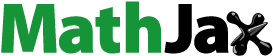
ABSTRACT
There is no easy in situ way to monitor large waterbodies for their aquatic vegetation change, especially during mowing works. The objective of this study is to choose the best automatic workflow that would estimate a change in the reed bed area and density over time. This workflow will assess the mowing effect on reeds over 3 years in the Plateliai Lake (Lithuania). Sentinel-2/MSI images were used to derive reed beds using water adjusted vegetation index (WAVI) and normalised difference water index (NDWI). The indices were classified using seven different binary thresholding algorithms. Results were validated with orthophotos gathered from unmanned aerial vehicle surveys in mowed regions and one reference area. Analysis demonstrated that using the NDWI together with the Yen thresholding algorithm generated the best accuracy results, with the highest accuracy resulting with high vegetation areas where the area under the curve values were 0.85 ± 0.17. The changes in estimated density did not show a significant correlation between mowed and reference areas and years. The results indicate that Sentinel-2/MSI is a feasible tool for the evaluation of reed bed change. On this basis, it is recommended to implement it as an additional monitoring tool that covers larger areas than in situ monitoring.
Introduction
One of the most common invasive species in inland waterbodies is considered a common reed (Phragmites australis), which is an easily grown plant that thrives in deep moisture-retentive soils (Duke, Citation1983). Due to its ability to acclimatise, a common reed has spread all over Europe, Northern America, Asia and Australia, as well as other parts of the world (Eller et al., Citation2017). Reed expansion is generally considered undesirable in nature reserves because areas dominated by common reeds generally have lower plant species richness than the initial marsh or fen vegetation. Commonly, the mowing of reeds is performed in wetlands for animal species requiring an open vegetation structure (Güsewell et al., Citation2000). Moreover, the mowing of reeds also removes nutrients from the water and keeps a waterbody attractive to tourists. Management programs have proven that common reeds can be controlled, and in most cases, natural vegetation will return; however, it is also important to note that some areas have been so heavily manipulated and degraded that it may be impossible to eliminate common reeds from them. There is no doubt that physical removal of reeds is useful in the short term but long-term effects of mowing are still unclear (e.g. how intensive mowing should be performed and what optimal height of mowing reeds), and recommendations to restore reed-invaded fen meadows by mowing in the summer are based on circumstantial evidence and still need experimental testing (Güsewell et al., Citation1998).
Monitoring provides the data needed to decide if control measures are necessary to control invasive macrophyte populations. When a control program is begun, it is important to monitor targeted macrophyte populations so that the program’s effectiveness can be determined and, if possible, leave untreated control areas that could be monitored as well for comparison. Relying only on in situ measurements can be time-consuming, hard to cover large areas and do frequent measurements, as well it is often difficult to get aquatic vegetation samples as accessibility to them is hard (Silva et al., Citation2008; Vis et al., Citation2003). It is imperative to continue monitoring even if a control program succeeds initially because common reeds may reinvade and the sooner new patches of infestation of an earlier stage are detected, the easier and cheaper they can be suppressed, also control techniques may need to be applied several times or, perhaps, regularly (Datta et al., Citation2021). Reed change due to mowing disturbances and herbicides has been thoroughly studied by Derr (Citation2008) and it was found that reeds do not fully recover from the first year of mowing and reed regrowth rate the next year after mowing could be reduced by approximately 55% if no herbicide is applied; nevertheless, it was done in a small area of 4.5 m2 as well in controlled containers. Monitoring effects of reed mowing could be improved by using more efficient methods such as remote sensing data (Bresciani et al., Citation2011).
Earth Observation-based (EO) products can potentially support public authorities in managing inland waters, e.g. for controlling invasive aquatic plants by giving spatial information useful to evaluate the invasion dynamics (Pinardi et al., Citation2018). Some studies were already performed for common reed detection using EO data. Detection of macrophytes in these studies was done by calculating vegetation indices that also allowed the assessment of the health of vegetation as well as complexity (Villa et al., Citation2013). Most of the studies use Landsat 7 ETM+ or Landsat 8 OLI data that has a long timeframe starting from 1984 with spectral (six spectral bands in the visible and near-infrared regions) and temporal (revisiting time of 16 days) resolution, which allow vegetation change monitoring. Even though Landsat 7/8 has a sufficient spatial resolution (up to 30 m), Sentinel-2 MSI surpassed it with up to 10 m resolution and is more useful for monitoring smaller changes in vegetation (Qin et al., Citation2021). The simplest normalised vegetation index (NDVI) algorithm was used for reed detection in several studies (Bresciani et al., Citation2009; and references therein). In Lake Garda (Italy), NDVI demonstrated 91% of accuracy in reed bed spatial coverage detection (Villa et al., Citation2013). Relying only on NDVI can generate misleading classification, as some features in water, e.g. cyanobacteria surface accumulations, have similar reflectance as macrophytes. In past decades, more sophisticated algorithms such as Water Adjusted Vegetation Index (WAVI) and Normalised Difference Aquatic Vegetation Index (NDAVI) were developed that are adjusted to the water environment (Villa et al., Citation2013, Citation2014, May), and together with higher Sentinel-2 resolution, these algorithms improve vegetation monitoring accuracy. Experiments that were done in Lake Garda and Mantua Lake system (Italy) showed that NDAVI and WAVI demonstrated high sensitivities, comparable and often slightly higher than the other indices (NDVI, leaf area index, soil adjusted vegetation index), in particular over aquatic vegetation (Villa et al., Citation2014, May). Despite the high detection accuracy of vegetation indices, it is a challenge to delineate transitional zones such as between water and floating-leaved or emerged macrophytes (helophytes) and between helophytes and land vegetation, therefore, using normalised difference water index (NDWI) can improve delineation between canopy and water as shown by some studies (Gao, Citation1996; McFeeters, Citation2013; Szabó et al., Citation2016).
Interest in the mapping of wetland environments and the classification of their resources using EO is a growing topic as spectral signatures may vary substantially in different wetlands, and they are hard to access for detailed in situ analysis (Amani et al., Citation2018; Bhatnagar et al., Citation2020). The studies on calculating Above Ground Biomass (AGB) in wetlands from EO images acquired efficient results such as an accuracy of R2 = 0.59 and RMSE = 194 g m−2 with UAV-derived reed heights and ratio vegetation index in the Nan Da Gang Wetland Reserve (Lu et al., Citation2022). A higher AGB accuracy (R2 = 0.65 and RMSE = 168 g m−2) was achieved by combining the LiDAR and hyperspectral images in the Zhangye National Wetland Park (Luo et al., Citation2017). Although this parameter is useful for wetland management (including the effectiveness of reed mowing), extensive in situ measurements together with laboratory analysis should be done first to calibrate the reed height estimates derived from UAV or LiDAR, as non-local models should be used with caution (Bojórquez et al., Citation2020).
Efficient and automatic segmentation of vegetation indices is an important step for evaluating the mowing effect. For single-band images, thresholding is the most common pre-processing step and the simplest form of thresholding is binary, where foreground and background are separated. The goal of using an automatic threshold is to reduce the need for in situ measurements that can be costly and time-consuming. The most commonly used thresholding algorithm for remote sensing images is Otsu (Otsu, Citation1979), which was used for vegetation detection (Srinivas et al., Citation2019), coastal aquaculture area (Lu Yewei and Lu Yewei, Citation2015), shoreline mapping (Sunder et al., Citation2017), etc. Despite the apparent easiness of the thresholding process, it is a complex procedure given the vast array of circumstances and environments that are unique to each image (Carabias, Citation2012).
