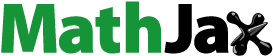
ABSTRACT
With population growth, water will increase in the following decades tremendously. The optimization of water allocation for agriculture requires accurate soil moisture (SM) monitoring. Recent Global Navigation Satellite System Reflectometry (GNSS-R) studies take advantage of continuously emitted navigation signals by the Global Navigation Satellite System (GNSS) constellations to retrieve spatiotemporal soil moisture changes for soil with high clay content. It presents the advantage of sensing a whole surface around a reference GNSS antenna. This article focuses on sandy SM monitoring in the driest condition observed in the study field of Dahra, (Senegal). The area consists of 95% sand and in situ volumetric soil moisture (VSM) range from ~3% to ~5% durinf the dry to the rainy season. Unfortunately, the GNSS signals’ waves penetrated deep into the soil during the dry period and strongly reduced the accuracy of GNSS reflectometry (GNSS-R) surface moisture measurements. However, we obtain VSM estimate at low/medium penetration depth. The correlation reaches 0.9 with VSM error lower than 0.16% for the 5–10-cm-depth probes and achieves excellent temporal monitoring to benefit from the antenna heights directly correlated to spatial resolution. The SM measurement models in our research are potentially valuable tools that contribute to the planning of sustainable agriculture, especially in countries often affected by drought.
1. Introduction
Water resource management is considered one of the critical problems in the world, especially in the context of the growth of the population, the development of the industry, the need for increased food production and the pollution of the water resource due to various human activities. In addition, it is also necessary to ensure sustainable agricultural development (Zhang et al., Citation2017) and flood control (Rodriguez-Iturbe & Porporato, Citation2004).
A vital parameter of the water resource is soil moisture (SM). Indeed, its impact on the soil-vegetation-atmospheric exchange is exceptionally high in three regions: the Great Plains of North America (Xu et al., Citation2018), India and the Sahel, where rainfall is strongly influenced by SM (Koster et al., Citation2004). In many countries like Mali, or Senegal, water resources basically come from groundwater reservoirs that are declining and not renewable. There are no clear conclusions in previous studies whether or not this groundwater depends on SM variations due to the limitation of SM data and the complexity of hydrological phenomena (Fatras et al., Citation2012; Lebel et al., Citation2009; Lopez et al., Citation2016; Mougin et al., Citation2009). Knowledge of these phenomena is fundamental for a proper understanding (Rascon et al., Citation2021; Steelman et al., Citation2012) of water resources management, in ecology, in the development of agricultural strategies, as well as in case of extreme events (He et al., Citation2012; Martínez-Fernández et al., Citation2021; Sun et al., Citation2015).
As a result of its importance, various methods have been developed to measure SM indices, with three main approaches. The most direct one consists of using gravity surveys to carry out measurements in the field (Gehman et al., Citation2009), and it enables calibrating the measurement probes. These probes are today the most widely used field measurement techniques, even with the information being scarce and very local. Modeling enables the provision of large-scale SM values but remains dependent on the availability of other variables such as meteorological data (Kumar et al., Citation2008; Mitchell et al., Citation2004), the selection of model parameters and the model itself (e.g. WGHM (Döll et al., Citation2003), Global Land Data Assimilation System models (Rodell et al., Citation2004)).
Remote sensing in the microwave domain is based on the gap presets and is advantageous in that it can work any time of day or night, regardless of the weather conditions at the time of acquisition. It records information directly related to SM using the soil dielectric properties and electromagnetic (EM) radiation in the microwave region 0.5–100 cm (Karthikeyan et al., Citation2017; Sun & Cui, Citation2021; Wang & Qu, Citation2009). Many research and experiments have used the SM inversion method from an active microwave, which assumes that heat exchange in the ground happens through thermal conduction (Han et al., Citation2018; Kerr, Citation2007; Xu et al., Citation2020). However, these spectral windows are also susceptible to cloud cover, limiting their use due especially to their low spatial and temporal coverage.
Meanwhile, passive microwaves use a sensor which emits an EM wave in a known frequency and polarization in the direction of a target, such as its surface (Xu et al., Citation2020). Passive microwaves are preferred for estimating SM (Chew & Small, Citation2018; Entekhabi et al., Citation2010; Peng & Loew, Citation2017). Passive microwave sensors including Scanning Multichannel Microwave Radiometer, Special Sensor Microwave/Imager sensors, Advanced Microwave Scanning Radiometer Earth Observing System and Soil Moisture Ocean Salinity Satellite/Soil Moisture Active Passive mission have been used successfully to measure SM (Ahmad et al., Citation2011; Jones et al., Citation2010; Kerr et al., Citation2001; Njoku & Chan, Citation2006; Paloscia et al., Citation2001; Wen et al., Citation2005; Wigneron et al., Citation2021). However, spatial and temporal resolution is significantly lower than the infrared range and is thus considered a significant limiting factor for SM measurements.
In addition, recent used EM waves come from Global Navigation Satellite System(GNSS) (GPS, GLONASS, Galileo, etc.), which extract the various geographic parameters of the earth surface with high spatiotemporal resolution (Darrozes et al., Citation2016). This opportunistic remote sensing technique is called GNSS reflectometry (GNSS-R), based on the evaluation of GNSS waves obtained by an antenna after reflection on the earth surface (Aranyossy & Ndiaye, Citation1993; Alonso-Arroyo et al., Citation2014; Darrozes et al., Citation2016; Roussel et al., Citation2014).
Most GNSS signals are received directly by a geodetic antenna’s upper hemisphere, as Right-Hand Circular Polarization (RHCP) waves. Meanwhile, a portion comes from below the horizon of the antenna after reflections from surrounding surfaces. Fortunately, the lower antenna gain pattern is mainly Left-Hand Circular Polarization (LHCP) and records LHCP signal corresponding to the reflected signal. EM interferences between direct (RHCP from upper part of the antenna) and reflected waves (LHCP from lower part) are especially obvious in the total signal-to-noise ratio (SNR) recorded by the receiver.
This SNR technique is applied in various studies of Earth Sciences, particularly SM analysis. The temporal resolution can be several minutes, hours or days, depending on the calculating algorithm applied to assess the SM variation (Chew et al., Citation2014; Larson et al., Citation2008).
The reflected GNSS signal changes are due to the surface variations (soil composition, moisture, roughness) and their characteristics. Therefore, the SNR is then evaluated to predict SM content. This SNR method is applied using any conventional GNSS receiver/antenna without any hardware modifications. The GNSS R technique has SM retrieval accurately at 2–5 cm depth over clayed soils (Rodriguez-Alvarez et al., Citation2011; Roussel, Citation2015). Nevertheless, if the SM content is less than 10% volumetric soil moisture (VSM), it causes cyclic wrapping of the phase.
The present study aims to develop a comprehensive approach for desert or semiarid climates where the SM levels are weak. According to the theory of radar waves, these weak SM levels induce a significant wave penetration (Ulaby & Moore, Citation1986). This penetration depends on the soil composition. For clay soils or rich clay soil content, even in case of extreme dryness, the waves are reflected in the first centimeters, and the SM retrieval on the surface is well correlated to the in situ SM probe at 5 cm depth (Vey et al., Citation2016; Zhang et al., Citation2018). For sandy soils, the penetration is more critical and exceeds the meter in conditions of intense drought. Our approach estimating SM is based on state-of-the-art GNSS-R for SM retrieval. It is combined with the phase unwrapping, generally used in SAR imagery (Benoit et al., Citation2020; Lu & Zebker, Citation1998) for very weak values of SM, which induces the intense penetration for the sandy soil of the study area. The method using SNR data incorporates both direct and reflected signals, continuously collected by the geodetic antenna. The variation in soil moisture is not the only parameter that will affect the reflected signal of radar waves; two other aspects will interfere with bare soil: (i) the surface roughness of the soil and (ii) variations in soil composition. However, of these three parameters, only variations of the surface soil moisture (SSM) has a cyclical response related to seasonal changes of this Sahelian area. SSM changes the EM properties of the reflected waves, i.e. the complex dielectric constant that depends on electrical conductivity and relative permittivity of the media. Two parameters, namely the phase and the frequency of the multipath contribution to SNR, are analyzed to connected with the antenna height above the reflecting surface (Lopez et al., Citation2016). Results obtained from the total of the different GNSS satellite constellations will be evaluated applying independent SM records measured in the field with Theta probe sensors (Roussel, Citation2015).
