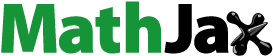
ABSTRACT
Non-photosynthetic vegetation (NPV) plays a key role in soil conservation, which in turn is important in sustainable agriculture and carbon farming. For mapping NPV image spectroscopy proved to outperform multispectral sensors. PRISMA (PRecursore IperSpettrale della Missione Applicativa) is the forerunner of a new era of hyperspectral satellite missions, providing the proper spectral resolution for NPV mapping. This study takes advantage from both spectroscopy and machine-learning techniques. Exponential Gaussian Optimization was used for modelling known absorption bands (cellulose-lignin, pigments, water content and clays), resulting in a reduced feature space, which is split by a decision tree (DT) for mapping different field conditions (emerging, green and standing dead vegetation, crop residue and bare soil). DT training and validation exploited reference data, collected during PRISMA overpasses on a large farmland. Mapping results are accurate both at pixel and parcel level (O.A. > 90%; K > 0.9). Field status and crop rotation trajectories through time are derived by processing 12 images over 2020 and 2021. Results proved that PRISMA data are suitable for mapping field conditions at parcel scale with high confidence level. This is important in the perspective of other hyperspectral missions and is a premise toward quantitative estimates of NPV biophysical variable.
Introduction
Non-photosynthetic vegetation cannot convert solar energy into chemical energy for its growth and development (Asner, Citation1998). It is generally composed by plant litter, standing dead or dying vegetation (SDV), and crop residues (CR). The last two forms, corresponding to vegetation at late mature growth stages and plant senescent material left after harvesting (Daughtry et al., Citation2004), play a strategic role in the framework of sustainable agriculture (Hank, Citation2019) and more recently of carbon farming (Smith et al., Citation2020). Their importance stands on their role in carbon, nutrients and water cycles, and in particular in soil conservation. In fact, the presence of CR on the fields provides protection against soil erosion (Arsenault & Bonn, Citation2005), controls temperature and moisture levels, increases soil organic carbon (Haddaway et al., Citation2017; Lal et al., Citation1999), reduces soil compaction due to agricultural machinery and improves the soil structure. Keeping crop residues is therefore a fundamental aspect for preserving soil-related agri-ecological conservation functions based on low-intensity tillage, permanent soil cover and crop rotation (Ranaivoson et al., Citation2017). The relative abundance of CR is an index of crop management practices since it is connected to tillage, crop rotations, and harvest methods (Daughtry, Citation2001; Daughtry et al., Citation2004; Daughtry & Hunt, Citation2008; Hively et al., Citation2021, Citation2018).
Previous studies proved that the detection and assessment of NPV through aerial (Kokaly et al., Citation2009; Roberts et al., Citation1993; Zheng et al., Citation2012) and satellite remote-sensing (RS) techniques are faster, more accurate and objective as compared to traditional field operator-based reports (Asner & Heidebrecht, Citation2002; Daughtry, Citation2001). By mapping the presence and abundance of NPV, remote sensing can certainly contribute to monitor/control the implementation and rate of conservation practices in agriculture (European Commission, Citation2018), providing also information for eco-system services in other domains, in a way that this has become a priority issue in the design of satellite missions (Hank et al., Citation2019, Berger et al., Citation2021; Hively et al., Citation2021).
Many studies of NPV in croplands exploit differences in the (VNIR-SWIR) reflectance spectra of the three main categories, such as NPV (mainly crop residues – CR), bare soil (BS) and green vegetation (GV) to accomplish class detection. The underpinning science is largely related to proximal hyperspectral remote-sensing studies (Kolaly et al., Citation2009; Daughtry, Citation2001; Daughtry et al., Citation2004; Daughtry & Huntjr, Citation2008), exploiting the differences within the 400–700 nm (VNIR) and the 1500–2500 nm (SWIR) ranges. Actually, the reflected radiation in the VNIR region is dominated by leaf pigments in green vegetation, while in the SWIR region, it is ruled by biochemical non-pigment plant constituents, i.e. carbon-based constituents (such as cellulose, lignin, proteins, hemicellulose and starch) (Fourty, et al., Citation1996). Carbon-based constituents show several diagnostic absorption features in the SWIR interval, in particular those of cellulose and lignin, between 2100 and 2300 nm. On this basis, several previous studies focused on NPV mapping through the computation of spectral indices from multispectral broadband data (Hively et al., Citation2018).
Hyperspectral narrow bands data proved to be more efficient (Bannari et al., Citation2015; Daughtry et al., Citation2005) and reliable than multispectral data; the reason is two-fold: (i) hyperspectral sensors allow for the recognition of even subtle absorption features, as those diagnostic of carbon-based constituents (Berger et al., Citation2021, Citation2020); (ii) main absorption features of carbon-based constituents are centred in the SWIR, a spectral region where most multispectral sensors provide incomplete information, by having few and broad resolution bands.
Past NPV research with hyperspectral data were based on ground and aerial data, since spaceborne sensors were very limited in number. PRISMA is considered as a precursor to the new era of upcoming hyperspectral missions; it is designed to address selected EO applications related to environmental monitoring and protection, and sustainable development. In the ASI PRISMA mission, the first pillar application is agriculture, as demonstrated by recently published studies (Berger et al., Citation2021; Cogliati et al., Citation2021; Pepe et al., Citation2020; Tagliabue et al., Citation2022; Verrelst et al., Citation2021). The narrow swath (30 km) and revisiting time of PRISMA are not optimal for operational monitoring. Notwithstanding such limitations, acquisitions could be frequent enough for within-season studies (Marshall et al., Citation2021).
Early studies based on PRISMA data already proved mission potentials in NPV mapping and quantification. Pepe et al. (Citation2020) exploited spectroscopic approaches and modelled the well-known cellulose-lignin absorption feature centred near 2100 nm, to map CR presence at parcel level, and assessed the absorption band characteristics in some cereal crops. Berger et al. (Citation2021) used a quantitative hybrid approach to train machine-learning regression algorithm, on the base of radiative transfer model simulations, to assess NPV biomass in croplands; this study confirmed SWIR absorption bands (1700, 2100 and 2300 nm) to be highly diagnostic of carbon-based constituents.