The objective of this study was to determine the best approach for the evaluation of reed bed area changes due to mowing using remote sensing methods and automatic thresholding algorithms. This was done by testing water and aquatic vegetation indices by masking open water. We also assessed, if and how the determined approach accuracy of detecting reed beds depends on their density. Based on this method, we hypothesised that the mowing effect from EO data should be more apparent in larger than 0.1 ha reed bed areas since such area almost corresponds to the area of Sentinel-2/MSI 10 m2 pixel size with a buffer of one pixel in all directions.
Materials and methods
Study area
Plateliai Lake (area 1.200 ha, average depth 10.5 m, maximum depth of 49.1 m) is an oligo-mesotrophic lake (2.4 ± 1.8 µg chlorophyll-a l−1 in the period 2001–2010) that belongs to the Žemaitija National Park, located in the western part of Lithuania (). Vegetated areas by common reeds are not spread evenly throughout the lake, and more reed beds are in the eastern part (Sinkevičienė et al., Citation2005). From 2017 to 2019, the Žemaitija National Park directory implemented mowing of reed beds in the littoral zone of the lake in 7 areas (). In total, eight AOIs (area of interest) were selected for further analysis. Seven AOIs correspond to the areas where the mowing of reed beds was performed, and one AOI was selected as a reference, where the mowing was not performed (). The first seven AOIs were numbered after the technical specification document of reed mowing (Technical specification of Plateliai Lake reed mowing, Citation2017). All areas combined cover 18 ha, 1.5% of the whole lake area. A common reed dominated in selected AOIs, while lakeshore bulrush (Schoenoplectus lacustris) formed small stands only in a few AOIs. Therefore, all macrophyte species within the reeds were considered as part of the reed bed in this study.
Figure 1. (a) Plateliai Lake with areas of interest (AOIs) indicated by numbers (where mowing of reed beds was performed), “reference” (where mowing of reed beds was not performed) and their size (in ha), (b) UAV image (acquired on the 10th July 2018) of AOI 5, where reed beds were mowed every second line and mowing process, (c) example of reed bed (AOI 4) before mowing.

The exact date of each year’s mowing is not known, but it was always performed between 20 July and 10 September. The mechanical cutting method was applied from a boat in deeper areas () and using a scythe in shallower areas. In depths ≥0.5 m, reeds were cut by leaving 30 cm stems from the bottom, and in shallow areas (<0.5 m depth), they were cut to the bottom. In most of the areas, reeds were entirely mowed, except for one AOI that is relatively the largest (AOI 5), where the mowing was performed in stripes of 5 m width by leaving a part of the reed beds to enhance biodiversity conditions and habitats for local bird and fish species ().
The study covered the period from 2016 to 2020. The mowing of reeds was performed in 2017, 2018 and 2019, while 2016 and 2020 are considered the reference years since mowing was not performed.
UAV data for validation
UAV images were acquired in several AOIs (, AOI: 1, 4, 5, 10, 11, reference) to compose orthophotos and validate the mapped reed beds that were derived from satellite data (see Sect 2.4). The images were acquired using a DJI Phantom 4 multi-rotor UAV that has a 1” CMOS 20-megapixel RGB camera with an 84° field of view. Flight missions were planned before flight using the DroneDeploy app with the following parameters: flight height of 40 m; 75% front and side overlap between images; flight angle facing towards or away from the sun to minimize a sun glint effect (Joyce et al., Citation2019); coverage polygon larger than mapped AOI. With these parameters, UAV aerial images had a ground spatial resolution of 2 cm per pixel.
Table 1. Dates of sentinel 2 image acquisition with corresponding dates of validation from the UAV images and AOI and mowing status of reed beds (see Sect 2.2).
Table 2. Performance of reed bed classification using seven thresholding algorithms for WAVI (top) and NDWI (bottom).
Four ground control points (GCPs), made from plastic, were used to georeference orthophotos and get more accurate results. GCPs were measured with a Leica 1200 smart GPS rover that uses the Global navigation satellite system. Image mosaicking was performed using the OpenDroneMap (OpenDroneMap, Citation2020) software that implements structure from motion to build orthophotos, digital elevation models and 3D models of the area. Homogenous water surface lacks tie-points (distinct pixels matching in two pictures taken next to each other), therefore only areas with emerged macrophytes were mosaicked.
After composing orthophotos, 12 of them out of 16 were selected (with clouds and water waves not influencing the final result) for validation with classified rasters derived from satellite images. Reed beds were manually delineated from the orthophotos using QGIS version 3.16 (QGIS.org, Citation2022, 2022). The density of reeds was evaluated visually by dividing orthophotos into 10 m2 polygons equal to Sentinel-2 pixel size. Polygons without vegetation were classified as water (0% vegetation, n = 540), while polygons with reeds were classified into three density classes: low density (25% vegetation, n = 687), medium density (50%, n = 461) and high density (100%, n = 360).
Satellite data
The Multispectral Instrument (MSI) on board Sentinel-2A and Sentinel-2B data were used. Sentinel-2/MSI has a spatial resolution of 10 m (4 bands), 20 m (6 bands) and 60 m (2 bands) and a spectral resolution ranging from 440 nm to 2202 nm in 12 bands. Over Plateliai Lake Sentinel-2/MSI revisit time is every 3 days.
Most Sentinel-2/MSI products were already processed to Level-2A data and are available for download in the Copernicus Open Access Hub (https://scihub.copernicus.eu/). The atmospherically corrected (Level-2A) images before September 2017 were not available for download, therefore Sen2Cor processor was used for the atmospheric correction of Top-Of-Atmosphere (TOA) Level-1C images. All Sentinel-2/MSI images were resampled to a final spatial resolution of 10 m. In total, 16 Sentinel-2/MSI images were used (), considering the date closest to the beginning and end of the mowing period (i.e. between the 20 of July and the 10 of September). Most of the images were cloud-free, except for AOI 5, where the image closest to the mowing of reeds (6 September 2020) contained cloud shadows; therefore, the image acquired on the 1 September 2020 was used instead, in AOI 5. In addition, the 19 June 2016 image had a cloud shadow on half of AOI 13, but since there were no cloudless images during this time, the image was used excluding AOI 13 from the analysis.
Calculation of the area and density covered by reeds using sentinel-2/MSI data
The effect of reed mowing in Plateliai Lake was tested using two parameters: changes in the area covered by a common reed and changes in its density within AOIs. The evaluation was performed in five major steps indicated in the workflow ().
Figure 2. Workflow of remote-sensing data processing, validation and analysis. Step 1- masking land; step 2 - masking water; step 3 - validation of reed bed areas and density with UAV data; step 4 - extracting data in considered AOIs; step 5 - assessment of the changes in the area covered by common reed and their density.

Step 1: Masking the land. The Sentinel-2 image from mid-spring was used for masking the land (). The image acquired in mid-spring represents the period when aquatic vegetation had not yet started growing. The normalized difference vegetation index (NDVI) was utilized (Rouse & Haas, Citation1973) since it is most commonly used for land vegetation mapping and is also suitable for aquatic plants (Powell et al., Citation2014; Sakuno & Kunii, Citation2013). This algorithm uses a red spectral band, where radiation is mostly absorbed by vegetation, and Near Infrared (NIR) band, which appears relatively bright on vegetation. In the case of Sentinel-2/MSI, NDVI (Eq. 1) was calculated using Band 8 (NIR) and Band 4 (Red). The NDVI raster was classified with an Otsu binary algorithm (Otsu, Citation1979) and the class representing the land was masked for further analysis.
where RrsRed is remote sensing reflectance in a red satellite band and RrsNIR is remote sensing reflectance in the NIR satellite band.
