed as a contribution in precision farming applications.
Study area and crop significance
Rice is cultivated worldwide and represents a key crop to provide food security in many countries. In Europe, rice is cultivated along the Mediterranean area, and Italy contributes to almost 50% of total European production (Agri-food Data Portal, Citation2019). shows the rice district in North of Italy, an area of more than 210000 ha between Lombardia and Piemonte. The locations of field experiments (red dot) and of the Lomellina area (red box), used for mapping demonstration, are depicted .
Figure 2. Panel a: North of Italy rice district covering more than 210000 ha of cultivated land (light green). The red circle points at the field experiments (Opera: latitude 45°23’, longitude 9°11’). Panel b: study area indicating test farms (green polygons) and monitored fields (Orange polygons). Panel c: field where it was compared variable rate technology (dark red) and standard fertilisation (pink).

In this intensively cultivated area, a test of precision farming fertilisation supported by satellite information and ground measurements was conducted in 2018 (SATURNO project). The selected fields were split into two halves and managed with Variable Rate Technology (VRT) and traditional uniform (Standard) fertilisation. Management Unit Zone (MUZ) were identified by statistical segmentation of vegetation index maps derived from Sentinel-2 images acquired few days before fertilisation. The specific fertilisation dose for VRT treatments were defined according to crop conditions assessed in field with a smart scouting approach within the identified MUZ. Details on the experimental fields, ground measurements and precision farming fertilisation are described in Nutini et al. (Citation2021). shows one of the monitored field where both VRT fertilisation (red) and standard fertilisation (pink) were tested.
Datasets exploited in the study
This study exploited two datasets available from previous research: (i) ground measurements collected in 2004 and 2006 on a plot level experiment and (ii) ground measurements and ancillary information collected in 2018 for several fields on a wide area for real farming condition monitoring.
Plot-level experimental dataset
Controlled plot-level experiments were conducted in 2004 and 2006 (red dot in ). Full resolution FieldSpec® spectra, plant traits measurements and crop parameters were collected for different rice varieties in different phenological stages during the crop season and managed with different fertilisation conditions (5 and 4 fertilisation levels in 2004 and 2006, respectively). Crop parameters included LAI, plant nitrogen concentration (PNC), leaf mass area (LMA), SPAD unit and biomass (). These experiments were conducted using two different rice sowing techniques: traditional broadcast sowing in flooded conditions (2004) and modern drill sowing in dry soil (2006). During the field campaigns, several reflectance spectra of paddy soil in different conditions (dry, humid and flooded) were measured with FieldSpec®. Besides the effect on crop growth, the collected data assessed the influence of different background conditions on crop spectral measurements during crop growth. The literature indicated this aspect as very important for RTM simulation (Adeluyi et al., Citation2021; M. Campos-Taberner et al., Citation2016a). A detailed description of experimental conditions and protocol for ground and proximal sensing measurements are described in (Stroppiana et al., Citation2009). summarises the experimental field data used to assess the PROSAIL-PRO RTM simulation and set up the hybrid retrieval model.
Table 1. Summary of plot-level experimental field data.
Real farming condition dataset
A dataset of field measurements acquired in real farming conditions was collected in 2018, in the framework of the SATURNO project (Nutini et al., Citation2021). On-site data were measured in three selected farms (see, ) during five field campaigns (14 June, 4 July, 11 July, 23 July, 2 August). Two fields were selected in each farm and for each field, ground data were measured in six Elementary Sampling Units (ESUs) with the size of 5 m x 5 m. The location of the ESUs was selected according to vegetation patterns identified, before each campaign measurements, using vegetation index map generated with the most recent cloud-free Sentinel-2 image (see below for the description of the satellite data).
For each ESU, LAI and Nmass were estimated using the smartphone apps PocketLAI (Confalonieri et al., Citation2013) and PocketN (Paleari et al., Citation2019) as the average of five measurements (four at the vertices and one at the centre of each ESU). In two campaigns (14 June and 11 July), destructive plant samples (20 plants) and plant density measurements were also performed to estimate above-ground biomass needed to calculate PNU. Overall, 180 samples were available for LAI and Nmass (3 farms x 2 fields x 6 ESUs x 5 dates). Seventy-two samples were instead available for PNU (3 farms x 2 fields x 6 ESUs x 2 dates). Digital fertilisation prescription maps were also available to analyse the spatio-temporal consistency of CNC estimates produced by Sentinel 2 data with observed crop dynamics (see below for the description of the satellite data). summarises the objectives of the study phases.
Table 2. Summary of the objectives of the study phases and exploited data set (n.a. = not available).
On-site CNC measures to validate the model retrieval were available only for 2006. Thus, PNU data from the 2004 and 2018 experiments were also used. It is worth mentioning that while CNC and PNU refer to different quantities of nitrogen (CNC is the nitrogen accumulated in the leaves only and PNU is the total nitrogen uptake stored in all the plant organs), in the first development stages, when stems are not fully developed and grains are not yet present, CNC and PNU are comparable. Thus PNU is a good proxy for CNC in early phenological stages.
Satellite images
Nine Sentinel-2 level-2A images were available for the crop season (1 June, 16 June, 21 June, 26 June, 1 July, 6 July, 26 July, 31 July, 5 August), and they were downloaded with the Sen2r application (Ranghetti et al., Citation2020). Sen2cor Scene Classification Map layer was used to mask clouds and shadow. The whole time series was used to perform mapping demonstrations and assess the contribution of Earth Observation in precision farming activities. Moreover, five Sentinel-2 scenes out of nine were imaged during ground measurements. They were used to assess the hybrid model performance with independent data.
Investigated crop traits
The following parameters were investigated for the calibration and validation of the hybrid model:
Leaf area index: LAI [m2 m−2] was available as field indirect measurements using LAI2000 Licor Plant Analyser for 2004 (48 samples) and 2006 (52 samples). For 2018, LAI was available through the smartphone app PocketLAI (180 samples);
Canopy nitrogen content: CNC [
] was computed for 2006 (52 samples) as follows:
where Nmass [%], LAI [m2. m−2], LMA [g cm−2]. and 100 to convert units to [g m−2].
Methods
Phase #1: Simulation of rice spectra
The PROSAIL-PRO model has been widely used to obtain plant biochemical and structural variables in the agricultural context (Berger et al., Citation2018). It combines the PROSPECT leaf model (Jacquemoud & Baret, Citation1990) and the 4SAIL canopy model (Verhoef, Citation1984). Specifically, the PROSPECT model requires a small number of biophysical and biochemical input parameters and calculates the radiative interactions at leaf level producing continuous leaf reflectance and transmittance spectra in the 400–2,500 nm optical domain.
The most recent version, called PROSPECT-PRO, introduced specific absorption coefficients to differentiate between carbon-based (CBC) and protein-based (CP) leaf constituents (Féret et al., Citation2021). On the other hand, 4SAIL requires as input the leaf reflectance and transmittance, canopy density information (i.e. LAI), leaf orientation (average leaf angle – ALA), background spectral properties and the illumination and viewing angles.
Model parameterisation
PROSAIL-PRO simulations were performed with MATLAB routines implemented to couple PROSPECT-PRO with 4SAIL. Simulated spectra were compared to ground spectral measurements according to measured crop parameters and background conditions.