2. Methodology
2.1.1 Interference Pattern Technique
Interference Pattern Technique (IPT) is a method based on analyzing the multipath signature present in the GNSS SNR data recorded continuously by a GNSS receiver and its associated single geodetic antenna (). Multipath propagation affects the SNR recorded by a GNSS receiver by generating frequency oscillations with lower amplitudes than the direct signals. These frequency oscillations depend on the satellite elevation (SE) angle and vary from the intermediate frequency at Low Satellite Elevation (LSE) to the high frequency at High Satellite Elevation (HSE).
Figure 1. (a) Transmission of EM waves to the single geodetic antenna: direct GNSS signal path with RHCP and the reflected path with LHCP; (b) SNR in dB-Hz of GNSS signals and SNR multipath (SNRm) signals removed the direct contribution of signal (parabolic red line) from the initial SNR profile (Modified from CitationRoussel et al., Citation2016 and Darrozes et al., Citation2016).

Because the antenna gain pattern prefers the direct signal over the reflected signal by construction, and during reflection will also reduce the signal energy; the primary influence of the structure of the SNR time series is the direct one (). The SNR total show a low frequency which corresponds to the main variation of the energy of the direct signal as a function of the SE angle. The multipath (, lower graph) under medium frequency oscillation is visible for low elevations while for higher elevations of the satellite these multipaths do not emerge or poorly emerge of noise level.
For multipath component analysis, the direct signal contribution must be eliminated by fitting and subtracting a simple parabolic polynomial from the raw time series of SNR to obtain the residual of SNR multipath (SNRm) component (Larson et al., Citation2008; , red curve).
The influence of multipath interference appears in SNR measurements at different frequencies of the L-bands, such as L1, L2 and L5. According to Larson et al. (Citation2010), for a static or quasi-static surface (vertical velocity of the surface is lower than 10–6 m.s−1). the oscillations frequency is approximated as a sinusoidal function of the SE:
where is the effective antenna height measured by the antenna height Ho () and λ is the signal wavelength. The multipath oscillation frequency
and the effective antenna height
are directly proportional, as shown in equation above, and can be calculated for each satellite at each epoch and for each specular point of the time series where the moisture measurement occurs.
When the GNSS wave penetrates the reflecting surface, varies depending on the characteristics of the non-moving or weakly moving surface (e.g. vegetation density, SM, soil type, ).
Figure 2. (a) Theoretical penetration depth of the GNSS waves when the ground consists of 100% clay (red), 95% sand (blue, our study) and 100% sand (green); (b) theoretical penetration depth of the various GNSS wavelengths and for various SE for a sandy soil; (c) representation of the unwrapping phase process (Zebker & Yanping, Citation1998). Red arrow shows a positive phase slip while green arrow shows a negative one.

Frequency is estimated, based on harmonic Fourier decomposition of the Lomb–Scargle periodogram (Lomb, Citation1976; Scargle, Citation1982), using a moving window from the SNRm time series. We also add, before Lomb–Scargle analysis, a step of phase unwrapping (, see Section 2.2). The retained periodogram peaks must reach a significance level equal to 1% (Li et al., Citation2018) and defining a local maximum between
and
using user-defined hmin,hmax,; in our case, the penetration of the wave leads to hmax = Ho + Pd, where Pd is the penetration depth of the wave (see next section).
According to the results of Larson et al. (Citation2010), and a given quite constant , SNRm can be expressed as follows:
where is the amplitude of the multipath oscillations and
is the phase of the multipath oscillations.
denotes the backscatter intensity of ground reflections, including both the gain pattern and multipath power. According to field studies,
and
are strongly correlated with the level of SM (Larson et al., Citation2010), and SM is more sensitive to
than
(Chew et al., Citation2015; Roussel et al., Citation2016). Consequently, the three metrics of
,
(above) and
(derived from
, see EquationEquation (1)
(1)
(1) ) can be used to estimate SM variations.
2.2 Phase unwrapping method
2.2.1 Unwrapping SNR phase method
The GNSS waves penetration depth depends on the soil dielectric properties (Njoku & Entekhabi, Citation1996), the SM content and the SE (). The zenith signal’s penetration depth , according to Njoku and Entekhabi (Citation1996) and Behari (Citation2006), can be expressed as follows:
where and
are the real and imaginary parts of the reflecting surface relative permittivity, respectively.
Recent studies applying IPT on clay soils give excellent results with high accuracy SM estimation at 2–7 cm depth because the penetration depth of the wave is less than the wavelengths of the GNSS signals (). However, if we are in the conditions of sandy soils and VSM has low values, i.e. in the driest season, the wave penetration can exceed the wavelength and causes the cyclic phase wrapping (). Theoretical, the penetration depth of GNSS signals shows a significant increase when the soil is 100% clay (red) or 100% sand (green), especially for sandy soil when VSM is very low (<10%) (). This penetration also depends on the SE and the wavelength of the GNSS signal (). When SE is low, the penetration of the wave will be lower, while for high SE, the energy of the EM signal being more important, the depth of penetration will be greater. Still, from a theoretical point of view, we see that when the wavelength of the signal increases, its penetration depth also increases (). Thus, when the penetration depth is greater than the wavelength
used, the phase undergoes one or more cycle slips. As a result, the three metrics estimated from SNRm does not correlate with the SM. Therefore, in the sandy field, we propose using an additional phase unwrapping algorithm to solve this problem by reconstituting the phase cycle slip that appears in the estimated
, thus obtaining continuous phase variations ().
shows the example of a discrete noisy phase signal whose amplitude exceeds the range [-π, π] by processing phase wrapping operation
with the original continuous noisy phase signal. The wrapped phase
is calculated using the four-quadrant arctangent (atan2) function of
and
. We must remove the 2π slips that are present in the wrapped phase signal () to eliminate the phase cycle slip and restore the continuous form, allowing the phase variation
usable in analysis or further processing (). This process is called phase unwrapping and can be represented as follows:
where is the unwrapped phase signal,
is the operation of phase unwrapping and
is an integer relating to the cycle number of phase slips. To apply the phase unwrapping procedure for the estimated phase
from the SNRm time series, we must calculate the difference
between two adjacent successive samples on its left. Once a phase wrap is detected,
is less than –π or greater than +π, 2π is either subtracted (negative phase slip, k < 0) or added (positive phase slip, k > 0), from this sample to the right-hand side. In addition, this phase unwrapping method is ideally suited to noisy data, such as GNSS signals (Gdeisat, Citation2018) or InSAR images (Hooper & Zebker, Citation2007; Smith, Citation2002; Zebker & Yanping, Citation1998).
2.2.2 New metrics
Following EquationEquation (2)(2)
(2) , we can obtain the three metrics
, Am and Φm from SNRm for each GNSS constellation (see IPT). Considering the Am depending on the antenna gain pattern and multipath intensity, it is constant. However, the phase unwrapping algorithm processing changes the phase
to obtain a variation of continuous phase
, the effective antenna height
(proportional to the frequency
, EquationEquation (1)
(1)
(1) ) must be re-calculated. The new height effective
after using the unwrapping phase is determined as follows:
2.3 Estimating SM from SNR signals using IPT and unwrapping phase method
Following the GNSS-R theory presented in the previous parts, a complex processing chain integrating IPT and phase unwrapping methods has been developed to determine SSM in sandy environments (). In this processing chain, we employ a new time series of , calibrated with in situ VSM to remove negative correlation of
, to better retrieve variations of SM and to establish the SM maps.
Figure 3. The flow chart represents the complex processing chain for SSM detection/observation in sandy environments using an integration of IPT and phase unwrapping methods: the input, the various processing steps and the output.

As defined in EquationEquation (3)(3)
(3) , the new variable
is computed using the effective height
derived from the unwrapping phase process:
where is the antenna height from the phase center to the ground without any penetration and
corresponds to the total error term due to the measurement error for each GNSS wavelength and errors associated with the phase unwrapping and the surface roughness, which will further increase the measurement noise.