Several applications addressed to soil conservation highlighted the importance of reliable cover maps (Daughtry et al., Citation2005; Zheng et al., 2021), with categories of interest, such as: bare soil (BS, i.e. after tillage or no-till), emerging vegetation (EV, i.e. crops at emergence after sowing or in the first developing phases, plant emergence or regrowth on crop residues), green vegetation (GV, i.e. fully developed or highly covering plant presence), standing dead or dying vegetation (SDV, i.e. senescence or dead maturity plants) and crop residue (CR, i.e. plant stems/stalks after harvesting). The objective of the present work is to define a paradigm for the classification of these different field conditions from satellite hyperspectral data, in order to create a reliable map to be used for: i) quantitative evaluation of NPV, to accurately separate standing dead vegetation and crop residues from other field conditions, prior to the retrieval of the variable of interest (crop residue and/or standing dead vegetation cover/biomass); ii) monitoring field condition over time and in turn soil conservation practices. To this aim, the approach is based on spectral analysis of the investigated surfaces, and spectroscopic modelling of absorption features. Machine-learning techniques are then used to infer decision rules valid for a series of satellite PRISMA images.
Materials & methods
The hypothesis at the base of this study is that the use of knowledge-based spectral features diagnostic of different surface characteristics – i.e. the presence of pigments, canopy water, cellulose-lignin, clay minerals – allows reducing dimensionality, preserving significant information, and increasing class separability. In addition, the method robustness when accounting for different crop types and growing seasons is assessed, for time series analysis.
As mentioned, the preliminary feature selection of diagnostic intervals is based on knowledge (Curran, Citation1989; Fourty, et al., Citation1996; Curran, Citation1989; Li et al., Citation2016; Berger et al., Citation2021); then, feature reduction is accomplished by Exponential Gaussian Optimization – EGO (Pompilio et al., Citation2010, Citation2009, Citation2014) – to retrieve feature parameters, which form a new feature space. On this space, machine learning is used to infer classification rules – by a Decision Tree approach – exploiting samples labelled according to in situ observations of the five classes of interest (BS, EV, GV, SDV, CR). Of such field data, part is used for training and part for validation. Classification is performed by applying the same decision tree at pixel scale, for a time series of PRISMA imagery acquired over two crop seasons. Then, classification results are aggregated at parcel level – on the basis of pixel predictions – to obtain thematic maps useful for farm-level agro-practices monitoring and assessment. Besides traditional rigorous validation of classification results, parcel level maps for the two seasons are analysed to assess temporal consistency, and compared to independent data regarding crop types, calendar, rotation and practices to evaluate information content.
Materials: PRISMA and field data
PRISMA was launched on 22 March 2019 by the Italian Space Agency (ASI) on a low-Earth, Sun-synchronous orbit at 615 km altitude. The mission takes advantage of the combination of: (i) two hyperspectral sensors in the spectral range 400–2500 nm (around 240 channels, overlapping between 920 and 1010 nm), with a ground sampling distance (GSD) of 30 m, and average spectral resolution of less than 10 nm; (ii) a panchromatic camera working in the spectral range 400–700 nm, with a GSD of 5 m. Revisiting time is 29 days at-nadir, but also off-nadir acquisitions are possible and frequently made to increase frequency over sites of interest, while PRISMA tasking is on demand. The system is important for the manifold applications requiring Hyperspectral narrow band data.
This study grounds on a dataset of PRISMA images (and concurrent field campaigns) acquired over a large farm in Northern Italy, which is a validation site belonging to scientific cal/val activities of the ASI PRISMA mission (PRISCAV project), and also a test site of ESA CHIME Mission. The observation period corresponds to two crop-seasons: 2019–2020 and 2020–2021, for the sake of clarity hereafter indicated as 2020 and 2021. Acquisitions are tasked and then standard Level 2D (L2D) data products (Bottom of Atmosphere Reflectance geocoded product) downloaded from PRISMA portal and used for this study. Only hyperspectral data are used; the two data cubes that compose L2D data – VIS-NIR and NIR-SWIR – are converted in a single hyperspectral cube of 231 narrowbands ranging from 400 to 2500 nm, with no overlaps, using the “prismaread” R package (Busetto & Ranghetti, Citation2020). Some geometrical inconsistency still remains in L2D products after orthorectification and geocoding, since the ground control point network for increasing geocoding accuracy was nonoperational at the time of data acquisition. Given that the error, at the scale of the study area, could be considered as just a shift in coordinates, we manually shifted (without further spatial transformation) image corners thus to align each scene to the study area field boundaries. reports the dates of satellite overpasses, together with the indication of image quality according to atmospheric condition and cloud presence.
Table 1. PRISMA acquisition dates and corresponding image quality on Jolanda di Savoia study area.
The farm belongs to Bonifiche Ferraresi S.p.A., the Jolanda di Savoia estate is located in the Po Plain (Emilia-Romagna region; 44°53′N; 11°59′E) and covers about 3800 ha, with a diversified crop production, as shown in .
Figure 1. Location of the Jolanda di Savoia estate, and crop maps of the study area for the seasons: a) 2020 and b) 2021.

Besides the crop maps, the ancillary data set available consists of farm information on agricultural practices carried out within the two seasons (e.g. types and temporal intervals of sowing, harvesting, soil preparation, etc.), direct observations of crop status (phenology, crop conditions), and spectro-radiometric measurements concurrent to some PRISMA overpasses. In-situ spectral measurements are not directly discussed in this study, although they were used to assess PRISMA L2D product quality, as part of PRISCAV project activities, and are part of the background knowledge regarding NPV and other surfaces’ spectral behaviour used for the methodology design (as in Pepe et al., Citation2020).
Methodology
The methodological phases of the study could be outlined in terms of four main steps:
PRISMA spectra extraction and analysis for the categories of interest according to field reference information;
knowledge-based Spectroscopic modelling of PRISMA data and statistical exploration of features extracted for each category;
PRISMA Classification by Machine-Learning: Training, Classification, and Validation of results at pixel level, and parcel level maps generation by polygon level aggregation;
analysis of parcel trajectories through time according to knowledge on cultivated crops and timing of management practices for two seasons.
Sample spectra extraction
A selection of reference spectra from PRISMA imagery is used for: i) the identification of diagnostic and distinctive spectral features; ii) modelling each absorption feature and iii) training the classification algorithm used for land-use classification.
