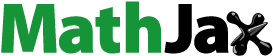
ABSTRACT
In this paper, we evaluated the results in terms of accuracy and coverage of the LiDAR-UAV system DJI Zenmuse L1 and Digital Aerial Photogrammetric system (DAP – UAV) DJI Zenmuse P1 in a forested area under leaf-off conditions on three sites with varying terrain ruggedness/tree type combinations. Detailed reference clouds were obtained using terrestrial scanning by Leica P40. Our results show that branches pose no problem to the accuracy of LiDAR-UAV and DAP-UAV derived terrain clouds. Elevation accuracies for photogrammetric data were even better than for LiDAR data – as low as 0.015 m on all sites. However, the LiDAR system provided better coverage, with almost full coverage at all sites, while the DAP-UAV coverage declined with the increasing density of branches (being worst in the young forest). In the very dense young forest (Site 1), the coverage by photogrammetrically extracted terrain cloud using high calculation quality and no filtering achieved 80.7% coverage, while LiDAR-UAV reached almost 100% coverage. The importance of the use of multiple (or last) returns when using LiDAR-UAV systems was demonstrated by the fact that on the site with the densest vegetation, only 11% of the ground points were represented by first returns.
Introduction
Digital terrain models (DTMs) are important geodetical products of land surveying, obtained by geodetic measurements using a total station and/or a Global Navigation Satellite System (GNSS, usually in the Real-Time Kinematic, RTK, variant) receiver. These methods, although accurate, are slow, laborious and lacking in detail and, therefore, they are being replaced by modern bulk data collection methods, such as laser scanning (from a static position, a ground vehicle or a manned/unmanned aerial vehicle), or photogrammetry (ground, aerial and especially UAV photogrammetry using the Structure from Motion, SfM, method). Both these approaches basically produce similar results (point cloud with centimeter accuracy), from which DTMs in the form of a triangular irregular network (TIN), contours or raster DTM can easily be created by filtering out unwanted objects (vegetation, buildings, etc.). However, due to the principal differences in data acquisition, the results of both approaches may differ, especially in problematic areas, such as forested terrain.
Thus, acquired data can serve various purposes. For example, DAP-UAV was used for the investigation of geological structures (Piras et al., Citation2017), evaluation of geohazards (Nikolakopoulos et al., Citation2017), monitoring of tidal systems (Taddia et al., Citation2021), determination of topographic characteristics for precise agriculture needs (Moravec et al., Citation2017), identification of wild game crop damage (Kuželka & Surový, Citation2018), estimating forest structure and biomass (Almeida et al., Citation2020), creation of DTMs for the needs of sea-level rise scenarios (Leal-Alves et al., Citation2020), creation of bare-earth digital elevation models on barrier islands (Enwright et al., Citation2021) or debris flows mapping (Fraštia et al., Citation2019). Similarly, LiDAR-derived data have also been used for various purposes, including vegetated riverscape topography modelling (Resop et al., Citation2019) identification of individual trees (Balsi et al., Citation2018), for forest structure mapping (LiDAR-UAV; Prata et al., Citation2020), or determining vegetation structure (Moudrý et al., Citation2021). Several studies have also used LiDAR-UAV and DAP-UAV on the same landscape and compared their performance. For example, Hartley et al. evaluated tree nursery trials based on a UAV system mounted with both LiDAR and standard camera (used for the acquisition of imagery for photogrammetry; Hartley et al., Citation2020). Other similar studies included mapping of the passability of terrain vehicles through vegetation using aerial photos and LiDAR data (Rybansky, Citation2022) and quantifying sediment deposition volume after a hurricane from the perspective of safety hazards in vegetated areas using DAP-UAV and manned airborne LiDAR system
(Emtehani et al., Citation2021).
Most of the above-mentioned studies used multicopters, more rarely fixed-wing UAVs; however, similar results were achieved also by other systems using, for example, a UAV airship (Jon et al., Citation2013), carrying technology commonly used for both DAP-UAV and LiDAR-UAV. Both methods, photogrammetric and LiDAR, have their pros and cons and although they can be both used in a wide range of applications, their suitability for individual purposes differs. LiDAR is generally expected to provide better terrain coverage than SfM thanks to its superior penetration capabilities.