The input parameters of PROSAIL-PRO are listed in . These data include the crop parameters acquired during field measurements. Missing data were replaced with average literature values reported for rice (see, legend for details).
Table 3. PROSAIL-PRO model parameters used for rice simulation and LUT generation. Values are from: measured (M) or estimated (E) ground data and literature (L). For LUT generation, Probability Density Function (PDF) and value ranges (min-max) are reported. Legend: 1 Manuel Manuel Campos-Taberner et al. (Citation2016); 2 Boschetti et al. (Citation2006); 3 Danner et al. (Citation2019); 4 Katja Berger et al. (Citation2018); 5 HYB PDF is the Probability Density Function for the parameter’s simulation; 6 min and max values in case of Uniform PDF, μ and σ values in case of Normal PDF; 7 S1 = Dry soil condition, S2 = Humid soi condition, S3 = Flooded soil condition (see, ).
Figure 3. Measured background spectra given as input to the PROSAIL-PRO model for 2004 and 2006 datasets. Soil legend: S1 = Dry soil condition, S2 = Humid soi condition, S3 = Flooded soil condition.

. PROSAIL-PRO model parameters used for rice simulation and LUT generation. Values are from: measured (M) or estimated (E) ground data and literature (L). For LUT generation, Probability Density Function (PDF) and value ranges (min-max) are reported. Legend: 1 Manuel Manuel Campos-Taberner et al. (Citation2016); 2 Boschetti et al. (Citation2006); 3 Danner et al. (Citation2019); 4 Katja Berger et al. (Citation2018); 5 HYB PDF is the Probability Density Function for the parameter’s simulation; 6 min and max values in case of Uniform PDF, μ and σ values in case of Normal PDF; 7 S1 = Dry soil condition, S2 = Humid soi condition, S3 = Flooded soil condition (see, ).
Leaf and canopy parameters
The new PROSAIL-PRO estimates LMA as the sum of proteins (Cp) and carbon-based constituents (CBC) that includes cellulose, lignin, hemicellulose, and starch (Féret et al., Citation2021). For model simulation, Cp was calculated from leaf nitrogen content using the protein-to-nitrogen conversion factor of 4.43, as shown in the following equations:
The structure parameter (N) was calculated using the following equation (Danner et al., Citation2019):
The leaf equivalent water thickness parameter (EWT or ) was tied to the dry matter content following the equation proposed by Manuel Campos-Taberner et al. (Citation2016).
According to Baret et al. (Citation2007), green leaves have a relative water content () varies within a relatively small range. We considered
as mean value of the reported ones (min = 0.6 max = 0.8) by Manuel Campos-Taberner et al. (Citation2016).
For the 2006 dataset, SPAD chlorophyll readings were converted to physical values of leaf chlorophyll content, according to Equation 8 (Manuel Campos-Taberner et al., Citation2016):
LAI values were set according to available LAI200 measurements (see “investigated crop traits”). ALA values for the two considered varieties and phenological stages were derived from LAI2000 mean tilt angle estimates of the 2006 dataset (Boschetti et al., Citation2006). In contrast, for the 2004 dataset was used an average value from literature.
Background reflectance
The reflectance spectra of dry, humid and flooded paddy soil, collected on-site during 2004 and 2006 campaigns, were post-processed to smooth atmospheric disturbances registered in three spectral regions (1,350–1,425 [nm]; 1,800–1,975 [nm]; 2,350–2,500 [nm]). Spectra were interpolated using a spline smoothing fitting with 60 degrees of freedom SplineSmoothGapfilling in the Field Spectroscopy CC package (Wutzler, Migliavacca, and Julitta Citation2016; ).
Viewing geometry parameters
The Sun Zenith Angle (SZA) was computed as follows:
where the Elevation values were obtained from the NOAA Solar Calculator (NOAA, Global Positioning Laboratory, Citationn.d.), by providing location coordinates, date and time of the measurements.
The skyl parameter describes the ratio of diffuse to total incident radiation. It was calculated for an average mid-latitude atmosphere using the following formula (Danner et al., Citation2019):
Phase #2: Development of the hybrid model
Hybrid methods for biophysical variables retrieval use machine learning regression algorithms (MLRAs) trained with spectra simulated by physically-based radiative transfer models (RTMs). Since RTMs simulate spectra from a wide range of leaf and canopy parameters, the MLRA training is more generalised compared to those carried out using only measured data. The input parameters used in this study (see, ) were randomly sampled from probability density functions with ranges set according to:
Realistic ranges of crop biophysical variables measured during the experimental field campaign of 2006;
Reference data from literature (Manuel Campos-Taberner et al., Citation2016);
Range of different backgrounds (dry/wet soil and flooded).
All the 1-nm spectra generated by running the PROSAIL-PRO model in the direct mode were resampled to the Sentinel-2 spectral configuration. Band #2, corresponding to blue spectral range 458–523 [nm], was excluded as residual atmospheric attenuation may lead to bad retrieval results, as suggested by ESA SENTINEL2 Toolbox ATBD (Weiss & Baret, Citation2016) and confirmed by recent works (Adeluyi et al., Citation2021; Delloye et al., Citation2018; Upreti et al., Citation2019).
Finally, LAI, CNC and corresponding Sentinel-2-like spectra were organised in a Look Up Table (LUT) of 2,000 records. This cardinality is a trade-off between training computational cost and characterisation of different crop conditions (Verrelst et al., Citation2020).
The LUT was used to train a Gaussian Process Regression (GPR) algorithm, one of the most promising kernel-based ML methods for vegetation properties retrieval, with the advantage of providing uncertainty estimates on the predictions (Verrelst et al., Citation2020). As proposed in other works (Verrelst et al., Citation2020), 5% Gaussian noise was added to all the input parameters before model training to generalise the model and prevent overfitting issues. This standard configuration of the hybrid model is referred to as HYB in the following sections.
The Active learning (AL) approach was also tested to reduce the size of the LUT by optimally selecting samples from the larger data pool. This optimisation procedure is proposed in the literature to improve model performance through an intelligent sampling of training data which compares results with available ground truth data. According to the state-of-the-art literature, this approach provided promising results (Berger et al., Citation2021; Verrelst et al., Citation2020).
Following the approach proposed in Verrelst et al. (Citation2020),we used the Kernel Ridge Regression (KRR) algorithm to select an optimal subset of the training data. Thus, 1% of the simulated spectra (20 samples) were randomly selected and used as the initial training. At each iteration, a new spectrum was added to the previous set, according to heuristics based on diversity or uncertainty criteria, and a new model is trained: if the new sample improves the model validation statistics, it is kept in the training pool, otherwise it is rejected. In this study, five different diversity and uncertainty criteria where tested: Euclidean distance-based diversity (EBD), angle-based diversity (ABD) cluster-based diversity (CBD), variance-based pool of regressors (PAL), and residual regression AL (RSAL). This process was iterated to test the contribution of all the simulated spectral signatures, leading to a reduced LUT used to train the GPR model. This configuration of hybrid model exploiting an optimised LUT is referred as HYB-AL in the following.