Step 2: masking water. The most complicated task is to find the optimal threshold (Carabias, Citation2012) that accurately masks water and leaves only aquatic vegetation. For this, two indices were tested, which demonstrated the highest potential in particular over aquatic vegetation (Villa et al., Citation2014, May): Normalised Difference Water Index (NDWI) and Water Adjusted Vegetation Index (WAVI). NDWI (Eq. 2) utilizes remote sensing reflectance in the green and NIR spectral regions that were corresponded by Band 3 and Band 8 in the case of Sentinel-2/MSI data (McFeeters, Citation2013), and WAVI (Eq. 3) utilizes remote sensing reflectance in the blue and NIR spectral regions that are corresponded by Band 2 and Band 8 in case of Sentinel-2/MSI data:
where RrsGreen is remote sensing reflectance in a green satellite band, RrsBlue is remote sensing reflectance in a blue satellite band, and RrsNIR is remote sensing reflectance in the NIR satellite band. L is the WAVI background correction factor.
For the WAVI background correction factor L, a single value of 0.5 was used, which is the best option in the case where no a priori data about vegetation density is available, as suggested by Huete (Citation1988), and confirmed by other studies (Qi et al., Citation1994; Rondeaux et al., Citation1996).
NDWI and WAVI indices were selected considering the band spatial resolution in Sentinel-2/MSI images, as both of them use bands (blue, green and NIR) that have 10 m spatial resolution. NDAVI was not used as it uses the same bands as WAVI, which is better adjusted for the water environment (Guanhua Zhou et al., Citation2018).
Both indices (WAVI and NDWI) were automatically classified into water and reeds using seven binary thresholding algorithms, selected out of 16 available in Fiji software (Schindelin et al., Citation2012), after visually inspecting two images (17 July 2018 and 26 August 2019 used for validation with UAV orthophotos) with calculated indices, using function try all. Seven thresholding algorithms were left for further analysis: Otsu (Citation1979), Yen et al. (Citation1995), RenyiEntropy (Kapur et al., Citation1985), Triangle (Zack et al., Citation1977), Mean and Iso (T. W. Ridler and S. Calvard, Citation1978). The simplest example of these algorithms would be the mean thresholding (Eq. 4) where the threshold is calculated by the mean of two peak values of an image histogram.
where t is the threshold value, gpeak1 is the first maximum value in a histogram, and gpeak2 is the second maximum value in a histogram. Sezgin and Sankur (Citation2004) described 40 thresholding techniques under unified notation and grouped these thresholding algorithms according to information they are exploiting from an image histogram: shape, measurement space clustering, entropy, object attributes, spatial correlation and local grey-lever surface. According to this classification, Otsu, Mean and Iso are defined as clustering thresholding algorithms, Yen and RenyiEntropy as entropy thresholding algorithms and Triangle as a geometric thresholding algorithm.
Step 3: validation of reed bed areas with UAV data. Two satellite images were used for validation: 17 July 2018 and 26 August 2019. The most suitable index with a threshold was selected after validation with 12 UAV orthophotos. Polygons of 10 m2 from orthophotos were classified as vegetation or water and compared to overlapping pixels of reed beds derived from satellite data (step 2). The accuracy of satellite-derived reed beds was assessed by the means of a confusion matrix (Ting, Citation2017), where the true positive and negative values indicated the number of matches between, respectively, presence and absence of reed beds derived from the UAV and satellite data (i.e. observed and predicted reed beds). From the confusion matrix, the Area Under the Curve (AUC; Egan, Citation1975) together with sensitivity and specificity was calculated to determine the most accurate approach for reed bed area mapping using satellite imagery. Sensitivity shows how accurately the true (vegetation) class was predicted, and specificity shows how well the false class (water) was predicted, whereas the AUC estimate covers both aspects. The thresholding algorithm was considered suitable, if the value for sensitivity and specificity was equal to or higher than the arbitrary value of 0.5, meaning correct classification was achieved for 50% of the data. After rejecting unsuitable thresholding algorithms, the post-hoc Bonferroni test was used on AUC values to test which method, using indices (WAVI or NDWI) together with the thresholding algorithm, performed the best at masking water and leaving aquatic vegetation.
To find whether at a certain density thresholding algorithm becomes unreliable, the confusion matrix of the best performing Sentinel-2/MSI index with thresholding algorithm was tested again (using the same accuracy metrics) considering each vegetation density (low, medium, high) derived from the UAV orthophotos. To reduce the likelihood of type one error, the multiple comparisons Dunnett’s post hoc test was used to compare the mean AUC of the treatment group (each vegetation density) to a control group (AUC value of 0.5), representing poor discrimination between classes (Mandrekar, Citation2010).
Step 4: validation of reed bed density with UAV data. The estimated density values of reed beds in AOIs were retrieved from Sentinel-2/MSI data using WAVI (Villa et al., Citation2014, May). The performance of WAVI was validated with the density visually determined from UAV orthophoto and classified polygons (see Sect 2.2). From WAVI values, averaged over each class of vegetation in percentages and including water class, linear regression was derived and linear regression function was used to convert WAVI values to estimated density values.
Step 5: assessment of the impact of reed mowing. The effect of reed bed mowing was evaluated in each AOI, comparing satellite-derived and classified (with the most accurate index and threshold algorithm) areas covered by reeds before and after mowing during the corresponding year (2016–2020). The differences in areas were classified according to three aspects: lost area (reed bed areas decreased), remaining area (reed bed areas have not changed) and gained area (reed bed areas increased). The analysis was performed using a zonal statistic in QGIS version 3.16 (QGIS.org, Citation2022, 2022). Results are presented in hectares as well as in percentages relative to each AOI size, considering it to be 100% (). To find whether mowing occurred in an AOI, an arbitrary threshold of 0.1 ha vegetated area (equal to one Sentinel-2/MSI pixel (10 m2) together with a buffer of surrounding pixels combined) was considered significant.
Results
Validation of water and vegetation indices for reed bed area determination
Results from confusion matrices comparing vegetation areas (reed beds) calculated from WAVI and NDWI indices in each AOI to vegetation areas derived from UAV orthophoto (see . UAV data) showed that five thresholding algorithms (Triangle, Iso, Li, Mean for WAVI and Triangle for NDWI) incorrectly identified water as a vegetated area in more than 50% (specificity lower than 0.5) of the cases (). The rest of the thresholding algorithms showed a larger than 0.5 specificity rate (correct distinction of water for more than 50% of data), while the sensitivity (correct distinction of macrophytes for more than 50% of data) of the four thresholding algorithms (Otsu, RenyiEntropy, Yen for WAVI and Otsu for NDWI) were lower than 0.5. Therefore, previously mentioned thresholding algorithms were removed from further analysis, and only five thresholding algorithms for the NDWI index (RenyiEntropy, Yen, Iso, Li and Mean) were compared.
The Yen thresholding algorithm for the NDWI index had the highest mean AUC (); however, using the Dunnett post hoc test it was not statistically different (p > 0.05) compared to the other thresholding algorithms. The mean specificity obtained by the Iso, Li and Mean was 30% lower than the one determined by the RenyiEntropy and Yen, which resulted in many false-positive values in one of the validation images (). Consequently, the RenyiEntropy and Yen thresholding algorithms were selected and compared with each other. The mean AUC and specificity of RenyiEntropy were slightly lower than the Yen thresholding algorithm, therefore the Yen thresholding algorithm was selected as the final method for classification between vegetated area and water.