Many recent studies have investigated the accuracy and coverage in forested areas using aerial laser scanning (ALS) data acquired from a much greater height than UAV data, which leads to a significantly larger footprint and lower accuracy (typically several decimeters). Jensen and Mathews (Citation2016) evaluated the accuracy of georeferencing of DAP-UAV and ALS data based on reference points determined using GNSS under a canopy. The results suggest an overestimation of the elevation in DAP-UAV compared to LiDAR-UAV (Guerra-Hernández et al., Citation2018); however, an in-depth analysis of the accuracy of individual technologies is missing, which would be beneficial as GNSS accuracy under a canopy is known to be much poorer than on open terrain. Goodbody et al. (Citation2018) compared DAP-UAV and LiDAR-UAV from the perspective of coverage, showing that DAP-UAV provides lower coverage under a canopy. Graham et al. (Citation2019) reported that DAP-UAV data are more sensitive to the tree crown size than to the slope; nevertheless, in their study, they reported deviations of control points up to several meters from DAP-UAV, which appears to be excessive. The use of ALS as a reference dataset was also put in doubt by Simpson et al. (Citation2017) who compared accurate (long-observation) GNSS georeferencing to LiDAR ALS and found that while on vegetation-free surfaces, the deviations are approximately 0.2 m, this greatly increases under a canopy (to approx. 0.8 m).
Other studies evaluate DAP-UAV data accuracy compared to discrete points, typically georeferenced using GNSS. Guerra-Hernández et al. (Citation2017) acquired DAP-UAV imagery with a ground sample distance (GSD) of approx. 6 cm and found the control point deviations to be approx. 5 cm (i.e. a better result than the 1–2× GSD, which is generally considered the best possible DAP accuracy). A similar study performed in a tropical forest recommends the use of DAP-UAV for determining biomass amount (Kachamba et al., Citation2017). Salach et al. (Citation2018) performed an interesting study comparing data acquired by DAP-UAV, LiDAR-UAV and ALS (control points were referenced by GNSS) and found that in vegetation-covered regions, LiDAR-UAV performed better than DAP-UAV. (Jurjević et al., Citation2021) performed a similar study comparing DAP-UAV, LiDAR-UAV, terrestrial laser scanning (TLS) and mobile laser scanning (MLS) using total station/GNSS georeferenced control points. The results have shown that all used technologies slightly (several centimeters) overestimate the terrain; this, however, can be caused by the use of discrete points using a total station with (as is usually the case) a pointed rod, which might have penetrated into the ground and thus actually underestimate the reference points. provides an overview of existing studies related to DTM with sub-centimeter precision.
Table 1. An overview of existing studies.
In view of this, we believe that TLS might be a more suitable reference method for evaluation of DAP-UAV and LiDAR-UAV clouds, facilitating high-accuracy assessment of the coverage as well as the vertical deviation of UAV-based point clouds. Besides, it is necessary that data from individual technologies are in the same coordinate system, which is not mentioned in most aforementioned studies. We have ensured this by georeferencing special high-reflectivity targets for LiDAR-UAV, ground control points for DAP-UAV and special black and white markers for TLS using a high-accuracy total station (Leica P40).
Our team has recently proposed several methods for improving the accuracy of DTM creation using UAV data. The first method used high-quality georeferencing of LiDAR-UAV data utilizing high-reflectivity targets (Štroner, Urban, & Línková, Citation2021), the other was a ground-filtering method combining structural and geometrical filtering (Štroner, Urban, Lidmila, et al., Citation2021). In addition, the quality and accuracy of the terrain point cloud derived from DAP-UAV data can also be improved using oblique imagery (Nesbit & Hugenholtz, Citation2019; Teppati Losè et al., Citation2020), which allows a great reduction in the number of used ground control points (GCPs), thus making the measurement, especially in forested areas, much easier and more economical. The presented paper aims to (i) evaluate the accuracy of DTMs obtained when applying all these improvements and (ii) compare the terrain coverage provided by LiDAR and photogrammetry-derived DTMs in a forested area under leaf-off conditions.
