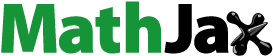
ABSTRACT
In order to improve the effect of urban road congestion analysis, this paper aims to study the mechanism of traffic congestion diffusion under the condition that users have real-time traffic information, and analyze the urban road congestion situation combined with big data visualization technology. According to the characteristics of traffic flow propagation, this paper conducts multi-granularity abstraction and multi-scale modeling of node-intersection-link-network for the complex and dynamic traffic congestion process, and establishes an improved SIS virus propagation model for traffic congestion propagation. In addition, this paper uses the method of state transition probability to construct an interactive dynamic model of traffic congestion propagation and early warning information propagation in a multi-layer network. The experimental research results show that the big data visualization technology introduced in this paper can play an important role in urban road congestion.
Introduction
The rapid increase in urban vehicle ownership is the main factor leading to urban traffic congestion, but the unreasonable traffic planning, design methods and road control strategies are also important factors. The contradiction between motor vehicles’ demand for the transportation system in time and space and the outdated road infrastructure and management methods is becoming increasingly acute. The reason why there is serious traffic congestion at road intersections is that it regulates the flow of traffic in all directions, and the repeated diversion, merging and crossing of vehicles have caused conflicts in time and space, and the road situation has become very complicated. Traffic congestion are produced by a physical impairment or obstruction on the roadway, which forces automobiles to migrate into some other channels. The extent to which this kind of interruption affects flow of traffic determined by the number of vehicles that must combine in a given location in a predetermined period of time. Moreover, in the operating rules of level intersections, intersection conflict points, merging points, and diversion points often affect vehicle speed, road capacity, and driver’s psychological characteristics, leading to imbalances in the dynamics of people, vehicles, and roads, resulting in traffic congestion. Facing the increasingly severe “urban vascular disease”, many scholars have proposed various models and methods to solve traffic congestion.
Traffic flow is produced by the movement of various vehicles on prescribed roads, and is the aggregation of traffic demand and supply in a limited time and local space. The traffic flow system is a typical self-driving multi-particle complex giant system far away from equilibrium. Among them, the evolution of traffic flow is a complex nonlinear dynamic process, and the phase change mechanism from steady-state flow, to meta-stable flow, to crowded flow is not yet clear (Chen et al., Citation2019). In traffic control, nonlinear dynamics of traffic jams refers to the shift from instabilities in traffic flow to the establishment of traffic jams. The features of traffic flow are by far the most straightforward way to examine an air traffic system, and also a precondition for implementing efficient relating to decision administration in air traffic control. Phase space reconstructions, repetition graph, and other techniques were used to highlight the nonlinear dynamic properties of air traffic flow from numerous points of view. The study of traffic flow requires the cross-penetration and mutual development of multiple disciplines such as fluid mechanics, nonlinear dynamics, statistical physics, behavioral science, information technology, computer science, and traffic engineering (Zhang et al., Citation2018). It’s an Information digital big data platform that gives consumers a multiprocessing area and a collaboration forum for publishing multimodal traffic data, as well as mapping and solving complex traffic issues. The main content of traffic flow research is to comprehensively apply knowledge of behavioral science, traffic engineering and information science, use mathematical and physical models to describe vehicle movement, and reveal the self-organized evolution of urban traffic flow and the emergent trajectory of congestion. It provides effective help for preventing and alleviating urban traffic congestion, and provides a scientific basis for urban planning and decision-making (Carter et al., Citation2020). These insights can be used to detect pedestrian hotspot and to advise potential traffic light and roadway configuration sites in attempt to implement the city more passenger friendlier. Furthermore, the knowledge obtained can be applied to other sets of data, such as bicycle traffic, to advise city infrastructure improvements.
As an indispensable element of modern residents’ travel, with the development of the economy and society and the advancement of science and technology, the various performances such as rapidity, comfort, and aesthetics are also getting higher and higher. At present, the transportation options for residents to travel mainly include: bicycles (including electric), motorcycles, buses (buses, subways, light rails, etc.), taxis, private cars and official vehicles, etc. In transportation research, transportation can be classified into two categories: motor vehicles and non-motor vehicles. Most of the motor vehicles in many foreign cities are small cars, the types of vehicles are relatively simple, and the speed of the vehicles is basically the same on a certain road section, so the traffic flow is relatively simple. The motor vehicles in my country’s cities are complex, with large differences in speed and performance, so the traffic flow is more complex.