Figure 3. NDWI index (derived from Sentinel-2 image acquired on 17 July 2018) with Li threshold showing overestimation of reed beds in the open lake part (a), and with Yen thresholding showing the distribution of reed beds with no overestimation (b).

The Dunnett post hoc test showed greater values for high (df(43) = 5.78, p < 0.05) and medium (df(43) = 4.30, p < 0.05) vegetation density AUC, compared to the AUC value of 0.5 that indicates no discrimination between classes (Mandrekar, Citation2010). No significant difference (df(43) = 2.12, p = 0.1) was found when comparing the same AUC value of 0.5 to the AUC of low-density vegetation. The mean specificity for all densities was similar and was higher than 0.97 (see ). For high and medium vegetation density, the mean sensitivity values were higher than 0.5 and therefore reliable; whereas, for low vegetation density, the sensitivity was <0.5.
The changes in vegetated area induced by reed mowing
The changes in estimated reed bed area in all AOIs during the whole period of study were diverse; nevertheless, significant changes in the vegetated area derived from the satellite images acquired before and after mowing were evident (). The reed bed area in the reference AOI did not significantly change in 2016 and 2017; however, the vegetated area significantly increased (>0.32 ha) in later years, i.e. 2018, 2019 and 2020.
Figure 5. Estimated reed bed areas (ha) in 8 AOIs derived from sentinel-2/MSI images after removal of water pixels determined by the Yen thresholding algorithm for NDWI (a). The estimated reed bed areas (ha) that were reduced (b), remained (c) and gained (d) during the study years. Scale is different for each parameter to highlight specific cases of change. Significant (> 0.1 ha) vegetation area or change is highlighted in bold.

Similar patterns as in the reference AOI were observed in AOI 9 where the reed bed area increased significantly in the same years. The most evident decrease in the vegetation area was observed in AOI 4 during the years, when the mowing was performed, while the increase in the vegetated area was observed in the reference years (2016 and 2020). The vegetated area decreased in AOI 5 by a significant amount in 2018 (0.40 ha) and 2019 (0.70 ha); however, it increased significantly (1.07 ha) in the first year of mowing 2017. The largest one-time loss throughout the study was in AOI 13, where the vegetated area was reduced by half of its area (0.79 ha) in the first year of mowing and remained relatively small through the rest of the years. In 2020, when the mowing was not performed, the vegetated area recovered in all AOIs.
The reduction of reed bed areas in the reference AOI was observed in two investigated years, in 2016 it decreased by 3.1% of the total area of AOI, and in 2017 by 2.9% (). In 2017, the loss of reed bed area is relatively low in comparison with the reduced vegetation area in other AOIs (on average 19.9 ± 14.8%). The remaining vegetation area was relatively stable over the studied period in the reference AOI with an average of 38.7 ± 3.9% of the total area of AOI (). A gradual increase of the gained vegetation area in the reference AOI was determined starting from the first study year, when it was 0.08 ha (1.6% of the total area), to 2019, when gained vegetated area comprised 0.84 ha, i.e. 16.3% of the total area, and 2020 with 0.83 ha of gained vegetated area, i.e. 16.1% of the total area ().
In AOI 4, the most evident reduction of the vegetated area was observed in 2017, 2018 and 2019, i.e. the investigated years, when the mowing of reed beds was performed (). The reduced area accounted for 0.61 ha (43.5% of the total AOI area), in 2018 it was 0.38 (27.1% of the total AOI area), while in 2019 the reduced vegetated area was 46.4% of the total AOI area. The remaining vegetation in AOI 4 was the largest in 2016 at 1.2 ha (85.6% of the total AOI area) as well as significant areas remained in 2017 at 0.26 ha (18.5% of the total AOI area).
In AOI 5, the reduced vegetation area followed a similar pattern as in the reference AOI in the first reference year of 2016 reducing by 0.18 ha and in the first mowing year of 2017 reducing by 0.08 ha (2.9% and 1.3%, respectively). In the following two mowing years of 2018 and 2019, reduced vegetation areas were highest in AOI 5 of 0.41 ha (6.6%) and 0.81 ha (13.1%), respectively. As this AOI is one of the largest, the remaining vegetation was significant in all years, with the lowest being in 2018 and 2020 at 1.2 ha (19.2% of the total AOI area) and the largest in 2016 at 2.7 ha (31.0%). Gained vegetation area was significant during most of the study years except for 2018 when gained vegetation area was only 0.01 ha (0.2%).
In AOI 9, reduced vegetation area was low with the only change being in 2017 of 0.08 ha (24.7% of the total AOI area). The remaining vegetation area was only significant in 2016 with 0.25 ha (77.1%). Gained vegetation area for most mowed AOIs was not different from reference AOI (p > 0.5) except for AOI 9 with 32.1 ± 14.9%, which was significantly different (df(5) = 3.9, p < 0.01) from reference AOI that averaged 8.6 ± 7.1%. In AOI 9 gained vegetation was significant with 0.16 ha (49.3% of the total AOI area) in 2016 and 0.12 ha (37.0%) in 2019 and 2020.
AOI 10 reduced vegetation area only in mowing years; however, this reduced area was not significant, with 0.04 ha (11.7%) in 2017 and 0.05 ha (14.6%) in 2018 and 2019. The remaining vegetation area was significant in 2016 and 2017 with 0.23 ha (67.3%) and 0.15 ha (43.9%), respectively. Gained vegetation area was only significant in 2020 with 0.22 ha (64.4% of the total AOI area).
A significant reduction of vegetation area in AOI 11 was observed in 2019 of 0.12 ha (8.4% of the total AOI area). The remaining vegetation area was significant in all years ranging from 0.27 ha (18.9%) in 2017 to 0.76 ha (53.2%) in 2016, but comparing the mean remaining vegetation area in AOI 11 (31.9 ± 13.2%) to reference AOI that also had significant remaining vegetation in all years, it did not significantly (df(5) = −2.78, p = 0.025) differ from the mean (38.7 ± 3.9%) in the reference AOI.
AOI 12 reduced in vegetation area significantly in 2017 with 0.2 ha (20.1% of the total AOI area). This AOI had a significant remaining area in most of the years except for 2017 when it was 0.08 ha (8.0%). AOI 12 gained significant vegetation area in reference years with 0.13 ha (13.0%) in 2016 and the same amount in 2020 in 2019 with 0.11 ha (11.0%).
AOI 13 had one of the biggest reduced vegetation areas during a single year of 0.8 ha (31.7%) in 2017, and also in 2019 this AOI reduced a significant vegetation area by 0.19 ha (7.5%). The remaining area variation in AOI 13 was small 6.3 ± 3.1% compared to its area, but the data is missing in 2016 when the highest remaining vegetation was in all other mowed AOIs. Gained vegetation area was observed in 2020 of 0.38 ha (15.0%).
In the reference years, average reduced vegetation area was significantly (df(35) = −6.3, p < 0.01) lower (0.8 ± 1.1%) than in years when mowing was performed (14.7 ± 13.0%). In the same years, the remaining vegetation was higher (35.5 ± 28.6%) than in mowing years (18.3 ± 11.6%) with a significant difference (df(35) = 3.46, p < 0.01). The average gained vegetation area of all AOIs were highly influenced by vegetation gained in 2020 (the year after mowing was completed) where the average of all AOIs was significantly (df(21)= −5.2, p < 0.01) higher 30.4 ± 18.8% (0.69 ± 0.74 ha) compared to 2017 of 7.2 ± 6.8%, 2018 of 11.8 ± 16.9% and 2019 10.7 ± 12.5%.