Materials and methods
The study area was represented by the Ďáblice Forest () located in close vicinity to Prague (the Czech Republic). Sessile oak, larch and lime trees are the most common trees within the forest. Three areas within the forest were sensed with a DJI Zenmuse P1 (DAP-UAV) camera and a DJI Zenmuse L1 (LiDAR-UAV) LiDAR from a DJI Matrice 300 UAV. Reference data serving for evaluation of the accuracy were obtained by terrestrial scanning with a Leica P40. A network of stabilized points that subsequently served as a basis for further evaluation of all methods was created through measurement with a total station and a GNSS-RTK receiver. The experiment took place on 1 February 2022, between 10:00 and 16:00.
Figure 1. Study area location (a) and sites (b): A - young forest (red); B - old forest with rugged terrain (green); C - old forest (cyan).

Instrumentation
A Trimble S9 HP robotic total station (standard deviation of length measurement 0.8 mm + 1 ppm D, the standard deviation of the horizontal direction and zenith angle meas-urement 0.3 mgon) paired with Trimble R2 GNSS RTK receiver (dual-frequency receives GPS, Glonass, Galileo, BeiDou satellite system signals; for RTK network, the horizontal accuracy expressed as RMSE is 10 mm + 0.5 ppm, vertical accuracy 20 mm + 0.5 ppm, respectively) with Trimble TSC3 was used for the total station reference measurements.
A Leica P40 terrestrial scanner with a field of view of 360°x270°, distance measurement accuracy of 1.2 mm + 10 ppm, angle accuracy of 8“, liquid compensator with an accuracy of 1.5“, maximum measurement distance of 270 m (considering 18% reflectances) and a scanning speed of 1 million points per second was used for terrestrial laser scanning. To combine individual scans, we used 20 cm spherical targets; georeferencing of the entire combined cloud acquired by scanning from multiple positions within a site utilized black and white targets Leica GZT21 4.5”.
A DJI Zenmuse P1 camera with a DL 35 mm F2.8 LS ASPH lens and a resolution of 8192 × 5460 pixels (45 Mpix, detailed information can be found on the manufacturer’s website - https://www.dji.com/cz/zenmuse-p1/specs) was used for the acquisition of imagery for SfM processing. LiDAR-UAV scanning was performed using the DJI Zenmuse L1 scanning system, with a manufacturer-declared accuracy of 0.1 m per 50 m in the horizontal plane and 0.05 m in the vertical direction (standard deviation). The maximum range at surface reflectivity of 10% is 190 m and the measurement speed is 240,000 points/second (max 480,000 points/second when registering multiple reflections), registering up to 3 reflections per beam. The accuracy of the distance measurement itself is 0.03 m per 100 m. The scanner measurement beam divergence is 0.28° × 0.03° (vertical × horizontal, i.e. 0.24 m × 0.03 m at 50 m). A 20 Mpix colour camera is also present in the system, which is primarily intended for assigning points with true colours. More details can be found on the manufacturer’s website https://www.dji.com/cz/zenmuse-l1/specs. Both systems were carried out by the UAV DJI Matrice 300 RTK, a quadrocopter weighing 6.3 kg equipped with a GNSS RTK receiver with a payload of 2.7 kg, a maximum flight time of 55 min and manufacturer-declared range of 8 km (https://www.dji.com/cz/matrice-300/specs).
Study area
The study area lies near Prague (the Czech Republic, Central Europe) in the Ďáblice Forest. Three sites within this area were chosen, differing in terms of the nature of the forest and the terrain. The area and individual sites are depicted in . The outer polygons indicate the area used for the evaluation of the coverage (completeness of the captured surface); the inner polygons were used for accuracy assessment. The reason for the smaller area used for accuracy evaluation lies in the difficulty of performing terrestrial scanning (reference measurement) with sufficient detail at greater distances from the scanner position in the forest environment due to the presence of trees occluding the ground. Site 1, “young forest” (), is characterized by a dense stand of younger trees with thin trunks and dense branches. The terrain here is essentially flat, covered with fallen leaves.