This article aims to study the mechanism of traffic congestion diffusion under the condition that users have real-time traffic information, and seek appropriate theories and technologies. Moreover, this paper combines big data visualization technology to provide theoretical guidance for formulating traffic congestion management measures, which is academically attractive and practical for solving urban traffic problems. After this section, recent survey on traffic congestion monitoring and regulatory survey path analysis is discussed. Following, interactive communication model of traffic congestion and early warning information and eventually role of big data visualization technology in urban road congestion is carried out and ended up with conclusion
Related work
In order to control urban road traffic congestion, the literature (Stipancic et al., Citation2019) mainly has four main principles: the principle of reducing the total amount of traffic, the principle of equalizing the traffic volume, the principle of traffic continuity and the principle of traffic separation, and proposed three types of countermeasures for traffic congestion control: one is The total amount of traffic demand is controlled. On the one hand, the use of public transportation is encouraged through the collection of license fees, congestion charges and other oriented policies; Urban road capacity. The second is traffic system management, strengthening the degree of informatization of urban roads and improving the technical content of urban traffic management, so as to realize the coordination and cooperation of various traffic elements such as people, vehicles and roads. The genesis of portable navigation systems used in vehicle transport can be traced back to urban traffic control. This is attributable to the fact that the urban environment quickly showed the difficulties produced by the widespread use of automobiles, as well as the possibility for communications-based solutions to solve them. The third is to optimize the road traffic structure, including strengthening the construction of the urban express backbone road network and vigorously developing rail transit. As an important method to improve the urban transportation structure, the bus priority policy has been favored by many experts in recent years. Projects encouraged by foreign public transport priority policies include rapid bus systems and rail transit. Literature (Zheng et al., Citation2019) puts forward the basic principle of giving priority to the development of public transportation in cities. Literature (Brennan et al., Citation2019) pointed out that to truly reflect the “public transport priority”, it is necessary to give priority to public transport policy, technology, awareness and planning, and put forward many good suggestions for the implementation of “public transport priority”. In terms of technical research, literature (Yang et al., Citation2020) has done some research on the setting form of dedicated bus lanes; literature (Kumar et al., Citation2018) has discussed the signal control strategy of bus priority intersections, and literature (Grayson, Citation2020) has discussed the design of dedicated bus entry lanes at intersections. The setting, the benefit evaluation of bus priority, and the optimal bus ratio for bus-only passages are studied. Another concentrated research direction of congestion management is the hierarchical management of urban traffic congestion. You can categorize cities and build a link between transportation structure and important urban metrics using hierarchical road congestion control. As a result, sidewalks will improve, pollutants capita emissions will diminish, and health statistics will improve. Literature (Kuftinova et al., Citation2021) uses the principle of fuzzy mathematics to construct a spatiotemporal hierarchical model of regional traffic state, which covers the microscopic, mesoscopic and macroscopic traffic parameters of the traffic network, including the spatiotemporal information of the traffic network and the traffic state information, which can combine different traffic conditions in the road network. It is recommended that a fuzzy inference-based strategy be used to account for the uncertainties of the different congestion metrics. It needs to take into account the difference in speed among crucial hours, the link between automobile quantities and roadway section capacities, and the proportion of average maximum allowable velocity. The intersections of the traffic state are separated into different levels of accessibility. On this basis, the accessibility of the intersection, the connectivity of the road section and the traffic state of the road network are analyzed, and the time-space state information of congestion is analyzed. Literature (Cai et al., Citation2018) puts forward the general theory of urban market, and the contradiction of building roads is increasingly intensified; this is the reason why traffic management departments analyze the cause of congestion in a specific city and a specific traffic environment, but it may not be applicable to other cities, and it lacks universality. Literature (Celes et al., Citation2019) analyzes and summarizes the research on the causes of traffic congestion by many scholars, and summarizes the general reasons for urban traffic congestion: the overall level of government management of road traffic is not high. Specifically, the total amount of traffic has not been reasonably controlled, and the pace of road construction is lower than the development of automobiles; the urban layout is unreasonable, people cannot freely choose where to live, and shopping, school, and work cannot be solved nearby; road construction, transportation organization Lack of scientific planning, unreasonable road network structure, low design standards, insufficient functions, incomplete facilities and low traffic capacity in road construction. The traffic structure is unreasonable, especially the development of public transport is lagging behind; the pricing of transport vehicles is distorted and the development is unbalanced; the development and utilization of existing road resources are not high, the management level is low, and the scientific and technological content is low; the quality of professional teams including traffic police is not high. Literature (Shan et al., Citation2018) believes that the traffic management in most cities is still at the level of empirical management, and changes in the day and night, orders that do not work, or random travel orders occur from time to time; the use of roads is also unscientific, individual roads are divided into traffic, and the phenomenon of mixed traffic has not been obtained. Good governance; the overall synergy of the participation of all relevant departments and the linkage of the whole society has not been fully formed; the public’s traffic awareness is not commensurate with the times. This inductive research method can identify some major influencing factors from a macro perspective, but the analysis of the problem is not deep enough, and it only stays at the level of comments, which cannot serve as a good reference.