Temporal changes in reed density
The averaged WAVI values were highly correlated with the emergent vegetation density (r = 0.99, p < 0.05) derived from the UAV data. As WAVI could be considered as a proxy for reed density, the derived linear regression function () was used to convert WAVI values to relative coverage of reeds. According to the linear regression function, the values below 0.04 were considered as water (0% vegetation) and any value over 0.22 was considered as fully covered by reeds (100% vegetation).
Figure 6. The relationship between the reed bed density (0%, 25%, 50%, 100%) estimated from the UAV images and averaged WAVI values. The regression line is provided with 95% confidence intervals.

Comparing individual AOI vegetation density values to the reference AOI, AOI 13 corresponded almost to a similar density in 2017 and was lower in 2018 (). None of the mowed AOIs had an average estimated vegetation densities as high as the reference AOI 84.6 ± 30.1% (p < .05) except for AOI 5 where the difference was not significantly different (p > .05) and the estimated vegetation density average was 72.7 ± 24.6%. The second-largest reed density was in AOI 11 with 54.8 ± 19.4%. The lowest estimated vegetation density was in AOI 9 of 23.0 ± 18.7%, this AOI is also the smallest in size.
Figure 7. The reed density values in percentages of 8 AOIs (a) estimated from WAVI values retrieved from sentinel-2/MSI images that represent the period before and after mowing of the investigated years. Density differences in each year before and after reed growth season (b).

Nonetheless, comparing before and after mowing estimated vegetation densities, a significant difference in average change () was found in years 2016 with vegetation density being higher by 36.2 ± 19.2%, lower in 2018 by −17.9 ± 11.3% and higher again in 2019 by 28.0 ± 21.1%; however, significant (df(7) = 0.43, p > 0.05) change was not found in the first mowing year with an average of −1.8 ± 26.4% lower in the vegetation density, and the reference year 2020 lower on average by −12.0 ± 35.1% (df(7) = 0.2, p > 0.05).
Even though the lower vegetation densities were in 2018 when the smallest vegetation areas were determined (), no relationship (r = .05, p > .05) was observed between the change in the vegetated area () and estimated vegetation densities (), and as well no relationship (r = .05, p > .05) was found comparing only AOIs where the significant loss or gain in the vegetation area occurred ( bolded values).
Discussion
The remote sensing of the vegetated area by reeds: methodological considerations
The workflow in this study is based on the concept that remote sensing can evaluate areas of reeds in the whole lake, as it is fairly simple to delineate between water and dense vegetation as well as mask the land from the water environment. Methods of masking all pixels automatically that were not related to aquatic vegetation, were chosen as this approach does not require any prior measurements, whereas most other similar studies (Bresciani et al., Citation2009; Powell et al., Citation2014; Villa et al., Citation2013) relied on in situ data for thresholding.
In general, remote sensing for vegetation area evaluation showed a good correlation between UAV-derived vegetation and dense vegetation detected from a satellite. Nonetheless, one satellite image (17 of July 2019) that was used for validation with UAV images had a low intra-class variance due to a cloud haze () that was not corrected by Sen2Cor atmospheric correction (Gao & Li, Citation2012; Richter et al., Citation2011). This could have an influence on the final results of this image classification by some algorithms (Triangle, Iso, Mean, and Li) and also on density values that do not match area results (area decreased but density increased). For this reason, it is important to thoroughly inspect images before using them for classification or testing other atmospheric correction algorithms that are available for Sentinel-2, e.g. iCOR (De Keukelaere et al., Citation2018), ATCOR (Schläpfer & Richter, Citation2014), ACOLITE (Martins et al., Citation2017). In this image, the water area appeared more similar to vegetated areas, and the unvegetated shallow bottom was classified as the background, which shows that binary thresholding should be chosen carefully and might not be the best option for classifying areas in some cases; therefore, more sophisticated algorithms such as clustering or artificial neural network-based segmentation could be tested that might improve results (Singh et al., Citation2020; Zheng & Chen, Citation2021).
Overall, results from the study demonstrated that the mowing effect can be detected from the EO data, especially in mowed AOIs larger than 0.1 ha, where the reduced area was significantly different from the reference AOI, and also the reduced vegetation area was significantly higher in the mowing years. Vegetated areas derived from the satellite images were slightly underestimated compared to the areas delineated by UAV orthophotos. These small differences might be due to high spatial resolution differences between orthophotos 2 cm/pixel and Sentinel-2/MSI 10 m/pixel (Bollas et al., Citation2021). Another reason for the underestimation was that low-density vegetation was included in a total estimation of vegetation area, which did not reach a reliable AUC threshold as low-density vegetation had lower index values, making overall discrimination between water and aquatic vegetation poorer. For better water vegetation detection, it is suggested to implement a short-wave infrared (SWIR) band that has significantly different reflections comparing aquatic vegetation and cyanobacteria (Oyama et al., Citation2015). Modified normalized difference water index (MNDWI) implements SWIR band to water index calculations and is claimed to reach better results than NDWI (Xu, Citation2005) as well as Sentinel-2 Water Index (SWI) that showed higher classification accuracy than NDWI (Jiang et al., Citation2020). Even though these indices showed good potential to distinguish between water and vegetation, the SWIR band has to be resampled from 20 to 10 m resolution to match other bands in indices, and looking at smaller scales, as in this study, can lead to misclassifications.
The reed beds with a density of ≥50% were proven to be classified more accurately (50–85%) than reed beds with a density of 25% (31% accuracy). Therefore, the tested approach of reed bed delineation in this study is only suggested to use for the densest reed beds, which may lead to an underestimation of 15%. The highest estimated vegetation density was in AOI 5 and the reference AOI throughout the study period. The reason for this might be that this is the only AOI (5th) where different cutting methods were applied; nonetheless, this area still gives a signal to satellite images and pixels were counted as vegetated areas. In general, a higher estimated vegetation density (WAVI higher by 0.02 on average) was detected after mowing at the end of the vegetation season since the reeds reach their physiological maturity at the end of August (Villa et al., Citation2013).
Practical use of the results in waterbody management
A method using Yen thresholding on the NDWI index applied to Sentinel-2 images showed good results in the separation of water from reed beds, therefore it is recommended to use as a tool for evaluating area change during mowing works in waterbodies. Although the accuracy for determining the density is not sufficient, especially when it comes to medium- and low-density classes, having reference AOIs with 100% density allows for a robust way to assess the effect of mowing, follow the recovery, and plan mowing works accordingly.
The management program of 2017–2019 showed a significant reduction of reed bed areas compared to reference years, meaning successful nutrient removal from the lake environment. The gained vegetation area was significantly higher in the year after mowing ended; suggesting that the reed bed areas will recover over time and mowing will have to be repeated after a few years.
In 2018 and 2019, the reduced vegetated area was still higher compared to the reference years but lower than in the first year (2017) of mowing. This could be explained by the fact that vegetation still had not fully recovered from the first year of mowing, and as suggested by Derr (Citation2008), the reed regrowth rate the next year after mowing could be reduced by approximately 55% if no herbicide is applied. The recovery of reed beds after mowing works implies that it has to be performed continuously (Ailstock et al., Citation2001; Asaeda et al., Citation2006; Derr, Citation2008).