Training performance of HYB and HYB-AL methods were assessed through a cross-validation procedure with a random partitioning of observations in 10 subsets (k-fold strategy). The best models were then validated against independent dataset from both controlled field experiment and real farm conditions. In particular, LAI estimations were validated exploiting ground measurements from 2004, 2006 and 2018 dataset. CNC estimations were validated against 2006 data and compared to PNU data for 2004 and 2018 dataset (see, ). Both HYB and HYB-AL model training were performed using the Automated Radiative Transfer Models Operator (ARTMO) toolbox for MATLAB (Verrelst et al., Citation2011).
Phase #3: Map generation and assessment of information content
The hybrid models identified in phase #2 were applied to the Sentinel-2 time series. Maps of LAI and CNC were generated with the ARTMO toolbox and evaluated with QGIS. According to the ancillary information available at district level for several fields from different farms, temporal trends of LAI maps were discussed. In particular, LAI temporal profiles for the analysed farm were investigated in relation to cultivated varieties and sowing dates. CNC maps, considered an important input for fertilisation managed with VRT technologies, were investigated by extracting their temporal profiles from sample areas in a field for which different fertilisation management were performed according to defined prescription maps.
Results
Phase #1: Simulation of rice spectra
provides an example of seasonal rice spectral dynamics for the crop sown in flooded conditions (2004) and cultivated with dry sowing practice (2006). Overall, the simulated spectra with PROSAIL-PRO (blue lines) agree with the field measurements (orange lines). The influence of soil conditions on crop reflectance is mostly evident at early stage of rice development in field data, as supposed, and it is well reproduced by RTM simulation when specific background is used.
Figure 4. Examples of dynamics of measured field spectra (Orange) and simulated spectra (blue) for 2004 (upper graph) and experimental dataset (lower graph). LAI values, Days After Sowing (DAS) and Development Stage (DVS) are reported to describe plant growth. Legend: TrS = Transplantation Stage; TiS = Tillering Stage; SE = Stem Elongation; PI = Panicle Initiation; HS = Heading Stage; FS = Flowering Stage; DS = Dough Stage; MS = Mature Stage.

reports the Mean Absolute Error (MAE) graphs between the simulated spectra and field measurements for all the samples available in 2004 (48) and 2006 (87). This analysis was performed to assess the influence of different soil conditions when are used as simulation input (S1 = Dry soil; S2 = Humid soil; S3 = Flooded soil). When using only dry soil spectra (S1) as background for all simulation across the season, the data from 2004 (broadcast sowing in flooded conditions) and 2006 (modern drill sowing in dry soil condition) showed a mean absolute error (MAE) greater than 20% and 10%, respectively. Differently, the MAE was between 5% and 10% when using only humid (S2) or flooded (S3) backgrounds for both years. Furthermore, the MAE dropped to less than 5% when using in simulation different backgrounds for the different dates/dataset according to the reported actual field conditions. The results showed how soil conditions substantially affect the simulated spectra, mainly in the early stage of rice development. Thus, they significantly impact the retrieval of crop parameters across season and space in hybrid approaches. Hence, the inclusion of different soil backgrounds is a prerequisite to simulate realistic crop reflectance spectra in phase #2.
Figure 5. Mean Absolute Error (MAE) of PROSAIL-PRO simulations for the 2004 dataset (left panels) and 2006 (right panels) using different background spectra. Legend: S1 = Dry soil condition; S2 = Humid soil condition; S3 = Flooded soil condition; M = ”Match”, meaning real soil spectrum as observed in the field. MAE error lines 5% (green), 10% (yellow) and 15% (red) are highlighted.

Phase #2: Retrieval of crop parameters from Hybrid model
Cross-validation results
and summarise the cross-validation results for the standard hybrid model (HYB) and the hybrid model with active learning optimisation (HYB-AL). Among the active learning methods tested, cluster-based diversity and Euclidean distance-based diversity were the most accurate. In particular:
Figure 6. Correlation of LAI (upper panels) and CNC (lower panels) in cross-validation. Left panels: results of the HYB model using all the 2,000 records of the LUT. Right panels: results of the HYB-AL with optimised LUT for LAI (305 samples) and CNC (153 samples).

for LAI, estimates and measures were well correlated on the whole range of values for both HYB (R2 = 0.78) and HYB-AL (R2 = 0.71). For HYB-AL, cluster-based diversity provided an optimised training set of 305 spectra that achieved the best performance among the tested AL algorithms;
for CNC, estimates had a low correlation on the whole range of values measured in the field. For HYB-AL, Euclidean distance-based diversity provided the best results with an optimised training set of 153 spectra. In this case, the model with active learning improved the results obtained by the standard hybrid (R2 = 0.37 for HYB and 0.45 for HYB-AL) showing also a reduced saturation in the estimates (slope = 0.37 for HYB and 0.46 for HYB-AL).
Table 4. Cross-validation of LAI and CNC estimates.
Validation with field data
reports the scatter plots of estimates vs measured data, while summarise the accuracy metrics of LAI and CNC estimates. The validation against field data shows that LAI estimates were always well correlated with measured values, whereas CNC estimates had the same saturation issue above 4 [g m−2] already observed for cross-validation. The best results for LAI estimation were achieved for the real farming condition (2018 dataset; R2 = 0.86) using both HYB and HYB-AL approaches on actual Sentinel-2 data.
Table 5. Validation of LAI and CNC estimates with field data.
Active Learning optimisation improve LAI estimation in particular for 2018 data set, RRMSE decreases from 27.8% to 19.6%. Regarding CNC estimates HYB-AL led to better results especially when estimates are compared to measured values lower than 4 [], in this range RRMSE decreases from 50% to 45%.
Phase #3: Map generation and assessment of information content
Map generation
LAI maps of the study area () were generated using the HYB-AL model and Sentinel-2 time series, acquired from rice emergence to heading stage (June-August 2018). Maps were analysed to evaluate their temporal accordance with typical rice growth in the study area. shows LAI maps in four key dates of rice development for the entire study area (left panels – a1, b1, c1 and d1) and a details to better visualise LAI variability at field level (right panels – a2, b2, c2 and d2). Red, Orange and yellow dots, in the left panels, highlight selected fields for which LAI temporal profiles analysis was performed (); yellow, red and orange polygons, in the right panels, show the extent and position of the selected fields for two neighboring farms. These maps provide a broad overview of the changes occurring in different rice cropping systems due to multiple factors related to specific farm agro-practices such as cultivated rice varieties, sowing dates, water management and soil characteristics and fertility. At the beginning of June (), paddy fields had an almost null LAI value corresponding to crop emergence; slightly higher values (LAI about 1 [m2 m−2]) can be found for early sowed fields that were already at the beginning of tillering, this condition can be appreciate in -a2 (see yellow polygon). Between the end of June and the beginning of July (), LAI values in most of the maps quickly increase to 4 [m2 m−2] due to the stem elongation phase. July maps () show mainly dark green areas and some brighter spots corresponding to late-sowed fields that has a delay in crop development. The two zooms of (panels b2 and c2) clearly highlight LAI difference between an early (yellow polygon) and a late (red polygon) sowed field. Finally, all paddies reached their maximum LAI (about 5–6 [m2 m−2] by the end of the vegetative phase (booting to flowering) at the beginning of August ().
