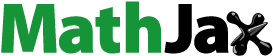
ABSTRACT
The geometric and polarimetric characteristics of the melting layer (ML) during the pre-summer rainy season over South China (PRSC) are investigated from 389 S-band polarimetric radar volume scans. The existing ML detection algorithm is slightly improved, in which the ML is automatically detected by using vertical gradients of radar reflectivity factor for horizontal polarizations (ZH), and co-polar cross-correlation coefficient (ρHV) from quasi-vertical profiles. And these profiles are obtained by azimuthal averaging of polarimetric radar variables at antenna elevation 19.5°. The comparison between the detected ML top and the 0°C isotherm heights from ERA5 demonstrates that the detection algorithm has high accuracy with an offset of 158 m. Due to larger snowflakes, the average ML thickness in PRSC is thicker (i.e. 150 m) than that in other climatic regions. The ML is characterized by large ZH, large differential reflectivity (ZDR) and small ρHV, with average values of 32 dBZ, 0.91 dB and 0.94. Furthermore, the peak values of ZH and ZDR in the ML are obviously larger than that from rain regions and snow regions, while the opposite is true for ρHV distribution.
Introduction
The melting layer (ML herein) is usually found beneath the 0°C isotherm. In the ML, ice-phase particles (e.g. ice crystals and snowflakes) melt and gradually evolve into rain droplets (Ryzhkov & Zrnic, Citation2019). During melting process, the shapes, dielectric properties and densities of particles change due to the increment of the liquid water fraction (Bringi & Chandrasekar, Citation2001), which lead to appearance of the bright band (BB) characterized by enhancement of equivalent radar reflectivity factor in radar scans (Austin & Bemis, Citation1950). Developing a thorough understanding of the ML is essential for the operational radar applications and the research of cloud microphysics. In particular, the accurate ML designation (i.e. the intensity, height, and thickness) will improve the quantitative precipitation estimation (QPE), the hydrometeor classification (Qiao et al., Citation2021), and the correction of radar attenuation (Falconi et al., Citation2016).
In the past, the conventional weather radars were only able to observe the BB. At present, many radars are equipped with dual polarimetry (Huuskonen et al., Citation2014; Kumjian, Citation2013), which can provide polarimetric measurements, such as the radar reflectivity factor for horizontal and vertical polarizations (ZH/V), differential reflectivity (ZDR), specific differential phase (KDP), and co-polar cross-correlation coefficient (ρHV). Except BB, the ML also is characterized by a drop in ρHV and peaks in ZDR measurements (Giangrande et al., Citation2008; Wolfensberger et al., Citation2016). In the ML, the addition of liquid meltwater on the particles increases the pre-existing variability in particle shapes and orientations by giving them a larger complex relative permittivity. As a result, ρHV is significantly lower (0.9) in the ML than in stratiform rain or in solid precipitation (Giangrande et al., Citation2008; Yao et al., Citation2016). Meanwhile, the accumulation of liquid water also produces a larger refractive index, which causes to ZH and ZDR increase. For example, small, pristine crystals that begin to melt can produce very large ZDR values of 6 dB (Schuur et al., Citation2014).
Based on these distinctive weather radar signatures, the meteorologists have developed several algorithms to locate ML in the past decades (Allabakash et al., Citation2019; Gourley & Calvert, Citation2003; Sánchez-Diezma et al., Citation2000; Wolfensberger et al., Citation2016; Xiao et al., Citation2010). In the early stages, many studies (Fabry & Zawadzki, Citation1995; Rico‐Ramirez & Cluckie, Citation2007; Xiao et al., Citation2010) used the vertical profile of radar reflectivity (VPR) and the gradients of reflectivity to identify the height, depth, and peak value of BB. However, the BB is one of the typical features of the ML, and the melting process begins above the BB (Willis & Heymsfield, Citation1989). Adding more measurements will help to identify the ML more comprehensively and accurately. Therefore, the ML detection techniques based on the polarimetric radar have been developed (Baldini & Gorgucci, Citation2006; Brandes & Ikeda, Citation2004; Hall et al., Citation2015). Matrosov et al. (Citation2007) combined the traditional VPR and ρHV to determine the ML boundaries. Giangrande et al. (Citation2008) designated the ML top and bottom rather than producing a single estimate of the ML height, using ZH, ZDR and ρHV measurements. Its root-mean-square error (RMSE) is as low as 200 m within 60 km of the radar range. Sanchez-Rivas and Rico-Ramirez (Citation2021) evaluated all possible combinations of polarimetric radar measurements and found the algorithm achieves the best ML detection when combining ZH and ρHV the gradient of the radial velocity.