High-level traffic regulation such as rational planning of urban spatial layout and industrial layout can most effectively reduce traffic congestion (Kong et al., Citation2019), but the duration of urban planning and traffic planning determines the inevitability of urban transformation. The co-occurrence pattern in human mobility has demonstrated great utility in parts of urban planning, commercial, and social applications, including such shopping online marketing strategy formulation and disease transmission. To process trajectory data and uncover urban regional characteristics, temporal partitioning and mapping segment were used. When urban population and vehicle ownership reach a certain level At the limit, the past planning has been unable to meet people’s traffic needs, and at this time, immediate technologies and means are needed to alleviate traffic congestion, such as the use of traffic engineering technology, traffic planning and management methods, traffic flow control and induction, etc. to adjust and control . In recent years, a large number of technologies and means have been applied to the traffic management of the actual traffic network. However, due to insufficient understanding of the diffusion law of traffic congestion; little is known about the effect of the measures taken to alleviate traffic congestion; most of the management measures implemented. It is based on experience and lacks scientific theoretical guidance, resulting in frequent traffic jams that plague people’s daily production and life (Welch & Widita, Citation2019).
Model of interactive communication of traffic congestion and early warning information
The problem of urban traffic congestion has become a serious problem faced by many cities in the world today. When urban traffic is severely congested, it is usually accompanied by the dissemination of “road congestion” warning information, which affects human travel activities, which in turn affects the spread of traffic congestion to a certain extent. In order to deeply analyze the dynamic evolution process of urban traffic network congestion, this chapter studies the establishment of an improved SIS virus propagation model for traffic congestion propagation. This paper uses the state transition probability method to analyze the dynamic behavior characteristics of traffic congestion and the interactive communication of early warning information based on a multi-layer complex network, and reveals the impact of early warning information dissemination on the propagation of traffic congestion. Because the number of vehicles on the road is increasing, the road maintenance personnel is finding it difficult to satisfy the demands for prompt road repairs. As a result, an early warning system is useful for identifying road building difficulties and disseminating information about the issue in order to move forward. Dynamic Evaluation splits the urban traffic system into market and road networks using complicated network theories. Then, depending on the types of the demand network and the road network, several connectivity models were built.
The network structure factors that govern the propagation of infections in traffic flow are shown by the Susceptible-Infected-Susceptible (SIS) transmission model. The infection rate is related to the movement among divisions in the strength of the association providing a self-regulatory influence on disseminating dynamics, whereas the propagation model provides an increased flow of issues that occurred in the congested traffic. The classic SIS virus transmission model is generally used to describe diseases in which the recovered individual cannot possess immunity, such as influenza. Therefore, in the SIS virus propagation model, nodes are divided into two categories: susceptible nodes and infected nodes. In the process of virus transmission, when an infected node encounters a susceptible node, the infectious disease will be transmitted to the susceptible node at the infection rate . At the same time, due to the role of the individual’s own safety mechanism after the infection, the recovery rate
will be transformed into a susceptible node. Moreover, once the susceptible node is infected, it will act as the infected node and continue to spread the virus.