Figure 8. Sentinel-2 LAI maps retrieved at district level in four dates (left panels) and details for some fields (right panel). Zoom area is highlighted by red box drawn in a1 panel. The considered dates are: 1 June 2018 (a), June 21st (b), 7 July 2018 (c) and 5 August 2018 (d). Dots highlight the locations of the LAI temporal profiles shown in . Six locations are from the field monitored in the SATURNO project (Orange). Two locations are from fields with late (yellow) and early (red) sowing dates as reported by the farmer. Color polygons highligth position and extent of selected fields in the zoom area (rigth polygons).

Assessment of information content
shows the average LAI temporal profiles for the fields highlighted in . Yellow and red profiles show LAI trends that, according to farmer communication, had very late and early sowing, respectively. Orange profiles show LAI values extracted from the six monitored fields (see, ). These fields were cultivated with the same cultivar (Selenio) and had identical management in terms of sowing date and fertilisation period. Consequently, resulted in a similar crop development, as confirmed by comparable LAI temporal series. Nevertheless, some remarkable differences are visible due to soil fertility differences, especially at the beginning of the season. The graph also shows:
Figure 10. Panel a) prescription map from SATURNO project for the VRT fertilisation (17 July). Panel b) CNC map estimated for 6 July 2018. Coloured triangles/circles represent the selected samples for different CNC values. In the VRT managed zone: high CNC (red triangle), medium CNC (blue triangle) and low CNC (green triangle). In the homogeneous management zone: high CNC (red circle) and low CNC (green circle).

LAI time series of an early-sowed rice (yellow points) with an anticipated anomalous crop growth (LAI above 2 [m2 m−2] at the beginning of June), followed by a slow increase of LAI;
LAI time series of a late-sowed rice (red points) which starts growing at the beginning of July and reaches LAI levels of other varieties in few weeks.
Both temporal behaviours are coherent with the rice varieties usually cultivated in this district. Early sowing is performed with slow-growing rice varieties with a long life cycle whereas late sowing is performed with modern and fast-growing varieties (SATURNO End-Users personal communication).
also indicates that the LAI time series can identify fields with similar management strategy but different crop growth. These kind of information can be helpful to identify growing anomalies and to support management strategies, when provided during the season.
CNC maps were also compared to actual prescription maps generated for VRT fertilisation application (). The reliability and value-added information provided by CNC maps was assessed by performing a field-level analysis to investigate within-field variability.
shows the prescription map used to fertilise the field with VRT on July 17th. Specifically, the upper part of the field was managed with three target doses of urea (140, 175 and 220 [kg ha−1] according to crop conditions identified in the field (see Material section). The lower part of the field was managed with a standard homogeneous fertilisation dose (175 [kg ha−1]; Nutini et al., Citation2021).
shows the CNC map for July 6th generated with the Sentinel-2 image acquired before fertilisation and the location of 5 investigated sample areas. Three samples were selected in the upper half of the field with VRT fertilisation: one with a high CNC estimate inside the VRT low target dose (red triangle), one with a medium CNC estimate inside the VRT medium target dose (blue triangle), and one with a low CNC estimate inside the VRT high target dose (green triangle). Two additional samples were selected in the lower half of the field with standard fertilisation: one with a high CNC (red circle) estimate and one with a low CNC estimate (green circle).
shows the temporal trend of CNC estimated from Sentinel-2 images on the locations highlighted in . Interestingly, after the VRT fertilisation, which provided different urea doses according to the plant status, the differences in CNC estimates decreased. This effect is particularly evident for the medium CNC samples which received a standard dose of fertiliser (175 [kg ha−1]) compared to the high CNC samples that received a 25% reduced dose (140 [kg ha−1]). For the medium CNC sample, after fertilisation, the CNC increased from 3.3 [g m−2] up to 4.25 [g m−2] (estimated from the image of 27 July). Despite the reduced fertilisation, the high CNC sample kept growing, and its CNC increased from 4.2 [g m−2] to 4.35 [g m−2] (). A significant improvement was also recorded for the low CNC sample that received 25% more fertiliser (220 [kg ha−1].). In this case, after fertilisation, its CNC passed from ~3 [g m−2] to ~4 [g m−2].
Figure 11. Panel a) Temporal series of CNC estimates from Sentinel-2 images for the five locations highlighted in Figure 11. Panels b) and c) Detail of the values before fertilisation (6 July) and after fertilisation (23 July, 31 July and 5 August).

As shown in , the CNC level of the three samples included in the VRT part of the field were comparable (~4.5 [g m−2]) at the end of the investigated period (5 August). On the contrary, differences in rice crop development were still present in the homogeneously managed half of the field, after the fertilisation (). The provision of standard dose (175 []) to the lower half of field resulted in a significant difference at the end of the investigation: high CNC sample reached 5 [g m−2], whereas the low CNC sample stopped at 4.2 [g m−2]. This behaviour reflects a non-optimised N management, which in the end will affect the yield, as confirmed by the study of Francesco (Nutini et al., Citation2021).
Discussion
Simulation of rice spectra
The generation of a large set of realistic spectra linked to leaf and canopy parameters is a prerequisite for the hybrid retrieval approach, and this study support the use of PROSAIL-PRO as a reliable and accurate model to simulate rice spectra over the whole season and in different environmental conditions. From the comparison of measured and simulated spectra, a MAE value lower than 5% was obtained along the full spectral range for both 2004 and 2006 datasets, when information on the existing background (i.e. specific soil spectra according to actual conditions) were exploited. Conversely, higher differences between measured and simulated spectra were obtained (up to 20% of MAE) when using only a single background spectra. Results are very satisfying considering the different conditions in the two experiments: different varieties, phenological stage, fertilisation level and sowing type (flooded or dry soil). Thus, tests confirmed that the reflectance of the background represents a significant component of the overall rice spectral signature and a correct characterisation of soil conditions, as input to the PROSAIL-PRO model, is mandatory in order to obtain realistic simulations. These findings are in agreement with the study of M. Campos-Taberner et al. (Citation2016a), conducted in the Mediterranean area, where best LAI estimations were obtained when a spectral library of underlying soil background composed by flooded and dry soils was considered as input to the model, whereas high errors were found during the initial plants’ development stages when considering only a flooded or a dry background.
Performance of the hybrid model and contribution of the active learning
Given LAI and CNC’s importance in assessing rice crop nutritional status, this study evaluated the added value of hybrid approaches either with or without an active learning optimisation strategy in plant traits retrieval.
Regarding LAI, cross-validation on simulated spectra showed good estimates when using the standard HYB approach ( = 0.78; RMSEcv = 0.63) which slightly decreased using HYB-AL although resulting still accurate (
= 0.71; RMSEcv = 0.63). The robustness and exportability of the retrieval models were further confirmed by quantitative validation using independent datasets. Considering HYB model, we obtained good results with an overestimation for the 2004 dataset (R2 = 0.72; RMSE = 1.05) and a slight underestimation for 2006 (R2 = 0.74; RMSE = 0.91) and 2018 (R2 = 0.86; RMSE = 0.77) datasets. Using a hybrid approach, applied to Sentinel-2 data in the Mediterranean environment, Campos-Taberner et al. (Citation2017) obtained similar performances for rice (R2 = 0.95; RMSE = 0.69), which showed some overestimation. On the contrary, significative underestimation of LAI and worst performances were recently reported in the study of Adeluyi et al. (Citation2021) (R2 = 0.82; RMSE = 1.65). When HYB-AL model is adopted, differences between estimates and ground measurements are reduced with the majority of retrievals falling close to the 1:1 line. RRMSE values are reduced by 4 to 8% for all the three considered data set (see, ). It is interesting to notice that the retrieval for the 2018 dataset provided the best results (R2 = 0.86; RMSE = 0.54). Compared to similar study, the HYB-AL schema achieved better results than those reported in Pipia et al. (Citation2021), (R2 = 0.63; MAE = 0.58; RMSE = 0.73), where a GPR model with AL optimisation was applied to S2 data for greenLAI estimation for multiple crops. Considering that 2018 dataset was taken in real farm conditions results are very promising for applications in operational monitoring systems.