According to farm information and field observations, we randomly extracted 150 pixels for each of the five categories of interest (total 750 sample spectra). Samples of crop residues (CR), emerging vegetation (EV), green vegetation (GV), and standing dead vegetation (SDV) were selected from image acquired on 21 June 2021, while bare soil (BS) samples were collected from both June 21 and April 24 images. In Northern Italy, during April farmers accomplish soil preparation and sowing of the summer crops such as maize, rice and soybean, while during June winter crops are in dead maturity condition or already harvested and summer crops are in vegetative stages. Therefore, few plots show exposed bare soil in April, that correspond to land preparation for following crops.
Spectroscopic modelling and data exploration
On the basis of a priori knowledge about the spectral characteristics of the investigated surfaces, and their diagnostic features, four spectral intervals are considered, as detailed below:.
Δλ1: centred near 680 nm and covering the red edge interval, related to canopy pigments content (PRISMA band interval [530–866 nm]);
Δλ2: centered at 1200 nm, and related to vegetation water content (PRISMA band interval [1078–1262 nm]);
Δλ3: centered at 2100 nm, and mainly related to the cellulose-lignin content in NPV (actually in vegetation, but detectable only in dry and non-photosynthetic vegetation) (PRISMA band interval [2036–2199 nm]);
Δλ4: centred near 2200 nm, and related to the presence of clay minerals in soils (PRISMA band interval [2183–2261 nm]).
All analyses are performed using R Statistical Software (v4.1.2; R Core Team, Citation2021). Continuum removal transformation of log reflectance spectra is computed per image pixel, according to the convex hull method in “hsdar” package (Lehnert et al., Citation2019). A convex hull continuum is established between the local maxima of the log reflectance in each referred wavelength interval, and then removed by subtraction. A Savitzky-Golay smoothing has been previously applied to reflectance spectra in order to prevent eventual spikes to be accounted as local maxima. Subsequently, the continuum removed spectra, as log reflectance, are modelled with an Exponential Gaussian function (Pompilio et al., Citation2009) which is displayed in (EquationEquation 1(1)
(1) ).
where λ is the wavelength, s is depth, xc the centre and w the width of the modelled absorption feature; t and k represent saturation and asymmetry of the absorption peak, as described in Pompilio et al. (Citation2009); (Citation2010). In this step of the methodology, the t value in the above equation is set to 0.001, and not allowed to vary during optimization of model residuals, because saturation effects are unlikely to occur in the features of interest.
Parameters returned by the Exponential Gaussian Optimization (s, xc, w and k per absorption feature) are used as a new and reduced feature space for image classification.
Before classification, in order to understand the behaviour of classes in the reduced feature space and to confirm evidences of the selected spectral intervals, a data exploration is accomplished by representing the distributions of parameters in the training set, according to the categories of interest.
Classification by machine learning
Once the hyperspectral space is reduced to a dimension of 16 (4 spectral intervals with 4 model parameters each), a machine-learning approach is used to classify the reduced PRISMA data according to the categories of interest. Since the feature extraction phase is based on well-known diagnostic absorptions, and the spectral information is preserved with spectroscopic modelling, a simple decision tree method was chosen. This technique also accounts for the possibility of an unmediated interpretation of decision rules. Thus, the third step (classification) is in accordance with a usual outline: training, classification and validation of the results.
The training of the Decision Tree (hereafter referred as DT) is accomplished using the 750 total samples extracted from images as described above. The “rpart” R package (Therneau & Atkinson, Citation2022) is used for the purpose. Then, the resulting rules are validated on the same set of training samples. This first assessment is referred in the literature as classifier accuracy, being an indication of the abstraction capabilities of the classifier, and accounts for the prediction capabilities of the DT model.
Classification accuracy
A quantitative assessment of classification results is accomplished with field data observations collected in an extensive campaign carried out on 21 June 2021, on a relevant part of the farm. To this aim, 300 independent pixels per class (1500 test samples) were randomly extracted from June 21 image in parcels belonging to the reference map. The test samples are used for deriving the confusion matrix and accuracy indices (Congalton, Citation1991) in order to evaluate the performance of classification results.
Then, the DT model is applied to all the reduced PRISMA images for both crop seasons. For each date the decision rules are applied at pixel level in order to generate: a) discrete classification maps; b) five probability maps (one per class), with the class probability values (percentages) corresponding to each pixel.
Finally, per pixel classified maps were spatially aggregated at parcel level, which is the desired scale being the parcel the minimal management unit in a farm, thus producing:
a map of predicted classes, obtained assigning, for each acquisition date, the class with the majority occurrence among pixels belonging to the parcel;
five maps of class probabilities (one per class), obtained for each acquisition date by averaging probability values of pixels belonging to the parcel.
Analysis of parcel trajectories over time
To study the temporal behaviour of mapping results more in detail, an analysis of parcel trajectories – assumed as changes in crop conditions over time – is also performed. The objective of this analysis is two-fold: i) account for the quality of DT predictions on the whole PRISMA data set (12 images), even when a reference field map is not available; ii) evaluate the applicability of the DT approach in the perspective of monitoring crop practices and land management. Analysis of trajectories was carried out by tracking the classes changes during time for each single parcel, given the crop type and cycles (i.e. main phenological phases, on the base of sowing/harvesting dates and those of some other management practices).
Detailed information on some parcels regarding sowing and harvesting, main phenological stages, together with some other information regarding winter and summer crops presence (e.g. crop rotation, crop calendars) for the two.
Results and discussion
Results
Exploratory data analysis
The average PRISMA reflectance spectra within each class of the training set (150 spectra per class), are shown in , together with their standard deviations. The spectral intervals considered for the EGO modelling are also highlighted with coloured boxes. By comparing the spectral behaviour of the five classes in each wavelength interval, the main absorption features are clearly observable, according to the respective spectral class. shows the best EGO fitting results in the classes of interest: VEG (Δλ1 and Δλ2); CR (Δλ3) and BS (Δλ4). As shown in , very weak features occur in Δλ3 and Δλ4 intervals, which are meaningful for the detection of crop residues and/or bare soil. However, EGO modelling is indeed able to preserve and enhance information that is subtle otherwise.
Figure 2. Average spectra of the selected samples for the five categories of interest (Bare Soil, Emerging Vegetation, Green Vegetation, Standing Dead Vegetation and Crop Residues); standard deviations are drawn in gray colour; coloured boxes display the four spectral intervals investigated.