Macrophyte monitoring is commonly performed every 3 years (e.g. EPA Ireland and EPA Illinois) along several transects, where macrophyte relative abundance is estimated in situ. The tested method in this study allows monitoring of reed bed hotspots in the whole lake area and not just mowed AOIs; therefore, remote sensing techniques can provide a synoptic, more frequent in time view over a wide range of ecosystems and are very cost-effective in monitoring changes. Frequent satellite image data allow choosing the correct time of mowing as it is crucial to get information on the timescale for all reed stands since different lakes require optimal conditions for mowing depending on the end goal of lake management whether it would be to reduce reed aboveground biomass or remove nutrients from the lake (Fogli et al., Citation2014).
This approach could be used for the long-term (retrospective) analysis of aquatic vegetation dynamics, by adding, for example, the Landsat satellite series. The retrospective analysis could allow the indication of the areas in a lake that continuously had higher vegetation areas and densities, and where new mowing areas could be selected for better management of the lake environment. However, before using other satellite datasets, testing of the workflow presented in the study should be done first, as they might have different spectral, spatial and radiometric resolutions than Sentinel-2.
It is common to use in situ observations as validation data for satellite images (Lawley et al., Citation2016), although popularity grows on using UAV data for validation, which is relatively accurate and covers a relatively larger area (Kattenborn et al., Citation2019). UAV flights could be conducted by mowing personnel or lake manager, while the use of a structure from motion allows the high accuracy of above ground biomass (Meneses et al., Citation2018).
Conclusion
After analysis, the presented method using Yen binary thresholding on NDWI index calculated from Sentinel-2/MSI images is proposed, as it had the best performance. The conclusion is as follows: (1) Suggested method is suitable for medium and dense reed beds; (2) A larger reduction in reed bed areas was detected during the period of mowing; (3) Results showed a strong correlation between WAVI and visually evaluated reed densities; (4) No correlation was detected between estimated vegetation densities and vegetation area changes in mowing period.
It is hard to monitor vegetation just from satellite images alone because many variables can skew the results. Our workflow is recommended to use this method as an additional one to in situ measurement. It is relatively quick and covers large areas to evaluate the effectiveness of reed and other emergent macrophytes mowing in waterbodies.
By using the developed approach, it would be possible to evaluate the mowing effect also without prior knowledge of when and where the mowing was performed. In such cases, a longer period, e.g. covering the entire vegetation season or evaluation of several years, should be considered. Time series of relatively frequent satellite images, combining data originating from different optical sensors, or synergistic use of optical and active microwave remote sensing data (Anderson et al., Citation2021), would also be an advantage. However, a primary understanding of vegetation temporal dynamics, phenology and relation with environmental conditions, like air temperature and precipitation, is highly important. This additional information and sufficient time series of satellite images would allow the detection of intra-seasonal changes in coverage or density, less related to a natural environmental impact, but with a mowing performed.
In addition, UAVs could also be used for the robust estimation of the above-ground biomass of reeds after the calibration with additional reed height data collected in the field (Lu et al., Citation2022). Several studies have already proved that the estimation of biomass from reed beds could also be performed using satellite images (Jabłońska et al., Citation2021; Luo et al., Citation2017). In this way, this information would support the estimation of how much nutrients could be removed by mowing, how much biomass can be further used for bioenergy heating or as a green construction material (Sluis et al., Citation2013), and therefore significantly contribute to the management objectives.
Disclosure statement
No potential conflict of interest was reported by the authors.
References
- Ailstock, M.S., Norman, C.M., & Bushmann, P.J. (2001). Common reed phragmites australis: Control and effects upon biodiversity in freshwater nontidal wetlands. Restoration Ecology, 9(1), 49–15. https://doi.org/10.1046/j.1526-100x.2001.009001049.x
- Amani, M., Salehi, B., Mahdavi, S., & Brisco, B. (2018). Spectral analysis of wetlands using multi-source optical satellite imagery. Isprs Journal of Photogrammetry and Remote Sensing, 114, 119–136. https://doi.org/10.1016/j.isprsjprs.2018.07.005
- Anderson, C. J., Heins, D., Pelletier, K. C., Bohnen, J. L., & Knight, J. F. Mapping invasive phragmites australis using unoccupied aircraft system imagery, canopy height models, and synthetic aperture radar. (2021). Remote Sensing, 13(16), 3303. Article 16. https://doi.org/10.3390/rs13163303
- Asaeda, T., Rajapakse, L., Manatunge, J., & Sahara, N. (2006). The effect of summer harvesting of phragmites australis on growth characteristics and rhizome resource storage. Hydrobiologia, 553(1), 327–335. https://doi.org/10.1007/s10750-005-1157-6
- Bhatnagar, S., Gill, L., Regan, S., Naughton, O., Johnston, P., Waldren, S., & Ghosh, B. (2020). Mapping vegetation communities inside wetlands using sentinel-2 imagery in Ireland. International Journal of Applied Earth Observation and Geoinformation, 88, 102083. https://doi.org/10.1016/j.jag.2020.102083
- Bojórquez, A., Martínez-Yrízar, A., Búrquez, A., Jaramillo, V. J., Mora, F., Balvanera, P., & Álvarez-Yépiz, J. C. (2020). Improving the accuracy of aboveground biomass estimations in secondary tropical dry forests. Forest Ecology and Management, 474, 118384. https://doi.org/10.1016/j.foreco.2020.118384
- Bollas, N., Kokinou, E., & Polychronos, V. (2021). Comparison of sentinel-2 and UAV multispectral data for use in precision agriculture: An application from Northern Greece. Drones, 5(2), 35. https://doi.org/10.3390/drones5020035
- Bresciani, M., Sotgia, C., Fila, G. L., Musanti, M., & Bolpagni, R. (2011). Assessing common reed bed health and management strategies in Lake Garda (Italy) by means of leaf area index measurements. Italian Journal of Remote Sensing, 43(2), 9–22. https://doi.org/10.5721/ItJRS20114321
- Bresciani, M., Stroppiana, D., Fila, G., Montagna, M., & Giardino, C. (2009). Monitoring reed vegetation in environmentally sensitive areas in Italy. Italian Journal of Remote Sensing, 41(2), 125–137. https://doi.org/10.5721/ItJRS20094129
- Carabias, D.M. (2012). Analysis of image thresholding methods for their application to augmented reality environments. Universidad Complutense De Madrid.
- Datta, A., Maharaj, S., Prabhu, G. N., Bhowmik, D., Marino, A., Akbari, V., Rupavatharam, S., Sujeetha, J. A. R., Anantrao, G. G., Poduvattil, V. K., Kumar, S., & Kleczkowski, A. (2021). Monitoring the spread of water hyacinth (Pontederia crassipes): Challenges and future developments. Frontiers in Ecology and Evolution, 9, 1–8. https://doi.org/10.3389/fevo.2021.631338
- De Keukelaere, L., Sterckx, S., Adriaensen, S., Knaeps, E., Reusen, I., Giardino, C., Bresciani, M., Hunter, P., Neil, C., Van der Zande, D., & Vaiciute, D. (2018). Atmospheric correction of Landsat-8/OLI and sentinel-2/MSI data using iCOR algorithm: Validation for coastal and inland waters. European Journal of Remote Sensing, 51(1), 525–542. https://doi.org/10.1080/22797254.2018.1457937
- Derr, J. F. (2008). Common reed (Phragmites australis) response to mowing and herbicide application. Invasive Plant Science and Management, 1(1), 12–16. https://doi.org/10.1614/IPSM-07-001.1
- Duke, J. A. (1983). Handbook of energy crops. Centre for New Crops and Plant Products, Purdue University.