Regarding CNC, retrieval models with both approaches show moderate performance in cross-validation with slightly better results achieved by active learning (HYB-AL: = 0.45; RMSEcv = 1.36) with respect to standard approach (HYB:
= 0.37; RMSEcv = 1.41). Both CNC models showed a saturation behaviour that limits the retrieval accuracy for moderate/high CNC values approximately above 4 [g m−2]. However, we can observed how active learning, by selecting the most significative training from the initial LUT, contribute to reduce such effect (slope = 0.37 for HYB and 0.46 for HYB-AL). This result is confirmed by validation with ground data, where HYB-AL shows slightly better performance (R2 = 0.63; RMSE = 0.89) than traditional HYB (R2 = 0.60; RMSE = 1.02) approach reducing RRMSE of about 5% when all values range is considered (0–7 [g m−2]). The improvement is even more evident for low CNC values, below 3–4 [g m−2], with most retrievals falling close to the 1:1 line (see, ). This finding is very encouraging for estimating rice nutritional status with satellite Earth Observation. In fact, CNC is a critical information between tillering and stem elongation phases, when the first and second topdressing fertilisation applications are conducted: in this phenological period, the crop CNC values are in general in the range 2–4 [g m−2] (Stroppiana et al., Citation2009). Therefore, the developed model is promising to provide useful information for precision farming management.
Results achieved in this study are anyway satisfying if considering that estimates are obtained from multispectral satellite images through an transferable and data-independent approach. Similar or more accurate estimates of N in rice crops, also at leaf level, can be achieved when using data driven approach and/or hyperspectral ground or aerial data. Stroppiana et al. (Citation2009) obtained good correlation between narrow bands optimised vegetation index, from field spectroradiometric data, and ground nitrogen concentration measurements (R2 = 0.65). Inoue et al. (Citation2012) estimates CNC with hyperspectral aerial data exploiting both vegetation indices and machine learning approaches (Partial Least-Squares Regression) obtaining very promising results with potentially operational transferability (R2 = 0.9; RMSE = 0.8).
Colorado et al. (Citation2020) recently demonstrated the utility of multispectral UAV images for leaf nitrogen estimates as an alternative approach to traditional field measurements: different results in terms of accuracy were obtained depending on the tested specific machine learning algorithm (R2 = 0.57 for Support Vector Machine; R2 = 0.92 for multi-variable linear regression; R2 = 0.97 for Neural Network).
Anyway, the source of under estimation for medium/high values needs to be further investigated, also considering that the direct estimation of CNC from RTM is a quite new approach in remote sensing and, to date, it was mainly performed with hyperspectral data (Berger et al., Citation2020b; Candiani et al., Citation2022; Tagliabue et al., Citation2022; Verrelst et al., Citation2021b).
As an example, Tagliabue et al. (Citation2022) used a similar HYB-AL GPR model to predict CNC for multi crops, comprising rice, from PRISMA hyperspectral images obtaining very good results with no saturation effect (CNC: R2 = 0.79; NRMSE = 23.7%). Indeed, hyperspectral sensors with multiple and narrow bands in the short-wave infrared region, where plant nitrogen compound shows their optical absorption features (Berger et al Citation2020a), are considered the most appropriate for the estimation of CNC. The availability of large spectroscopic data streams from the already available PRISMA (Loizzo et al., Citation2019) and HISUI sensors (Matsunaga et al., Citation2020), together with the forthcoming EnMAP (Guanter et al., Citation2015), SBG (Thompson et al., Citation2020) and CHIME (Rast et al., Citation2019) satellite missions, could be the key for the aforementioned investigation.
Maps generated information content
The application of the HYB-AL GPR model to Sentinel-2 images made it possible to produce useful information on the crop status to highlight spatio-temporal differences at both district and field level. Beside the good quantitative performances of LAI estimates, as obtained by comparison with field data in the model validation step, the LAI maps generated from June to August resulted reliable EO-derived products for crop monitoring. The identified spatio-temporal patterns are in agreement with the expected physical process of cultivated rice variety, with LAI estimates increasing in time from 0 to 6 [m2 m−2], coherently with previous studies (M. Campos-Taberner et al., Citation2016b). Analysing results for the whole study area (see, ), makes it clear how those maps provide a visual overview of the differences related to rice cultivars, sowing dates, water management, or soil quality, occurring in the rice cropping systems. LAI estimates are a valuable information that can be useful to identifies different cultivated varieties, agropractices and occurrence of phenological stages (Boschetti et al., Citation2018). Moreover, these geo-information at decametric scale can be a fundamental input for crop modelling devoted to estimate yield changes at field level (Gilardelli et al., Citation2019).
The generated maps are also very promising as a value-added information to support crop management. CNC maps highlighted within-field spatial variation of crop nutritional status, coherently with the heterogeneity observed in the field and in agreement with pre and post fertilisation conditions. The comparison of CNC estimates in areas where different fertilisation techniques were applied (i.e. the VR and the standard), highlighted how the obtained maps are able to describe crop conditions and response to nitrogen management. The analysis of CNC temporal trends in VRT-managed zone demonstrated how a smart management system, able to provide higher dose (220 []) in critical areas characterised by low CNC values, produced the positive effect to recover rice crop condition. Moreover, lower doses of fertiliser, applied to those zones with high CNC values (before fertilisation application), allowed to save up to 20% on N application while ensuring proper crop growth, as showed in . Conversely, CNC trends in homogeneously managed zone showed how low and high pre-fertilisation CNC conditions are maintained during time, highlighting a suboptimal crop management performance with potential economically and ecologically drawbacks.
Conclusions
In this study, an experimental activity was conducted to estimate rice crop LAI and CNC parameters from S2 data, testing HYB and HYB-AL approaches based on RTM simulations as input for GPR MLRA. The final goal is the achievement of an operational and accurate monitoring framework able to support optimised crop production, as well as preserving the environment. This study assessed not only the performance of biophysical parameter retrieval but also the capacity of the radiative transfer model to reproduce rice spectra according to different background conditions, quantifying the effect of a wrong parameterisation on the simulated spectra.
The performed test showed that rice spectra, simulated using the PROSAIL-PRO model, were in agreement with measured data when a representative database of background reflectance is provided as input (overall reflectance MAE lower than 5%).