Figure 3. Representative samples of EGO modelling results in the four spectral intervals of interest (Δλn). Each plot is subdivided into 2 sectors with different scales of reflectance values. On bottom, the target absorption feature in each wavelength interval (points), the continuum model (dashed lines) and the EGO fit (red lines); on top, the model residuals (dash-dot lines). Each feature is selected from spectra belonging to Green Vegetation (Δλ1 and Δλ2), Crop Residues (Δλ3) and Bare Soil (Δλ4) classes.

After the application of EGO fitting to all the spectra in the training set, distributions of the 16 resulting parameters (4 for each interval) are analysed by means of box plots, as already mentioned in the Methodology section and shown in .
Figure 4. Box plot distributions of band depth (a), centre (b), width (c), and asymmetry (d), as results from modelling the spectra of the training samples in each class of interest and wavelength interval (Δλ1 to Δλ4, as in ).

Looking at distributions in some considerations could be drawn.
As expected, within Δλ1 (canopy pigments’ absorption region) band depth (s) is significantly diagnostic of vegetation, either green or at emergence (i.e. GV and EV). In addition, the narrow distribution around average values of centre (xc), width (w) and asymmetry (k), for those two classes allows to further characterize the absorption feature within Δλ1 as diagnostic of vegetation. Thresholds of parameters are suitable of discriminating GV from EV classes.
Regarding Δλ2 (canopy water absorption region), it is interesting to notice that the green vegetation class shows band centre (xc) – with the highest values near 1160 nm –, band width (w) – the largest values – and band asymmetry (k) – the less skewed shape – as clearly diagnostic with respect to all the other classes.
As expected, within Δλ3 (cellulose-lignin absorption region), band depth (s) and width (w) are clearly different for SDV, CR and BS. Although the high average depth of BS class, even with a widespread distribution of values, we can distinguish BS on the hand from SDV and CR on the other hand based on the centre position (xc). Therefore, within the Δλ3 interval, CR and SDV classes are clearly separated from the remaining classes.
Within Δλ4 (clay minerals absorption region), there is no clear evidence of class separability.
Decision tree model and classification results
shows decision tree as trained with the 150 pixels per class extracted from the two PRISMA images for which field conditions were known. Looking at the resulting rules, the best splitting parameters are within the Δλ1 and Δλ3 spectral intervals, in accordance with the exploratory analysis. In particular, the band depth parameter is diagnostic to separate green vegetation and NPV-related classes (CR and SDV) from the others, while band centre of Δλ3 is conclusive to separate BS.
Figure 5. Decision tree model and rules resulting from a train set of 150 samples per class (750 total samples). Reference classes are colored as in the legend; percentages in each box refer to the split fraction of the corresponding class as resulted from the previous decision rule.

As introduced in the Methodology section, a first validation is accomplished to account for the performance in abstraction achieved by the DT, by producing the confusion matrix, with related indices, on the same samples used for training.
provides classifier accuracy assessment on the training set. An Overall Accuracy (OA) of 0.96 is obtained with a K coefficient close to 0.95. Moreover, both sensitivity and specificity resulted very high (0.94 and 0.97, respectively).
Table 2. Confusion matrix and indices for the abstraction accuracy (training samples: 750 pixels).
When it comes to the classification accuracy – as mentioned in the Methodology section – only for one date (21 June 2021), the knowledge of field conditions is wide-ranging enough in the farm for the creation of a reference map (see, ). Hence for this date, which is also one of the two of the training data (the one with the majority of sample pixels), the error matrix is computed by randomly extracting 300 pixels per class (500 total samples) from the classification map (see, ) to be compared with the reference map. This error matrix is reported in .
Figure 6. Base map and classification results. (a) PRISMA imagery acquired on 21 June 2021, in standard false color composite (R:NIR, G:RED, B:GREEN). (b) Reference map from field observations; (c) pixel level classification map; and (d) parcel level aggregated map. Legend in (c) and (d) is the same as for (b).

Table 3. Confusion matrix and indices for mapping results obtained from PRISMA data of 21 June 2021, as calculated on the base of the test sample (1500 pixels).
As a rule, the performance in prediction is less accurate than the one in abstraction, but anyway the drop is not so significant, while OA is 0.94 and K coefficient counts 0.92. The statistics at parcel level are alike the ones at pixel level, with 0.93 OA and K up to 0.90. This confirms the high performance in prediction of the decision tree, which is also highlighted in comparing mapping results (c at pixel and d at parcel level) with reference map (b).
Going into detail, pixel-level classification map () not only shows a good agreement with reference map, but also allows to identify patches that are reasonable according to physical condition of the target. In fact, CR (orange) patches occur in plots with winter crops (e.g. wheat, barley and emmer) that, at the moment of the image acquisition, were completely mature and not yet harvested (–SDV in yellow).
Given the high level of performance achieved, the DT is applied to the whole dataset, after hyperspectral data reduction by EGO modelling, at pixel level, and later aggregated at parcel level. Classification results at parcel level for the two crop seasons over the farm are reported in .
Figure 7. Base maps in standard false color composite and Classification maps at parcel level, as a result of the application of the Decision Tree model shown in , and subsequent aggregation at the parcel scale of the whole PRISMA time series 2020–2021 (acquisition dates are reported on top rows. The legend is the same as in

Analysis of parcel trajectories
Methodology
shows an example of the analysis of trajectories, performed as described in the Methodology section, useful for understanding the quality of mapping on two different parcels during the 2021 crop season. One parcel represents a winter crop (soft wheat), and the other parcel is an example of a summer crop (maize). The crop map on top of is the ancillary information provided by the commercial farm and used for the interpretation of trajectories. From the knowledge of sowing (31/10/2020 for wheat and 10/04/2021 for maize) and harvesting dates (01/07/2021 for wheat and 11/09/2021 for maize), together with crop calendar reports, some major phases (vegetative, reproductive, crop residue/bare soil) are derived and portrayed as coloured time bars. Soft wheat parcel is mapped with the following labels: as GV for acquisitions that occur during the vegetative and reproductive stages (from April to early June); as SDV at the end of reproductive phase, which is maturity (late June); as CR after harvesting. The only inconsistency is in late May acquisition, but this is undoubtedly due to cloud contamination (highlighted by grey circle in the image) not detected by the L2D cloud mask processor (see, false colour composite at the corresponding date).
Figure 8. Particular of the crop map (top) and classification results at pixel and parcel level (middle) for the growing season 2021. In particular two plots are highlighted (maize and soft wheat on top map) and the corresponding colored time bars are drawn (bottom). The time bars show the sequence of days of the year (DOY) from the beginning of March (day 60) up to the end of October (day 300), according to the main crop phenology phases derived from crop calendars and sowing and harvesting dates. PRISMA acquisition dates are also marked with vertical lines; dots and connectors are used to point out the corresponding maps.

