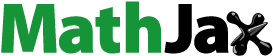
ABSTRACT
This study focused on monitoring the water status of vegetation and soil by exploiting the synergy of optical and microwave satellite data with the aim of improving the knowledge of water cycle in cultivated lands in Egyptian Delta and Tunisian areas. Environmental analysis approaches based on optical and synthetic aperture radar data were carried out to set up the basis for future implementation of practical and cost-effective methods for sustainable water use in agriculture. Long-term behaviors of vegetation indices were thus analyzed between 2000 and 2018. By using SAR data from Sentinel-1, an Artificial Neural Network-based algorithm was implemented for estimating soil moisture and monthly maps for 2018 have been generated to be compared with information derived from optical indices. Moreover, a novel drought severity index was developed and applied to available data. The index was obtained by combining vegetation soil difference index, derived from optical data, and soil moisture content derived from SAR data. The proposed index was found capable of complementing optical and microwave sensitivity to drought-related parameters, although ground data are missing for correctly validating the results, by capturing drought patterns and their temporal evolution better than indices based only on microwave or optical data..
Introduction
The regions characterized by low values of aridity index (expressed as the ratio of the total annual precipitation, as indicator of water supply, to potential evapotranspiration (ET), as indicator of water consumption) are classified as dry lands; among them, the semi-arid regions are those with an aridity index between 0.20 and 0.50 (Lal, Citation2004). These regions are populated by about 1.5 billion people, for the most part belonging to the “needy” category of individuals living in rural areas and without security of food (Ryan & Spencer, Citation2001).
Semi-arid regions are characterized by severe climate with high temperatures, scarce and difficultly predictable rainfalls and high evaporation: these conditions deeply affect the availability of water for the agricultural practices (Kottek et al., Citation2006; Sommer et al., Citation2011). Despite these limitations, semi-arid ecosystems act as effective Carbon sinks, playing a relevant role in the global Carbon cycle as well as climate regulators. With 0.04 Pg C year−1 on a global 0.07 Pg C year− 1, semi-arid ecosystems contribute more than 57% to the interannual variability of land CO2 sink (Ahlström et al., Citation2015).
Climate changes affect the Mediterranean area stronger than average global trends; worldwide increase of 2°C could mean an increase of 3°C in the Mediterranean region (MedECC, Citation2020) that can be therefore considered as a “hot spot” for climate change (Giorgi & Lionello, Citation2008; Lionello & Scarascia, Citation2018; Tramblay et al., Citation2020) locally leading to a reduction (10–30%) of summer precipitation as well as to phenomena of intense drought and desertification (IPCC, Citation2019; MedECC, Citation2020).
Moreover, irrigation water request is expected increasing from 4 to 18% by 2100 (MedECC, Citation2020) due to both agricultural intensification and global warming, thus bringing the problem of water management in arid and semi-arid countries to the fore. Water management is not an easy problem to be tackled due to the multiple aspects of the problem related to socio-economic development of each region and available economic resources.
Microwave and optical remote sensing data, available in a wide range of different spatial resolution to be used in local, regional and global environments, have demonstrated their effectiveness in describing land cover characteristics and changes due to climatic variations coming from both natural and anthropic factors (Roy et al., Citation2014). Large quantity of bands in optical remote sensing data, such as multispectral and hyperspectral ones, together with previously mentioned wide range of spatial resolution, allows deriving dedicated spectral indexes for monitoring a wide spectrum of phenomena at different scales.
Among the others, Normalized Difference Vegetation Index (NDVI) has been used to analyze the spatial and temporal evolution of drought and vegetation conditions in arid environment (Akinyemi, Citation2021) pointing out its strong ability in detecting temporal and spatial variability in vegetation productivity between drought and non-drought conditions. NDVI has also been used to conduct long-term greenery analysis (De Jong et al., Citation2011), land cover change monitoring in arid and semi-arid environments (Allam et al., Citation2019) and, in southern Tunisia, (Fayech & Tarhouni, Citation2021) to highlight a positive relation between NDVI and rainfall as well as a direct correlation between rainfall and vegetation status. Also Enhanced Vegetation Index (EVI) has been used to highlight the correlations between environmental components: a good correlation has been reported among EVI, rainfall and temperature over a semi-arid region in Mexico (Huete et al., Citation2002; Justice et al., Citation1998; Olmos-Trujillo et al., Citation2020) but also with estimation and prediction of agricultural crop biomass (Abdel-Kader, Citation2019; Jaafar & Ahmad, Citation2015), Gross Primary Productivity (John et al., Citation2008) and land surface temperature (LST; Xie et al., Citation2022). The anomalies in EVI time series were found to be useful in describing vegetation degradation (Javzandulam et al., Citation2005) and the effects of drought on vegetation productivity (Zhu et al., Citation2021), since water deficiencies and EVI values were strictly correlated, in particular during vegetation growing season regardless of land cover type (natural vegetation or agricultural zones).
Another index that has been proven to be very effective in drought assessment in semi-arid zones (F. N. Kogan, Citation1995) was the Vegetation Health Index (VHI), able to detect extreme drought events (Ekundayo et al., Citation2021) better than meteorological parameters such as the Standard Precipitation Index (Mckee et al., Citation1993). The direct correlation between VHI and rainfall has also been pointed out by studies performed in semi-arid areas in Ethiopia for long-term agricultural drought monitoring (Gidey et al., Citation2018), thus confirming the results of a research carried out in North America (Sun & Kafatos, Citation2007) where lower NDVI and LST values identify poor vegetation health condition.
In addition to temperature, ET is one of the key elements of the hydrological cycle whose significant and representative measurements are very difficult to be obtained, especially in arid and semi-arid areas where water loss may be extremely variable in time and space (Bhaskar, Citation2014). The Moderate Resolution Imaging Spectroradiometer (MODIS) dedicated product (MOD16) is based on the model described by Qiaozhen et al. (Citation2011) and provides ET estimates by improving, with a frequent time acquisition (every 8 days), previously available similar data (Bastiaanssen et al., Citation2005, Citation2005). As an example, MOD16 was used to estimate and monitor hydrological parameters in the Tibet plateau (Yuan et al., Citation2021) and Australia (Khan et al., Citation2020), trying to mitigate the constraints due to low spatial resolution (Mingzhu et al., Citation2019).
The previously described optical products can be integrated by microwave remote sensing data, both active and passive, that are limited neither by the absence of solar light nor by cloud cover; moreover, microwave signal is mainly influenced by the dielectric constant that is related to the water content of the observed bodies.
The effectiveness of active sensors, especially SAR operating at L-band, in estimating soil moisture content (SMC) at regional and local scales has been confirmed by studies realized in agricultural test sites in India (Zribi et al., Citation2019) using Phased Array L-band Synthetic Aperture Radar (PALSAR) data. The results of this and past studies highlight that from C to L bands the backscatter sensitivity to SMC is generally reduced when moving from bare soil to vegetated areas (Anna et al., Citation2011).
As the European Space Agency (ESA) Sentinel-1 (S-1) constellation provides open data, scientific community has the access to free SAR images regularly acquired with high spatial and temporal resolutions with respect to previous satellites.
C-band backscatter, although with a lower sensitivity to soil moisture, due to the scarce soil penetration depth and the strong influence of vegetation cover and surface roughness with respect to L-band, has provided encouraging SMC estimates, also due to the large quantity of available images and the application of machine learning approaches (Emanuele Santi, Citation2016; Hachani et al., Citation2019; S. Paloscia et al., Citation2013).
The integration of microwave and optical observation can be helpful especially for the monitoring of global changes in semi-arid regions, affected by increasing drought and water waste processes.