A Savitzky–Golay (Sgoley) filtering method (Baba et al., Citation2014; Schafer, Citation2011) was applied for to minimize errors caused by interference values (noisy signal whose frequency span is large) to obtain the filtered effective height
(EquationEquation (7))
(7)
(7) . This improvement in the height reduces the noise of the time series and increases the accuracy of SM estimations. The principle of this filter is to replace each
data point with some combination of the values contained in the moving window centred at that point. Sgoley filtering process can be described as follows:
where is the result from the Sgoley filter,
is the input data and
is the moving window length.
New time series ,
and
were normalized to minimum and maximum of in situ SM variables recorded by a classical SM sensor (Theta probe). Pearson correlation coefficient R is quantified to determine the correlation between these normalized time series with VSM measured by the Theta probe. We can also describe the differences between them in terms of the totalized root mean squared error (
) as follows:
where is an error caused by the difference in position between the reflected point used to retrieve the SM and the Theta probe used to measure the reference moisture;
is the error caused by the difference in spatial observation of the two moisture measurement methods (ML3 Theta probe is
0.03 m2, GNSS-R IPT is from 0.8 to 137 m2 for an antenna height Ho = 1.61 m with SE of 2–70°); and
is the error caused by the GNSS instrument affected by SE, the roughness of the ground and the GNSS wave penetration depth.
Although the statistics parameters described above are helpful in research for linear problems, they cannot reflect all of the correlations seen in wave field studies. As a result, we propose an additional method for analyzing waveform data to consider the correlations known as Wavelet Transform Coherence (WTC) (Grinsted et al., Citation2004). The WTC is a method to analyze the phase lag and coherence between two-time series S (time series of /
/
and in situ measurements) as a function of time and frequency.
To compute the WTC analysis, we first have to transform our time series into a wavelet coefficient map. The wavelet coefficient power map corresponds to the convolution in the frequency domain of the times series
and a set of wavelet functions
(EquationEquation (9)
(9)
(9) ). This set is derived from a mother function well known as Morlet wavelet function, a Gaussian-windowed complex sinusoid. For each wavelet function, a coefficient is computed for the time series’ time index n, and a defined period a. This period a varying from the minimal period, i.e. 1/5 of day, to the maximal period ~1 month.
WTC analysis performs detection of phase difference (= time delay), phase angle (cross magnitude), non-stationary and also wavelet coherence between the two-time series (EquationEquation (10)
(10)
(10) ). The phase difference between the two-time series is indicated by the arrows’ directions, and we can gain confidence in causal and geophysical test relationships using phase angle statistics. The two-time series are in-phase if the arrow is horizontal and pointing to the right, in-phase opposition if the arrow is horizontal but pointing to the left, and phase quadrature if the arrow is pointing up or down. According to Torrence and Webster (Citation1999) and Grinsted et al. (Citation2004), wavelet coherence
can be calculated using the following formula:
3 Experimental setup
3.1 Measurement site
Senegal has a strong diversity in climate, and it crosses three different climate zones: in the north the desert, and then crosses the semiarid zone, which leaves the place, in its southward part, to tropical climate (). Senegal is crossed by the West African river, which gave name to the country. Senegal River is 1800 km long. However, the catchment area is six times smaller than the two principal African basins, i.e. Congo and Nile; its area covers ~0.5 million km2, with an average discharge of ~630 m3. s−1.
Figure 4. Map of African Climate using Köppen climate classification, the studied watershed is delimited by a dark red line and associated hydrographic network corresponds to dark blue line for main rivers until white line for small tributaries or gully (Modified from Peel et al., Citation2007; Ramillien et al., Citation2021). The white rectangle corresponds to the study area presented in .

The study area is located near Dahra, Senegal, where the climate is semiarid. The main rainfall occurs during the rainy season, extending from mid-June to mid-October () with relative humidity ranging from 60% to 75%. It is followed by a dry season that extends from late October to late May, with relative humidity ranging from 20% to ~0%. This season is marked by a rise in temperatures, reaching 33°C, and a sharp drop in rainfall. It is also a slightly windier period with winds up to 3.5 m. s−1. A detailed description of the field site can be found in Tagesson et al. (Citation2015). Agriculture is one of Senegal’s strengths, but while the south (tropical climate) has very favorable conditions, this is less true in the semiarid Sahelian zone. We then understand the importance of mapping SM to water the fields while saving this rare water commodity.
Figure 5. Dahra region charts of (a) monthly precipitation (mm), (b) relative humidity (%), (c) air temperature at 2 m (°C) and (d) wind speed at 2 m (m . s−1) from 2015 to 2017. Black vertical dashes correspond to January for each year (Modified from Laouali et al., Citation2021).

To make this mapping, we have installed a Leica GR25 receiver with an AR10 antenna in a sparsely wooded grassland in Dahra experiment (). In terms of geology and soil, we have a homogeneous area of rubified sand from a continental dune (Faye et al., Citation2017) of further square kilometers. Therefore, this field is considered to have no significant topography changes (), and in the modeling of the specular point, we assume that it is a flat area, and we do not use digital elevation model corrections. GPS and GLONASS signals were continuously acquired at a 1 Hz sampling frequency from October to December 2016.
Figure 6. (a) The experiment site of Dahra (Senegal), (b) location of the AR10 antenna (15°24ʹ5.36” N; 15°26ʹ0.13” W); (c) photography of the AR10 antenna with a Leica GR25 receiver installed on sandy soil, this flat area is covered by grassland and sparce trees. The ring of thorny trees surrounding the antenna is clearly visible and serves to protect the equipment from animals.

3.2 Data used for validation
The SSM is monitored by an SM station located about 500 m away from the GNSS receiver (). Some authors (e.g. CitationBaup et al., Citation2007; CitationRosnay et al., Citation2009) have shown that in semiarid Sahelian areas, with equivalent soil and vegetation, in situ SM measurements are representative of much larger area. This is why, we consider in this case of very homogeneous geological/soil (Faye et al., Citation2017), the moisture station represents a large area, including the GNSS-R station and can be used for calibration. Soil content of the Dahra experiment is ~95% of sand associated with few percent of organic matter in surface and few percent of clay. In order to avoid surface disturbances (presence of this small concentration of organic matter), we took soil samples at 10 cm depth. These samples, as shown in , are representative of the soil column down to at least 1 m depth (no visible soil change).
Figure 7. (a) Configurations of the SM station (15°43ʹ42.89” N, 15°00ʹ55.69” W). The station equipped with six Theta probes (green): (number 1, 3): 5 cm, (2, 4): 10 cm, (6): 100 cm; (b) VSM recorded by Theta probes during the study period in 2016 which corresponds to the transition between rain season and dry season. The gray rectangle corresponds to a non-acquisition period, light blue corresponds to the precipitation (mm of water) during the study period.

For the GNSS frequencies which are in L-band, we calculated the theoretical curves for a soil consisting of 100% sand and another consisting of 100% clay (). This figure shows that the depth of penetration becomes important as soon as the SM is weak and below 10% the penetration exceeds 7 cm to reach 40 cm for SM~1%. The response of our soil (blue curve) is close to the theoretical green curve (100% sand).
The SM station is equipped with two soil temperature sensors and 5 ML3 Theta probe sensors with a sampling frequency of 15 min (accuracy of ± 0.1%), installed at 5 cm (1, 3), 10 cm (2, 4) and 1 m depth (5 in ). shows the variation of SM measured by the Theta probes. During the transition phase from the rainy season to the dry period, the VSM at 5 cm depth (P5cm) varied from 2.23% to 4.39%, the VSM at 10 cm depth (P10cm) varied from 2.87% to 4.76% and the VSM at 1 m depth (P1m) varied from 5.03% to 5.63%. At P5cm and P10cm depth, VSM shows a daily sinusoidal pattern.
4 Results
4.1 Importance of the unwrapping in sandy soil
For sandy soils of Dahra, we have a very noisy phase dataset () without any correlation with the in situ probes. So we applied the unwrapping technique to this phase dataset and we make an inspection of the unwrapped/wrapped signals to identify possible fake slips due to the noise. To calculate the number of fake slips, we compute the difference between two adjacent samples of the wrapped signal. If the computed difference is upper than twice the noise variance threshold criteria (TC), we assume we have a fake slip. In that case, the signal after the fake slip is affected by “miss wrap” due to the error propagation.