Likewise, the maize parcel is mapped as BS before sowing (beginning of April) and at the very beginning of leaf development (late April); as EV during the vegetative stage (late May, beginning of June); as GV at the reproductive phase (late June); as SDV just before harvesting (which occurred the day after the PRISMA acquisition). The May PRISMA image is not hampered by clouds in the portion corresponding to this particular parcel.
As mentioned in the Methodology section, the parcel level aggregation process is used to produce not only parcel level maps, but also line graphs representing, for each parcel (identified by its ID number in the farm map), the trajectory of crop condition changes over the two seasons, according to parcel level class assignment and probability for each class on each acquisition date. Examples are shown in . As for parcel level maps, in these graphs the sequence of changes in crop conditions can be followed, but this representation is even more suitable to assess the mapping results in the perspective of monitoring/reporting crop practices and land management over time.
Figure 9. Example of parcel trajectories over time based on class probability. The plot referred to as 0238 underwent crop rotation from maize (Orange in the top left panel, season 2020) to soft-wheat (dark yellow in the top right panel, season 2021).

Figure 10. Same as for a plot referred to as 0358b, in which durum-wheat (dark yellow in top left panel, season 2020) is followed by maize (Orange polygons in top right panel, season 2021).

bottom panels provide representation of class assignment according to probabilities for the same plot in both seasons. It is apparent the 2020 sequence BS (April), EV (May), GV (June to July) and BS (September) is consistent with the development of maize crops during the summer season. Trajectories also reveal that in 2021 this particular plot was cultivated with a winter crop, according to the fact that the parcel is mapped first as GV (April to June), and later as CR (from end of June to September); this also means that after harvesting no tillage occurred in the field for at least two months.
shows another example, with a different crop rotation. Field 0358b was sown with durum wheat in 2020 and maize in 2021. Analysis of trajectories reveals the following sequence: 2020 observation starts with crop in vegetative status (GV, from April to May), getting mature crop before harvest (SDV, in June), and then harvested (CR, mid-July), followed by vegetation regrowth before tillage (EV, end of July to September); 2021 observation starts with maize sowing bed (BS, April), followed by crop emergence (EV, may and beginning of June), full crop development (GV, late June) and crop maturity (SDV, September).
Discussion
Feature reduction space with hyperspectral data
The spectroscopic approach, based on the selection of four representative spectral features in hyperspectral data and their modelling with Exponential Gaussian Optimization, allows to create a representative reduced (enhanced) feature space suitable for classification. So far, CAI (Cellulose Absorption Index) and LCAI (Ligno-Cellulose absorption index) are recognised as the most useful indices for crop residues estimation, as shown in Ali et al. (Citation2017) and references therein. Both indices require radiance measurements in at least 3 channels in the SWIR spectral region, at specific positions (shoulders and minimum of the lignin-cellulose absorption band). The computation of such indices is not allowed through actual broadband spectral sensors, and thus hyperspectral sensors, as well as properly designed multispectral sensors are required. The technique described in the present study is independent from the centre wavelength of the specific sensor channels involved in computation, although it requires hyperspectral narrowband sensors capable of measuring SWIR radiances. The improvement over CAI and LCAI which actually measure the relative depth of the lignin-cellulose absorption feature, is that EGO modelling of hyperspectral data is able of retrieving 4 parameters per absorption band, in order to account for the whole spectral shape of the diagnostic feature. However, according to Daughtry and Huntjr (Citation2008), the three narrow bands used for both CAI and the correction for moisture content, could be incorporated into advanced multispectral satellite sensors, such as Landsat 10, which are not yet available.
As the exploratory analysis shows (), EGO modelling allows for a transformation of the hyperspectral space into a reduced number of significant parameters, useful to separate classes of different field conditions. In particular, of the four investigated, at least three spectral intervals (Δλ1, Δλ2, Δλ3,) are well suited to identify parameters with high separability potential. The lack of a diagnostic parameter within the Δλ4 interval could be due to the fact that the absorption feature, where present, is quite narrow (also as compared to the other ones, see, ) and, as observed from in situ spectral observations concurrent with PRISMA overpasses and as already reported in the literature (Cogliati et al., Citation2021; Mzid et al., Citation2022), there is a known drop in radiance in this part of the SWIR spectrum of PRISMA data, due to lower signal to noise ratio.
Classification of field conditions with hyperspectral data
A machine-learning approach takes advantage of the modelled feature space for abstracting rules, which proved to be accurate and applicable on several images acquired in different seasons and in turn with different field/crop conditions, even if the training makes use of examples coming almost from one date. Indeed, the trained decision tree reaches a high level of accuracy in abstracting the classification rules from the sample (as shown in ); the few errors are anyway coherent with possible mixed field conditions (crop residue with bare soil, crop residue with emerging vegetation) or due to differences in cellulose-lignin abundance (crop residue and standing dead vegetation). The only surprising confusion is between EV and SDV, as observed in omission errors to class EV in , but as very few occurrences.
The performance of the classification method is very good at pixel level for the unique date in which a reference map is available, with an AO accuracy of 0.94 and a K coefficient of 0.92. Moreover, maps derived from images acquired in different dates (and partially shown in ) allow evidencing additional features: i) patches of SDV in fields mainly classified as CR corresponding to areas not yet harvested; ii) emergence vegetation pixels occur in green vegetation fields of summer crops, or in bare soil ones where patches of vegetation are likely to exist (weeds or vegetation regrowth); iii) big anomalous patches correspond to clouds/cloud shadow presence.
A brief discussion is required in order to account for the moisture effect. Both soil and residue moisture will affect the performance of Δλ3 and Δλ4 in terms of reducing the depth and width of the diagnostic features, as shown in Kokaly et al. (Citation2009), and Daughtry and Huntjr (Citation2008). However, the complete obliteration of the diagnostic features, due to water, which in turn determines the attribution to a different cover class, requires the saturation of terrains or canopy residues is reached. This condition has never been observed during this study. Nevertheless, an assessment of scene water content is crucial for accurately estimating crop residue cover when moisture conditions may vary from scene to scene or within a scene due to topography. A specific investigation of the behaviour of the classification algorithm here described, as a function of water content is required for quantitative mapping, which is beyond the purpose of this study.