Many research projects have been funded in recent years by international and national organizations to contribute to the improvement of water management and to provide more exhaustive information and accurate models concerning the hydrological cycle and the vegetation status monitoring. H2020 EC recent calls addressed the issue of water scarcity and drought not only in North Africa, but also in European countries where climate change, combined with water competition, dramatically worsened the situation. Among them we can cite e.g. the Water Footprint Network for the Improving Predictions and Management of Hydrological Extremes (IMPREX, https://www.imprex.eu/) and the Transforming Water, Weather, and Climate Information through In Situ observations for Geo-Services in AFRICA (TWIGA, https://website.twiga-h2020.eu/), both projects funded through the European Union Horizon-2020 grant programme.
Recently, a research project, which aimed at proposing practical and cost-effective solutions for implementing innovative water management systems in the agricultural Mediterranean environment, was carried out in the framework of an ERANET-MED project (OptiMed-Water). This project involved partners from Italy (Institute of Applied Physics of National Research Council, CNR-IFAC), Germany (German Aerospace Center, DLR), Tunisia (National Agronomic Institute of Tunisia, INAT, and Institute of Arid Regions, IRA) and Egypt (Cairo University, CU, and the Central Laboratory for Agricultural Climate, CLAC). Optical and microwave satellite data for monitoring soil and vegetation water status have been exploited with the aim of improving the knowledge of the water cycle in arid and semi-arid regions. Some agricultural areas in Egypt and Tunisia, that are characterized by chronic water scarcity, recurrent droughts and strong impacts of climate change, were selected and monitored by using satellite data and available in situ ancillary information. An available database of historical data was considered and used for an in-depth investigation of these key-areas, which are of particular interest in view of the socio-economic development of the regions. Dedicated experimental campaigns, mainly in Tunisia test areas, have been also carried out for collecting ground measurements of soil and crop parameters.
An investigation of the soil and vegetation water status was carried out during this project, and some algorithms capable of exploiting remote sensing data for estimating the main parameters of the hydrological cycle have been developed. In the first part of the paper, the analysis of vegetation indices and their anomalies over Nile Delta using a long-term exploitation of MODIS data was described, followed by an investigation of ET processes in both Nile Delta and in the two areas located in Tunisia (Boulet et al., Citation2019; El-Shirbeny et al., Citation2015; Hesham & Khalil, Citation2015). In parallel, the SMC of the three study areas was monitored at high spatial resolution by using C-band SAR data derived from S-1 in 2018. The SMC retrieval concept was based on Artificial Neural Network (ANNs) by reappraising the methodology proposed in (Emanuele Santi, Citation2016), which was proven to be capable of estimating SMC in semi-arid environments too (Hachani et al., Citation2019).
The comparison of SMC retrievals with the ET and vegetation indices confirmed an overall agreement, by also pointing out peculiar sensitivities of optical- and microwave-derived parameters that have suggested combining them for setting up an integrated microwave/optical drought severity index (CDSI). CDSI has been developed during the project and was found to be capable of monitoring the drought conditions of the three test areas, by also identifying that drought patterns than optical data or microwave SMC alone were not capable to detect.
The paper is structured as follows: a synthetic description of test areas and datasets is provided in in sections 1 and 2, respectively; the methods based on optical and microwave data for monitoring vegetation and soil conditions are described in section 3 where the newly developed CDSI is also proposed. The results are shown in section 4 and, finally, their discussion is carried out in section 5. Appendix I provides additional details on study areas while Appendix II contains full set of images for the multitemporal analysis results.
Test areas and data
Study areas
Project study areas are located in semi-arid zones of Tunisia (Mergeullil and Medenin test sites) and Egypt (Nile Delta) as shown in .
Figure 1. On the left, the boundaries of the test areas of Medenine (red) in the South-East of Tunisia, and Merguellil basin (purple) in North-West of Tunisia are shown. On the right, Nile Delta test site in Egypt is highlighted by a blue line.

The main reasons for selecting these areas consist of the fact that these zones are mainly dedicated to agricultural practices, both irrigated and rainfed, and have been deeply studied in the past, by gathering satellite and auxiliary data, thus representing priority areas for rural analysis in both countries. Additional information concerning extension, climatic data and more detailed maps are reported in Appendix I.
Egypt – Nile Delta
The Nile Delta () contains more than 60% of the cultivated lands of the country, thus representing the most important agricultural area in Egypt (Hamza, Citation2006). The Nile Delta is also a problematic region due to land degradation processes, such as drought, soil compaction and salinization (Fishar, Citation2018).
The test area selected for the project is approximately 160 × 250 km and covers the entire Kafr El-Shikh, Damietta, Port Said, Dakahlia, Gharbia, Behera, Menoufia, Sharkia, Alexandria, Ismailia and Qalyubia governorates.
Tunisia – Medenine
The test area of North Medenine is centred on the North of the homonymous province located in the south of Tunisia (). The frame of the test area, which is managed by IRA, is 170 × 120 km.
The Province of Medenine is strategically located in the center of the Mediterranean basin. According to Tunisian South Development Office (2015), land cover of this area is dominated by rangelands (65.5%) followed by agriculture (22.1%), which is based on olive tree farming that covers 198,500 ha, representing therefore more than 84% of the total cultivable area in the governorate and includes more than 4.5 million trees (JICA, Yachiyo Engineering Co., Kaihatsu Management Consulting and Corporation Ingérosec, Citation2015).
Tunisia – Merguellil
The North Tunisian test area is centred on the Merguellil basin, South of Kairouan city (35°40ʹ12” N, 10°5ʹ24” E) and its monitoring is managed by INAT ().
The Upper Merguellil is a hilly area of about 1200 km2, with altitude variable between 200 and 1200 m a.s.l., that is rather different from the very flat lower Merguellil plain (more than 3000 km2).
Cereals, tomato, melon, watermelon and chili are cultivated, although olive is the main crop of that area making Tunisia one of the main distributors of olive products (fruits and oil) in the world.
Satellite data
Time series of satellite data available on the test areas from both optical and microwave sensors have been identified and downloaded from the respective data portals. The period investigated by each series of data ranged from one to several years depending on the specific goal of each performed analysis.
Optical satellite data and products
Optical data have been derived from the MODIS instrument onboard the Terra Satellite as described later.
ET maps have been obtained from 46 images for the region of Nile Delta (Egypt) and 42 for Merguellil basin in Tunisia in the period November 2017–October 2018 from MOD16A2 ET/Latent Flux 500 m pixel resolution product. Unfortunately, no images were found to produce the same ET maps on the Medenine test site. Monthly NDVI maps (2001–2017) have been obtained from the MODIS-Terra NDVI V6 with 500 meters spatial horizontal resolution (MOD13A1) where the 16-day series best available pixel value is derived. MODIS-Terra EVI maps V6 (MODIS13Q1 product having 250-m spatial resolution, 16-day composites) series of the meteorological vegetation period, from 2000 to 2018, were analyzed both annually and seasonally.
The MODIS-Terra Land Surface Temperature/Emissivity Daily L3 (MOD11A1 global product at 1-km resolution) collected for the period 2001–2017 has been also considered for the scopes of this research.
SAR data
The SAR data are derived from the ESA (S-1) constellation as C-band backscatter in VV and VH polarizations.
The high spatial resolution (around 10 m) and the frequent revisit time (6 days) of C-band SAR S-1 data have been exploited for mapping SMC over the Nile Delta, Merguellil basin and Medenine test sites. The Interferometric Wide Swath Mode – High Resolution Ground Range (IW-GRDH) product was selected and scenes from orbits 88 and 58 were downloaded and processed for Tunisia and Egypt, respectively. Finally, 140 S-1 frames for Tunisia and 120 frames for the Nile Delta were acquired in the period 2017–2019.
Radiometric calibration and geocoding were applied for each image and polarization: geocoding transforms the two-dimensional coordinates in range and azimuth of the backscatter coefficient (σ°) values into three-dimensional coordinates referred to a cartographic reference system. To do this, an interpolated Digital Elevation Model of the test areas was derived from Shuttle Radar Topography Mission (SRTM) data considering 30 m pixel size (http://srtm.csi.cgiar. org/srtmdata/). In addition to the geocoded image, a map of local incidence angles was generated and, finally, the calibrated and geocoded satellite images were co-registered and saved in a single stack of data to compare each satellite image “pixel by pixel”.