Figure 8. Impact of the phase unwrapping for sandy soils (a) before the unwrapping and (b) after the unwrapping. The correlation factor reaches 0.9.

This part of the time series is affected by a phase offset that can be positive or negative depending on the noise difference sign. We need to apply the unwrapping process to correct these harmful effects until all the computed differences are lower than TC. The resulting phase correlates with the in situ VSM measurements (P5cm, (1) ) with a calibration function in this type of soil of VSM~1 x unwrapped phase where the bias is close to 0 () which shows that we do not need to calibrate the phase to obtain VSM because of VSM ~. The rest of the study will use the
dataset to compute the antenna height parameters (see 4.2).
4.2 Comparisons between 
, 
and 
parameters for estimating SM in Dahra experiment
lists the results of root mean square error (RMSE) and correlation coefficient of Pearson (R) between ,
, and
(
after Sgoley filtering) with in situ VSM measured by the Theta probes at P5cm and P10cm for different constellations from October to December 2016 in the study area. We do not use P1m due to the weak correlation with subsurface SNRm measurement. This observation shows that the wave penetration depth is less than 1 m, which agrees with the theoretical model for VSM upper than 2.5% (). In the Darha experiment, where the soil consists of over 95% sand, retrieving SM from GNSS-R data became more complicated. The Am values can be disturbed by vegetation on the surface around the antenna. Thus, we used
and
to retrieve variations of the VSM. The three parameters show a good correlation with the in situ measurements of SM at 5 and 10 cm. Indeed, R is always higher than 0.73 in the rainy season and higher than 0.51 during the dry season. However, during the rainy season,
has a lower correlation than
and results quite similar to those of
regardless of the probe depth, the SE or even the constellation used. Conversely,
has the slightest deviation from the in situ measurements during the dry period, with an RMSE varying between 0.18% and 0.08%. It also has the best correlation, with R ranging between 0.92 and 0.95 ().
Table 1. Comparison between (,
, and
) and VSM measured by P5cm/P10cm (RMSETotal/R) for difference constellations, and for SNR from LSE (2–30°) and HSE (30–70°) from October to December 2016 in Dahra site.
Finally, the parameter that gives the best results over the two seasons is clearly with the best correlation, with the in situ measurements at 10 cm depth, which is always higher than 0.91 and an RMSE lower than 0.16%. This parameter is less influenced by the vegetation density and soil roughness. It is also less sensitive to the constellation used: GPS and GLONASS alone and GPS+GLONASS have very closed results. Nevertheless, the number of specular points increases when both constellations are used simultaneously, resulting in more accurate moisture maps (more points with a better spatial distribution). Furthermore, the elevation of GNSS satellites has little influence on this parameter, and the results are very favorable for low and high angles of incidence (). On the other hand, the parameter
, even if it presents globally good results, generally shows the weakest correlation of the three parameters studied here and the most significant differences with the in situ measurements of SM. In bold: results with a correlation greater than or equal to 0.9.
4.3 Wavelet analysis of the SNR time series
For WTC analysis, it is necessary to construct wavelet coefficient maps of two-time series, i.e. and
derived from the in situ time series of the SM probe and the SM retrieved from
. We used only the probe at 5 and 10 cm depth. The sensor probe at 1 m is too deep, and GNSS waves cannot reach this depth due to the moisture conditions (>2.4%) ().
shows the time series of VSM P10cm and VSM derived from the and
() and the wavelet coefficient map of the three time series of VSM P10cm (),
() and
(), respectively. These time series have quite similar coefficient maps with the main period of more than 8 days. The results show a normalized coefficient ranging from 0.6 to 0.8, with a period of 1 day for the in situ time series and a period of 1–2 days for the GNSS-R time series derived from the
and
. Two GNSS-R time series have a power noise close to 0.2/0.3 at the period varying from half a day to a quarter of the day. shows a good correlation between VSM P10cm with
in the rainy season.
Figure 9. Time series and magnitude maps calculated from Torrence and Compo (Citation1998). (a) In situ P10cm and ,
time series and associated wavelet coefficient maps; (b)
; (c)
; (d)
.

4.4 Wavelet Coherency Analysis between VSM estimated from 
and 
with VSM measured by in situ probe
The correlation between VSM estimated from and
with VSM measured by probes at P5cm () and P10cm () exhibited significant changes by period. Figures show that the VSM derived from
/
and in situ VSM measured has no significant correlation at the period of less than a half-day. During the dry season, a negligible correlation was found in the period of fewer than 4 days. This decorrelation is mainly due to the wave penetration. In this period GNSS wave reaches more than 10 cm and does not correspond to the surface condition.
Figure 10. The wavelet coherence (M2) of WTC analysis between VSM derived from GNSS-R parameters and in situ VSM at P5cm (a) , (b)
.

Figure 11. The wavelet coherence (M2) of WTC analysis between VSM derived from GNSS-R parameters and in situ VSM at P10cm. (a) , (b)
.

Nevertheless, the results in show a phase-shift (arrow field oriented toward the left) and ranging from π to π/2 between and SM measured by the probe at more or less than 1-day period. While the VSM estimated from
has a weak phase shift ranging from 0 to π/4 (arrow oriented ~horizontally toward the right) with VSM in situ. Low frequencies show high homogeneity with a high value of M2 and no phase delay (horizontal vector field) between in situ and GNSS-R data for periods ranging from 8 days to a month.
Inflexion of the trend related to the transition between the rainy and the dry seasons is observed during the period ranging from 29 October 2016 to 11 November 2016 over 2-/4-day period with the probe at 10 cm () and to a small extent with the one at 5 cm over a very temporally localized to 2-day period and only for the parameter (). shows a significant phase shift ranging from π/2 and π/4 between in situ data and GNSS-R ones during this transition period. It means that GNSS-R detects SM variations a little bit before the probe at 10 cm records SM. shows a better correlation between
and the in situ P10cm data during the transition and dry periods. In general, the
parameter presents a better correlation than
. Globally,
parameter is more homogeneous in terms of phase shift and M2 over all periods and for both in situ P5cm and P10cm measurements.
4.5 SM mapping based on GNSS-R data
As final results, shows SM time series obtained combining GLONASS and GPS and using of parameter. These time series range between October and December 2016, i.e. from rainy to dry season. However, the VSM at P5cm and P10cm depth only changed 2% during the entire study period. At the end of the rainy season of 2016, we see that the rains are already almost non-existent. shows that less than 3cm of water fell during October to decrease to less than 1 mm in November and disappear entirely in early December. The SM is following the same trend with an apparent decrease from ~4.5% of volume moisture to almost 3% for P10cm and even 2.5% for P5cm. The transition period is marked by quasi-constant SM of ~3.7%.
Figure 12. Evolution of the final results for all the time series – during period from October to December 2016. Results are obtained using Unwrapping phase algorithm for GPS+GLO satellites (dark blue dot) and compared to the in situ VSM measured by P10cm (a) and P5cm (b);
in m and the curve in red is a fit of
using a cubic spline. Rectangle blue correspond to the precipitation during this period.

The time series of shows a very clear anti-correlation with SM. Indeed, when the SM decreases, the GNSS waves penetrate more strongly into these sandy soils, resulting in an increase of
. We also notice that
has a minimum height that can be lower than the measured height of the ground, which is 1.61 m. This result is due to different factors. The first one is the multi-centimetric measurement noise which explains this phenomenon partly. Another one is the existence of some periodogram of a double peak indicating two possible surfaces of reflection. Moreover, a significant decrease in satellites visibility causes an increase in measurement error, as demonstrated by Zeiger et al. (Citation2021).
shows SM maps created from the results of in the study area. The specular points used to retrieve the SM maps have been computed thanks to the simulator developed by Roussel et al. (Citation2014) which allows to do the accurate ray tracing for each satellite according to the following parameters: orientation and elevation of the satellite, tropospheric refractive indices, local topography around the antenna, antenna height and the altimetric reference surface chosen – here the ellipsoid of the WGS84 datum. The time step for calculating spatial variability is set to 3 days. SM content changed from 2.87% to 4.76% during the rainy, transition and dry periods from October to December 2016. The temporal variation of the SM maps shows that the SM content gradually decreases as it transitions to the dry period. This soil drying increases toward the southeast with a spatial variability lower than 0.01%. The results demonstrate that the unwrapping phase technique improves the accuracy of SM retrievals compared to phase measurements.