Compared with the transmission process of the SIS virus, the transmission of traffic congestion has a similar transmission process. However, due to the complex characteristics of the transportation system, its propagation process has typical multi-scale interaction characteristics (node-intersection-section-network). In the process of traffic congestion propagation, when the congested node turns into a smooth node at the recovery rate , the recovery process is related to the road conditions of the downstream road. The more unobstructed roads downstream of the intersection, the shorter the time it takes to dissipate the congestion. However, the recovery process of the infected node has nothing to do with the state of the surrounding node (Chen et al., Citation2019).
Therefore, this paper improves the SIS virus propagation model and divides nodes into two categories: unblocked nodes (corresponding to susceptible nodes) and congested nodes (corresponding to infected nodes). When the traffic congestion propagation rate exceeds a certain threshold, the congested node will use the propagation rate
to turn the surrounding unblocked nodes into congested nodes. At the same time, in the process of congestion dissipating, the congested node will be transformed into a smooth node with the dissipation rate
due to the traffic conditions of the surrounding nodes.
In the information communication network, the node set is the same as that of the urban transportation network, and the edge represents whether the nodes can communicate normally. For information communication networks, a normal-warning-normal (NWN) information dissemination model is used to describe the dissemination of “road congestion” warning information in the network. For traffic information sharing, the NWN information dissemination paradigm offers flexibility, adaptability, and ease of maintenance. When a vehicle gets information from some other vehicle, it modifies its data stored and leaves it up delivering the knowledge until the next broadcasting time. Because the amount of broadcast messages is restricted but they do not flood the system, the distribution technique ought to be sustainable. In the NWN information dissemination model, network nodes have two forms: nodes that have and can forward warning information (that is, the state of the node is W) and nodes that do not have warning information or have limited communication (that is, the state of the node is N). In the process of dissemination of early warning information, when drivers obtain the “road congestion” warning information, they will often consider re-selecting the route, thereby reducing the traffic volume entering the congested section and speeding up the dissipation of congestion. However, individuals who have not received the warning information will not take measures to reduce the risk of congestion (Zhou et al., Citation2018).
We assume that there are two main sources of early warning information: one is from the node that generated and issued the early warning information in the information communication layer, that is, the node without early warning information communicates with the node that issued the early warning information, and the early warning is received with probability and transform it into a node with warning information. The other is from the congested nodes in the traffic network layer, that is, the congested nodes in the traffic network layer spontaneously turn into nodes with early warning information in the communication network layer. At the same time, as the road traffic changes from a congested state to a smooth state, the early warning information gradually stops disseminating, which causes the nodes with early warning information to become nodes without early warning information with probability
.
In the above-mentioned traffic congestion-early warning information interactive communication model, we assume that the probability of congestion at the unwarned traffic exit is , and the probability of congestion dissipating is
. However, the probability of congestion occurring at the early warning of unobstructed traffic crossing is
, and the probability of congestion dissipating is
. It is known that the probability of congestion at the clear traffic entrance that has been warned is generally lower than that of the unwarned traffic entrance. At the same time, the traffic congestion intersections that have been warned take less time to dissipate than the traffic congestion intersections that have not been warned.
Therefore, we assume that the probability of congestion occurring at a clear traffic entrance that is warned is corresponding to a factor times the traffic congestion that is not being warned, that is,
. However, the unwarned traffic congestion intersection is equivalent to the traffic congestion intersection that is warned and the congestion dissipation process is correspondingly performed by a factor
times, that is,
. As a special case,
means that there will be no congestion at the early warning traffic entrance. In the same way,
means that the unwarned traffic congestion intersection will not change the congestion state in a short time (Liu et al., Citation2020).