In general, the operational radars only contain several plan position indicators (PPI) with low vertical resolution. But the quasi-vertical profiles (QVPs) technique can provide new useful insights into the bulk vertical precipitation structure of widespread storms, even those that exhibit horizontal heterogeneities (Kumjian & Lombardo, Citation2017). The QVPs are constructed via azimuthally averaging data collected at high fixed-antenna-elevation angles and converting the range coordinate to height (Ryzhkov et al., Citation2016). In the last decade, the QVPs have been widely used to study the microphysical characteristics of the dendritic growth layer and ML (Griffin et al., Citation2018, Citation2020; Kumjian & Lombardo, Citation2017). Therefore, the QVPs data with high vertical resolution can also be applied to the ML identification (Lee et al., Citation2020; Sanchez-Rivas & Rico-Ramirez, Citation2021).
South China is one of the rainiest regions in the world. Especially, a large number of heavy rainfall events with stratus cloud occur from April to mid-June, which is known as pre-summer rainy season over South China (PRSC, Luo et al. (Citation2020)). Its prediction remains challenging in operational forecasting and research communities (Huang & Luo, Citation2017; Wu et al., Citation2020). A better understanding of cloud microphysics in PRSC is favorable for the improvement of numerical weather prediction. At present, most studies on the ML focus on high latitude regions, such as North China and Jianghuai region (Hu et al., Citation2022; Shusse et al., Citation2011; D. Wang et al., Citation2012; Yang et al., Citation2019). But little research has studied the characteristics of the ML during the PRSC. In recent years, many polarimetric radars have been deployed in this region, which provides reliable data for our in-depth study of the microphysical characteristics of ML.
In this paper, we will document the characteristics of ML during the PRSC. In section 2, the S band polarimetric radar and the dataset are described as well as data processing (i.e. quality-control and QVPs). The ML detection algorithm is explained in detail in section 3. Section 4 illustrates statistical characteristics of the ML. Then the relationship between ML, rain and snow area are given in section 5. Finally, section 6 gives a summary of the main results and concludes this work.
Data and methods
S band polarimetric radar
To improve our understanding of melting layer during the PRSC, scans from the operational S-band polarimetric radars located in South China have been analyzed. In the last 5 years, these radars have been upgraded to dual-polarization in the same way as the polarimetric upgrade to Weather Surveillance Radar-1988 Doppler (WSR-88D) radar (Wang et al., Citation2019). The spatial distribution of the five polarimetric radars in Guangdong province is shown in , in which the red dotted circles represent the detection ranges (50 km) of the polarimetric radar. The basic radar performance parameters are shown in . The scanning strategy for the polarimetric radar is the volume coverage pattern 21 (VCP21) scanning mode, which consists of full scans at nine elevations (0.5°, 1.5°, 2.4°, 3.3°, 4.3°, 6°, 9.9°, 14.6°, 19.5°) with a volumetric update interval of 6 min.
Figure 1. The topography (gray shaded region) of Guangdong and the S band polarimetric radar location (blue dot). The red dotted circles represent the radar observation ranges of 50 km.

Table 1. The parameters of the S-band polarimetric radar.
Pre-processing of radar data
The non-meteorological echo and non-standard blockage on the polarimetric radar measurements is substantial and cannot be ignored. The non-standard blockage is mitigated following the procedure documented in Tang et al. (Citation2013); while the non-meteorological echo is removed by fuzzy logic hydrometeor classification (Park et al., Citation2009). The other quality control processes include ρHV, SNR and KDP threshold checks; despeckling; 7-point median filtering; and 7-point radial smoothing. The details of the quality control procedures can be found in Wang et al. (Citation2018).
After strict quality control, the polarimetric radar observations of 7 storms during 2019 and 2020 are used to analyze the ML in PRSC. provides the details of these storms, including the dates, radars, and periods. All storms occurred after the conventional weather radar were upgraded to dual-polarization capabilities. The dataset used in this study includes a total of 389 polarimetric radar volume scans.
Table 2. 7 ML events over South China, including their dates, radars, and periods.
Quasi-vertical profiles
The input data for ML detection algorithm come from QVPs of the polarimetric radar variables (Broeke et al., Citation2016; Kumjian et al., Citation2013; Ryzhkov et al., Citation2016). In this study, QVPs are obtained via azimuthally averaging of the data from 19.5° elevation PPI and converting the range coordinate to height. Only the gates with ρHV above 0.8 and ZH above 5 dBZ are used to calculate QVPs. QVPs geometry shows that the effective vertical resolution depends on the range resolution, beam width and the slant range, for example, to about 100 m at 2 km height and 400 m at 8 km height for the S-band polarimetric radar. Though such averaging (e.g. over circles of 28 and 57 km at 5 and 10 km height, respectively) tends to filter out fine scale horizontal structures, the averaging reduces the statistical errors of the radar variables’ estimates. Because the standard deviations of the ZDR and ΦDP estimates are proportional to M−1/2, where M is the number of samples.
The QVPs presented in is a time-versus-height format for the period 0300–0700 UTC on 20 April 2019, which spans 39 standard volume scans updated every 6 min.