Figure 2. Site 1 (“young forest”) – a terrestrial photo with hypsometric expression of the terrain shape (altitude contours are at a step of one meter altitude).

Geodetic measurements
To acquire all measurements in the same coordinate system, in view of the large area to be measured as well as of the unreliability of GNSS RTK measurements under a canopy, it was necessary to perform geodetic measurements and create a primary point network by terrestrial methods and in places where it was possible to connect to the GNSS RTK network. The primary points identified in this measurement are shown in (primary points measured by GNSS RTK used for overall georeferencing are marked 4001–4009 and were subsequently used for terrestrial geodetic measurements of points 5001–5111, which could not have been reliably georeferenced by GNSS RTK due to being under the canopy). The coordinates of the ground control points (GCPs) for further measurements were subsequently determined using this network. The Trimble S9 HP total station, employing the automatic prism targeting function in two groups and in both faces of the telescope, was used for these measurements. The distances were corrected for the effect of altitude and cartographic projection. The measurements were adjusted by the least-squares method. The average standard deviation of the horizontal coordinates is 6 mm and of the elevation coordinate 4 mm. GNSS-RTK measurements were performed twice with a minimum time interval of 1 h. The coordinate differences between the two measurements did not exceed 0.02 m in any case (the average difference was 1 mm for position coordinates and 4 mm for height coordinates). The GNSS-RTK measurements were performed, while the drone was operating. The ground control points for ground-based laser scanning (nine in total, three at each site), for DAP-UAV (eight in total) and for LiDAR-UAV (12) were also georeferenced with total stations in one group (two faces of the telescope).
Data acquisition
All mass data collection methods were performed on the same day in a mutually independent way. The flight path for the LiDAR-UAV data acquisition is depicted in in two individual flights (covering separately the northern and southern areas) due to the limited flight time. The flight speed was set to 6 m/s, scanning density to 600 points/m2, and the mean flight height was 100 m above the terrain. The side overlap was set to 65%, the scanning mode to “non-repetitive” and registering to up to three returns. To facilitate further processing, square (0.5 × 0.5 m) ground control points covered with high-reflectivity foil were deployed throughout the area (), enabling the transformation of the entire cloud into a common coordinate system (as described in (Štroner, Urban, & Línková, Citation2021)). GCPs are numbered 7001–7012 in . The flight was planned and piloted by the current version of the DJI Pilot software. In total, 556,751,612 points were acquired (see ). All flights were performed under partly cloudy to cloudy conditions between 10 AM and 4 PM local time at a temperature of 7–11°C. The wind speed (southwest wind) ranged between 2 and 6 m/s.
The DAP-UAV camera imagery was acquired using the same UAV, software and flight height. Both side and frontal overlaps were set to 65%; the image acquisition was, however, not only in the nadir direction – rather, we employed the “smart oblique” method alternating the nadir, oblique left, oblique right, oblique forward and oblique backward image acquisitions (the angling of oblique imagery was 30° from the nadir direction). Compared to the sole nadir acquisition, this method supports (i) a stronger photogrammetric model with a better determination of internal orientation elements (Štroner, Urban, Seidl, et al., Citation2021) and (ii) a better capture of the space (for example, below tree crowns). In all, 1,583 images were taken in the north part and 2,516 in the south part. For further processing, square black and white targets 0.5 × 0.5 m () were deployed as GCPs throughout the area to facilitate georeferencing of the cloud into a common coordinate system. GCPs are labelled 6001–6007 in . Due to the size of the area, relatively small number of GCPs were used in both cases; this was possible since the UAV was fitted with a GNSS RTK receiver, the imagery was, therefore, georeferenced with centimeter accuracy. This, together with the use of oblique images according to (Štroner, Urban, & Línková, Citation2021), ensured that this small number of GCPs is sufficient for control. Scanning with the Leica P40 (TLS) was only performed in the smaller test areas; area 1 has 85,393,269 points (scanning from 5 positions), area 2 has 160,199,145 points (9 positions), and area 3 has 124,167,726 points (7 positions).