From our findings, LAI estimates from hybrid retrieval approach, and in particular adopting the active learning technique, resulted always robust and can be considered with operational quality, even though a saturation effect on CNC retrieval is evident for CNC values greater than 4 [g m−2]. This observation needs further investigations to improve model performance, also adopting different active learning scheme and exploit necessary new ground data, although such limitation should not represent a problem for prototyping application of fertilisation agro-practices in rice, which are usually performed during phenological stages with low CNC values. The detected CNC within-field variability is in fact the foreseen information requested by user community for a quantitative support of sustainable fertilisation.
LAI and CNC maps generated from Sentinel-2 images through HYB-AL provided crop monitoring information at both district and farm level, exploiting the high spatial and temporal resolution of such sensor. The analysis clearly shows the positive effect of the VRT fertilisation according to developed prescription map: plants presenting different nutritional status before the fertilisation application achieved a final homogeneous N plant content which is the scope of precision farming applications, devoted to obtain optimised yield by reducing input.
From these findings, it can be stated that such digital geo-products obtained from spaceborne imaging multispectral data represent a promising contribution to support crop monitoring and fertilisation management purposes. In conclusion, the proposed method demonstrated the feasibility of a direct estimation of rice biophysical variables from S2 products, as a contribution for precision farming applications.
Acknowledgements
The research activities have been framed in the CNR- DIPARTIMENTO DI INGEGNERIA, ICT E TECNOLOGIE PER L’ENERGIA E I TRASPORTI project “DIT.AD022.180 Transizione industriale e resilienza delle Società post-Covid19 (FOE 2020)”, sub task activity “Agro-Sensing”.
Disclosure statement
No potential conflict of interest was reported by the author(s).
Data availability statement
The data that support the findings of this study are available from the corresponding author, M.R., upon reasonable request.
References
- Adeluyi, O., Harris, A., Verrelst, J., Foster, T., & Clay, G. D. (2021). Estimating the phenological dynamics of irrigated rice leaf area index using the combination of PROSAIL and gaussian process regression. International Journal of Applied Earth Observation and Geoinformation, 102, 102454. https://doi.org/10.1016/J.JAG.2021.102454
- Agri-food Data Portal. (2019). DG AGRI - Rice Production. https://agridata.ec.europa.eu/extensions/DashboardRice/RiceProduction.html
- Baret, F., Houlès, V., & Guérif, M. (2007). Quantification of plant stress using remote sensing observations and crop models: The case of nitrogen management. Journal of Experimental Botany, 58(4), 869–20. https://doi.org/10.1093/jxb/erl231
- Berger, K., Rivera Caicedo, J. P., Martino, L., Wocher, M., Hank, T., & Verrelst, J. (2021). A survey of active learning for quantifying vegetation traits from terrestrial earth observation data. Remote Sensing, 13(2), 287. https://doi.org/10.3390/rs13020287
- Berger, K., Verrelst, J., Féret, J.-B., Hank, T., Wocher, M., Mauser, W., & Camps-Valls, G. (2020a). Retrieval of aboveground crop nitrogen content with a hybrid machine learning method. International Journal of Applied Earth Observation and Geoinformation, 92, 102174. https://doi.org/10.1016/j.jag.2020.102174
- Berger, K., Verrelst, J., Féret, J. B., Hank, T., Wocher, M., Mauser, W., & Camps-Valls, G. (2020b). Retrieval of aboveground crop nitrogen content with a hybrid machine learning method. International Journal of Applied Earth Observation and Geoinformation, 92, 102174. https://doi.org/10.1016/j.jag.2020.102174
- Berger, K., Wang, Z., Danner, M., Wocher, M., Mauser, W., & Hank, T. (2018, July). Simulation of spaceborne hyperspectral remote sensing to assist crop nitrogen content monitoring in agricultural crops. International Geoscience and Remote Sensing Symposium, 3801–3804. https://doi.org/10.1109/IGARSS.2018.8518537
- Boschetti, M., Bocchi, S., Stroppiana, D., & Brivio, P. A. (2006). Estimation of parameters describing morpho-physiological features of Mediterranean rice varieties for modelling purposes. The Italian Journal of Agronomy, 49, 40–49. http://www.agrometeorologia.it/wp-content/uploads/2010/11/5.pdf
- Boschetti, M., Busetto, L., Ranghetti, L., Haro, J. G., & Bassini, V. (2018). TESTING MULTI-SENSORS TIME SERIES OF LAI ESTIMATES TO MONITOR RICE PHENOLOGY: PRELIMINARY RESULTS institute for electromagnetic sensing of the environment, Italian national research council, department of earth physics and thermodynamics, faculty of P. IGARSS 2018-2018 IEEE. International Geoscience and Remote Sensing Symposium, 8221–8224. doi: 10.1109/IGARSS.2018.8518494.
- Campos-Taberner, M., García-Haro, F. J., Camps-Valls, G., Grau-Muedra, G., Nutini, F., Busetto, L., Katsantonis, D., Stavrakoudis, D., Minakou, C., Gatti, L., Barbieri, M., Holecz, F., Stroppiana, D., & Boschetti, M. (2017). Exploitation of SAR and optical sentinel data to detect rice crop and estimate seasonal dynamics of leaf area index. Remote Sensing, 9(3), 248. https://doi.org/10.3390/rs9030248
- Campos-Taberner, M., Garcia-Haro, F. J., Camps-Valls, G., Grau-Muedra, G., Nutini, F., Crema, A., & Boschetti, M. (2016a). Multitemporal and multiresolution leaf area index retrieval for operational local rice crop monitoring. Remote Sensing of Environment, 187, 102–118. https://doi.org/10.1016/j.rse.2016.10.009
- Campos-Taberner, M., García-Haro, F. J., Camps-Valls, G., Grau-Muedra, G., Nutini, F., Crema, A., & Boschetti, M. (2016). Multitemporal and multiresolution leaf area index retrieval for operational local rice crop monitoring. Remote Sensing of Environment, 187, 102–118. https://doi.org/10.1016/j.rse.2016.10.009
- Campos-Taberner, M., Garcia-Haro, F. J., Confalonieri, R., Martinez, B., Moreno, A., Sanchez-Ruiz, S., Gilabert, M. A., Camacho, F., Boschetti, M., & Busetto, L. (2016b). Multitemporal monitoring of plant area index in the Valencia rice district with PocketLAI. Remote Sensing, 8(3), 202. https://doi.org/10.3390/rs8030202
- Candiani, G., Tagliabue, G., Panigada, C., Verrelst, J., Picchi, V., Rivera Caicedo, J. P., & Boschetti, M. (2022). Evaluation of hybrid models to estimate chlorophyll and nitrogen content of maize crops in the framework of the future CHIME mission. Remote Sensing, 14(8), 1792. https://doi.org/10.3390/rs14081792
- Clevers, J. G. P. W., & Gitelson, A. A. (2013). Remote estimation of crop and grass chlorophyll and nitrogen content using red-edge bands on Sentinel-2 and −3. International Journal of Applied Earth Observation and Geoinformation, 23, 344–351. https://doi.org/10.1016/J.JAG.2012.10.008