Figure 2. The height-vs-time representation of QVPs of (a) ZH (unit: dBZ), (b) ZDR (unit: dB), and (c) ρHV (unit: −1) retrieved from Guangzhou polarimetric radar data collected at elevation 19.5°on 20 April 2019. Overlaid are contours of ML bottom and top.

illustrates the evolution of the convective and stratiform part of the squall line as it passed over the Guangzhou polarimetric radar with high vertical resolution. The convective part lasts from 0318 to 0418 UTC (), corresponding to ZH and ZDR values larger than 40 dBZ and 1.5 dB, respectively. At about 0530 UTC, the melting layer signature is characterized by ZH exceeding 45 dBZ, ZDR exceeding 2.4 dB at the bottom of ML, and ρHV reduced to 0.9.
shows another QVPs of ZH, ZDR and ρHV generated from the Shanwei polarimetric radar data collected throughout a meso-scale convective system case at elevations of 19.5° on 13 June 2019. One can see an obvious ML between 0312 and 0418 UTC (), in which ZH is between 35 and 45 dBZ, ZDR is close to 1.8 dB, and ρHV is less than 0.9. Throughout the QVPs herein (some events not shown), the ML is indicated by enhanced ZH and ZDR and a low ρHV around 5 km height.
Detection of the ML based on QVPs
From the results of the previous studies (Trömel et al., Citation2019; Wolfensberger et al., Citation2016) and section 2.3 of this study, the ML is characterized by the combination of a layer of small ρHV values, a transition from low to high ZDR and the presence of high values in ZH on polarimetric QVPs or RHI scans. Therefore, based on the vertical gradient of the polarimetric variables, a ML detection algorithm could be developed (Sanchez-Rivas & Rico-Ramirez, Citation2021). In this study, we adapted the ML detection algorithm introduced by Wolfensberger et al. (Citation2016) for RHIs to QVPs.
ML detection
Firstly, ZH and ρHV are normalized (i.e. [10, 60] dBZ → [0, 1] for ZH and [0.65, 1] → [0, 1] for ρHV), in order to give a similar weight to both variables (). And then, the normalized ZH and ρHV are combined into a single parameter – the melting layer factor MLF = ZH(1-ρHV). Subsequently, the vertical gradients of ZH, ρHV and MLF are calculated. In order to detect only gradient extremes that are strong enough to correspond to a potential ML edge, only data points with absolute value larger than 0.02 are kept. At last, the maximum of the vertical MLF gradient and the minimum of the vertical ZH gradient between 1 km and 6 km above the radar site are taken – different from Wolfensberger et al. (Citation2016), p. – as the bottom, top of ML (). It should be noted that the results with the following conditions would be eliminated: 1) the ML top is below the ML bottom; 2) the average of ρHV within the melting layer is larger than 0.96 or less than 0.8; 3) the rate of valid ZH below 7 km is less than 70%. The ML depth is then defined as the height difference between ML top and bottom; the extremal values of ZH, ZDR and ρHV are determined within this height interval.
Figure 4. (a) Normalized values and (b) gradients of ZH and ρHV and the MLF around the ML on a QVP recorded at Guangzhou (0500 UTC 4 April 2019).

Two examples of ML detection during stratiform situations are shown in . The ML bottom matches well the sharp transition to smaller values of ρHV and larger ZDR inside the ML and the ML top corresponds well with the top of the BB. It should be noted that the QVPs before 0400 UTC contain a very short ML detection event, but these QVPs should be classified as convective region (). Therefore, it is a misidentification. In future studies, we will introduce the convective and stratus clouds separation technique to reduce misidentification in convective regions.
Comparison between the ML top and the 0°C isotherm
The sounding is the most accurate data for evaluating ML detection algorithm, but these soundings are only available twice per day, and only the Heyuan radar has corresponding sounding observations. Therefore, we will choose the fifth-generation European Centre for Medium-Range Weather Forecasts reanalysis (ERA5, Hersbach et al. (Citation2018)) as the truth, which has comparable accuracy to the sounding (not shown). The horizontal (vertical) resolution of ERA5 is 0.25° (~600 m). We obtain the 0°C height from the variables of temperature and geopotential through bilinear (inverse distance-weighted) interpolation at the horizontal (vertical) direction. The time interval of ERA5 is 60 minutes, while that of radar scans is 6 minutes. To deal with this issue, only the radar scans at the same time as ERA5 were used for the algorithm evaluation.
Although the ML top and the 0°C isotherm do not coincide, their height comparison is still a reliable way to evaluate the ML detection algorithm. Therefore, presents the comparison of the ML top height obtained from the polarimetric QVPs and the ERA5-derived 0°C isotherm. The results show that most height pairs are located above the 1:1 line, suggesting that the ML top is below the ERA5-derived 0°C. Specifically, the average heights of ML top and ERA5-derived 0°C isotherm are 4577 and 4735 m, respectively. The physical process behind this phenomenon is that it takes the ice-phase particles some time in positive temperatures to actually start melting, which is also documented by Romatschke (Citation2021). In terms of RMSE and mean absolute error (MAE), the corresponding values of this study are 259 and 215 m, which is comparable to that of S-band polarimetric WSR-88D radar (RMSE: 220 m) in North America Giangrande et al. (Citation2008), and larger than that in X-band polarimetric radar with MAE of 94 m (Wolfensberger et al., Citation2016).