Methods
The drought temporal and spatial behaviors have been investigated in the three test areas by analyzing some vegetation and soil indices derived from both optical and microwave data. A flow chart summarizing the scheme of the activities is presented in . In the following, the different methods applied for reaching these tasks are described.
Vegetation and soil water status assessment
The vegetation anomalies have been investigated by using NDVI temporal trends, which is considered a quick and efficient way for identifying green vegetation due to its correlation with the level of photosynthetic activity and consequent stress level (Senay et al., Citation2015). With the aim of identifying the priorities or “hot spot” regions in the Nile Delta domain, the positive and negative changes in the NDVI data have been analyzed for the years from 2013 to 2017 and compared with the average NDVI for the 2001–2012 period. The vegetation density was grouped in four classes by using NDVI (0–0.3: No vegetation, 0.3–0.5: Low vegetation, 0.5–0.7: Mild vegetation and >0.7: High vegetation). Given the lower vegetation density in the two test areas of Tunisia, this analysis was not repeated.
The drought spatial patterns and their temporal behaviors have been analyzed with the support of two other optical indices, the VHI (Felix N. Kogan, Citation2001) and the Vegetation Soil Difference Index (VSDI), which was firstly defined in Ning et al., (Citation2013). VHI is obtained by combining normalized EVI and LST and then it has been classified into five classes. VHI has been analysed in the Nile Delta area, from 2000 to 2017, by considering the MODIS TERRA available data in the periods from October to May. The other index (VSDI) is based on the difference between the short-wave infrared and red bands, which are sensitive to moisture, and the blue reference band. In vegetated surfaces the VSDI ranges between 0, corresponding to very dry conditions, and 1, corresponding to very moist conditions. The effectiveness of VSDI was demonstrated in Ning et al., (Citation2013). VSDI values have been computed for the three test areas and both spatial patterns and temporal trends of averaged values have been identified.
The drought tendencies were also investigated by using a drought model based on the EVI MODIS 16-day composite images. EVI is effective in the determination of drought characteristics, both as temporal variability and patterns, due to its high dependence on changes in canopy type and architecture as well as in plant physiognomy.
The model inputs were the EVI QA layer at 250 m spatial resolution derived from MODIS13Q1 16-days composite in Version 6 and the MODIS Land Cover Type Product MCD12Q1 that provides a 500 m spatial resolution land cover mapping at a global scale. Land cover was used for the Nile Delta and the Merguellil basin, while a shapefile including the limits of all areas cultivated with olive trees was used for the Medenine region. The time-series analysis was carried out on more than 700 MODIS images between 2001 and 2018 using only data with QA layer class = 0 pixel; negative values have been masked. Calculations of the 16-day composite long term medians have been performed together with the calculation of their anomalies, computed as differences between composite and long-term median for every 16-day composite.
A temporal aggregation was then carried out to compute monthly EVI deviances for the total vegetated area and croplands. This processing was composed of two steps: first, the pixel-wise standard deviations have been calculated and then the pixels with EVI value higher than μ + 2σ or lower than μ-2σ per month have been counted and averaged for vegetated area, cropland and olive trees. The processing of data was implemented in Google Earth Engine: a flow chart of the drought model is shown in .
Soil moisture
To further investigate the regional water cycle and assess the soil water status, which has been already pointed out by optical indices, the SMC has been monitored in the three test areas by using the SAR data and in particular the available S-1 images. The retrieval algorithm was based on ANNs that have been already demonstrated to be very effective for this kind of applications in previous studies (e.g. Baghdadi et al., Citation2002; Del Frate et al., Citation2003; S. Paloscia et al., Citation2013; Rodríguez-Fernández et al., Citation2015); the SMC algorithm proposed here is an updated implementation of the one proposed in Emanuele Santi, (Citation2016). Given the limited amount of in situ data, a hybrid data + model driven approach has been implemented here for training the algorithm, by merging the experimental data with simulation based on a coupled implementation of IEM (Fung, Citation1994) and Water Cloud Model (Attema & Fawwaz, Citation1978), to generate a training set composed of surface parameters and corresponding simulated, or measured, σ° values (Emanuele Santi, Paloscia, Pettinato, Fontanelli et al., Citation2016).
The training set was then subsampled in three subsets composed of 60%, 20% and 20% of the dataset, for applying the so-called early stopping rule, aimed at preventing overfitting. The optimal number of neurons and hidden layers for the given problem is defined by the iterative search described in Tarpanelli et al., (Citation2019). The search returned an ANN having two hidden layers of 10 neurons each, with a transfer function of type “logsig”. Inputs of the ANN were the σ° at the two available polarizations, namely VV and VH, and the output was the SMC. The training/validation process is summarized in the flow chart of : a schematic representation of the ANN with inputs and outputs is shown in the box on the right.
Figure 4. Flowchart of the training/test set generation. The simplified scheme of ANN is shown in the bottom right box.

Before applying it and generate SMC maps, the algorithm has been validated by using the available in situ measurements for Tunisia: the scatterplot of ANN estimated vs. in situ SMC is shown in ; the corresponding statistics are R = 0.88 and RMSE< 2(% of SMC).
By applying the ANN algorithm to the S-1 time series available on the three test areas, maps of SMC (%) have been generated at a spatial resolution of 100 m and with the same revisiting frequency (6 days) of the S-1 SAR images considered as algorithm inputs. The analysis has been then focused on the entire 2018 year, for observing a complete seasonal cycle. The 81 SMC maps generated on the three areas for 2018 have been then monthly averaged for enabling the comparison with the optical indices.
Finally, the derived optical and microwave parameters have been integrated in a novel index, which enhances their own peculiar sensitivities to soil and vegetation parameters. Microwaves, given their longer wavelength and consequent higher penetration, are more influenced by the soil contribution, while visible and shortwave infrared are better sensitive to the vegetation canopy. To exploit these different sensitivities, a Combined microwave + optical/infrared Drought Severity Index (CDSI) is proposed here, by combining the SMC derived from microwave data and the VSDI derived from optical/infrared data.
The combined index was computed as follows: first, SMC derived from microwave data is rescaled to the 0–1 range:
where SMCn is the normalized soil moisture, SMC is the actual soil moisture and SMCmax and SMCmin are the highest and lowest values recorded in the considered time period (one year).
The CDSI is then computed as the weighted average of SMCn and VSDI:
where w is a weighting coefficient with value between 0 and 1; in this study it has been empirically set to 0.5 after comparing the CDSI obtained for different w values with the in situ information. The obtained CDSI, that ranges between 0 and 1, is then classified into five classes ():
Table 1. Drought severity classification based on CDSI.
Results
Due to the different environmental conditions which characterize the test areas considered in this work, the result has been described separately for each test area. The analysis included a spatial assessment of the NDVI, EVI, VDSI and SMC patterns and a comparison of the temporal trends. Based on the obtained results, the drought severity was assessed by applying the combined optical + microwaves CDSI which has been developed in this study.
Vegetation anomalies
The NDVI maps for the month of January in the Nile Delta Governorates are displayed in . The reference NDVI map for the period 2001–2012 is shown in top-left panel: the map well reflects the expected vegetation condition by showing that the areas with higher vegetation density are located inside the Nile Delta. The maps of vegetation changes derived from NDVI for the years from 2013 to 2017 are shown in the other panels of .
Figure 6. Vegetation map of Nile Delta in January of 2001–2012 (top left) compared with the change (+ve green and -ve red) maps during the years from 2013 to 2017.