5 Discussion
In the study area, the GNSS-R signal used to retrieve soil geophysical parameters of the soil represents a more complex observation of soil surface moisture. In areas with very low SM, still has a good correlation but is negatively correlated due to wave penetration. The effective height time series shows the best correlation with SM after it is corrected by the unwrapping SNR method and filtered by the Sgoley method to reduce the influence of vegetation and surface roughness. EquationEquation (5)
(5)
(5) shows that the
has an anti-correlation with
, and it could be explained as the increases of SM in any soil leads to the penetrations depth of GNSS signals decreases. Hence, as the effective height
also decreases as demonstrated in previous studies using
to estimate SSM and positively correlates with the in situ measurements (Roussel et al., Citation2016).
Another advantage of this method is the ability to provide spatial and temporal variability of the SM (). The illuminated spatial area depends on a critical parameter: the antenna height. In this case, 1.61 m antenna height corresponds to a radius of the reflection area of about 12 m, an average area of 452 m2 and a mean volume of ~100 m3. The comparison between GPS and GLONASS constellations shows that GPS satellites have a better correlation with in situ probes than GLONASS satellites. Here we show the effect of the signal noise of GLONASS constellations (lower SNR) which is more important than that of the GPS constellation. We choose to use both constellations to increase the number of specular points, and consequently, it improves over time to obtain long time series with a proper time step. It is possible to obtain two decades of data with time steps close to 30 s or less using the international GNSS network, which is essential to look at the effects of climate change, for example, and to highlight local and rapid phenomena like agricultural irrigation.
Another important aspect is that the antenna height adjustment can calibrate many space missions, e.g. a 2 m antenna height, with a coverage radius of few ten meters, could be useful to calibrate the Sentinel I, RADARSAT-2, ALOS-2 missions. On the other hand, a 60-m antenna with a coverage radius of few kilometers could be used to calibrate CYGNSS or SMAP data worldwide using GNSS stations of national (RGP for France; NGS for USA, CMONOC GNSS network for China, etc.) or international networks (IGS, EUREF, etc.). These stations are selected to identify those that cover either bare ground, agricultural fields or even forests. From the investigated surfaces, the results pointed out that the total of the first Fresnel surface depends on three main factors: the height of the antenna; the height of satellite ε, which can receive from broadcast ephemeris or precise one; and the many satellites visible at time t. So the different scales can be determined by dividing the visible satellites into low elevations and high elevations. Then, surfaces of a few square meters can be evaluated using the antenna approximately 5 m with an ε range of 35–70°. While surfaces from 100 to 10,000 m2 can be analyzed using the 100-m antenna with ε < 35 °. However, this method also has disadvantages due to the maximum height of the antenna, which must be less than the wavelength of the C/A code (293 m); otherwise, there is the problem of the construction of the interference pattern (Ribot et al., Citation2016).
GNSS-R with the single antenna is considered an excellent alternative solution to complement the in situ technique in order to monitor SM for various types of soil: bare soil (Roussel et al., Citation2016), bare and vegetated soil (Chew et al., Citation2016), cobbly clay loam (Larson et al., Citation2010), etc., and even areas where the VSM is too low or rapidly changes. The in situ technique requires calibrated reference data by applying the reference technique in the laboratory condition (Walker et al., Citation2004). Various sensors depend on daily temperature variation like the Theta probe (Holzman et al., Citation2017). Other sensors like time domain reflectometry are less sensitive to thermal artifacts (Walker et al., Citation2004), while GNSS-R is more sensitive to thermal artifacts. Besides, GNSS-R integrates a larger volume and deeper penetration than the Theta probe method, and its signal is more correlated to SM at ground level as humidity increases. Roxy et al. (Citation2010) indicate that daily moisture variations correspond to daily variations of surface albedo. These variations decrease from the dew point until the maximum sun illumination and reversely.
One of the challenges in improving the spatiotemporal resolution is that they are inversely proportional to each other. Various researches have justified the good results of the humidity calibration method using in situ probes in homogeneous soil (Holzman et al., Citation2017; Koyama et al., Citation2017). However for a non-homogeneous soil with strong variations in humidity, several in situ sensors have needed to monitor SM in a large area. GNSS-R allows a sensor to measure SM over a wide area to limit these gaps and reduces monitoring and human resources costs.
6 Conclusions
Long-term continuous SM data are essential in climatic research (Longobardi, Citation2008) and describe the region characteristics (Cleverly et al., Citation2016; Kirkby, Citation2016). Various automated techniques, namely in situ measurements and remote sensing, have been usable in the last years. The IPT is presented by Larson et al. (Citation2008) justified an excellent retrieval of SM for the soil rich in clay (Chew et al., Citation2014; Vey et al., Citation2016; Zhang et al., Citation2017). Our study developed a novel combination between technical IPT and phase unwrapping method to obtain a continuous phase measurement of the SNR multipath, used to monitor SM even for dry to very dry sandy soils (2.5% <VSM <8%). In this case, the correlation coefficient between SM variations in our technique and VSM observed in situ at ~10 cm depth is 0.97 over the study area (95% of sand). Furthermore, the combination of all GPS/GLONASS/GALILEO/BEIDOU satellites in the SNR data treatment can be applied to generate a complete series of time precision, which considerably increase the spatial and temporal resolution from 1 day to 10 min.
Currently, GNSS networks exist in various countries and can be applied GNSS-R tool for free. Another critical point is the number of GNSS satellites which increase continuously from 1990 (~40 compared to more than 120 in 2030 (Gao & Enge, Citation2012)). This factor increases the spatiotemporal monitoring of the Earth, except in the pole areas due to the structures of GNSS orbital planes. GNSS-R SM maps can be established continuously worldwide and can provide powerful time series. Monitoring SM by GNSS-R will be a crucial parameter to improve the understanding of spatial variability of droughts at all spatial and temporal scales in the context of global climate change.
Acknowledgments
This research was funded by the Vietnam National Foundation for Science and Technology Development (NAFOSTED) under grant number 105.08-2020.17, by the University of Toulouse, and by the foundation of Thematic Network for Advanced Research (RTRA) in Science and Technology for Aeronautics and space (STAE) under the MOMMHYS (Mesures d’Opportunités pour la Modélisation Météo-Hydro-Surfaces continentals) project.
Disclosure statement
No potential conflict of interest was reported by the authors.
Data Availability Statement
The data that support the findings of this study are available from the corresponding author, upon reasonable request.