A good agreement is still apparent while progressing to final map at parcel level (), with AO and K of 0.93 and 0.90 respectively. Overall, the temporal distribution of classes according to different field conditions is consistent with the expected conditions of the field, as inferred by farm information and direct observations through time. As an example, results show that the majority of bare soil parcels occur in April images, for both the years. During April, in fact, the plots are ploughed and sown with summer crops (i.e. maize, rice and soybean). On the other hand, classification results of late June images show several plots in classes of standing dead vegetation and crop residues. June is indeed the period of the year when winter crops are senescent or near to senescence or recently harvested.
Likewise, such performances remain high when final maps are evaluated for the whole time series by checking consistencies of condition changes in time with respect to other independent information (crop sowing and harvesting dates, soil preparation practices and phenological stages occurrence and crop rotation). An example of the best-case scenario could be as follows: bare soil at the beginning of the season is followed by vegetation at emergence, which become then green vegetation, and later on standing dead vegetation, followed by crop residues after harvesting and, after tillage/reduced tillage, ready to start again as bare soil for the following crop cycle.
The analysis of parcel trajectories demonstrates the consistency between DT predictions and field conditions. Obviously, since the acquisition frequency of PRISMA data is not constant, and both winter and summer crops have their own cycles, some crop conditions may not be captured in the time series. Moreover, vegetation regrowth over CR or weeds presence in different amounts could complicate the real cases.
This demonstrated that inferring simple rules based on known optical properties of the surfaces of interest, modelled through the EGO approach, lead to reliable results as referred to the objective of mapping crop conditions and field management. Classification maps, compared with indication about phenology/practices, proved to be consistent with the field crop conditions along the season.
As a final remark, we found that the two spectral intervals related to pigments and cellulose lignin absorption features are of utmost importance for classification accuracy, as confirmed for instance, by Daughtry et al. (Citation2005). Despite results of this study may suggest the other spectral ranges to be less important, we can presume that the use of different machine-learning approaches (e.g. PLSR, random forest) would take advantage also of their contribution. It is important to recall that this experiment is only a first step in the direction of quantitative Remote Sensing of NPV.
Study limitations and improvements
In our study, the supervision of classification is made once, and then the decision tree model is used over a time series including different seasons, and with several kinds of crops and conditions. In addition, the selection of the train sample is image-based. Although useful when no additional spectral dataset is available, this approach represents a limitation from a certain point of view. In fact, in order to widely apply the decision tree model to several types of field conditions (even those not observed in the present study) and for a quantitative investigation, a reference spectral library should be available and include a wide variety of surface spectra measured under controlled conditions. Those libraries should be used for training the machine-learning paradigm, so as to refine the splitting rules and account for a variety of crops and crop conditions, as well.
The same spectral libraries could also be useful for testing performances of various narrow-band remote sensors with possible different spectral configurations, since the Exponential Gaussian fitting of known absorption features allows for that. As a future vision, the approach described so far to the analysis of PRISMA data, in combination with soon available EnMAP (Environmental Mapping and Analysis Program, DLR) mission’s data, will allow additional surface covers showing diagnostic features in their spectra to be identified and mapped, thus representing a strong contribution to future operational missions, such as ESA CHIME (Copernicus Hyperspectral Imaging Mission).
To overcome some limitations and further improve the classification of field conditions in agricultural landscapes, we envisage the accomplishment of the following tasks: the exploitation of spectral libraries regarding NPV fractions, such as the one of USGS (Hively et al., Citation2021) for the creation of a large training sample modelled with EGO, useful to move ahead to a quantitative estimation of the relative fractions of the different categories of interest (NPV-related, green vegetation and soil); and the use of different machine-learning techniques for improving the classification of PRISMA imagery. Furthermore, simulated spectra, from Radiative Transfer Models, such as the recent developed PROSPECT-PRO (Feret et al., Citation2021) able to simulate the effect of carbon-based constituents on leaf spectra in relation to changing plant moisture content, could be used to widen the spectrum of training samples, under known crop (canopy) conditions. Further tuning of the classification is also planned, through the inclusion of the slope of continua in the reduced dataset, which could improve the results, especially in case of subtle absorption features.
Conclusions
We present a two-step classification approach for mapping crop conditions as related to NPV and soil conservation practices from spaceborne imaging spectroscopy PRISMA data. To achieve this, the approach encompasses spectroscopic and machine-learning techniques. At first, an Exponential Gaussian Optimization modelling is applied to four diagnostic spectral intervals, so that the hyperspectral space is reduced to the model parameters. Second, a Decision Tree is trained at pixel level, to infer decision rules for the classification of bare soil, emerging vegetation, green vegetation, standing dead vegetation and crop residues. Post processing involved the aggregation of classification results at a parcel level, which is the scale of interest, in particular when EO data will be used to monitor/report adoption at farm level of conservative agro-practices, being the parcel the minimum management unit.
The training data set belongs to reference crop conditions, surveyed for the most part on one date (21 June 2021) over a large farm in Italy. The DT is tested and validated on the corresponding image, reaching very accurate results both at pixel and parcel levels (overall accuracy and K coefficient greater than 0.9).
The same DT is successfully applied on a PRISMA hyperspectral image time series, which includes 12 images acquired on the test site during 2020 and 2021, and resulted in field condition maps at parcel level over the two crop seasons. The predictions performances have been assessed and analysed considering trajectories of target condition’s changes over time, which is also an information of interest for monitoring practices of soil conservation. The independent data set used, in this case, includes crop maps, sowing/harvesting calendars, rotation and land preparation practices, as provided by the commercial farm. The results of this analysis of trajectories proved that classification results are consistent with independent data, and confirm that the approach is accurate for field condition mapping. This also testifies that the exploitation of spectroscopic techniques constrained on known optical properties of the investigated surfaces, together with machine-learning classifiers, provide a reliable and transferable mapping workflow, to apply over time series.
Acknowledgments
The Authors would like to thank Lorenzo Genesio and Franco Miglietta (CNR-IBE), and Roberta Falone and Patrizio Tempesta (Telespazio) for their support for satellite data procurement. We are also very grateful to Giorgia Verza (CNR-IREA) for collecting data about phenological stages and crop practices. The authors also thank three anonymous reviewers for their helpful comments which contributed to manuscript improvement. Project carried out using ORIGINAL PRISMA Products - © Italian Space Agency (ASI); the Products have been delivered under an ASI License to Use.