Based on the above-mentioned traffic congestion-warning information interactive dissemination mechanism, this paper divides the nodes in the multi-layer network into three categories: traffic intersections that are warned, traffic congestion intersections that are warned, and traffic intersections that have not been warned. The three corresponding states are: Warning-Susceptible (WS) state, Warning-Infected (WI) state and Normal-Susceptible (NS) state. Warning Susceptible insist the user to move and analyses the channel that may reduce the flow of traffic and sometimes it may lead to turbulence in case of no interaction. Human operational errors, such as inattention, misbehavior, and distraction, are the primary causes of normal susceptibility. It might have aided in the improvement of driving safety and the reduction of traffic accidents. In the dynamic interaction process, we assume that the unwarned traffic congestion intersection will immediately be converted into the traffic congestion intersection that has been warned. Therefore, the normal-infected (Normal Infected, NI) state can be ignored in describing the traffic congestion-early warning information interactive propagation model. In summary, the state transition diagram of different types of nodes in the traffic congestion-warning information interactive propagation model is shown in , where the main involved symbols and specific descriptions involved are shown in . Depending on the input information, the interactive propagation model is in charge of identifying the commonly occurring dispersion pathways on the network of the state’s road system and estimating the transmission probability and estimated dissemination durations connected with the pathways.
Figure 1. Schematic diagram of node state transformation of traffic congestion-early warning information model in multi-layer network.

Table 1. The recognition effect of big data visualization technology in urban road congestion.
We assume that the total number of nodes in each layer of the complex network in the multi-layer network is M, and and
represent the adjacency matrix of the information communication network and the urban transportation network, respectively. The mathematical description of a topographical road infrastructure is an adjacency matrix. It clusters neighboring links that happen at about the same time and defines the nearest neighbor relation, which is connected to the reasons for non-recurrent congestion events. When node i and node j are adjacent, the value is 1. Otherwise, it is 0. At the initial moment, a node i is randomly selected in the network as a traffic congestion node with congestion information.
We assume that and
are the probability that node i is in the WI, WS, and NS states at time t, respectively. Since the interactive communication model of traffic congestion warning information satisfies the continuous-time Markov process, it satisfies the normalization condition, as shown in formula (1; Zhu et al., Citation2018). A continuous-time Markov model is a continual random process of transforming state including an exponential random variable for every state and then moves to a new state as prescribed by the probability of a random vector.
Using the probability of node i in each state at time t to describe the dynamics of each node, the following traffic congestion propagation dynamics equation can be established:
Among them, is the probability that node i in the communication network layer is affected by neighbors with early warning information to receive the early warning information,
is the probability of the node changing from unblocked to ed under the influence of the congested node with early warning information, and
is the probability of the occurrence of congestion and dissipation under the condition of warning information.
Among them, there are:
According to the traffic congestion-early warning information interactive propagation dynamics equation established above, it can be seen that there are many parameters that affect the propagation of traffic congestions. Therefore, it is necessary to find the influential parameters that have an important role through threshold analysis. When , the total number of nodes in the three states NS, WS and WI are
,
respectively. Using steady-state analysis methods, this set of dynamic equations can be used to obtain the critical value of propagation. This paper replaces
in the equations with
, and
with
. According to condition (1), formulas (3) and (4) can be rewritten as (Zin et al., Citation2018):
Among them, there are:
Formulas (11) and (12) are a nonlinear cloud as a mechanical system, and the initial node of the system is a fixed point. It can analyze the local stability of formulas (11) and (12) at the initial point through the Jacobian matrix:
Among them, is the identity matrix. The identity matrix allows us to display the structure of currently recognized road traffic problems as well as potential solutions based on periods for each proportion.
When is satisfied, the initial node is locally stable. Thus, we can get:
Among them, and
are the maximum eigenvalues of matrix B and matrix A, respectively. It can be seen from formula (19) that the parameters
and
are the most influential parameters, and their value will greatly affect the propagation of traffic congestion. At the same time, the maximum eigenvalues of matrix B and matrix A will also affect the propagation of traffic congestions. Therefore, the propagation process of traffic congestion is not only related to the traffic congestion propagation threshold and the traffic congestion dissipation threshold, but also to the dynamics of early warning information dissemination in the traffic network.