The areas that moved from mild/high density vegetation to low or no-vegetation cover (-ve change) are represented in red color while areas where vegetation density has increased from no/low density to mild or high are shown in green color (+ve change). The negative changes are widespread all over the study area, occupying a total surface of 600 km2 except for 2015 (1200 km2) and 2017 (800 km2), with higher concentration in the center of Delta, whereas positive changes are concentrated in the Delta borders.
According to the analysis of MODIS NDVI in the month of January between 2013 and 2017 for each of the 11 Delta governorates, it has been observed that there is a general increase of negative changes especially in the “Beheira” Governorate where negative affected area increased from 94 to 291 km2. Looking at the geographic distribution of the regions, Al Sharqia, Beheira, Gharbia, Kafer Elshikh and Qalyubia represent the most affected governorates in the East, West, Central, North and South Delta respectively, and they could thus be defined as hot spot areas for further investigations in the Nile Delta domain and identified as the regions with the highest priority for the management needs.
Applying the same analysis to July and September, which are the Nile summer and mid-season months, it has been found that the degraded (-ve change) regions are located in the heart of Delta, especially in September and during the period 2014–2016. The maps of the results for these months are detailed in Appendix II.
This situation can be attributed more to modified agronomic practices than to climate change, as, for instance, the overlapping seeding dates in these seasons, the changes in crop farming and the decision of some farmers to extend the main agricultural season in the wintertime. Hence, it has been decided to rely on the January examination results only to define the priority areas in Egypt, which have been identified as Sharqia, Beheira, Gharbia, Kafer Elshikh and Qalyubia Governorates.
EVI temporal trends
A long-term analysis of EVI has been carried out over the three test areas by considering the period between 2001 and 2018 and separating rainy and dry seasons. The temporal evolution of monthly EVI mean anomalies, averaged for the entire regions, is represented in .
Figure 7. EVI mean anomalies, for dry (brown bars) and rainy (green bars) seasons, in the period 2001–2018 for: a) Nile Delta, b) Merguellil basin and c) olive trees fields in Medenine.

The agricultural fields at MODIS subpixel scale have been discarded from the analysis to avoid biases in the drought mapping.
The annual fluctuations are evident, with marked negative trends in the first part of 2000s for all the three areas, especially in Medenine and Merguellil basins, due to the significant drought event that impacted Tunisia during that period. In the Nile Delta, the trend is less pronounced since the irrigation of agricultural crops smoothed the yearly fluctuations. The most recent years seem to be characterized by positive trends of EVI.
The patterns observed for the three areas in revealed that the years 2001–2005 and 2010 have been severely affected by negative anomalies, both in rainy and dry seasons. In particular, the years 2002 and 2010 represent the highest negative crop conditions, which are also correlated to the percentage of pixels (10% in 2001 and 11% in 2010) with EVI values smaller than the double of the standard deviation (μ-2σ). The regions of Merguellil () and Medenine () presented similar negative trends in 2002 and especially in 2001, when the percentage of pixels having EVI values lower than (μ-2σ) is 36% and 6%, respectively. Unlike the Nile Delta, the regions of Merguellil and Medenine presented significant negative anomalies in 2012, when EVI standard deviation values reached respectively −0.13 and −0.15 in the rainy season. On the other hand, all the regions in the years 2017 and 2018 revealed higher values compared both with the long-term standard deviation and for the number of pixels having a value higher than μ + 2σ. To analyze more in-depth the drought spatial patterns, mean ET values (annual, dry and rainy season) was estimated for the year 2018 () on the Nile Delta region and in the Merguellil basin.
Figure 8. Mean ET values in 2018: a) annual, b) dry season and c) rainy season. On the left column, the Nile Delta region and on the right column, the Merguellil basin.

shows the mean annual ET values (a) estimated from MODIS in 2018 on the Nile Delta region and in Merguellil basin, along with the mean ET values for the dry (b) and rainy seasons (c).
The test area of Medenine, due to the very arid environment of the region, shows only few scattered ET values and it has not therefore been shown. As it has been already noted for the previous analysis on Nile Delta region, the presence of irrigation practices of agricultural crops smoothed the differences between dry and rainy seasons, although, especially in the central and western part of the region, there is a decrease of ET during the dry season. The patterns are clearer in the Merguellil basin, where ET significantly increases during the wet season, mainly in the central area of the basin.
The most interesting monthly EVI anomalies are represented in for Delta Nile, for Medenine and for Merguellil: the years on display for each study region have been selected based on the exceptional conditions of drought or moisture.
Figure 9. Monthly vegetation MODIS EVI anomalies over selected months in the Nile Delta pointing out droughts in red tones (2003; 2004) and water abundance in green tones (2012; 2018).

Figure 10. Monthly vegetation MODIS EVI anomalies over selected months in the Medenine area in evidence of droughts (2001; 2010) and water abundance (2011; 2018).

Figure 11. Monthly vegetation MODIS EVI anomalies over selected months in the Merguellil basin in evidence of droughts (2001; 2008) and water abundance (2014; 2017).

shows the maps of monthly vegetation MODIS EVI anomalies in the Nile Delta for the years 2003 and 2004 (drought condition with strong negative trend on vegetative conditions) as well as in 2012 and 2018 (very humid periods having an overall positive trend with higher values from October to December in 2018). As already pointed out by the results in the previous sections, there is a dynamic related to the season and to the annual variations, with anomalies particularly evident in spring and summer.
The results obtained on the test areas in Tunisia are shown in , for Medenine and Merguellil basin, respectively. Differently from Nile Delta, in Merguellil basin the monthly vegetation MODIS EVI negative anomalies are concentrated between February and April, whereas in Medenine only positive anomalies can be observed, probably due to the higher rainfall recorded in the area during 2017–2018 (very wet years).
A detailed analysis of the spatial condition in Medenine () shows a severe EVI negative anomaly in olive plantations for the years 2001, 2010 and 2011. However, a slight recovering is presented between November and December 2011. As for Medenine, the Merguellil region () suggests severe negative vegetation condition anomalies in 2001 when both regions presented high vegetation stress between April and May while in 2017/2018 EVI anomalies are positive for the same period of the year.
Additional investigations on agricultural drought in the Nile Delta have been carried out using VHI derived from MODIS Terra sensor. The index has been calculated for the period October–May from 2000 to 2017. The results show severe drought conditions in the seasons 2009–2010 and 2010–2011, when most of the area was in the “High drought” category. Conversely, in the season 2006–2007 most of the area was in the “No drought” category, although some “High drought” subareas can be detected in this season too. Among all maps, the western and eastern parts seem to be more vulnerable to drought than other parts (see, ).
A more detailed description of the trends for the three target areas is reported in Appendix II, where Nile Delta NDVI maps for the months of July and September, as well as MODIS EVI maps of temporal anomalies on the three test sites in the four selected years, are shown. VHI annual series from 2000 to 2017 are also included in Appendix II for a more complete understanding of the described trends.
Soil moisture maps
The averaged SMC maps obtained for the Nile Delta test area are shown in . The maps clearly show the SMC dynamics, with high values in fall and winter and generally wetter conditions than the rest of the Egypt along the entire year. The SMC conditions reflect the mean ET values trends for dry and wet seasons in the same year as shown in .
Figure 13. Monthly averaged maps of SMC (%) for the Nile Delta test area obtained from the S-1 SAR images by using the IFAC ANN retrieval algorithm for 2018.

The same methodology, applied to the two test areas in Tunisia, pointed out drier conditions, with SMC values that do not exceed 15%, and limited seasonal variations, slightly more pronounced for the Medenine test area (), which is close to the Mediterranean Sea and affected by its mitigating influence on the climate. Even drier conditions have been found in the Merguellil basin, which is characterized by very low SMC values with small seasonal variations (). For this area, S-1 images of August 2017 are missing.
Figure 14. Monthly averaged maps of SMC (%) for the Medenine test area obtained from the S-1 SAR images by using the IFAC ANN retrieval algorithm in 2018.