2.5 LiDAR-UAV data processing
Data registered during the flight were downloaded and processed using DJI Terra software 3.3.0; the point clouds were smoothed in Terrasolid TerraScan software ver. 022.004 (https://terrasolid.com/products/terrascan/). This procedure can improve the internal quality but not the overall georeferencing. This can, however, be improved using the algorithm described in (Štroner, Urban, & Línková, Citation2021), in which, based on the high intensity of the returns from high reflectivity foils (numbered as 70 × X in , a group of such high-intensity points was selected for each GCP. For each such group of points, an intensity cutoff was set to yield a point cloud corresponding in size to the control point (0.5 × 0.5 m). Subsequently, the coordinates of the centers of the targets were calculated as the mean coordinates of thus selected points (Štroner, Urban, & Línková, Citation2021).
Figure 8. Histograms of vertical differences from the TLS-based reference model for a) LiDAR-UAV; b) DAP-UAV (high quality, depth filtering disabled); c) DAP-UAV (medium quality, depth filtering disabled) for Site 1 (young forest).

The knowledge of the coordinates of these targets based on terrestrial measurements allowed us to calculate the magnitude of systematic errors in individual coordinates, i.e. to calculate parameters of a linear transformation fitting the target coordinates from the point cloud to those determined by total station measurements.
The coordinates of thus acquired GCP centers were subsequently used for determining transformation parameters (least-squares method) and transformation of the entire cloud in CloudCompare ver. 2.12 alpha. When two flights were performed, each of these clouds was transformed separately. The transformation quality was expressed by the post-transformation RMSEs (0.040 m for the northern part with 7 GCPs and 0.096 for the southern part with 6 GCPs), which corresponds to the manufacturer-declared accuracy. This transformation resulted in a point cloud in the S-JTSK coordinate system and the Baltic vertical datum – after adjustment (Bpv) elevation system (see ).
DAP-UAV data processing
The processing was carried out using Agisoft Metashape 1.8.1 Professional 64-bit software and was solved separately for the northern and southern parts as the two flights were not performed with an overlay. All images from each flight were aligned at high quality using all constants of the internal orientation and lens distortion elements (f, cx, cy, k1–k4, p1–p4); the calculation was performed using automatically retrieved GCPs (visually verified by the operator) with coordinates from a geodetic terrestrial survey (assumed accuracy of 0.01 m). Image acquisition coordinates obtained via an onboard GNSS RTK receiver obtained by converting the original WGS84 coordinates to S-JTSK and Bpv using EasyTransform 2.3 software were also used in the calculation. In view of the large amount of data, the dense clouds were calculated directly for the individual test sites (with a negligible overlap that was subsequently cropped to remove artefacts at the margins). All combinations using high (pre-calculation downsampling 1:2), or medium (1:4) quality of dense cloud calculation and various settings of depth filtering (disabled, mild, moderate, aggressive) were compared; the “ultra” quality (calculation from the full resolution photos) was not used as this would not increase the quality further while greatly increasing the computational demands, which is impractical.
Terrestrial scanning data processing
The data were processed by combining scans from each site in each area using three spherical targets and three black and white targets, which were also used for georeferencing. The coordinates of these targets were determined as part of the geodetic measurements.
The RMSE of the registration and georeferencing per coordinate was 3.5 mm, and the calculation was performed in Leica Cyclone software ver. 2021.1.2.
Ground filtering
For quality evaluation, a clipping curve was created for each tested site (delimited by the inner curves in each area, see ) and used for clipping all respective data. The data were further diluted to a minimum point spacing of 1 cm and cleaned of vegetation using the procedure described in (Štroner, Urban, Lidmila, et al., Citation2021), namely using a combination of the structural filtering method CANUPO (Braun et al., Citation2021) and (Brodu and Lague Citation2012) and the CSF geometric filter (Zhang et al., Citation2016), both implemented in CloudCompare. In view of the differing nature of the data acquired by SfM and laser scanning, in particular the magnitude of the noise, the CANUPO filter was used with two different classifiers – one (derived from TLS data) was used for CANUPO filtering of DAP-UAV and TLS data, the other (derived from a subset of the LiDAR data) was used on the LiDAR dataset. The CSF filter settings were constant: scene: steep slope, cloth resolution: 0.2 m, classification threshold: 0.5 m. Data from Study site 2 were the only exception from the rule as due to its ruggedness, it was necessary to refine the cloth resolution to 0.1 m.