In order to verify the correctness of the propagation critical value analyzed by the above-mentioned state probability equation and further analyze the role of the influencing parameters in the propagation process of traffic congestion, BA scale-free network and WS small-world network are used as the topological structure model of complex network urban transportation network. Furthermore, we assume that the two layers of the two-layer network are both the same BA scale-free network or WS small-world network, and the network scales are both M = 1000. Based on Monte Carlo simulation, at the initial moment of traffic congestion propagation and early warning information propagation, a node is randomly selected from the traffic network layer as the traffic congestion node. At the same time, the randomly selected node is the first node with “road congestion” warning information in the information communication layer. The steady-state density of congested nodes in the multi-layer network is and the steady-state density of nodes with early warning information is
.
Since , when
, it means that nodes with warning information will not be congested, that is,
. When
, it means that the node with early warning information has not taken measures to reduce the probability of congestion, that is,
. shows the relationship between the steady-state density of congestion and the congestion propagation rate
for three different traffic congestion propagation models (that is,
) under the scale-free network and the small-world network. We assume that the pre-warning information dissemination rate is
, the pre-warning information disappearing rate is
, the traffic congestion dissipation rate with pre-warning information is
, and the multiple is
. shows that the interactive propagation model of congestion warning based on the multi-layer network has enhanced the critical value of traffic congestion propagation and significantly reduced the scale of traffic congestion outbreaks. In addition, the congestion-early warning interactive propagation model
does not cause congestion at all nodes with early warning information. It is obviously more effective in reducing the risk of traffic congestion outbreak than the interactive propagation model in which nodes with early warning information are congested with a probability of
. Moreover, it more effectively restrains the spread of congestion in the road network, and reduces the scale of the final outbreak of traffic congestion.
Figure 2. When , the change of
with
of the traffic congestion model.

Since , when
, it means that the node that has not been warned will not dissipate in a short period of time, that is,
. When
, it means that the process of dissipating traffic congestion is affected by the dissemination of early warning information, that is,
. shows the relationship between the congestion steady-state density and the congestion dissipation rate of three different traffic congestion propagation models (that is,
) under the scale-free network and the small-world network. The dissemination rate of early warning information is
, the disappearance rate of early warning information is
, the dissipation rate of traffic congestions with early warning information is
, and the multiple is
. According to the simulation results in , it can be seen that the dissipating process of traffic congestion with early warning information can significantly reduce the scale of traffic congestion. In the dissipation of congestion, it is simple to develop closed-loop congestion phenomena. The creation of the tails waits returning to the congestion of the beginning point is known as a congestion closed loop. In the absence of artificial intervention, traffic congestion is tough to disperse after it has established a closed circuit. Especially, when the dissipating process of traffic congestion is affected by the dissemination of early warning information
, the dissemination of early warning information has a greater impact on the scale of the congestion outbreak.
Figure 3. When , the change of
with
of the traffic congestion model.

In the actual road network, the scale of the traffic congestion outbreak is not only related to the traffic congestion propagation threshold at the congested intersection, but also related to the congestion dissipation capacity of the intersection. In addition, intersections that receive early warning information will improve their ability to resist congestion. shows the impact of different congestion dissipation probability on the scale of congestion outbreak under the condition of pre-warning information. It can be seen from the figure that under the scale-free network and the small-world network, the greater the impact of early warning information on the ability to dissipate congested intersections, the larger the congestion propagation threshold, and the smaller the scale of congestion outbreaks. It maintains node speed at low population densities, but grows quasi-exponentially above that of a threshold, where the information transfer speed is steady at the average level underneath the vehicle density threshold.
Figure 4. Traffic congestion-early warning information interactive propagation model under different values.

shows the relationship between the congestion propagation scale , the information dissemination scale
and the traffic congestion propagation rate
under different early warning information dissemination rates
. We assume that the node with early warning information reduces the degree of congestion occurrence as
, the disappearance rate of early warning information is
, the dissipation rate of traffic congestion with early warning information is
, and the multiple is
. shows that in scale-free networks and small-world networks, as the early warning information dissemination rate
increases, the scale of congestion dissemination and the scale of information dissemination decrease at the same time. This means that the spread of early warning information in the network slows down the spread of congestion and reduces the scale of congestion outbreaks.