Characteristic of the ML
In this section, we present the characteristic of the ML from the datasets presented in . Since the summer storms with stratiform cloud consist of nearly 400 scans and thousands of data points, the boxplots, composite histograms, and composite density scatterplots are used to demonstrate the statistical relationships of the polarimetric variables more effectively.
First, the ML geometry (i.e. ML thickness, altitudes of ML top and bottom) is described by the boxplots in . The mean altitudes of ML top and bottom are 4793 m and 4299 m with standard deviation of 432 m and 424 m (), respectively. While the ML thickness has an average of 493 m and a standard deviation of 122 m (). The ML thickness is larger than those of Wolfensberger et al. (Citation2016) and Griffin et al. (Citation2020) about 150 m. Giangrande et al. (Citation2008) observed typical ML thicknesses of approximately 300 m with a long right tail and Wolfensberger et al. (Citation2016) documented the ML thicknesses for the summer storms in Europe ranging between 175 and 600 m, with an average of 320 m. The ML thickness of winter storms over USA has been observed close to 340 m according to the polarimetric WSR-88D radar data (Griffin et al., Citation2020). On the basis of cloud microphysics theory, the larger snowflakes fall faster and take more time to melt, thus the ML thickness increases (Fabry & Zawadzki, Citation1995). The maximum ZDR in the ML (2.0 dB) for this study is obviously larger than that (1.3 dB) for USA (Griffin et al., Citation2020), which indicates the summer storms over PRSC have larger drops than the winter storms from other regions. The statistics of polarimetric variables will be discussed in detail in subsequent section.
Figure 6. Boxplots of the ML (a) top, bottom and (b) thickness, the mean and standard deviation are provided in the bottom-right-hand corner of the panel.

shows the histograms of ZH, ρHV, ZDR and Kdp in the ML derived from the QVPs time series for 7 events.
Figure 7. Histograms of (a) ZH, (b) ρHV, (c) ZDR and (d) Kdp in the ML. The quantile 10% and 90% are given by the red lines in each panel.

Average values are provided together with the 10th and 90th percentiles for all variables, which enables a direct comparison with the ML statistics from the rest of the world. In , the distribution of ZH in the ML ranges from 5 to 52 dBZ, with an overall average of 32 dBZ (), and ρHV in the ML ranges between 0.8 and 1.0, with an average of 0.94 (). Also, illustrates corresponding ZDR between −1.0 and 4 dB, with an average of 0.91 dB. A comparison of results from other studies (i.e. in Giangrande et al. (Citation2008), Fig. 15 in Wolfensberger et al. (Citation2016) and in Griffin et al. (Citation2020)) shows that, ZH distributions are obviously skewed toward higher value near 30 dBZ, while ZDR is mainly distributed between 0 and 2 dB, and the peak value of ρHV appears around 0.95.
Except that, these distributions also exhibit some differences in detail. KDP is more closely related to liquid water than ZH and ZDR. In , Kdp in the ML ranges between −0.5 and 1.2 deg km−1, with an average of 0.14 deg km−1. The distribution of Kdp is similar to that of Wolfensberger et al. (Citation2016), who observed mean Kdp in the ML of 0.20 deg km−1 with values ranging between approximately −0.5 and 1.5 deg km−1 at X band. While Griffin et al. (Citation2020) observed mean Kdp of 0.02 deg km−1 with values ranging between approximately 0.0 and 0.25 deg km−1, which is recorded for the winter storms of USA. In contrast, the dataset of this study was recorded in summer, a season during which high water content often occurs over South China, so part of this discrepancy could be due to this sampling effect.
shows a joint probability density distribution of minimum ρHV versus maximum ZDR in the ML.
Figure 8. The joint probability density distribution of minimum ρHV vs maximum ZDR in the ML (dB) in the ML. The black line represents the linear regression and the fitting equation is provided in the top of graph.

Maximum ZDR ranges mostly from 0.6 to 4.4 dB, with corresponding minimum ρHV between approximately 0.8 and 0.96. It is evident from that negative correlations (i.e. r = −6.3) exist between the variables and an almost linear relationship between both,
The ice phase particles begin to melt as they enter the ML, their density and dielectric properties both increase, and larger partially melted snowflakes will lead to an increase in ZDR and a decrease in ρHV (Griffin et al., Citation2020). Since ρHV is a polarimetric variable representing the shape, size, orientation, and phase composition of the particles. From the ML top to the ML bottom, due to the amount of liquid water is increasing, also the type, shape, and orientations of hydrometeors are diversifying, ρHV decreases through the ML.