Additional information
Funding
References
- Ahmad, A., Zhang, Y., & Nichols, S. (2011). Review and evaluation of remote sensing methods for soil-moisture estimation. SPIE reviews, 2. https://doi.org/10.1117/1.3534910
- Alonso-Arroyo, A., Camps, A., Aguasca, A., Forte, G., Monerris, A., Rüdiger, C., Walker, J. P., Park, H., Pascual, D., & Onrubia, R. (2014). Improving the accuracy of soil moisture retrievals using the phase difference of the dual-polarization GNSS-R interference patterns. IEEE Geoscience and Remote Sensing Letters, 11(12), 2090–19. https://doi.org/10.1109/LGRS.2014.2320052
- Aranyossy, J., & Ndiaye, B. (1993). Étude et modélisation de la formation des dépressions piézométriques en Afrique sahelienne. Revue des sciences de l’eau, 6(1), 81–96. https://doi.org/10.7202/705167ar
- Baba, K., Bahi, L., & Latifa, O. (2014). Enhancing geophysical signals through the use of Savitzky-Golay filtering method. Geofísica Internacional, 3(4), 399–409. https://doi.org/10.1016/S0016-7169(14)70074-1
- Baup, F., Mougin, E., Rosnay, P., Timouk, F., & Chênerie, I. (2007). Surface soil moisture estimation over the AMMA Sahelian site in Mali using ENVISAT/ASAR data. Remote Sensing of Environment, 109(4), 473–481. https://doi.org/10.1016/j.rse.2007.01.015
- Behari, J. (2006). Microwave dielectric behavior of wet soils. In:School of Environmental Sciences Jawaharlal Nehru University, New Delhi, India 1 (pp. 164). Dordrecht: Springer Dordrecht . doi:10.1007/1-4020-3288-9
- Benoit, A., Pinel-Puysségur, B., Jolivet, R., & Lasserre, C. (2020). CorPhU: an algorithm based on phase closure for the correction of unwrapping errors in SAR interferometry. Geophysical Journal International, 221(3), 1959–1970. https://doi.org/10.1093/gji/ggaa120
- Chew, C. C., & Small, E. E. (2018). Soil moisture sensing using spaceborne GNSS reflections: Comparison of CYGNSS reflectivity to SMAP soil moisture. Geophysical Research Letters, 45(9), 4049–4057. https://doi.org/10.1029/2018GL077905
- Chew, C., Small, E. E., & Larson, K. M. (2016). An algorithm for soil moisture estimation using GPS-interferometric reflectometry for bare and vegetated soil. GPS Solutions, 20(3), 525–537. https://doi.org/10.1007/s10291-015-0462-4
- Chew, C., Small, E. E., Larson, K. M., & Zavorotny, V. U. (2014). Effects of near-surface soil moisture on GPS SNR data: Development of a retrieval algorithm for soil moisture. IEEE Transactions on Geoscience and Remote Sensing, 52(1), 537–543. https://doi.org/10.1109/TGRS.2013.2242332
- Chew, C., Small, E. E., Larson, K. M., & Zavorotny, V. U. (2015). Vegetation sensing using GPS-interferometric reflectometry: Theoretical effects of canopy parameters on signal-to-noise ratio data. IEEE Transactions on Geoscience and Remote Sensing, 53(5), 2755–2764. https://doi.org/10.1109/TGRS.2014.2364513
- Cleverly, J., Eamus, D., Coupe, N. R., Chen, C., Maes, W., Li, L., Faux, R., Santini, N. S., Rumman, R., Yu, Q., & Huete, A. (2016). Soil moisture controls on phenology and productivity in a semi-arid critical zone. Science of The Total Environment, 568, 1227–1237. https://doi.org/10.1016/j.scitotenv.2016.05.142
- Darrozes, J., Roussel, N., & Zribi, M. (2016). The Reflected Global Navigation Satellite System (GNSS-R): From Theory to Practice. In N. Baghdadi & M. Zribi (Eds.), Microwave Remote Sensing of Land Surface (pp. 303–355). Elsevier. https://doi.org/10.1016/B978-1-78548-159-8.50007-4
- Döll, P., Kaspar, F., & Lehner, B. (2003). A global hydrological model for deriving water availability indicators: Model tuning and validation. Journal of Hydrology, 270(1), 105–134. https://doi.org/10.1016/S0022-1694(02)00283-4
- Entekhabi, D., Njoku, E., O’Neill, P., Kellogg, K., Crow, W., Edelstein, W., and Johnson, J. (2010). The soil moisture active passive (SMAP) mission Proceedings of the IEEE ,98(5), 704–716. doi:10.1109/JPROC.2010.2043918.
- Fatras, C., Frappart, F., Mougin, E., Grippa, M., & Hiernaux, P. (2012). Estimating surface soil moisture over Sahel using ENVISAT radar altimetry. Remote Sensing of Environment, 123, 496–507. https://doi.org/10.1016/j.rse.2012.04.013
- Faye, G., Frison, P.-L., Diouf, -A.-A., Wade, S., Kane, C. A., Fussi, F., … Mougin, E. (2017). Soil moisture estimation in Ferlo region (Senegal) using radar (ENVISAT/ASAR) and optical (SPOT/VEGETATION) data. The Egyptian Journal of Remote Sensing and Space Science. https://doi.org/10.1016/j.ejrs.2017.11.005
- Gao, G. X., & Enge, P. (2012). How many GNSS satellites are to many? IEEE Transactions on Aerospace and Electronic Systems, 48(4), 2865–2874. https://doi.org/10.1109/TAES.2012.6324666
- Gdeisat, M. (2018). Performance evaluation and acceleration of Flynn phase unwrapping algorithm using wraps reduction algorithms. Optics and Lasers in Engineering, 110, 172–178. https://doi.org/10.1016/j.optlaseng.2018.02.014
- Gehman, C. L., Harry, D. L., Sanford, W. E., Stednick, J. D., & Beckman, N. A. (2009). Estimating specific yield and storage change in an unconfined aquifer using temporal gravity surveys. Water Resources Research, 45(4), 0043–1397. https://doi.org/10.1029/2007WR006096
- Grinsted, A., Moore, J. C., & Jevrejeva, S. (2004). Application of the cross wavelet transform and wavelet coherence to geophysical time series. Nonlinear Processes in Geophysics, 11(5/6), 561–566. https://doi.org/10.5194/npg-11-561-2004
- Han, L., Chen, L., Zhang, Y., & Qin, X. (2018). A method of microwave soil moisture inversion without dependence on the field measurement data. ISPRS - International Archives of the Photogrammetry, Remote Sensing and Spatial Information Sciences, 3, 459–465. https://doi.org/10.5194/isprs-archives-XLII-3-459-2018
- He, Z., Zhao, W., Liu, H., & Chang, X. (2012). The response of soil moisture to rainfall event size in subalpine grassland and meadows in a semi-arid mountain range: A case study in northwestern China’s Qilian Mountains. Journal of Hydrology, 420, 183–190. https://doi.org/10.1016/j.jhydrol.2011.11.056
- Holzman, M., Rivas, R., Carmona, F., & Niclòs, R. (2017). A method for soil moisture probes calibration and validation of satellite estimates. MethodsX, 4, 243–249. https://doi.org/10.1016/j.mex.2017.07.004
- Hooper, A., & Zebker, H. A. (2007). Phase unwrapping in three dimensions with application to InSAR time series. Journal of the Optical Society of America A, 24(9), 2737–2747. https://doi.org/10.1364/JOSAA.24.002737
- Jones, L. A., Ferguson, C. R., Kimball, J. S., Zhang, K., Chan, S. T., McDonald, K. C., Njoku, E. G., & Wood, E. F. (2010). Satellite microwave remote sensing of daily land surface air temperature minima and maxima from AMSR-E. IEEE Journal of Selected Topics in Applied Earth Observations and Remote Sensing, 3(1), 111–123. https://doi.org/10.1109/JSTARS.2010.2041530
- Karthikeyan, L., Pan, M., Wanders, N., Kumar, D., & Wood, E. (2017). Four decades of microwave satellite soil moisture observations: Part 1. A review of retrieval algorithms. Advances in Water Resources, 109, 106–120. https://doi.org/10.1016/j.advwatres.2017.09.006
- Kerr, Y. (2007). Soil moisture from space: Where are we? Hydrogeology journal, 15(1), 117–120. https://doi.org/10.1007/s10040-006-0095-3