Evaluation of the vertical accuracy
The evaluation of vertical accuracy was performed by comparing the terrain clouds (i.e. point clouds representing the terrain obtained in the previous step) acquired by LiDAR-UAV and DAP-UAV (all variants) with the reference data represented by the TLS dataset. The comparison was performed in CloudCompare using the Compute cloud/cloud distance with the option “split X, Y and Z components” turned on and local modelling set to 2D1/2 Triangulation on 15 nearest points. Such calculations provided the description of RMSE data accuracy as well as the mean error and the standard deviation, with the RMSE showing the total root mean square error, the mean error (i.e. the systematic elevation error compared to the reference dataset) and the standard deviation indicating the residual random error. RMSEZ is defined as:
where ΔZ are deviations of the Z coordinates of the points in the tested cloud from the reference point (in our case, acquired using Leica P40 TLS) and the measurement from the particular point cloud (DAP-UAV, LiDAR-UAV).
The Mean Error Z is defined as:
and the Stdev Z as:
Evaluation of the coverage
The evaluation of the coverage (i.e. the percentage of the captured surface) was basically similar to the above-described approach; the outer clipping curves (see ) were used to delimit the area for comparison. After clipping, dilution and ground filtering, the area coverage was determined using rasterization and calculating the percentage of all grid squares containing at least one terrain point. Two grid sizes were applied −0.1 and 0.2 m. The area representing grid cells with at least one point was divided by the total area inside the respective clipping curve. We have considered using a TIN raster calculated from the point cloud but rejected this idea as the TIN model connecting all points would, especially for the LiDAR-UAV dataset (the data of which are extremely “bristly”), result in an artificially large area (see ).
Significance of multiple returns registration
DJI Zenmuse L1 (LiDAR-UAV) registers up to 3 returns from each pulse. We determined the percentage representation of individual returns in the terrain cloud to assess the significance of multiple returns registration.
Direct comparison of DAP-UAV and LiDAR-UAV datasets
To evaluate the agreement and differences between data acquired using DAP-UAV and LiDAR-UAV, a direct comparison of ground-filtered point clouds was performed. As LiDAR-UAV data are more complete (have a better coverage), the reference mesh was created from that dataset and distances of the DAP-UAV filtered cloud from this mesh were calculated. For mesh creation, LiDAR-UAV data had to be rasterized up to 0.1 m × 0.1 m cell size due to the magnitude of the noise. The clouds were compared in CloudCompare software using the function Compute cloud/mesh distance. From these distances, a mean difference, standard deviation of differences and the total RMSD for the vertical component were calculated for each site. Since the distances (differences between the clouds) may be slope-dependent, the slopes were also calculated and presented as graphs. This testing was performed only for a single variant of the calculations, namely the one with dense clouds generated using high quality and disabled filtering.
The RMSDZ is defined as:
where ΔZ are deviations of the Z coordinates of the individual point in the tested cloud from the reference LiDAR-UAV-based mesh.
Mean differencez is defined as
and the Stdevz as:
Results
Internal model quality evaluation
The internal quality of DAP-UAV models derived from individual flights are described by the RMSDs describing residual errors of the GCPs in .
Table 2. RMSDs representing differences between the calculated and measured camera/GCP positions in the SfM model (Agisoft Metashape).
Evaluation of the vertical accuracy
The results of the evaluation of the DTMs’ vertical accuracies for individual sites are presented in . indicate the histograms of vertical deviations from the reference TLS cloud for LiDAR-UAV, DAP-UAV (high quality, no filtering) and DAP-UAV (medium quality, no filtering). Histograms of the remaining calculation variants are very similar to those presented here.
Figure 9. Histograms of vertical differences from the TLS-based reference model for a) LiDAR-UAV; b) DAP-UAV (high quality, depth filtering disabled); c) DAP-UAV (medium quality, depth filtering disabled) for Site 2 (old forest with rugged terrain).