Similar scatterplot of maximum ZH versus maximum ZDR in the ML is presented in . In the ML, maximum ZH varies between 12 and 58 dBZ, while maximum ZDR ranges from 0.6 to 4.4 dB. Not surprisingly, the particle size in the ML quantified by maximum ZDR increases with the intensity of ML (maximum ZH). The fitting relationship between the variables is
Relationship between ML, rain, and snow
Many studies (Fabry & Zawadzki, Citation1995; Griffin et al., Citation2020; Matrosov et al., Citation2017; Trömel et al., Citation2019) have shown that there are some differences between polarimetric radar measurements within ML, rain regions and snow regions. For example, the melting of ice phase hydrometeors in the ML makes its reflectivity significantly higher than that in the rain regions. This phenomenon is often referred to as the BB, and results in overestimating of the rain rate at the surface. Therefore, it is important to quantitatively analyze the difference of polarimetric radar measurements in different regions (i.e. ML, rain, and snow regions), and that can help improve representation of ML microphysics in NWP models and polarimetric QPE in rain. In this study, the rain region is defined as 300 m below the ML bottom, which consists mainly of light-to-moderate rain. While the area above the ML top of 300 m is taken as snow region, where it is mostly filled with dry snow. In order to avoid the interference of supercooled water from the convective region on the separation of ML, rain and snow, this definition is only valid in the stratus clouds.
and give the histograms of polarimetric variables (i.e. ZH, ρHV, ZDR and Kdp) for the ML peak, rain regions and snow regions. Firstly, maximum ZH within ML roughly ranges from 24.5 to 47.5 dBZ (10th and 90th percentiles) with a mean value of 36.6 dBZ (), which is larger than that of rain and snow regions about 6 and 8 dBZ, respectively. Compared to rain and snow regions, the ZH distribution in ML more inclined to the high value with smaller samples in low value area. In , minimum ρHV in the ML varies between 0.81 and 0.91 with a mean value of 0.86. The peak value of ρHV distribution for rain and snow regions are both close to 1, while that in ML is significantly low to 0.88. Also, maximum ZDR in ML mainly varies between 1.04 and 3.06 dB with an average of 1.98 dB (). It is larger than that of rain and snow regions about 1.2 and 1.6 dB, respectively. But for KDP distribution, there is no significant difference between the ML peak, rain regions and snow regions, and they are all distributed from −0.6 to 1.6 deg km−1, with a peak value of 0.15 deg km−1.
Figure 10. Histograms of (a) ZH, (b) ρHV, (c) ZDR and (d) Kdp for the ML peak (blue line), rain (red line) and snow (green line).

Table 3. Statistics describing the distributions of the polarimetric variables within the ML, rain, and snow area.
Overall, these polarimetric statistics in the ML provide important information on microphysical properties within, above and below the ML, which can ultimately help improve the accuracy of polarimetric QPE and correction of radar attenuation caused by the ML. For instance, the quantitative analysis of the polarimetric variables between the ML, rain and snow regions can help to establish a realistic the vertical profile of reflectivity, which is a key factor in correcting the overestimation of QPE due to BB effects.
. Statistics describing the distributions of the polarimetric variables within the ML, rain, and snow area
Summary and conclusion
This study uses the quasi-vertical profiles (QVPs) obtained from S-band polarimetric radar data to document polarimetric characteristics of the melting layer (ML) in 7 summer storms during the pre-summer rainy season over South China (PRSC). Specifically, we have investigated the ML geometry, the distribution of polarimetric radar variables in ML and the relationship between ML, rain, and snow. The main conclusions of this study can be summarized herein.
(1) Firstly, the polarimetric QVPs are obtained by azimuthally averaging of the radar reflectivity factor for horizontal polarizations (ZH), differential reflectivity (ZDR), specific differential phase (KDP), and co-polar cross-correlation coefficient (ρHV) measured at antenna elevation 19.5°. Then the ML top and bottom are identified from the strong vertical gradients of ZH and ρHV in QVPs. The detected ML top height is compared to the ERA5-derived 0°C isotherm. The results show that the offset between them is about 158 m, which is comparable to that of S-band polarimetric WSR-88D radar reported by Giangrande et al. (Citation2008).
(2) The ML detection algorithm is used to characterize the ML during the PRSC in terms of geometric and polarimetric signatures.The depth of the ML changes from 250 to 920 m with a median value of about 493 m, which is thicker (about 150 m) than that of winter storms over North America (Giangrande et al., Citation2008) and summer storms in Europe (Wolfensberger et al., Citation2016). Several fascinating polarimetric signatures are observed. In the ML, the average of ZH ZDR and ρHV are 32 dBZ, 0.91 dB and 0.94, respectively. And the peak values of polarimetric variables in the ML can reach 36.6 dBZ, 1.98 dB and 0.88. The most striking feature is maximum ZDR occurs at the ML bottom, while minimum ρHV and maximum ZH appear at the middle of ML. Essentially, the melting of snowflakes in the ML causes its water content to increase continuously, which in turn leads to an increase of ZDR (Wang et al., Citation2016).