- Kerr, Y., Philippe, W., Wigneron, J.-P., Martinuzzi, J.-M., Font, J., & Berger, M. (2001). Soil moisture retrieval from space: The Soil Moisture and Ocean Salinity (SMOS) mission. IEEE transactions on Geoscience and remote sensing, 39(8), 1729–1735. https://doi.org/10.1109/36.942551
- Kirkby, M. J. (2016). Water in the critical zone: soil, water and life from profile to planet. SOIL, 2(4), 631–645. https://doi.org/10.5194/soil-2-631-2016
- Koster, R., Dirmeyer, P., Guo, Z., Bonan, G., Chan, E., Cox, P., Lawrence, D., Kowalczyk, E., Lawrence, D., Liu, P., Lu, C.-H., Malyshev, S., McAvaney, B., Mitchell, K., Mocko, D., Oki, T., Oleson, K., Pitman, A., Sud, Y. C., … Gordon, C. T. (2004). Regions of strong coupling between soil moisture and precipitation. Science, 305(5687), 1138–1140. https://doi.org/10.1126/science.1100217
- Koyama, C., Liu, H., Takahashi, K., Shimada, M., Watanabe, M., Khuut, T., & Sato, M. (2017). In-situ measurement of soil permittivity at various depths for the calibration and validation of low-frequency SAR soil moisture models by using GPR. Remote Sensing, 9(6), 580. https://doi.org/10.3390/rs9060580
- Kumar, S., Peters-Lidard, C., Eastman, J., & Tao, W.-K. (2008). An integrated high-resolution hydrometeorological modeling testbed using LIS and WRF. Environmental modelling and software, 23(2), 169–181. https://doi.org/10.1016/j.envsoft.2007.05.012
- Laouali, D., Delon, C., Adon, M., Ndiaye, O., Saneh, I., Gardrat, E., Dias-Alves, M., Tagesson, T., Fensohlt, R., & Galy-Lacaux, C. (2021). Source contributions in precipitation chemistry and analysis of atmospheric nitrogen deposition in a Sahelian dry savanna site in West Africa. Atmospheric Research, 251, 105423. https://doi.org/10.1016/j.atmosres.2020.105423
- Larson, K. M., Braun, J. J., Small, E. E., Zavorotny, V. U., Gutmann, E. D., & Bilich, A. L. (2010). GPS multipath and its relation to near-surface soil moisture content. IEEE Journal of Selected Topics in Applied Earth Observations and Remote Sensing, 3, 91–99. https://doi.org/10.1109/JSTARS.2009.2033612
- Larson, K. M., Small, E. E., Gutmann, E. D., Bilich, A. L., Braun, J. J., & Zavorotny, V. U. (2008). Use of GPS receivers as a soil moisture network for water cycle studies. Geophysical Research Letters, 35. https://doi.org/10.1029/2008GL036013
- Lebel, T., Cappelaere, B., Galle, S., Hanan, N., Kergoat, L., Levis, S., Vieux, B., Descroix, L., Gosset, M., Mougin, E., Peugeot, C., & Seguis, L. (2009). AMMA-CATCH studies in the Sahelian region of West-Africa: An overview. Journal of Hydrology, 375(1–2), 3–13. https://doi.org/10.1016/j.jhydrol.2009.03.020
- Li, F., Peng, X., Chen, X., Liu, M., & Xu, L. (2018). Analysis of Key Issues on GNSS-R Soil Moisture Retrieval Based on Different Antenna Patterns. Sensors, 18(8), 2498. https://doi.org/10.3390/s18082498
- Lomb, N. R. (1976). Least-squares frequency analysis of unequally spaced data. Astrophysics and Space Science, 39(2), 447–462. https://doi.org/10.1007/BF00648343
- Longobardi, A. (2008). Observing soil moisture temporal variability under fluctuating climatic conditions. Hydrology and Earth System Sciences Discussions, 5, 935–969. https://doi.org/10.5194/hessd-5-935-2008
- Lopez, T., Antoine, R., Kerr, Y., Darrozes, J., Rabinowicz, M., Ramillien, G., Cazenave, A., & Genthon, P. (2016). Subsurface hydrology of the Lake Chad Basin from convection modelling and observations. Surveys in Geophysics, 37(2), 471–502. https://doi.org/10.1007/s10712-016-9363-5
- Lu, Y., & Zebker, H. A. (1998). Phase unwrapping algorithms for radar interferometry: residue-cut, least-squares, and synthesis algorithms. Optical Society of America 15 3 586–598 doi:10.1364/JOSAA.15.000586 .
- Martínez-Fernández, J., González-Zamora, Á., & Almendra-Martín, L. (2021). Soil moisture memory and soil properties: An analysis with the stored precipitation fraction. Journal of Hydrology, 593, 125622. https://doi.org/10.1016/j.jhydrol.2020.125622
- Mitchell, K., Lohmann, D., Houser, P., Wood, E., Schaake, J., Robock, A., & Luo, L. (2004). The multi‐institution North American Land Data Assimilation System (NLDAS): Utilizing multiple GCIP products and partners in a continental distributed hydrological modeling system. Journal of Geophysical Research: Atmospheres, 109(D7). https://doi.org/10.1029/2003JD003823
- Mougin, E., Hiernaux, P., Kergoat, L., Manuela, G., Rosnay, P., Timouk, F., Le Dantec, V., Demarez, V., Lavenu, F., Arjounin, M., Lebel, T., Soumaguel, N., Ceschia, E., Mougenot, B., Baup, F., Frappart, F., Frison, P. L., Gardelle, J., Gruhier, C., … Mazzega, P. (2009). The AMMA-CATCH Gourma observatory site in Mali: Relating climatic variations to changes in vegetation, surface hydrology, fluxes and natural resources. Journal of Hydrology, 375(1–2), 14–33. https://doi.org/10.1016/j.jhydrol.2009.06.045
- Njoku, E. G., & Chan, S. K. (2006). Vegetation and surface roughness effects on AMSR-E land observations. Remote Sensing of environment, 100(2), 190–199. https://doi.org/10.1016/j.rse.2005.10.017
- Njoku, E. G., & Entekhabi, D. (1996). Passive microwave remote sensing of soil moisture. Journal of Hydrology, 184(1–2), 101–129. https://doi.org/10.1016/0022-1694(95)02970-2
- Paloscia, S., Macelloni, G., Santi, E., & Koike, T. (2001). A multifrequency algorithm for the retrieval of soil moisture on a large scale using microwave data from SMMR and SSM/I satellites. IEEE Transactions on Geoscience and Remote Sensing, 39(8), 1655–1661. https://doi.org/10.1109/36.942543
- Peel, M., Finlayson, B., & McMahon, T. (2007). Updated world map of the Köppen-Geiger climate classification. Hydrology and Earth System Sciences, 11(5), 1633–1644. https://doi.org/10.5194/hess-11-1633-2007
- Peng, J., & Loew, A. (2017). Recent advances in soil moisture estimation from remote sensing. Water, 9(7), 530. https://doi.org/10.3390/w9070530
- Ramillien, G., Seoane, L., & Darrozes, J. (2021). An innovative Slepian approach to invert GRACE KBRR for localized hydrological information at the sub-basin scale. Remote Sensing, 13(9), 1824. https://doi.org/10.3390/rs13091824
- Rascon, A., Martinez-Salvador, M., Sosa, G., Villarreal-Guerrero, F., Pinedo, A., Santellano-Estrada, E., & Corrales-Lerma, R. (2021). Soil moisture dynamics in response to precipitation and thinning in a semi-dry forest in Northern Mexico. Water, 13(105). https://doi.org/10.3390/w13010105
- Ribot, M. A., Botteron, C., & Farine, P.-A. (2016). Derivation of the Cramér-Rao bound in the GNSS-reflectometry context for static, ground-based receivers in scenarios with coherent reflection. Sensors, 16(12), 2063. https://doi.org/10.3390/s16122063
- Rodell, M., Houser, P. R., Jambor, U., Gottschalck, J., Mitchell, K., Meng, C.-J., Arsenault, K., Cosgrove, B., Radakovich, J., Bosilovich, M., Entin, J. K., Walker, J. P., Lohmann, D., & Toll, D. (2004). The global land data assimilation system. Bulletin of the American Meteorological Society, 85(3), 381–394. https://doi.org/10.1175/BAMS-85-3-381
- Rodriguez-Alvarez, N., Camps, A., Vall-llossera, M., Bosch-Lluis, X., Monerris, A., Ramos-Perez, I., Valencia, E., Marchan-Hernandez, J. F., Martinez-Fernandez, J., Baroncini-Turricchia, G., Perez-Gutierrez, C., & Sanchez, N. (2011). Land geophysical parameters retrieval using the interference pattern GNSS-R technique. IEEE Transactions on Geoscience and Remote Sensing, 49(1), 71–84. https://doi.org/10.1109/TGRS.2010.2049023