Figure 15. Monthly averaged maps of SMC (%) in the Merguellil test area obtained from the S1 SAR images by using the IFAC ANN retrieval algorithm for 2018.

Figure 19. Monthly MODIS EVI, NDVI and ET in 2018 for the three areas: Merguellil (a), Medenine (b) and Nile Delta (c). In the same diagram, averaged values of SMC and CDSI, estimated using the IFAC ANN algorithms are shown.

CDSI maps
The results shown in the previous sections demonstrated the peculiar sensitivities of microwave and optical parameters to ET and drought conditions of soil and vegetation, confirming the effectiveness of this index, which is capable of taking advantage of both the observation techniques. The maps of CDSI for a sub-area of the Nile Delta, which has been chosen to enhance differences in seasonal trends for irrigated agricultural zones, are shown in . The values of CDSI range from 0 (drought conditions) to 1 (humid conditions). In the maps CDSI was subdivided (see ) in five classes, ranging from very low to very high, describing the drought conditions of the area.
Figure 16. CDSI maps generated for Nile Delta from March to July 2018, for which SMC and VSDI data are available.

An overall drought severity from medium to high is evident. The peak values of CDSI found in May 2018 correspond to heavy rainfalls in the area, whose effects were captured by SMC but not by VSDI, thus confirming the effectiveness of the proposed synergy. Irrigation practices can instead justify the moister conditions in July, which are pointed out by high values of CDSI, despite the dry season.
The CDSI maps for the Medenine test area are shown in whose boundaries have been defined to include rainfed agricultural fields as well as a portion of “sebkha”, a flat sandy area with the presence of salt on the surface.
The overall drought severity, identified by the CDSI maps of , ranged between high and very high, and it is well described by both SMC and VDSI. Slightly moister conditions are recorded in February, corresponding to an increase in precipitation rate. The very high severity of drought conditions on the right side of the maps corresponds to the above-mentioned semi-desertic region with sandy soils.
The CDSI maps for the Merguellil basin are shown in : maps refer to the September 2017–July 2018 period. The analyzed portion of land has been identified to contain both irrigated and rainfed agricultural fields and strengthen differences in CDSI behavior.
The seasonal vegetation cycle is correctly reproduced by CDSI maps that point out a progressive decrease of drought severity from September to February and an increase from February to July. The two patterns of lower drought severity, which can be identified in most of the images, correspond to agricultural areas. The VDSI contribution was dominant for identifying these patterns, while SMC showed lower variability along the area.
Time-series comparison
To give a better insight on the relationships among weather conditions, SMC and vegetation indices, the monthly averaged time series of SMC, ET, EVI, NDVI and CDSI have been plotted along with the precipitation rate for the three test areas in , where NDVI, EVI and CDSI were multiplied by 100 to facilitate their visualization. This comparison was necessary to highlight the individual capabilities of different indices in characterizing the soil and vegetation features and to give a provisional validation among the different methods, considering the scarce availability of ground data.
points out a general agreement between ET and precipitation, except in the Nile Delta area, where the presence of irrigation of agricultural fields allows an increase of ET despite the absence of rainfall in summertime. The same trend can be noted also for the SMC behavior in the Nile Delta. In Tunisia, SMC is very low (especially in the Medenine site, being always <5%); however, in the Merguellil area, it well follows both precipitation and ET and CDSI trends. EVI and NDVI follow the winter crop cycle with rather scarce oscillations which is typical of semi-arid zones. Divergences among SMC, CDSI and rainfall can be attributed to the different areas involved in the analysis and the distance of the meteorological stations from the test area. It is worthy to notice that the dedicated training made the ANN algorithm capable of estimating also the very low moisture conditions of Tunisian areas, which are often around the 5% threshold, that represent a lower limit for the microwave sensitivity to the moisture conditions of the observed target (Dobson et al., Citation1985).
The increase in ET in Merguellil area, started in the middle of February, is concomitant with the winter crop growth which is pinpointed by the rise of NDVI and EVI while in Medenine area the ET follows the major change in precipitation as expected in rainfed condition. However, NDVI and EVI do not show any correlation to the precipitation pattern, which can be explained by regional rainfall that affects only small areas, thus negatively affecting the overall correlation. The low SMC values in Medenine can be related to small precipitation intensities that dry off quickly from the topsoil and does not show at a monthly level.
The decrease in precipitation after the middle of May, highlighted by the S-1 SMC product, led to a drop in ET due to both vegetation senescence and drying soil.
In Nile Delta ET, NDVI and EVI trends are in agreement to each other: in the middle of May a minimum of ET is pinpointed, highlighting the period of the year with the lowest vegetation coverage (harvest season). Increase in the ET, SMC, CDSI, NDVI and EVI values, noticeable after May, confirms a growing up of vegetation, which was made possible by irrigation since high values of SMC and CDSI are influenced by these agricultural practices.
Discussion
This work, which has been carried out in the framework of the ERANET-MED OptiMed-Water project, aimed at providing new methods based on remote sensing for the identification of water stress condition of soil and vegetation, in order to improve the irrigation management and reduce the water wastes (to be used as support for decision-makers). The research exploited the synergy of remote sensing techniques, based on both optical and microwave satellite data, for investigating the water cycle in arid and semi-aird areas of the Mediterranean basin and providing timely information on drought and vegetation stress conditions.
The effectiveness of the MODIS derived NDVI, EVI and ET for monitoring the vegetation conditions was already pointed out by previous studies (Chen et al., Citation2018; Wardlow & Egbert, Citation2010), as well as the S-1 capability in observing SMC at several resolution scales (Gao et al., Citation2017; Hornáček et al., Citation2012), including semi-arid areas (Hachani et al., Citation2019). The analysis carried out on each of the NDVI, EVI and ET indices, as well as the SMC, confirmed the good correlation of each of them with the corresponding reference data by evidencing interesting long-term behaviors. Furthermore, the most important outcome of this study consisted of demonstrating the effectiveness of the optical/microwave data synergy for monitoring the overall drought conditions in the observed areas, being the optical indices good descriptors of the vegetation status and the SMC derived from S-1 data well representative of the soil conditions. In this respect, a new combined optical and microwave drought severity index (CDSI) has been proposed. Taking advantage of the optical and microwave peculiar sensitivities, CDSI was able to capture the drought conditions that were encountered in the Tunisia test areas and the large variation of surface and vegetation water content that characterized the Nile Delta test area. This combined index can thus represent a suitable basis for setting up a prediction system, jointly based on optical and MW data, that will be the natural prosecution of this activity.
Some considerations should be also drawn about remote sensing and in situ data availability: first, the procurement of the satellite data required by this methodology is not an issue, given their free availability through dedicated portals. However, better availability of reference data from in-situ stations would be advisable to improve both algorithm calibration and result validation.
Concerning the temporal resolution, the almost daily revisiting of MODIS ensured a very frequent coverage of the areas, which are also scarcely affected by the data unavailability due to adverse weather. Although characterized by a slower revisiting, S-1 was in any case able to cover the areas at least every 6 days, independently on the weather conditions: such revisiting can be still sufficient, given the limited precipitation rate and the consequent small SMC dynamics. In Nile Delta, for instance, the revisiting was enough to capture the weather conditions associated with meteo alerts that the optical indices were not able to observe. In any case, the SAR temporal coverage could be improved by considering images acquired by S-1 under different geometries or data from another SAR mission: given the number of recently launched or incoming SAR satellite missions, the availability of data is indeed quickly increasing. Moreover, in some cases the new satellites are equipped with an instrument operating at L-band (e.g. ALOS2-3 and Rose-L (Suzuki, Citation2020)), which is even more appealing for the SMC monitoring, due to the higher penetration and smaller sensitivity to low vegetation. In this case, the SMC algorithm proposed here, being based on machine learning, can be easily adapted to data from different SAR sensors, thus significantly improving the temporal coverage (and possibly the accuracy) of SMC mapping.