Disclosure statement
No potential conflict of interest was reported by the author(s).
Additional information
Funding
References
- Ali, A. M., Darvishzadeh, R., Skidmore, A. K., & van Duren, I. (2017). Specific leaf area estimation from leaf and canopy reflectance through optimization and validation of vegetation indices. Agricultural and Forest Meteorology, 236, 162–16. https://doi.org/10.1016/j.agrformet.2017.01.015
- Arsenault, E., & Bonn, F. (2005). Evaluation of soil erosion protective cover by crop residues using vegetation indices and spectral mixture analysis of multispectral and hyperspectral data. Catena, 62(2–3), 157–172. https://doi.org/10.1016/j.catena.2005.05.003
- Asner, G. P. (1998). Biophysical and biochemical sources of variability in canopy reflectance. Remote Sensing of Environment, 64(3), 234–253. https://doi.org/10.1016/S0034-4257(98)00014-5
- Asner, G. P., & Heidebrecht, K. B. (2002). Spectral unmixing of vegetation, soil and dry carbon cover in arid regions: Comparing multispectral and hyperspectral observations. International Journal Remote Sensing, 23(19), 3939–3958. https://doi.org/10.1080/01431160110115960
- Bannari, A., Staenz, K., Champagne, C., & Khurshid, K. S. (2015). Spatial variability mapping of crop residue using Hyperion (EO-1) hyperspectral data. Remote Sensing, 7(6), 8107–8127. https://doi.org/10.3390/rs70608107
- Berger, K., Hank, T., Halabuk, A., Rivera-Caicedo, J. P., Wocher, M., Mojses, M., Gerhátová, K., Tagliabue, G., Dolz, M. M., Venteo, A. B. P., & Verrelst, J. (2021). Assessing non-photosynthetic cropland biomass from spaceborne hyperspectral imagery. Remote Sensing, 13(22), 4711. https://doi.org/10.3390/rs13224711
- Berger, K., Verrelst, J., Féret, J. B., Wang, Z., Wocher, M., Strathmann, M., Danner, M., Mauser, W., & Hank, T. (2020). Crop nitrogen monitoring: Recent progress and principal developments in the context of imaging spectroscopy missions. Remote Sensing of Environment, 242, 111758. https://doi.org/10.1016/j.rse.2020.111758
- Busetto, L., & Ranghetti, L. (2020). prismaread: A tool for facilitating access and analysis of PRISMA L1/L2 hyperspectral imagery v1.0.0. URL https://irea-cnr-mi.github.io/prismaread/
- Cogliati, S., Sarti, F., Chiarantini, L., Cosi, M., Lorusso, R., Lopinto, E., Miglietta, F., Genesio, L., Guanter, L., Damm, A., Pérez López, S., Schefflerh, D., Tagliabue, G., Panigada, C., Rascher, U., Dowling, T. P. F., Giardino, C., & Colombo, R. (2021). The PRISMA imaging spectroscopy mission: Overview and first performance analysis. Remote Sensing of Environment, 262, 112499. https://doi.org/10.1016/j.rse.2021.112499
- Congalton, R. G. (1991). A review of assessing the accuracy of classifications of remotely sensed data. Remote Sensing of Environment, 37, 35–46. https://doi.org/10.1016/0034-4257(91)90048-B
- Curran, P. J. (1989). Remote sensing of foliar chemistry. Remote Sensing of Environment, 30(3), 271–278. https://doi.org/10.1016/0034-4257(89)90069-2
- Daughtry, C. S. T. (2001). Discriminating crop residues from soil by shortwave infrared reflectance. Agronomy Journal, 93(1), 125–131. https://doi.org/10.2134/agronj2001.931125x
- Daughtry, C. S. T., & Hunt, E. R.Jr. (2008). Mitigating the effects of soil and residue water contents on remotely sensed estimates of crop residue cover. Remote Sensing of Environment, 112(4), 1647–1657. https://doi.org/10.1016/j.rse.2007.08.006
- Daughtry, C. S. T., Hunt, E. R., Doraiswamy, P. C., Jr, & McMurtrey III, J. E. (2005). Remote sensing the spatial distribution of crop residues. Agronomy Journal, 97(3), 864–871. https://doi.org/10.2134/agronj2003.0291
- Daughtry, C. S. T., Hunt, E. R., & McMurtrey, J. E. (2004). Assessing crop residue cover using shortwave infrared reflectance. Remote Sensing of Environment, 90(1), 126–134. https://doi.org/10.1016/j.rse.2003.10.023
- European Commission. (2018). Directorate-general for agriculture and rural development. Modernising and simplifying the CAP—Challenges for agriculture and rural areas: Environment and climate dimensions. In Agriculture and rural development background documents. EC.
- Féret, J.-B., Berger, K., de Boissieu, F., & Malenovský, Z. (2021). PROSPECT-PRO for estimating content of nitrogen-containing leaf proteins and other carbon-based constituents. Remote Sensing of Environment, 252, 112173. https://doi.org/10.1016/j.rse.2020.112173
- Féret, J. B., Berger, K., de Boissieu, F., & Malenovský, Z. (2021). PROSPECT-PRO for estimating content of nitrogen-containing leaf proteins and other carbon-based constituents. Remote Sensing of Environment, 252, 112173. https://doi.org/10.1016/j.rse.2020.112173
- Haddaway, N. R., Hedlund, K., Jackson, L. E., Kätterer, T., Lugato, E., Thomsen, I. K., Jørgensen, H. B., & Isberg, P. E. (2017). How does tillage intensity affect soil organic carbon? A systematic review. Environmental Evidence, 5, 1. https://doi.org/10.1186/s13750-016-0052-0
- Hank, T. B., Berger, K., Bach, H., Clevers, J. G. P. W., Gitelson, A., Zarco-Tejada, P., & Mauser, W. (2019). Spaceborne Imaging Spectroscopy for Sustainable Agriculture: Contributions and Challenges. Surveys in Geophysics, 40, 515–551. https://doi.org/10.1007/s10712-018-9492-0
- Hively, W. D., Lamb, B. T., Daughtry, C. S., Serbin, G., Dennison, P., Kokaly, R. F., Wu, Z., & Masek, J. G. (2021). Evaluation of SWIR crop residue bands for the landsat next mission. Remote Sensing, 13(18), 3718. https://doi.org/10.3390/rs13183718
- Hively, W. D., Lamb, B. T., Daughtry, C. S., Shermeyer, J., McCarty, G. W., & Quemada, M. (2018). Mapping crop residue and tillage intensity using WorldView-3 satellite shortwave infrared residue indices. Remote Sensing, 10(10), 1657. https://doi.org/10.3390/rs10101657
- Kokaly, R. F., Asner, G. P., Ollinger, S. V., Martin, M. E., & Wessman, C. A. (2009). Characterizing canopy biochemistry from imaging spectroscopy and its application to ecosystem studies. Remote Sensing of Environment, 113(Supplement 1), S78–S91. https://doi.org/10.1016/j.rse.2008.10.018
- Lal, R., Kimble, J. M., Follett, R. F., & Cole, C. V. (1999). The potential of U.S. cropland to sequester carbon and mitigate the greenhouse effect. Lewis Publishers.