Research on the application of big data visualization technology in urban road congestion
Analysis of urban traffic statistics and the influence of vehicle emissions on urban air quality is aided by big data visualization techniques. Individuals and government entities can use effective visualizations to spot trends, discover busy road segments at specified times, and implement and manage traffic sensors. Because road transportation is a major source of pollution, the influence of traffic on pollution levels has become a different concept that traffic visualization is addressing. The urban road traffic congestion identification technology based on big data visualization processing is implemented in two steps. One is the extraction of traffic characteristic parameters based on video processing, and the other is the discrimination of traffic congestion levels. The extraction of traffic characteristic parameters based on video processing is achieved through steps such as background modeling, vehicle target extraction, and tracking. The features required to work consistently in the hard situation experienced while scanning a vehicle head in both including overnight situations are combined in image acquisition. The resulting visual clarity is perfect for autonomous locating and recognizing. The traffic congestion judgment is realized on the basis of the acquired traffic characteristic parameters, as shown in . The following introduces the traffic characteristic parameter acquisition and the design method of traffic congestion discrimination based on video processing.
describes the process of segmentation of vehicle congestion areas based on big data analysis, and the segmentation is achieved through the method of template matching. Template matching is the technique of connecting a cleaned and noise canceling templates to an image sequence, allowing the image to be partitioned into distinct textured sections. Fitting a template to a message when the templates have a place of interruption might be understood as detecting the position of the discontinuities, a more specific task known as change point detection. The transportation industry has high hopes for leveraging Big Data, which has been made possible by the widespread adoption of Intelligent Transport Systems. The Vehicle Detection System was installed on a road infrastructure, which increased the network’s scale, density of system to detect distribution, and cognitive needs. After being separated, the two corner vertices are connected to divide a rectangular frame into two parts.
Figure 7. Regional separation effect diagram.

shows a case diagram of the big data visualization of vehicle congestion.
On the basis of the above research, the effect of the model proposed in this paper is verified, and the effect of the big data visualization technology proposed in this paper in the recognition of urban road congestion and the analysis effect of congestion is verified, and the results are shown in below.
Table 2. The analysis effect of big data visualization technology on urban road congestion.
From the above research, it can be seen that the big data visualization technology proposed in this paper can play an important role in urban road congestion.
Conclusion
Traffic flow is the study of a very complex multi-body system, and no single simplified method or technology can meet the requirements. At present, experts and scholars from various fields such as mathematics, physics, traffic engineering, and information technology are participating in the research of traffic flow, and are looking for reasonable and feasible methods and models to explain and analyze various characteristic phenomena of traffic flow. Therefore, scientific understanding of the process and evolution of traffic congestion will provide a scientific basis for the effective prevention and alleviation of traffic congestion and the design and planning of urban roads and highways. This article aims to study the mechanism of traffic congestion diffusion under the condition that users have real-time traffic information, seek appropriate theories and technologies, and combine big data visualization technology to provide theoretical guidance for the formulation of traffic congestion management measures. The experimental research results show that the big data visualization technology proposed in this paper can play an important role in urban road congestion.
Disclosure statement
No potential conflict of interest was reported by the author(s).
Data availability statement
All data generated or analyzed during this study are included in the manuscript.