The third aspect that should be considered deals with the spatial resolution required for this application enhancing the S-1 SAR data suitable for crop monitoring at farm scale too, since the SMC mapping can be obtained at 10–20 m resolution. Conversely, the optical indices derived from MODIS, which has a spatial resolution of 250 m, could not be sufficient for monitoring small agricultural fields. In the case of small-scale applications, a higher resolution sensor, as e.g. Landsat or Sentinel-2, should be considered, although significant changes in the vegetation conditions at MODIS subpixel scale are quite unlikely.
Conclusions
The performed analysis, which was carried out on different test areas for a period of several years, confirmed a good sensitivity of the remote sensing observations to the vegetation and the soil moisture conditions and an overall agreement between optical and microwave observations.
In detail, the NDVI and ET anomalies computed for a 4–18-year period from MODIS images showed a negative trend in vegetation cover, for the Nile Delta area, confirming the increase in drought conditions over time. The regions of Merguellil and Medenine exhibited similar negative trends, showing important negative anomalies of EVI.
The soil conditions, which have been described by the SMC values derived from the available S-1 images, are in agreement with the vegetation water status. The latter was pointed out by the optical indices, confirming the different aridity conditions of the areas: the Nile Delta showed significant seasonal fluctuations whereas the Tunisian sites were characterized by smaller SMC fluctuations in a very limited range (often <10%).
The remarked discrepancies between SMC and optical indices depend on the peculiar sensitivities of the two observation techniques to different physical properties of the surface.
Thus, optical and microwave observations have been exploited to develop a drought severity combined index (CDSI) that was capable of successfully capturing the drought patterns in the observed areas. A further comparison between NDVI, ET, EVI, SMC, CDSI and rainfall confirmed the agreement between satellite observation and reference ground data.
In summary, by exploiting the synergy between optical and microwave satellite data, it has been possible to highlight in a more accurate way the spatial and temporal variations in soil moisture and vegetation conditions over the years. A general trend toward increasing drought conditions has been noted, albeit with oscillations during the analyzed periods.
A future development of this research will focus on the implementation of a methodology based on the integration between optical and microwave indices for predicting soil and vegetation drought conditions. The proposed method could furthermore provide precise indications on the irrigation strategies and water waste solutions, with the aim of mitigating the effects of global changes.
Disclosure statement
No potential conflict of interest was reported by the author(s).
Data availability statement
Data are not going to be shared publicly. The data are currenlty stored in this FTP folder ftpes://[email protected]/SantiFTP/ that can be accessed only if login credentials are provided by IFAC responible.
Correction Statement
This article has been corrected with minor changes. These changes do not impact the academic content of the article.
References
- Abdel-Kader, F. H. (2019). Assessment and monitoring of land degradation in the northwest coast region, Egypt using Earth observations data. Egyptian Journal of Remote Sensing and Space Science, 22(2), 2. https://doi.org/10.1016/j.ejrs.2018.02.001
- Ahlström, A., Raupach, M. R., Schurgers, G., Smith, B., Arneth, A., Jung, M., Reichstein, M., Canadell, J. G., Friedlingstein, P., Jain, A. K., Kato, E., Poulter, B., Sitch, S., Stocker, B. D., Viovy, N., Wang, Y. P., Wiltshire, A., Zaehle, S., & Zeng, N. (2015). The dominant role of semi-arid ecosystems in the trend and variability of the land CO 2 sink. Science, 348(6237), 6237. https://doi.org/10.1126/science.aaa1668
- Akinyemi, F. O. (2021). Vegetation trends, drought severity and land use‐land cover change during the growing season in semi‐arid contexts. Remote Sensing, 13(5), 836. https://doi.org/10.3390/rs13050836
- Allam, M., Bakr, N., & Elbably, W. (2019). Multi-Temporal assessment of land use/land cover change in arid region based on landsat satellite imagery: Case Study in Fayoum Region, Egypt. Remote Sensing Applications: Society and Environment, 14. https://doi.org/10.1016/j.rsase.2019.02.002
- Anna, B., Mattia, F., Satalino, G., & Davidson, M. W. J. (2011). Dense temporal series of C- and L-band SAR data for soil moisture retrieval over agricultural crops. IEEE Journal of Selected Topics in Applied Earth Observations and Remote Sensing, 4(2). https://doi.org/10.1109/JSTARS.2010.2052916
- Attema, E. P. W., & Fawwaz, T. U. (1978). Vegetation modeled as a water cloud. Radio Science, 13(2), 2. https://doi.org/10.1029/RS013i002p00357
- Baghdadi, N., Gaultier, S., & King, C. (2002). Retrieving surface roughness and soil moisture from synthetic aperture radar (SAR) data using neural networks. Canadian Journal of Remote Sensing, 28(5), 701–23. https://doi.org/10.5589/m02-066
- Bastiaanssen, W. G. M., Noordman, E. J. M., Pelgrum, H., Davids, G., Thoreson, B. P., & Allen, R. G. (2005). SEBAL Model with remotely sensed dta to improve water-resources management under actual field conditions. Journal of Irrigation and Drainage Engineering, 131(1), 1. https://doi.org/10.1061/(asce)0733-9437
- Bhaskar, R. N. (2014). Comparative evaluation of different potential evapotranspiration estimation approaches. International Journal of Research in Engineering and Technology, 03(6), 544–552. https://doi.org/10.15623/ijret.2014.0306102
- Boulet, G., Delogu, E., Chebbi, W., Rafi, Z., Le Dantec, V., Mallick, K., Mougenot, B., Olioso, A., Zribi, M., Lili-Chabaane, Z., Er-Raki, S., Merlin O. (2019). Evapotranspiration and evaporation/transpiration retrieval using dual-source surface energy balance models integrating VIS/NIR/TIR data with satellite surface soil moisture information. International Archives of the Photogrammetry, Remote Sensing and Spatial Information Sciences - ISPRS Archives, 42. https://doi.org/10.5194/isprs-archives-XLII-3-W6-9-2019.
- Chen, Y., Dengsheng, L., Moran, E., Batistella, M., Vieira Dutra, L., Del Arco Sanches, I., Bicudo da Silva, R. F., Huang, J., José Barreto Luiz, A., & Falcão de Oliveira, M. A. (2018). Mapping croplands, cropping patterns, and crop types using MODIS time-series data. International Journal of Applied Earth Observation and Geoinformation, 69. https://doi.org/10.1016/j.jag.2018.03.005
- de Jong, R., de Bruin, S., de Wit, A., Schaepman, M. E., & Dent, D. L. (2011). Analysis of monotonic greening and browning trends from global NDVI time-series. Remote Sensing of Environment, 115(2),692–702. Elsevier. https://doi.org/10.1016/J.RSE.2010.10.011
- Del Frate, F., Ferrazzoli, P., Schiavon, G., Ahlström, A., Raupach, M. R., Schurgers, G., Smith, B., Arneth, A., Jung, M., Reichstein, M., Canadell, J. G., Friedlingstein, P., Jain, A. K., Kato, E., Poulter, B., Sitch, S., Stocker, B. D., Viovy, N., Wang, Y. P., … Zeng, N. (2003). Retrieving Soil moisture and agricultural variables by microwave radiometry using neural networks. Remote Sensing of Environment, 84(2), 174–183. https://doi.org/10.1016/S0034-4257(02)00105-0
- Dobson, M. C., Ulaby, F. T., Hallikainen, M. T., & El-Rayes, M. A. (1985). Microwave dielectric behavior of wet soil-Part II: Dielectric mixing models. IEEE Transactions on Geoscience and Remote SensingGE-, 23, 1. https://doi.org/10.1109/TGRS.1985.289498
- Ekundayo, O. Y., Okogbue, E. C., Akinluyi, F. O., Kalumba, A. M., & Orimoloye, I. R. (2021). Spatiotemporal drought assessment using vegetation health index and standardized precipitation index over Sudano-Sahelian region of Nigeria. African Geographical Review, 40(4), 4. https://doi.org/10.1080/19376812.2020.1841658
- El-Shirbeny, Alsersy, A., Saleh, N. H., Abu-Taleb, K. A., & El-Shirbeny, M. A. (2015). Changes in irrigation water consumption in the Nile Delta of Egypt assessed by remote sensing. Arabian Journal of Geosciences, 8(12), 12. https://doi.org/10.1007/s12517-015-2005-2
- Fayech, D., & Tarhouni, J. (2021). Climate variability and its effect on normalized difference vegetation index (NDVI) using remote sensing in semi-arid Area. Modeling Earth Systems and Environment, 7(3), 3. https://doi.org/10.1007/s40808-020-00896-6
- Fishar, M. R. (2018). Nile Delta (Egypt). In The Wetland Book II: Distribution, Description, and Conservation The Wetland Book. Springer (Vol. 2, pp. 1251–1260). Dordrecht: Springer Nature. https://doi.org/10.1007/978-94-007-4001-3_216
- Fung, A. K. (1994. . Microwave Scattering and Emission Models and Their Applications (Artech House Publishers) 0890065233 .