- Egan, J. P. (1975). Signal detection theory and ROC-analysis. Academic press.
- Eller, F., Skálová, H., Caplan, J. S., Bhattarai, G. P., Burger, M. K., Cronin, J. T., Guo, W. -Y., Guo, X., Hazelton, E. L. G., Kettenring, K. M., Lambertini, C., McCormick, M. K., Meyerson, L. A., Mozdzer, T. J., Pyšek, P., Sorrell, B. K., Whigham, D. F., & Brix, H. (2017). Cosmopolitan species as models for ecophysiological responses to global change: The common reed phragmites australis. Frontiers in plant science, 8, 1833. https://doi.org/10.3389/fpls.2017.01833
- Fogli, S., Brancaleoni, L., Lambertini, C., & Gerdol, R. (2014). Mowing regime has different effects on reed stands in relation to habitat. Journal of Environmental Management, 134, 56–62. https://doi.org/10.1016/j.jenvman.2014.01.001
- Gao, B. (1996). NDWI—A normalized difference water index for remote sensing of vegetation liquid water from space. Remote Sensing of Environment, 58(3), 257–266. https://doi.org/10.1016/S0034-4257(96)00067-3
- Gao, B. -C., & Li, R. -R. (2012). Removal of thin cirrus scattering effects for remote sensing of ocean color from space. IEEE Geoscience and Remote Sensing Letters, 9(5), 972–976. https://doi.org/10.1109/LGRS.2012.2187876
- Güsewell, S., Buttler, A., & Klötzli, F. (1998). Short-term and long-term effects of mowing on the vegetation of two calcareous fens. Journal of Vegetation Science, 9(6), 861–872. https://doi.org/10.2307/3237051
- Güsewell, S., Le Nédic, C., & Buttler, A. (2000). Dynamics of common reed (Phragmites australis trin.) in Swiss fens with different management. Wetlands Ecology and Management, 8(6), 375–389. https://doi.org/10.1023/a:1026553700571
- Huete, A. R. (1988). A soil-adjusted vegetation index (SAVI). Remote Sensing of Environment, 25(3), 295–309. https://doi.org/10.1016/0034-4257(88)90106-X
- Jabłońska, E., Winkowska, M., Wiśniewska, M., Geurts, J., Zak, D., & Kotowski, W. (2021). Impact of vegetation harvesting on nutrient removal and plant biomass quality in wetland buffer zones. Hydrobiologia, 848(14), 3273–3289. https://doi.org/10.1007/s10750-020-04256-4
- Jiang, W., Ni, Y., Pang, Z., He, G., Fu, J., Lu, J., Yang, K., Long, T., & Lei, T. (2020). A new index for identifying water body from sentinel-2 satellite remote sensing imagery. ISPRS Annals of the Photogrammetry, Remote Sensing and Spatial Information Sciences, V-3–2020, 33–38. https://doi.org/10.5194/isprs-annals-V-3-2020-33-2020
- Joyce, K. E., Duce, S., Leahy, S. M., Leon, J., & Maier, S. W. (2019). Principles and practice of acquiring drone-based image data in marine environments. Marine & Freshwater Research, 70(7), 952. https://doi.org/10.1071/MF17380
- Kapur J, Sahoo P and Wong A. (1985). A new method for gray-level picture thresholding using the entropy of the histogram. Computer Vision, Graphics, and Image Processing, 29(3), 273–285. 10.1016/0734-189X(85)90125-2
- Kattenborn, T., Lopatin, J., Förster, M., Braun, A. C., & Fassnacht, F. E. (2019). UAV data as alternative to field sampling to map woody invasive species based on combined sentinel-1 and sentinel-2 data. Remote Sensing of Environment, 227, 61–73. https://doi.org/10.1016/j.rse.2019.03.025
- Lawley, V., Lewis, M., Clarke, K., & Ostendorf, B. (2016). Site-based and remote sensing methods for monitoring indicators of vegetation condition: An Australian review. Ecological indicators, 60, 1273–1283. https://doi.org/10.1016/j.ecolind.2015.03.021
- Lu, L., Luo, J., Xin, Y., Duan, H., Sun, Z., Qiu, Y., & Xiao, Q. (2022). How can UAV contribute in satellite-based phragmites australis aboveground biomass estimating? International Journal of Applied Earth Observation and Geoinformation, 114, 103024. https://doi.org/10.1016/j.jag.2022.103024
- Luo, S., Wang, C., Xi, X., Pan, F., Qian, M., Peng, D., Nie, S., Qin, H., & Lin, Y. (2017). Retrieving aboveground biomass of wetland phragmites australis (common reed) using a combination of airborne discrete-return LiDAR and hyperspectral data. International Journal of Applied Earth Observation and Geoinformation, 58, 107–117. https://doi.org/10.1016/j.jag.2017.01.016
- Lu Yewei, L. Q. (2015). A method of coastal aquaculture area automatic extraction with high spatial resolution images. Remote Sensing Technology and Application, 30(3), 486–494. https://doi.org/10.11873/j.issn.1004-0323.2015.3.0486
- Mandrekar, J. N. (2010). Receiver operating characteristic curve in diagnostic test assessment. Journal of Thoracic Oncology, 5(9), 1315–1316. https://doi.org/10.1097/JTO.0b013e3181ec173d
- Martins, V. S., Barbosa, C. C. F., De Carvalho, L. A. S., Jorge, D. S. F., Lobo, F. D. L., & Novo, E. M. L. D. M. Assessment of atmospheric correction methods for sentinel-2 MSI images applied to Amazon floodplain lakes. (2017). Remote Sensing, 9(4), 322. Article 4. https://doi.org/10.3390/rs9040322
- McFeeters, S. K. (2013). Using the normalized difference water index (NDWI) within a geographic information system to detect swimming pools for mosquito abatement: A practical approach. Remote Sensing, 5(7), 3544–3561. https://doi.org/10.3390/rs5073544
- Meneses, N. C., Baier, S., Reidelstürz, P., Geist, J., & Schneider, T. (2018). Modelling heights of sparse aquatic reed (Phragmites australis) using structure from motion point clouds derived from rotary- and fixed-wing unmanned aerial vehicle (UAV) data. Limnologica, 72, 10–21. https://doi.org/10.1016/j.limno.2018.07.001
- OpenDroneMap. (2020). Authors ODM - a command line toolkit to generate maps, point clouds, 3D models and DEMs from drone, balloon or kite images. OpenDroneMap/ODM GitHub Page 2020; https://github.com/OpenDroneMap/ODM
- Otsu, N. (1979). A threshold selection method from gray-level histograms. IEEE Transactions on Systems, Man, and Cybernetics, 9(1), 62–66. https://doi.org/10.1109/tsmc.1979.4310076
- Oyama, Y., Matsushita, B., & Fukushima, T. (2015). Distinguishing surface cyanobacterial blooms and aquatic macrophytes using Landsat/TM and ETM+ shortwave infrared bands. Remote Sensing of Environment, 157, 35–47. https://doi.org/10.1016/j.rse.2014.04.031
- Picture Thresholding Using an Iterative Selection Method. IEEE Trans. Syst., Man, Cybern., 8(8), 630–632. 10.1109/TSMC.1978.4310039
- Pinardi, M., Bresciani, M., Villa, P., Cazzaniga, I., Laini, A., Tóth, V., Fadel, A., Austoni, M., Lami, A., & Giardino, C. (2018). Spatial and temporal dynamics of primary producers in shallow lakes as seen from space: Intra-annual observations from sentinel-2A. Limnologica, 72, 32–43. https://doi.org/10.1016/j.limno.2018.08.002
- Powell, S. J., Jakeman, A., & Croke, B. (2014). Can NDVI response indicate the effective flood extent in macrophyte dominated floodplain wetlands? Ecological indicators, 45, 486–493. https://doi.org/10.1016/j.ecolind.2014.05.009
- QGIS.org, 2022. (2022). https://www.qgis.org/en/site/
- Qi, J., Chehbouni, A., Huete, A. R., Kerr, Y. H., & Sorooshian, S. (1994). A modified soil adjusted vegetation index. Remote Sensing of Environment, 48(2), 119–126. https://doi.org/10.1016/0034-4257(94)90134-1
- Qin, Q., Xu, D., Hou, L., Shen, B., & Xin, X. (2021). Comparing vegetation indices from sentinel-2 and Landsat 8 under different vegetation gradients based on a controlled grazing experiment. Ecological indicators, 133, 108363. https://doi.org/10.1016/j.ecolind.2021.108363
- Richter, R., Wang, X., Bachmann, M., & Schläpfer, D. (2011). Correction of cirrus effects in sentinel-2 type of imagery. International Journal of Remote Sensing, 32(10), 2931–2941. https://doi.org/10.1080/01431161.2010.520346
- Rondeaux, G., Steven, M., & Baret, F. (1996). Optimization of soil-adjusted vegetation indices. Remote Sensing of Environment, 55(2), 95–107. https://doi.org/10.1016/0034-4257(95)00186-7
- Rouse, W., & Haas, R. H. (1973). Monitoring vegetation systems in the great plains with ERTS. Third Earth Resources Technology Satellite-1 Symposium, Goddard Space Flight Center at Washington, DC. 1, 309–317.