- Lehnert, L. W., Meyer, H., Obermeier, W. A., Silva, B., Regeling, B., Thies, B., & Bendix, J. (2019). Hyperspectral data analysis in R: The hsdar package. Journal of Statistical Software, 89(12), 1–23. https://doi.org/10.18637/jss.v089.i12
- Li, Z., & Guo, X. (2016). Remote sensing of terrestrial non-photosynthetic vegetation using hyperspectral, multispectral, SAR, and LiDAR data. Progress in Physical Geography, 40(2), 276–304. https://doi.org/10.1177/0309133315582005
- Marshall, M., Belgiu, M., Boschetti, M., Pepe, M., Stein, A., & Nelson, A. (2022). Field-Level crop yield estimation with PRISMA and Sentinel-2. ISPRS Journal of Photogrammetry and Remote Sensing, 187, 191–210. https://doi.org/10.1016/j.isprsjprs.2022.03.008
- Mzid, N., Castaldi, F., Tolomio, M., Pascucci, S., Casa, R., & Pignatti, S. (2022). Evaluation of agricultural bare soil properties retrieval from landsat 8, sentinel-2 and PRISMA satellite data. Remote Sensing, 14(3), 714. https://doi.org/10.3390/rs14030714
- Pepe, M., Pompilio, L., Gioli, B., Busetto, L., & Boschetti, M. (2020). Detection and classification of non-photosynthetic vegetation from PRISMA hyperspectral data in croplands. Remote Sensing, 12(23), 3903. https://doi.org/10.3390/rs12233903
- Pompilio, L., Pedrazzi, G., Cloutis, E. A., Craig, M. A., & Roush, T. L. (2010). Exponential gaussian approach for spectral modelling: The EGO algorithm II. Band asymmetry. Icarus, 208(2), 811–823. https://doi.org/10.1016/j.icarus.2010.03.020
- Pompilio, L., Pedrazzi, G., Sgavetti, M., Cloutis, E. A., Craig, M. A., & Roush, T. L. (2009). Exponential Gaussian approach for spectral modeling: The EGO algorithm I. Band saturation. Icarus, 201(2), 781–794. https://doi.org/10.1016/j.icarus.2009.01.022
- Pompilio, L., Pepe, M., Pedrazzi, G., & Marinangeli, L. (2014). Informational clustering of hyperspectral data. IEEE Journal of Selected Topics in Applied Earth Observations and Remote Sensing, 7(6), 2209–2223. https://doi.org/10.1109/JSTARS.2013.2294053
- Ranaivoson, L., Naudin, K., Ripoche, A., Affholder, F., Rabeharisoa, L., & Corbeels, M. (2017). Agro-ecological functions of crop residues under conservation agriculture. A review. Agronomy for Sustainable Development, 37(4), 1–17. https://doi.org/10.1007/s13593-017-0432-z
- R Core Team. (2021). R: A language and environment for statistical computing. R Foundation for Statistical Computing. URL https://www.R-project.org/
- Roberts, D. A., Smith, M. O., & Adams, J. B. (1993). Green vegetation, nonphotosynthetic vegetation and soils in AVIRIS data. Remote Sensing of Environment, 44(2–3), 255–269. https://doi.org/10.1016/0034-4257(93)90020-X
- Smith, P., Soussana, J. F., Angers, D., Schipper, L., Chenu, C., Rasse, D. P., Batjes, N. H., Van Egmond, F., McNeill, S., Kuhnert, M., Arias‐Navarro, C., Olesen, J. E., Chirinda, N., Fornara, D., Wollenberg, E., Álvaro‐Fuentes, J., Sanz‐Cobena, A., & Klumpp, K. (2020). How to measure, report and verify soil carbon change to realize the potential of soil carbon sequestration for atmospheric greenhouse gas removal. Global Change Biology, 26(1), 219–241. https://doi.org/10.1111/gcb.14815
- Tagliabue, G., Boschetti, M., Bramati, G., Candiani, G., Colombo, R., Nutini, F., Pompilio, L., Rivera-Caicedo, J. P., Rossi, M., Rossini, M., Verrelst, J., & Panigada, C. (2022). Hybrid retrieval of crop traits from multi-temporal prisma hyperspectral imagery. ISPRS J. Photogramm. Remote Sens, 187, 362–377. https://doi.org/10.1016/j.isprsjprs.2022.03.014. Under revision.
- Therneau, T., & Atkinson, B. (2022). rpart: Recursive Partitioning and Regression Trees. R package version 4.1.16. https://CRAN.R-project.org/package=rpart
- Th. Fourty Baret, F., Jacquemoud, S., Schmuck, G., & Verdebout, J. (1996). Leaf optical properties with explicit description of its biochemical composition: Direct and inverse problems. Remote Sensing of Environment, 56(2), 104–117. https://doi.org/10.1016/0034-4257(95)00234-0
- Verrelst, J., Rivera-Caicedo, J. P., Reyes-Muñoz, P., Morata, M., Amin, E., Tagliabue, G., Panigada, C., Hank, T., & Berger, K. (2021). Mapping landscape canopy nitrogen content from space using PRISMA data. ISPRS Journal of Photogrammetry and Remote Sensing, 178, 382–395. https://doi.org/10.1016/j.isprsjprs.2021.06.017
- Zheng, B., Campbell, J. B., & de Beurs, K. M. (2012). Remote sensing of crop residue cover using multi-temporal Landsat imagery. Remote Sensing of Environment, 117, 177–183. https://doi.org/10.1016/j.rse.2011.09.016