(3) Analyses indicate that maximum ZH in ML is larger than that in rain area (i.e. 0.3 km below ML) with value of 6 dBZ and snow area (i.e. 0.3 km above ML) with value of 8.6 dBZ. Also, this phenomenon occurs in ZDR distribution, whose difference values exceed 1.2 and 1.6 dB, respectively. And the minimum ρHV in ML smaller than that from rain and snow area. These ML statistics are particularly important for developing a technique (e.g. polarimetric vertical profiles of rain) to mitigate the impact of ML contamination on polarimetric QPE.
Overall, the results of this study provide a next step toward advancing understanding of ML microphysical processes during the PRSC. And the statistical analyses of polarimetric signatures in and near the ML have the potential to improve polarimetric QPE (Chen et al., Citation2020). But this work is only a preliminary study on the ML in South China, a comprehensive and in-depth understanding involve more cases in different seasons. And the application (e.g. the microphysical processes associated with ML) of polarimetric radar-based ML features also should be strengthened in the future studies. Considering the insufficiencies of ground-based observations, future studies would be launched by combining observations and high-resolution numerical simulations.
Data availability statement
The ERA5 is available online at https://cds.climate.copernicus.eu/cdsapp#!/home. These polarimetric radar data can be accessed upon request but whose usage may be restricted by pertinent Chinese government rules and regulations that are beyond the control of the authors (contact email: [email protected]).
Disclosure statement
No potential conflict of interest was reported by the authors.
Additional information
Funding
References
- Allabakash, S., Lim, S., & Jang, B.-J. (2019). Melting layer detection and characterization based on range height indicator–quasi vertical profiles. Remote Sens, 11(23), 2848. https://doi.org/10.3390/rs11202335
- Austin, P. M., & Bemis, A. C. (1950). A quantitative study of the “bright band” in radar precipitation echoes. J. Meteor, 7(2), 145–11. https://doi.org/10.1175/1520-0469(1950)007<0145:AQSOTB2.0.CO;2
- Baldini, L., & Gorgucci, E. (2006). Identification of the melting layer through dual-polarization radar measurements at vertical incidence. J. Atmos. Ocean. Technol, 23(6), 829–839. https://doi.org/10.1175/JTECH1884.1
- Brandes, E. A., & Ikeda, K. (2004). Freezing-level estimation with polarimetric radar. J. Appl. Meteor, 43(11), 1541–1553. https://doi.org/10.1175/jam2155.1
- Bringi, V. N., & Chandrasekar, V. (2001). Polarimetric Doppler weather radar: Principles and applications. Cambridge University Press.
- Broeke, M. S. V. D., Tobin, D. M., & Kumjian, M. R. (2016). Polarimetric radar observations of precipitation type and rate from the 2–3 March 2014 winter storm in Oklahoma and Arkansas. Wea. Forecasting, 31(4), 1179–1196. https://doi.org/10.1175/waf-d-16-0011.1
- Chen, C., Liu, L., Hu, S., Wu, Z., Wu, C., & Zhang, Y. (2020). Operational evaluation of the quantitative precipitation estimation by a CINRAD-SA dual polarization radar system. Journal of Tropical Meteorology, 26(2), 176–187. https://doi.org/10.46267/j.1006-8775.2020.016
- Fabry, F., & Zawadzki, I. (1995). Long-term radar observations of the melting layer of precipitation and their interpretation. J. Atmos. Sci, 52(7), 838–851. https://doi.org/10.1175/1520-0469(1995)0520838:ltroot>2.0.co;2
- Falconi, M. T., Montopoli, M., & Marzano, F. S. (2016). Bayesian statistical analysis of ground-clutter for the relative calibration of dual polarization weather radars. European Journal of Remote Sensing, 49(1), 933–953. https://doi.org/10.5721/EuJRS20164949
- Giangrande, S. E., Krause, J. M., & Ryzhkov, A. V. (2008). Automatic designation of the melting layer with a polarimetric prototype of the WSR-88D radar. J. Appl. Meteor. Climatol, 47(5), 1354–1364. https://doi.org/10.1175/2007JAMC1634.1
- Gourley, J., & Calvert, C. (2003). Automated detection of the bright band using WSR-88D data. Wea. Forecasting, 18, 589–599. https://doi.org/10.1175/1520-0434(2003)018<0585:ADOTBB2.0.CO;2
- Griffin, E. M., Schuur, T. J., & Ryzhkov, A. V. (2018). A polarimetric analysis of ice microphysical processes in snow, using quasi-vertical profiles. J. Appl. Meteor. Climatol, 57(1), 31–50. https://doi.org/10.1175/jamc-d-17-0033.1
- Griffin, E. M., Schuur, T. J., & Ryzhkov, A. V. (2020). A polarimetric radar analysis of ice microphysical processes in melting layers of winter storms using s-band quasi-vertical profiles. J. Appl. Meteor. Climatol, 59(4), 751–767. https://doi.org/10.1175/jamc-d-19-0128.1
- Hall, W., Rico-Ramirez, M. A., & Krämer, S. (2015). Classification and correction of the bright band using an operational C-band polarimetric radar. J. Hydrometeor, 531, 248–258. https://doi.org/10.1016/j.jhydrol.2015.06.011
- Hersbach, H., Bell, B., Berrisford, P., Biavati, G., Horányi, A., Muñoz Sabater, J., Nicolas, J., Peubey, C., Radu, R., Rozum, I., Schepers, D., Simmons, A., Soci, C., Dee, D., & Thépaut, J.-N. (2018). ERA5 hourly data on pressure levels from 1959 to present. Copernicus Climate Change Service (C3S) Climate Data Store (CDS).