- Sakuno, Y., & Kunii, H. (2013). Estimation of growth area of aquatic macrophytes expanding spontaneously in Lake Shinji using ASTER data. International Journal of Geosciences, 4(06), 1–5. https://doi.org/10.4236/ijg.2013.46A1001
- Schindelin, J., Arganda-Carreras, I., Frise, E., Kaynig, V., Longair, M., Pietzsch, T., Preibisch, S., Rueden, C., Saalfeld, S., Schmid, B., Tinevez, J. -Y., White, D. J., Hartenstein, V., Eliceiri, K., Tomancak, P., & Cardona, A. (2012). Fiji: An open-source platform for biological-image analysis. Nature Methods, 9(7), 676–682. https://doi.org/10.1038/nmeth.2019
- Schläpfer, D., & Richter, R. (2014). Evaluation of brefcor BRDF effects correction for HYSPEX, CASI, and APEX imaging spectroscopy data. 2014 6th Workshop on Hyperspectral Image and Signal Processing: Evolution in Remote Sensing (WHISPERS), 1–4. https://doi.org/10.1109/WHISPERS.2014.8077488
- Sezgin, M., & Sankur, B. (2004). Survey over image thresholding techniques and quantitative performance evaluation. Journal of Electronic Imaging, 13(1), 146–165. https://doi.org/10.1117/1.1631315
- Silva, T. S. F., Costa, M. P. F., Melack, J. M., & Novo, E. M. L. M. (2008). Remote sensing of aquatic vegetation: Theory and applications. Environmental Monitoring and Assessment, 140(1), 131–145. https://doi.org/10.1007/s10661-007-9855-3
- Singh, G., Reynolds, C., Byrne, M., & Rosman, B. (2020). A remote sensing method to monitor water, aquatic vegetation, and invasive water hyacinth at national extents. Remote Sensing, 12(24), 4021. https://doi.org/10.3390/rs12244021
- Sinkevičienė, D. Z., Matulevičiūtė, D. D., & Stankevičiūtė, D. J. (2005). Report on macrophyte monitoring in rivers and lakes.
- Sluis, T. V. D., Poppens, R. P., Kraisvitnii, P., Rii, O., Lesschen, J. P., Galytska, M., & Elbersen, H. W. (2013). Reed harvesting from wetlands for bioenergy: Technical aspects, sustainability and economic viability of reed harvesting in Ukraine (No. 2460). Alterra, Wageningen-UR. https://library.wur.nl/WebQuery/wurpubs/444612
- Srinivas, C., Prasad, M., & Sirisha, M. (2019). Remote sensing image segmentation using OTSU algorithm. International Journal of Computer Applications, 178(12), 46–50, 0975 – 8887.
- Sunder, S., Ramsankaran, R., & Ramakrishnan, B. (2017). Inter-comparison of remote sensing sensing-based shoreline mapping techniques at different coastal stretches of India. Environmental Monitoring and Assessment, 189(6), 290. https://doi.org/10.1007/s10661-017-5996-1
- Szabó, S., Gácsi, Z., & Balázs, B. (2016). Specific features of NDVI, NDWI and MNDWI as reflected in land cover categories. Landscape & Environment, 10(3–4), 194–202. https://doi.org/10.21120/LE/10/3-4/13
- Technical specification of Plateliai Lake reed mowing. (2017).
- Ting, K. M. (2017). Confusion Matrix. In C. Sammut & G.I. Webb (Eds.), Encyclopedia of machine learning and data mining (p. 260). Springer US. https://doi.org/10.1007/978-1-4899-7687-1_50
- Villa, P., Bresciani, M., Pinardi, M., & Bolpagni, R. (2014, May). Multitemporal assessment of macrophytes using aquatic vegetation indices. SENTINEL-2 for Science, Rome. https://doi.org/10.13140/2.1.3856.3525
- Villa, P., Laini, A., Bresciani, M., & Bolpagni, R. (2013). A remote sensing approach to monitor the conservation status of lacustrine phragmites australis beds. Wetlands Ecology and Management, 21(6), 399–416. https://doi.org/10.1007/s11273-013-9311-9
- Vis, C., Hudon, C., & Carignan, R. (2003). An evaluation of approaches used to determine the distribution and biomass of emergent and submerged aquatic macrophytes over large spatial scales. Aquatic Botany, 77(3), 187–201. https://doi.org/10.1016/S0304-3770(03)00105-0
- Xu, H. (2005). A study on information extraction of water body with the modified normalized difference water index (MNDWI). Journal of Remote Sensing, 9(5), 589–595.
- Yen J C, Chang F J, Chang S. (1995). A new criterion for automatic multilevel thresholding. IEEE Trans Image Process, 4(3), 370–8. 10.1109/83.366472
- Zack G W, Rogers W E and Latt S A. (1977). Automatic measurement of sister chromatid exchange frequency. J Histochem Cytochem., 25(7), 741–753. 10.1177/25.7.70454
- Zheng, X., & Chen, T. (2021). High spatial resolution remote sensing image segmentation based on the multiclassification model and the binary classification model. Neural Computing & Applications. https://doi.org/10.1007/s00521-020-05561-8
- Zhou, G., Ma, Z., Sathyendranath, S., Platt, T., Jiang, C., & Sun, K. (2018). Canopy reflectance modeling of aquatic vegetation for algorithm development: Global sensitivity analysis. Remote Sensing, 10(6), 837. https://doi.org/10.3390/rs10060837