- Colorado, J. D., Cera-Bornacelli, N., Caldas, J. S., Petro, E., Rebolledo, M. C., Cuellar, D., Calderon, F., Mondragon, I. F., & Jaramillo-Botero, A. (2020). Estimation of nitrogen in rice crops from UAV-captured images. Remote Sensing, 12(20), 1–31. https://doi.org/10.3390/rs12203396
- Confalonieri, R., Foi, M., Casa, R., Aquaro, S., Tona, E., Peterle, M., Boldini, A., De Carli, G., Ferrari, A., Finotto, G., Guarneri, T., Manzoni, V., Movedi, E., Nisoli, A., Paleari, L., Radici, I., Suardi, M., Veronesi, D., Bregaglio, S., … Acutis, M. (2013). Development of an app for estimating leaf area index using a smartphone. Trueness and precision determination and comparison with other indirect methods. Computers and Electronics in Agriculture, 96, 67–74. https://doi.org/10.1016/j.compag.2013.04.019
- Danner, M., Berger, K., Wocher, M., Mauser, W., & Hank, T. (2019). Fitted PROSAIL parameterization of leaf inclinations, water content and brown pigment content for winter wheat and maize canopies. Remote Sensing, 11(10), 1150. https://doi.org/10.3390/rs11101150
- Delloye, C., Weiss, M., & Defourny, P. (2018). Retrieval of the canopy chlorophyll content from Sentinel-2 spectral bands to estimate nitrogen uptake in intensive winter wheat cropping systems. Remote Sensing of Environment, 216, 245–261. https://doi.org/10.1016/j.rse.2018.06.037
- Fava, F., Colombo, R., Bocchi, S., Meroni, M., Sitzia, M., Fois, N., & Zucca, C. (2009). Identification of hyperspectral vegetation indices for Mediterranean pasture characterization. International Journal of Applied Earth Observation and Geoinformation, 11, 233–243. https://doi.org/10.1016/j.jag.2009.02.003
- Féret, J. B., Berger, K., de Boissieu, F., & Malenovský, Z. (2021). PROSPECT-PRO for estimating content of nitrogen-containing leaf proteins and other carbon-based constituents. Remote Sensing of Environment, 252, 112173. https://doi.org/10.1016/j.rse.2020.112173
- Gilardelli, C., Stella, T., Confalonieri, R., Ranghetti, L., Campos-Taberner, M., García-Haro, F. J., & Boschetti, M. (2019). Downscaling rice yield simulation at sub-field scale using remotely sensed LAI data. European Journal of Agronomy, 103, 108–116. https://doi.org/10.1016/j.eja.2018.12.003
- Guanter, L., Kaufmann, H., Segl, K., Foerster, S., Rogass, C., Chabrillat, S., Kuester, T., Hollstein, A., Rossner, G., Chlebek, C., Straif, C., Fischer, S., Schrader, S., Storch, T., Heiden, U., Mueller, A., Bachmann, M., Mühle, H., Müller, R., … Sang, B. (2015). The EnMAP spaceborne imaging spectroscopy mission for earth observation. Remote Sensing, 7(7), 8830–8857. https://doi.org/10.3390/rs70708830
- Hank, T. B., Berger, K., Bach, H., Clevers, J. G. P. W., Gitelson, A., Zarco-Tejada, P., & Mauser, W. (2019). Spaceborne imaging spectroscopy for sustainable agriculture: contributions and challenges, surveys in geophysics. Springer Netherlands. https://doi.org/10.1007/s10712-018-9492-0
- Hansen, P. M., & Schjoerring, J. K. (2003). Reflectance measurement of canopy biomass and nitrogen status in wheat crops using normalized difference vegetation indices and partial least squares regression. Remote Sensing of Environment, 86(4), 542–553. https://doi.org/10.1016/S0034-4257(03)
- Inoue, Y., Sakaiya, E., Zhu, Y., & Takahashi, W. (2012). Diagnostic mapping of canopy nitrogen content in rice based on hyperspectral measurements. Remote Sensing of Environment, 126, 210–221. https://doi.org/10.1016/j.rse.2012.08.026
- Jacquemoud, S., & Baret, F. (1990). PROSPECT: A model of leaf optical properties spectra. Remote Sensing of Environment, 34(2), 75–91. https://doi.org/10.1016/0034-4257(90)90100-Z
- Lassaletta, L., Billen, G., Garnier, J., Bouwman, L., Velazquez, E., Mueller, N. D., & Gerber, J. S. (2016). Nitrogen use in the global food system: Past trends and future trajectories of agronomic performance, pollution, trade, and dietary demand. Environmental Research Letters, 11(9), 095007. https://doi.org/10.1088/1748-9326/11/9/095007
- Lemaire, G., Tang, L., Bélanger, G., Zhu, Y., & Jeuffroy, M. H. (2021). Forward new paradigms for crop mineral nutrition and fertilization towards sustainable agriculture. European Journal of Agronomy, 125, 126248. https://doi.org/10.1016/J.EJA.2021.126248
- Liu, N., Townsend, P. A., Naber, M. R., Bethke, P. C., Hills, W. B., & Wang, Y. (2021). Hyperspectral imagery to monitor crop nutrient status within and across growing seasons. Remote Sensing of Environment, 255, 112303. https://doi.org/10.1016/J.RSE.2021.112303
- Loizzo, R., Daraio, M., Guarini, R., Longo, F., Lorusso, R., Dini, L., & Lopinto, E., 2019. Prisma mission status and perspective, in: IGARSS 2019-2019 IEEE International geoscience and remote sensing symposium. pp. 4503–4506. https://doi.org/10.1109/IGARSS.2019.8899272
- Matsunaga, T., Iwasaki, A., Tachikawa, T., Tanii, J., & Kashimura, O. (2020). HYPERSPECTRAL IMAGER SUITE (HISUI): ITS LAUNCH AND CURRENT STATUS national institute for environmental studies (NIES), Japan The University of Tokyo, Japan Japan Space Systems, Japan National Institute of Advanced Industrial Science and Technology. International Geoscience and Remote Sensing Symposium, 3, 3272–3273. https://doi.org/10.1109/IGARSS39084.2020.9323376
- NOAA, Global Positioning Laboratory. (n.d.). NOAA Solar Calculator. https://gml.noaa.gov/grad/solcalc/index.html
- Nutini, F., Confalonieri, R., Paleari, L., Pepe, M., Criscuolo, L., Porta, F., Ranghetti, L., Busetto, L., & Boschetti, M. (2021). Supporting operational site‐specific fertilization in rice cropping systems with infield smartphone measurements and Sentinel-2 observations. Precision Agriculture, 22(4), 1284–1303. https://doi.org/10.1007/s11119-021-09784-0
- Paleari L, Movedi E, Vesely FM, Thoelke W, Tartarini S, Foi M, Boschetti M, Nutini F, Confalonieri R. Estimating Crop Nutritional Status Using Smart Apps to Support Nitrogen Fertilization. A Case Study on Paddy Rice. Sensors. 2019; 19(4):981. https://doi.org/10.3390/s19040981
- Pipia, L., Amin, E., Belda, S., Salinero-Delgado, M., & Verrelst, J. (2021). Green lai mapping and cloud gap-filling using Gaussian process regression in google earth engine. Remote Sensing, 13(3), 1–25. https://doi.org/10.3390/rs13030403
- Ranghetti, L., Boschetti, M., Nutini, F., & Busetto, L. (2020). “sen2r”: An R toolbox for automatically downloading and preprocessing Sentinel-2 satellite data. Computers & Geosciences, 139, 104473. https://doi.org/10.1016/j.cageo.2020.104473
- Rast, M., Ananasso, C., Bach, H., Dor, E., Chabrillat, S., Colombo, R., Bello, U., Feret, J., Giardino, C., Green, R., Guanter, L., Marsh, S., Nieke, J., Ong, C., Rum, G., Schaepman, M., Schlerf, M., Skidmore, A., & Strobl, P. (2019). Copernicus hyperspectral imaging mission for the environment-mission requirements document. The European Space Agency. ESA-EOPSM-CHIM-MRD-3216. https://esamultimedia.esa.int/docs/EarthObservation/Copernicus_CHIME_MRD_v3.0_Issued_21_01_2021.pdf (accessed on 28 February 2019).