References
- Brennan, T. M., Gurriell, R. A., Bechtel, A. J., & Venigalla, M. M. (2019). Visualizing and evaluating interdependent regional traffic congestion and system resiliency, a case study using big data from probe vehicles. Journal of Big Data Analytics in Transportation, 1(1), 25–12. https://doi.org/10.1007/s42421-019-00002-y
- Cai, L., Zhou, Y., Liang, Y., & He, J. (2018). Research and application of gps trajectory data visualization. Annals of Data Science, 5(1), 43–57. https://doi.org/10.1007/s40745-017-0132-1
- Carter, E., Adam, P., Tsakis, D., Shaw, S., Watson, R., & Ryan, P. (2020). Enhancing pedestrian mobility in smart cities using big data. Journal of Management Analytics, 7(2), 173–188. https://doi.org/10.1080/23270012.2020.1741039
- Celes, C., Boukerche, A., & Loureiro, A. A. (2019). Crowd management: A new challenge for urban big data analytics. IEEE Communications Magazine, 57(4), 20–25. https://doi.org/10.1109/MCOM.2019.1800640
- Chen, X., Wang, H. H., & Tian, B. (2019). Visualization model of big data based on self-organizing feature map neural network and graphic theory for smart cities. Cluster Computing, 22(6), 13293–13305. https://doi.org/10.1007/s10586-018-1848-1
- Grayson, J. (2020). Big data analytics and sustainable urbanism in internet of things-enabled smart governance. Geopolitics, History, and International Relations, 12(2), 23–29. https://dx.doi.org/10.22381/GHIR12220203
- Kong, X., Li, M., Li, J., Tian, K., Hu, X., & Xia, F. (2019). CoPFun: An urban co-occurrence pattern mining scheme based on regional function discovery. World Wide Web, 22(3), 1029–1054. https://doi.org/10.1007/s11280-018-0578-x
- Kuftinova, N. G., Ostroukh, A. V., Karelina, M. Y., Matyukhina, E. N., & Akhmetzhanova, E. U. (2021). Hybrid smart systems for big data analysis. Russian Engineering Research, 41(6), 536–538. https://doi.org/10.3103/S1068798X21060137
- Kumar, D., Wu, H., Rajasegarar, S., Leckie, C., Krishnaswamy, S., & Palaniswami, M. (2018). Fast and scalable big data trajectory clustering for understanding urban mobility. IEEE Transactions on Intelligent Transportation Systems, 19(11), 3709–3722. https://doi.org/10.1109/TITS.2018.2854775
- Liu, F., Andrienko, G., Andrienko, N., Chen, S., Janssens, D., Wets, G., & Theodoridis, Y. (2020). Citywide traffic analysis based on the combination of visual and analytic approaches. Journal of Geovisualization and Spatial Analysis, 4(2), 1–17. https://doi.org/10.1007/s41651-020-00057-4
- Shan, Z., Pan, Z., Li, F., Xu, H., & Li, J. (2018). Visual analytics of traffic congestion propagation path with large scale camera data. Chinese Journal of Electronics, 27(5), 934–941. https://doi.org/10.1049/cje.2018.04.011
- Stipancic, J., Miranda-Moreno, L., Labbe, A., & Saunier, N. (2019). Measuring and visualizing space–time congestion patterns in an urban road network using large-scale smartphone-collected GPS data. Transportation Letters, 11(7), 391–401. https://doi.org/10.1080/19427867.2017.1374022
- Welch, T. F., & Widita, A. (2019). Big data in public transportation: A review of sources and methods. Transport Reviews, 39(6), 795–818. https://doi.org/10.1080/01441647.2019.1616849
- Yang, C., Clarke, K., Shekhar, S., & Tao, C. V. (2020). Big spatiotemporal data analytics: A research and innovation frontier. International Journal of Geographical Information Science, 34(6), 1075–1088. https://doi.org/10.1080/13658816.2019.1698743
- Zhang, J., Chen, Z., Xu, Z., Du, M., Yang, W., & Guo, L. (2018). A distributed collaborative urban traffic big data system based on cloud computing. IEEE Intelligent Transportation Systems Magazine, 11(4), 37–47. https://doi.org/10.1109/MITS.2018.2876560
- Zheng, L., Xia, D., Chen, L., & Sun, D. (2019). Understanding citywide resident mobility using big data of electronic registration identification of vehicles. IEEE Transactions on Intelligent Transportation Systems, 21(10), 4363–4377. https://doi.org/10.1109/TITS.2019.2940724
- Zhou, Y., Lau, B. P. L., Yuen, C., Tunçer, B., & Wilhelm, E. (2018). Understanding urban human mobility through crowdsensed data. IEEE Communications Magazine, 56(11), 52–59. https://doi.org/10.1109/MCOM.2018.1700569
- Zhu, L., Yu, F. R., Wang, Y., Ning, B., & Tang, T. (2018). Big data analytics in intelligent transportation systems: A survey. IEEE Transactions on Intelligent Transportation Systems, 20(1), 383–398. https://doi.org/10.1109/TITS.2018.2815678
- Zin, T. A., Lwin, K. K., Sekimoto, Y., & Sekimoto, Y. (2018). Estimation of originating-destination trips in Yangonby using big data source. Journal of Disaster Research, 13(1), 6–13. https://doi.org/10.20965/jdr.2018.p0006