- Gao, Q., Zribi, M., Jose Escorihuela, M., & Baghdadi, N. (2017). Synergetic use of Sentinel-1 and Sentinel-2 data for soil moisture mapping at 100 m resolution. Sensors (Switzerland), 17(9), 1966. https://doi.org/10.3390/s17091966
- Gidey, E., Dikinya, O., Sebego, R., Segosebe, E., & Zenebe, A. (2018). Modeling the spatio-temporal meteorological drought characteristics using the standardized precipitation index (SPI) in Raya and its environs, Northern Ethiopia. Earth Systems and Environment, 2(2). https://doi.org/10.1007/s41748-018-0057-7
- Giorgi, F., & Lionello, P. (2008). Climate change projections for the Mediterranean region. Global and Planetary Change, 63(2–3), 2–3. https://doi.org/10.1016/j.gloplacha.2007.09.005
- Hachani, A., Ouessar, M., Paloscia, S., Santi, E., & Pettinato, S. (2019). Soil moisture retrieval from Sentinel-1 acquisitions in an arid environment in Tunisia: Application of artificial neural networks techniques. International Journal of Remote Sensing, 40(24), 24. https://doi.org/10.1080/01431161.2019.1629503
- Hamza, W. 2006. The Nile Estuary. Handbook of Environmental Chemistry, Volume 5, Water Pollution 5 (PART H). https://doi.org/10.1007/698-5-025
- Hesham, Y.H., Khalil, A.A., M. El-Sayed, M. (2015). Atlas for Drought Monitoring over Egypt 978-977-302-445-1 . https://www.academia.edu/17344925/ATLAS_FOR_DROUGHT_MONITORING_OVER_EGYPT
- Hornáček, M., Wagner, W., Sabel, D., Linh Truong, H., Snoeij, P., Hahmann, T., Diedrich, E., & Doubková, M. (2012). Potential for high resolution systematic global surface soil moisture retrieval via change detection using Sentinel-1. IEEE Journal of Selected Topics in Applied Earth Observations and Remote Sensing, 5(4), 4. https://doi.org/10.1109/JSTARS.2012.2190136
- Huete, A., Didan, K., Miura, T., Rodriguez, E. P., Gao, X., & Ferreira, L. G. (2002). Overview of the radiometric and biophysical performance of the MODIS vegetation indices. Remote Sensing of Environment, 83(1–2), 1–2. https://doi.org/10.1016/S0034-4257(02)00096-2
- IPCC. (2019). Climate Change, Desertification, Land Degradation, Sustainable Land Management, Food Security, and Greenhouse gas fluxes in Terrestrial Ecosystems. IPCC. https://www.ipcc.ch/site/assets/uploads/2019/11/SRCCL-Full-Report-Compiled-191128.pdf.
- Jaafar, H. H., & Ahmad, F. A. (2015). Crop yield prediction from remotely sensed vegetation indices and primary productivity in arid and semi-arid lands. International Journal of Remote Sensing, 36(18), 18. https://doi.org/10.1080/01431161.2015.1084434
- Javzandulam, T., Tateishi, R., & Sanjaa, T. (2005). Analysis of vegetation indices for monitoring vegetation degradation in semi-arid and arid areas of Mongolia. International Journal of Environmental Studies, 62(2), 2. https://doi.org/10.1080/00207230500034123
- JICA, Yachiyo Engineering Co., Kaihatsu Management Consulting, and Corporation Ingérosec. 2015. “Project on Regional Development Planning of the Southern Region in the Republic of Tunisia Final Report Project on Regional Development Planning of the Southern Region in the Republic of Tunisia Final Report”, no. Mdici. https://openjicareport.jica.go.jp/pdf/12246732.pdf.
- John, R., Chen, J., Nan, L., Guo, K., Liang, C., Wei, Y., Noormets, A., Keping, M., & Han, X. (2008). Predicting plant diversity based on remote sensing products in the semi-arid region of inner Mongolia. Remote Sensing of Environment, 112(5), 2018–2032. https://doi.org/10.1016/j.rse.2007.09.013
- Justice, C. O., Vermote, E., Townshend, J. R. G., Defries, R., Roy, D. P., Hall, D. K., Salomonson, V. V., Privette, J. L., Riggs, G., Strahler, A., Lucht, W., Myneni, R. B., Knyazikhin, Y., Running, S. W., Nemani, R. R., Wan, Z., Huete, A. R., van Leeuwen, W., Wolfe, R. E., … Barnsley, M. J. (1998). The moderate resolution imaging spectroradiometer (MODIS): Land remote sensing for global change research. IEEE Transactions on Geoscience and Remote Sensing, 36(4), 4. https://doi.org/10.1109/36.701075
- Khan, M. S., Baik, J., & Choi, M. (2020). Inter-Comparison of evapotranspiration datasets over heterogeneous landscapes across Australia. Advances in Space Research, 66(3), 3. https://doi.org/10.1016/j.asr.2020.04.037
- Kogan, F. N. (1995). Application of vegetation index and brightness temperature for drought detection. Advances in Space Research, 15(11), 91–100. https://doi.org/10.1016/0273-1177(95)00079-T
- Kogan, F. N. (2001). Operational space technology for global vegetation assessment. Bulletin of the American Meteorological Society, 82(9), 1949–1964. https://doi.org/10.1175/1520-0477(2001)082<1949:OSTFGV>2.3.CO;2
- Kottek, M., Grieser, J., Beck, C., Rudolf, B., & Rubel, F. (2006). World Map of the Köppen-Geiger Climate Classification Updated. Meteorologische Zeitschrift, 15(3), 3. https://doi.org/10.1127/0941–2948/2006/0130
- Lal, R. (2004 Carbon Sequestration in Dryland Ecosystems Environmental Management). . In (Vol. 33 4 , pp. 528–544). . http://link.springer.com/10.1007/s00267-003-9110-9 doi:10.1007/s00267-003-9110-9
- Lionello, P., & Scarascia, L. (2018). The relation between climate change in the Mediterranean region and global warming. Regional Environmental Change, 18(5), 5. https://doi.org/10.1007/s10113-018-1290-1
- Mckee, T. B. T. B., Doesken, N. J. N. J., Kleist, J., Doesken, N. J. N. J., Kleist, J., Mckee, T. B. T. B., Nolan J. N.J, D., & Kleist, J. 1993. “The relationship of drought frequency and duration to time scales. Eighth Conference on Applied Climatology. American Meteorological Society, Boston”. Eighth Conference on Applied Climatology, no. 1.