- Schlemmer, M., Gitelson, A., Schepers, J., Ferguson, R., Peng, Y., Shanahan, J., & Rundquist, D. (2013). Remote estimation of nitrogen and chlorophyll contents in maize at leaf and canopy levels. International Journal of Applied Earth Observation and Geoinformation, 25, 47–54. https://doi.org/10.1016/j.jag.2013.04.003
- THE STATE OF FOOD AND AGRICULTURE. CLIMATE CHANGE, AGRICULTURE AND FOOD SECURITY. FAO 2016 ISBN 978-92-5-109374-0, ISSN 0081-4539. Available online: https://www.fao.org/3/i6030e/i6030e.pdf (accessed on 10 April 2021)
- Stroppiana, D., Boschetti, M., Brivio, P. A., & Bocchi, S. (2009). Plant nitrogen concentration in paddy rice from field canopy hyperspectral radiometry. Field Crops Research, 111(1–2), 119–129. https://doi.org/10.1016/j.fcr.2008.11.004
- Tagliabue, G., Boschetti, M., Bramati, G., Candiani, G., Colombo, R., Nutini, F., Pompilio, L., Rivera-Caicedo, J. P., Rossi, M., Rossini, M., Verrelst, J., & Panigada, C. (2022). Hybrid retrieval of crop traits from multi-temporal PRISMA hyperspectral imagery. ISPRS Journal of Photogrammetry and Remote Sensing, 187, 362–377. https://doi.org/10.1016/j.isprsjprs.2022.03.014
- Thompson, D. R., Schimel, D. S., Poulter, B., Brosnan, I., Hook, S. J., Green, R. O., Glenn, N., Guild, L., Henn, C., Cawse-Nicholson, K., Kokaly, R., Lee, C., Luvall, J., Miller, C. E., Nastal, J., Pavlick, R., Phillips, B., Schneider, F., Uz, S. S., … Wang, W. (2020). NASA’s surface biology and geology concept study: status and next steps. International Geoscience and Remote Sensing Symposium, 3269–3271. https://doi.org/10.1109/IGARSS39084.2020.9323295
- Upreti, D., Huang, W., Kong, W., Pascucci, S., Pignatti, S., Zhou, X., Ye, H., & Casa, R. (2019). A comparison of hybrid machine learning algorithms for the retrieval of wheat biophysical variables from sentinel-2. Remote Sensing, 11(5), 481. https://doi.org/10.3390/rs11050481
- Verhoef, W. (1984). Light scattering by leaf layers with application to canopy reflectance modeling: The SAIL model. Remote Sensing of Environment, 16(2), 125–141. https://doi.org/10.1016/0034-4257(84)90057-9
- Verrelst, J., Berger, K., & Rivera-Caicedo, J. P. (2020). Intelligent sampling for vegetation nitrogen mapping based on hybrid machine learning algorithms. IEEE Geoscience and Remote Sensing Letters, 18(12), 2038–2042. https://doi.org/10.1109/LGRS.2020.3014676
- Verrelst, J., Malenovský, Z., Van der Tol, C. et al. Quantifying Vegetation Biophysical Variables from Imaging Spectroscopy Data: A Review on Retrieval Methods. Surv Geophys, 40(3), 589–629 (2019). https://doi.org/10.1007/s10712-018-9478-y
- Verrelst, J., Rivera, J. P., Alonso, L., & Moreno, J. (2011, April). ARTMO: An Automated Radiative Transfer Models Operator toolbox for automated retrieval of biophysical parameters through model inversion. In Proc. EARSeL 7th SIG-Imag. Spectrosc. Workshop (pp. 11–13).
- Verrelst, J., Rivera-Caicedo, J. P., Reyes-Muñoz, P., Morata, M., Amin, E., Tagliabue, G., Panigada, C., Hank, T., & Berger, K. (2021a). Mapping landscape canopy nitrogen content from space using PRISMA data. ISPRS Journal of Photogrammetry and Remote Sensing, 178, 382–395. https://doi.org/10.1016/j.isprsjprs.2021.06.017
- Verrelst, J., Rivera-Caicedo, J. P., Reyes-Muñoz, P., Morata, M., Amin, E., Tagliabue, G., Panigada, C., Hank, T., & Berger, K. (2021b). Mapping landscape canopy nitrogen content from space using PRISMA data. ISPRS Journal of Photogrammetry and Remote Sensing, 178, 382–395. https://doi.org/10.1016/J.ISPRSJPRS.2021.06.017
- Wang, S., Guan, K., Wang, Z., Ainsworth, E. A., Zheng, T., Townsend, P. A., Liu, N., Nafziger, E., Masters, M. D., Li, K., Wu, G., & Jiang, C. (2021). Airborne hyperspectral imaging of nitrogen deficiency on crop traits and yield of maize by machine learning and radiative transfer modeling. International Journal of Applied Earth Observation and Geoinformation, 105, 102617. https://doi.org/10.1016/J.JAG.2021.102617
- Weiss, M.; Baret, F. S2ToolBox Level 2 Products: LAI, FAPAR, FCOVER. Available online: https://step.esa.int/docs/extra/ATBD_S2ToolBox_L2B_V1.1.pdf (accessed on 21 January 2020).
- Weiss, M., Jacob, F., & Duveiller, G. (2020). Remote sensing for agricultural applications: A meta-review. Remote Sensing of Environment, 236, 111402. https://doi.org/10.1016/j.rse.2019.111402
- Wutzler, T., Migliavacca, M., & Julitta, T. (2016). FieldSpectroscopycc: R package for Characterization and Calibration of spectrometers. R Package Version 0.5.227. Available online: https://rdrr.io/github/tommasojulitta/FieldSpectroscopyCC/ (accessed on 22 May)
- Zarco-Tejada, P. J., Hubbard, N., & Loudjani, P. (2014). Precision agriculture: an opportunity for EU farmers—potential support with the CAP 2014–2020. Joint Research Centre (JRC) of the European Commission. Available online: https://policycommons.net/artifacts/1339069/precision-agriculture/1948411/ (accessed on 12 July 2021)
- Zhang, K., Yuan, Z., Yang, T., Lu, Z., Cao, Q., Tian, Y., Zhu, Y., Cao, W., & Liu, X. (2020). Chlorophyll meter–based nitrogen fertilizer optimization algorithm and nitrogen nutrition index for in-season fertilization of paddy rice. Agronomy Journal, 112(1), 288–300. https://doi.org/10.1002/agj2.20036