- Huang, L., & Luo, Y. (2017). Evaluation of quantitative precipitation forecasts by TIGGE ensembles for south China during the presummer rainy season. J. Geophys. Res.: Atmos, 122(16), 8494–8516. https://doi.org/10.1002/2017jd026512
- Hu, X., Huang, H., Xiao, H., Cui, Y., Lv, F., Zhao, L., & Ji, X. (2022). Microphysical characteristics of precipitating stratiform clouds in north China revealed by joint observations of an aircraft and a polarimetric radar. Journal of the Atmospheric Sciences, 79(11), 2799–2811. https://doi.org/10.1175/JAS-D-21-0248.1
- Huuskonen, A., Saltikoff, E., & Holleman, I. (2014). The operational weather radar network in Europe. Bull. Amer. Meteor. Soc, 95(6), 897–907. https://doi.org/10.1175/bams-d-12-00216.1
- Kumjian, M. R. (2013). Principles and applications of dual-polarization weather radar. part I: description of the polarimetric radar variables. J. Oper. Meteor, 1(19), 226–242. http://dx.doi.org/10.15191/nwajom.2013.0119
- Kumjian, M. R., & Lombardo, K. A. (2017). Insights into the evolving microphysical and kinematic structure of northeastern U.S. winter storms from dual-polarization Doppler radar. Mon. Wea. Rev, 145(3), 1033–1061. https://doi.org/10.1175/mwr-d-15-0451.1
- Kumjian, M. R., Ryzhkov, A. V., Reeves, H. D., & Schuur, T. J. (2013). A dual-polarization radar signature of hydrometeor refreezing in winter storms. J. Appl. Meteor. Climatol, 52(11), 2549–2566. https://doi.org/10.1175/jamc-d-12-0311.1
- Lee, J. E., Jung, S. H., & Kwon, S. (2020). Characteristics of the bright band based on quasi-vertical profiles of polarimetric observations from an S-band weather radar network. Remote Sens, 12(24), 4061. https://doi.org/10.3390/rs12244061
- Luo, Y., Xia, R., & Chan, J. C. L. (2020). Characteristics, physical mechanisms, and prediction of pre-summer rainfall over South China: research progress during 2008-2019. J Meteor Soc Japan Ser II, 98(1), 19–42. https://doi.org/10.2151/jmsj.2020-002
- Matrosov, S. Y., Cifelli, R., White, A., & Coleman, T. (2017). Snow-level estimates using operational polarimetric weather radar measurements. J. Hydrometeor, 18(4), 1009–1018. https://doi.org/10.1175/JHM-D-16-0238.1
- Matrosov, S. Y., Clark, K. A., & Kingsmill, D. E. (2007). A polarimetric radar approach to identify rain, melting-layer, and snow regions for applying corrections to vertical profiles of reflectivity. J. Appl. Meteor. Climatol, 46(2), 154–166. https://doi.org/10.1175/jam2508.1
- Park, H., Ryzhkov, A. V., Zrnić, D. S., & Kim, K. E. (2009). The hydrometeor classification algorithm for the polarimetric WSR-88D: Description and application to an MCS. Wea. Forecasting, 24(3), 730–748. https://doi.org/10.1175/2008WAF2222205.1
- Qiao, J., Ai, W., Hu, X., Hu, S., & Yan, W. (2021). A recognition method of hydrometeor in tropical cyclones by using the GPM dual-frequency precipitation radar. Journal of Tropical Meteorology, 27(2), 161–168. https://doi.org/10.46267/j.1006-8775.2021.015
- Rico‐Ramirez, M. A., & Cluckie, I. D. (2007). Bright‐band detection from radar vertical reflectivity profiles. International Journal of Remote Sensing, 28(18), 4013–4025. https://doi.org/10.1080/01431160601047797
- Romatschke, U. (2021). Melting layer detection and observation with the NCAR airborne W-Band radar. Remote Sensing, 13(9), 1660. https://doi.org/10.3390/rs13091660
- Ryzhkov, A., Zhang, P., Reeves, H., Kumjian, M., Tschallener, T., Trömel, S., & Simmer, C. (2016). Quasi-vertical profiles—a new way to look at polarimetric radar data. J. Atmos. Ocean. Technol, 33(3), 551–562. https://doi.org/10.1175/JTECH-D-15-0020.1
- Ryzhkov, A. V., & Zrnic, D. S. (2019). Radar polarimetry for weather observations. Springer.