- MedECC. (2020). Climate and environmental change in the Mediterranean basin – current situation and risks for the future. First Mediterranean assessment report. Climate and Environmental Change in the Mediterranean Basin – Current Situation and Risks for the Future (pp.632). ISBN 978-2-9577416-0-1; doi: 10.5281/zenodo.4768833
- Mingzhu, H., Kimball, J. S., Yonghong, Y., Running, S. W., Guan, K., Moreno, A., Xiaocui, W., & Maneta, M. (2019). Satellite data-driven modeling of field scale evapotranspiration in croplands using the MOD16 algorithm framework. Remote Sensing of Environment, 230. https://doi.org/10.1016/j.rse.2019.05.020
- Ning, Z., Hong, Y., Qin, Q., & Liu, L. (2013). VSDI: A visible and shortwave infrared drought index for monitoring soil and vegetation moisture based on optical remote sensing. International Journal of Remote Sensing, 34, 13. https://doi.org/10.1080/01431161.2013.779046
- Olmos-Trujillo, E., González-Trinidad, J., Júnez-Ferreira, H., Pacheco-Guerrero, A., Bautista-Capetillo, C., Avila-Sandoval, C., & Galván-Tejada, E. (2020). Spatio-temporal response of vegetation indices to rainfall and temperature in a semiarid region. Sustainability (Switzerland), 12(5). https://doi.org/10.3390/su12051939
- Paloscia, S., Pettinato, S., Santi, E., Notarnicola, C., Pasolli, L., & Reppucci, A. (2013). Soil moisture mapping using Sentinel-1 images: algorithm and preliminary validation. Remote Sensing of Environment, 134. https://doi.org/10.1016/j.rse.2013.02.027
- Qiaozhen, M., Zhao, M., & Running, S. W. (2011). Improvements to a MODIS global terrestrial evapotranspiration algorithm. Remote Sensing of Environment, 115, 8. https://doi.org/10.1016/j.rse.2011.02.019
- Rodríguez-Fernández, N. J., Aires, F., Richaume, P., Kerr, Y. H., Prigent, C., Kolassa, J., Cabot, F., Jiménez, C., Mahmoodi, A., & Drusch, M. (2015). Soil moisture retrieval using neural networks: Application to SMOS. IEEE Transactions on Geoscience and Remote Sensing, 53(11), 5991–6007. https://doi.org/10.1109/TGRS.2015.2430845
- Roy, D. P., Wulder, M. A., Loveland, T. R., Woodcock, C. E., Allen, R. G., Anderson, M. C., Helder, D., Irons, J. R., Johnson, D. M., Kennedy, R., Scambos, T. A., Schaaf, C. B., Schott, J. R., Sheng, Y., Vermote, E. F., Belward, A. S., Bindschadler, R., Cohen, W. B., Gao, F., … Zhu, Z. (2014). Landsat-8: Science and product vision for terrestrial global change research. Remote Sensing of Environment, 145, 154–172. https://doi.org/10.1016/j.rse.2014.02.001
- Ryan, J. G., & Spencer, D. C. (2001). Future challenges and opportunities for agricultural development in the semi-arid tropics. Patanchura, 502, 324 http://oar.icrisat.org/id/eprint/1107.
- Santi, E. (2016). Neural networks applications for the remote sensing of hydrological parameters. Artificial Neural Networks - Models and Applications. https://doi.org/10.5772/63165
- Santi, E., Paloscia, S., Pettinato, S., Brocca, L., & Ciabatta, L. (2016). Robust assessment of an operational algorithm for the retrieval of soil moisture from AMSR-E data in central Italy. IEEE Journal of Selected Topics in Applied Earth Observations and Remote Sensing, 9(6), 6. https://doi.org/10.1109/JSTARS.2016.2575361
- Santi, E., Paloscia, S., Pettinato, S., & Fontanelli, G. (2016). Application of artificial neural networks for the soil moisture retrieval from active and passive microwave spaceborne sensors. International Journal of Applied Earth Observation and Geoinformation, 48. https://doi.org/10.1016/j.jag.2015.08.002
- Santi, E., Pettinato, S., Paloscia, S., Pampaloni, P., MacElloni, G., & Brogioni, M. (2012). An algorithm for generating soil moisture and snow depth maps from microwave spaceborne radiometers: HydroAlgo. Hydrology and Earth System Sciences, 16(10), 10. https://doi.org/10.5194/hess-16-3659-2012
- Senay, G.B., Velpuri, N.M., Bohms, S., Budde, M., Young, C., Rowland, J., Verdin, J.P. (2015). Drought Monitoring and Assessment: Remote Sensing and Modeling Approaches for the Famine Early Warning Systems Network. In Shroder, John F., Di Baldassarre, Giuliano, Paron, Paolo (Eds.), Hydro-Meteorological Hazards, Risks and Disasters (Elsevier) 233–262. ISBN: 9780123948465. https://doi.org/10.1016/B978-0-12-394846-5.00009-6
- Sommer, R., Ryan, J., Masri, S., Singh, M., & Diekmann, J. (2011). Effect of shallow tillage, moldboard plowing, straw management and compost addition on soil organic matter and nitrogen in a dryland barley/wheat-vetch rotation. Soil and Tillage Research, 115–116. https://doi.org/10.1016/j.still.2011.06.003
- Sun, D., & Kafatos, M. (2007). Note on the NDVI-LST relationship and the use of temperature-related drought Indices over North America. Geophysical Research Letters, 34(24), 24. https://doi.org/10.1029/2007GL031485
- Suzuki. 2020. “Contract signed for new copernicus ROSE-L Mission.” https://www.esa.int/Applications/Observing_the_Earth/Copernicus/Contract_signed_for_new_Copernicus_ROSE-L_mission
- Tarpanelli, A., Santi, E., Tourian, M. J., Filippucci, P., Amarnath, G., & Brocca, L. (2019). Daily river discharge estimates by merging satellite optical sensors and radar altimetry through artificial neural network. IEEE Transactions on Geoscience and Remote Sensing, 57(1), 1. https://doi.org/10.1109/TGRS.2018.2854625
- Tramblay, Y., Koutroulis, A., Samaniego, L., Vicente-Serrano, S. M., Volaire, F., Boone, A., Le Page, M., Llasat, M.C., Albergel, C., Burak, S., Cailleret, M., Cindrić Kalin, K., Davi, H., Dupuy, J., Greve, P., Grillakis, M. et al. (2020). Challenges for drought assessment in the mediterranean region under future climate scenarios. Earth-Science Reviews, https://doi.org/10.1016/j.earscirev.2020.103348
- Wardlow, B. D., & Egbert, S. L. (2010). A Comparison of MODIS 250-m EVI and NDVI data for crop mapping: A case study for Southwest Kansas. International Journal of Remote Sensing, 31(3), 3. https://doi.org/10.1080/01431160902897858
- Xie, C., Zhang, X., Zhuang, L., Zhu, R., & Guo, J. (2022). . In Scientific Reports, Vol.). Nature Publishing Group UK. . https://doi.org/10.1038/s41598-022-06363-9.
- Yuan, L., Yaoming, M., Chen, X., Wang, Y., & Zhaoguo, L. (2021). An enhanced MOD16 evapotranspiration model for the Tibetan Plateau during the unfrozen season. Journal of Geophysical Research: Atmospheres, 126(7). https://doi.org/10.1029/2020JD032787
- Zhu, X., Liu, Y., Kun, X., & Pan, Y. (2021). Effects of drought on vegetation productivity of farmland ecosystems in the drylands of northern China. Remote Sensing, 13(6). https://doi.org/10.3390/rs13061179
- Zribi, M., Baghdadi, N., Bousbih, S., El-Hajj, M., & Gao, Q. (2019). Surface moisture and irrigation mapping at agricultural field scale using the synergy Sentinel-1/Sentinel-2 Data. International Archives of the Photogrammetry, Remote Sensing and Spatial Information Sciences - ISPRS Archives, 42. https://doi.org/10.5194/isprs-archives-XLII-3-W6-357-2019