- Rodriguez-Iturbe, I., & Porporato, A. (2004). Ecohydrology of water-controlled ecosystems: Soil moisture and plant dynamics. Cambridge University Press. https://doi.org/10.1017/CBO9780511535727
- Rosnay, P. D., Gruhier, C., Timouk, F., Baup, F., Mougin, E., Hiernaux, P., LeDantec, V., & LeDantec, V. (2009). Multi-scale soil moisture measurements at the Gourma meso-scale site in Mali. Journal of Hydrology, 375(1–2), 241–252. https://doi.org/10.1016/j.jhydrol.2009.01.015
- Roussel, N. (2015). Application de la réflectométrie GNSS à l’étude des redistributions des masses d’eau à la surface de la Terre. PhD thesis, Université Paul Sabatier -. https://tel.archives-ouvertes.fr/tel-01417284
- Roussel, N., Frappart, F., Ramillien, G., Darrozes, J., Baup, F., Lestarquit, L., & Ha, M. C. (2016). Detection of soil moisture variations using GPS and GLONASS SNR data for elevation angles ranging from 2° to 70°. IEEE Journal of Selected Topics in Applied Earth Observations and Remote Sensing, 9(10), 4781–4794. https://doi.org/10.1109/JSTARS.2016.2537847
- Roussel, N., Frappart, F., Ramillien, G., Perosanz, F., Perosanz, F., Perosanz, F., & Biancale, R. (2014). Simulations of direct and reflected waves trajectories for in situ GNSS-R experiments. Geoscientific Model Development, 7(5), 2261–2279. https://doi.org/10.5194/gmdd-7-1001-2014
- Roxy, M. S., Sumithranand, V. B., & Renuka, G. (2010). Variability of soil moisture and its relationship with surface albedo and soil thermal diffusivity at Astronomical Observatory, Thiruvananthapuram, south Kerala. Journal of Earth System Science, 119(4), 507–517. https://doi.org/10.1007/s12040-010-0038-1
- Scargle, J. D. (1982). Studies in astronomical time series analysis. II - Statistical aspects of spectral analysis of unevenly spaced data. Astrophysical Journal, 263(2), 835–853. https://doi.org/10.1086/160554
- Schafer, R. W. (2011). What is a Savitzky-Golay filter? IEEE Signal Processing Magazine, 28(4), 111–117. https://doi.org/10.1109/MSP.2011.941097
- Smith, L. C. (2002). Emerging applications of Interferometric Synthetic Aperture Radar (InSAR) in geomorphology and hydrology. Annals of the Association of American Geographers, 92, 385–398. https://doi.org/10.1111/1467-8306.00295
- Steelman, C. M., Endres, A. L., & Jones, J. P. (2012). High‐resolution ground‐penetrating radar monitoring of soil moisture dynamics: Field results, interpretation, and comparison with unsaturated flow model. Water Resources Research, 48(9). https://doi.org/10.1029/2011WR011414
- Sun, H., & Cui, Y. (2021). Evaluating downscaling factors of microwave satellite soil moisture based on machine learning method. Remote Sensing, 13(133). doi:10.3390/rs13010133.
- Sun, F., Lu, Y., Wang, J., Hu, J., & Fu, B.-J. (2015). Soil moisture dynamics of typical ecosystems in response to precipitation: A monitoring-based analysis of hydrological service in the Qilian Mountains. Catena, 129, 63–75. doi:10.1016/j.catena.2015.03.001.
- Tagesson, T., Rasmus, F., Idrissa, G., Olander, R. M., Silvia, H., Cheikh, M., Jonas, A., Sandholt, I., Holm‐Rasmussen, B., Göttsche, F. M., Ridler, M.-E., Olén, N., Lundegard Olsen, J., Ehammer, A., Madsen, M., Olesen, F. S., Ardö, J., & Garcia, M. (2015). Ecosystem properties of semiarid savanna grassland in West Africa and its relationship with environmental variability. Global Change Biology, 21(1), 250–264. https://doi.org/10.1111/gcb.12734
- Torrence, C., & Compo, G. P. (1998). A practical guide to wavelet analysis. Bulletin of the American Meteorological Society, 79(1), 61–78. https://doi.org/10.1175/1520-0477(1998)079<0061:APGTWA>2.0.CO;2
- Torrence, C., & Webster, P. J. (1999). Interdecadal changes in the ENSO–Monsoon system. Journal of Climate, 12, 2679–2690. https://doi.org/10.1175/1520-0442(1999)012<2679:ICITEM>2.0.CO;2
- Ulaby, F. T., & Moore, R. K. (1986). Microwave remote sensing: Active and passive, from theory to applications (Vol. 3). NASA.
- Vey, S., Güntner, A., Wickert, J., Blume, T., & Ramatschi, M. (2016). Long-term soil moisture dynamics derived from GNSS interferometric reflectometry: a case study for Sutherland, South Africa. GPS Solutions, 20(4), 641–654. https://doi.org/10.1007/s10291-015-0474-0
- Walker, J. P., Willgoose, G. R., & Kalma, J. D. (2004). In situ measurement of soil moisture: a comparison of techniques. Journal of Hydrology, 293(1–4), 85–99. https://doi.org/10.1016/j.jhydrol.2004.01.008
- Wang, L., & Qu, J. (2009). Satellite remote sensing applications for surface soil moisture monitoring: A review. Frontiers of Earth Science in China, 3(2), 237–247. https://doi.org/10.1007/s11707-009-0023-7
- Wen, J., Jackson, T. J., Bindlish, R., Hsu, A. Y., & Su, Z. B. (2005). Retrieval of soil moisture and vegetation water content using SSM/I data over a corn and soybean region. Journal of Hydrometeorology 66, 854–863, http://www.jstor.org/stable/24910094
- Wigneron, J.-P., Jackson, T., O’Neill, P., De Lannoy, G., Rosnay, P., Walker, J., Das, N., Bircher, S., Grant, J. P., Kurum, M., Schwank, M., Munoz-Sabater, J., Das, N., Royer, A., Al-Yaari, A., Al Bitar, A., Fernandez-Moran, R., Lawrence, H., Mialon, A., … Ferrazzoli, P. (2021). Modelling the passive microwave signature from land surfaces: A review of recent results and application to the L-band SMOS & SMAP soil moisture retrieval algorithms. Remote Sensing of Environment, 192, 238–262. https://doi.org/10.1016/j.rse.2017.01.024
- Xu, C., Qu, J. J., Hao, X., Cosh, M. H., Prueger, J. H., Zhu, Z., & Gutenberg, L. (2018). Downscaling of surface soil moisture retrieval by combining MODIS/Landsat and in situ measurements. Remote Sensing, 10(2), 210. https://doi.org/10.3390/rs10020210
- Xu, C., Qu, J. J., Hao, X., & Wu, D. (2020). Monitoring Surface soil moisture content over the vegetated area by integrating optical and SAR satellite observations in the Permafrost Region of Tibetan Plateau. Remote Sensing, 12(1), 183. https://doi.org/10.3390/rs12010183
- Zebker, H. A., & Yanping, L. (1998). Phase unwrapping algorithms for radar interferometry: Residue-cut, least-squares, and synthesis algorithms. Journal of the Optical Society of America A, 15(3), 586–598. https://doi.org/10.1364/JOSAA.15.000586
- Zeiger, P., Frappart, F., Darrozes, J., Roussel, N., Bonneton, P., Bonneton, N., & Detandt, G. (2021). SNR-based water height retrieval in rivers: Application to high amplitude asymmetric tides in the Garonne River. Remote Sens, 13(9), 1856. https://doi.org/10.3390/rs13091856
- Zhang, S., Calvet, J.-C., Darrozes, J., Roussel, N., Frappart, F., & Bouhours, G. (2018). Deriving surface soil moisture from reflected GNSS signal observations from a grassland site in southwestern France. Hydrology and Earth System Sciences, 22(3), 1931–1946. https://doi.org/10.5194/hess-22-1931-2018
- Zhang, S., Roussel, N., Boniface, K., Minh Cuong, H., Frappart, F., Darrozes, J., Baup, F., & Calvet, J.-C. (2017). Use of reflected GNSS SNR data to retrieve either soil moisture or vegetation height from a wheat crop. Hydrology and Earth System Sciences, 21(9), 4767–4784. https://doi.org/10.5194/hess-21–4767-2017