- Sánchez-Diezma, R., Zawadzki, I., & Sempere-Torres, D. (2000). Identification of the bright band through the analysis of volumetric radar data. J. Geophys. Res.: Atmos, 105(D2), 2225–2236. https://doi.org/10.1029/1999jd900310
- Sanchez-Rivas, D., & Rico-Ramirez, M. A. (2021). Detection of the melting level with polarimetric weather radar. Atmos. Meas. Tech, 14(4), 2873–2890. https://doi.org/10.5194/amt-14-2873-2021
- Schuur, T. J., Ryzhkov, A. V., Forsyth, D. E., Zhang, P., & Reeves, H. D. (2014). Precipitation observations with NSSL’s X-band polarimetric radar during the SNOW-V10 campaign. Pure and Applied Geophysics, 171(1), 95–112. https://doi.org/10.1007/s00024-012-0569-2
- Shusse, Y., Takahashi, N., Nakagawa, K., Satoh, S., & Iguchi, T. (2011). Polarimetric radar observation of the melting layer in a convective rainfall system during the rainy season over the East China Sea. J. Appl. Meteor. Climatol, 50(2), 354–367. https://doi.org/10.1175/2010jamc2469.1
- Tang, L., Zhang, J., Qi, Y., Langston, C., & Howard, K. W. (2013). Non-standard blockage mitigation for national radar QPE products. 36th conference on radar meteorology, Colorado. American Meteorological Society.
- Trömel, S., Ryzhkov, A. V., Hickman, B., Mühlbauer, K., & Simmer, C. (2019). Polarimetric radar variables in the layers of melting and dendritic growth at X band—implications for a nowcasting strategy in stratiform rain. J. Appl. Meteor. Climatol, 58(11), 2497–2522. https://doi.org/10.1175/jamc-d-19-0056.1
- Wang, H., Kong, F., Jung, Y., Wu, N., & Yin, J. (2018). Quality control of S-band polarimetric radar measurements for data assimilation. J. Appl. Meteorol. Sci, 29(5), 546–558. in Chinese with an English abstract. https://doi.org/10.11898/1001-7313.20180504
- Wang, H., Kong, F., Wu, N., Lan, H., & Yin, J. (2019). An investigation into microphysical structure of a squall line in South China observed with a polarimetric radar and a disdrometer. Atmospheric Research, 226, 171–180. https://doi.org/10.1016/j.atmosres.2019.04.009
- Wang, D., Liu, L., Zhong, L., Wei, Y., & Wang, X. (2012). Analysis of the characters of melting layer of cloud radar data and its identification. Meteor. Mon.(6), 74–83. http://dx.doi.org/10.7519/j.1000-0526.2012.6.009
- Wang, H., Wan, Q., Yin, J., & Ding, W. (2016). Application of the dual-polarization radar data in numerical modeling studies: Construction of the simulator. Acta Meteorologica Sinica, 74(2), 229–243. in Chinese with an English abstract. https://doi.org/10.11676/qxxb2016.017
- Willis, P. T., & Heymsfield, A. J. (1989). Structure of the melting layer in mesoscale convective system stratiform precipitation J. Atmos. Sci, 46(13), 2008–2025. https://doi.org/10.1175/1520-0469(1989)046<2008:SOTMLI>2.0.CO;2
- Wolfensberger, D., Scipion, D., & Berne, A. (2016). Detection and characterization of the melting layer based on polarimetric radar scans. Quart. J. Roy. Meteor. Soc, 142(S1), 108–124. https://doi.org/10.1002/qj.2672
- Wu, N., Ding, X., Wen, Z., Chen, G., Meng, Z., Lin, L., & Min, J. (2020). Contrasting frontal and warm-sector heavy rainfalls over South China during the early-summer rainy season. Atmos. Res, 235, 104693. https://doi.org/10.1016/j.atmosres.2019.104693
- Xiao, Y., Liu, L., Li, Z., & Wang, H. (2010). Automatic recognition and removal of the bright band using radar reflectivity data. Plateau Meteor, 29(1), 197–205. http://www.gyqx.ac.cn/CN/Y2010/V29/I1/197#2
- Yang, Z., Xie, Y., Xiang, Y., & Zhou, S. (2019). Analysis on dual polarization radar observations of a heavy snowstorm event in Anhui in the beginning of January 2018. Torrential Rain and Disasters, 38(1), 31–40. in Chinese with an English abstract. https://doi.org/10.3969/j.1004-9045.2019.01.004
- Yao, X., Wei, M., Du, A., & Lu, M. (2016). The detection sensitivity analysis of dual polarization radar parameters for melting layer. Science Technology and Engineering, 16(21), 150–156. http://www.stae.com.cn/jsygc/article/abstract/1601755?st=search