Figure 10. Histograms of vertical differences from the TLS-based reference model for a) LiDAR-UAV; b) DAP-UAV (high quality, depth filtering disabled); c) DAP-UAV (medium quality, depth filtering disabled) for Site 3 (old forest).

Table 3. Results of the elevation accuracy – Site 1 (young forest).
Table 4. Results of the elevation accuracy – Site 2 (old forest with rugged terrain).
Table 5. Results of the elevation accuracy – Site 3 (old forest).
The DAP-UAV data show significantly better accuracy than the LiDAR-UAV data, both in terms of mean elevation error (maximum 0.022 m) and standard deviation (maximum of 0.014 m). The overall accuracy characterized by RMSE does not exceed 0.022 m. Further, we can conclude that the accuracy of the resulting point cloud is only negligibly affected by the quality of the dense cloud calculation, the improvement resulting from the high-quality calculation is no more than 1–2 mm. In all cases, the systematic shift is equal, i.e., the change is only in the standard deviation (which is logical as all SfM clouds share georeferencing). The accuracy does not change with the change of filtering quality, even though the number of points in the cloud is significantly affected – considering high quality without filtering to be a 100% reference, the aggressive filtering leads to its reduction to 77% for high quality and 75% for medium quality. This is valid for the “young forest” dataset; this reduction is lower for Old forest with rugged terrain and Old forest (reduction to 92% and 95%, respectively). The results of the LiDAR-UAV point cloud accuracy evaluation after rasterization are presented in . It is obvious that the RMSE has significantly decreased, almost to the level of DAP-UAV data. At the same time, the relatively slow increase of RMSE with the growing cell size implies that even in the highly rugged terrain of Site 2, rasterized data with relatively large cells can be successfully employed even though the level of detail will obviously be lower with the reduced number of grid cells.
Table 6. LiDAR-UAV elevation data quality after rasterization of the coverage.
The results of the ground coverage evaluation are summarized in , the visualizations are shown in . The data show a superior data coverage for LiDAR-UAV compared to DAP-UAV, with LiDAR-UAV data containing not a single 0.2 × 0.2 m grid cell without any ground points. For the cell size 0.1 × 0.1 m, only 0.7% of cells contained no points in Site 1, 0.1% in Site 2 and 0.2% in Site 3.
Figure 11. Site 1 “young forest” – terrain clouds acquired using: a) LiDAR-UAV; b) DAP-UAV (high quality, depth filtering disabled); c) DAP-UAV (high quality, mild depth filtering); d) DAP-UAV – (high quality, moderate depth filtering); e) DAP-UAV (high quality, aggressive depth filtering) f) DAP-UAV (medium quality, depth filtering disabled); g) DAP-UAV (medium quality, mild depth filtering); h) DAP-UAV (medium quality, moderate depth filtering); i) DAP-UAV (medium quality, aggressive depth filtering).

Figure 12. Site 2 “old forest with rugged terrain” – – terrain clouds acquired using: a) LiDAR-UAV; b) DAP-UAV (high quality, depth filtering disabled); c) DAP-UAV (high quality, mild depth filtering); d) DAP-UAV – (high quality, moderate depth filtering); e) DAP-UAV (high quality, aggressive depth filtering) f) DAP-UAV (medium quality, depth filtering disabled); g) DAP-UAV (medium quality, mild depth filtering); h) DAP-UAV (medium quality, moderate depth filtering); i) DAP-UAV (medium quality, aggressive depth filtering).

Figure 13. Site 3 “old forest” – – terrain clouds acquired using: a) LiDAR-UAV; b) DAP-UAV (high quality, depth filtering disabled); c) DAP-UAV (high quality, mild depth filtering); d) DAP-UAV – (high quality, moderate depth filtering); e) DAP-UAV (high quality, aggressive depth filtering) f) DAP-UAV (medium quality, depth filtering disabled); g) DAP-UAV (medium quality, mild depth filtering); h) DAP-UAV (medium quality, moderate depth filtering); i) DAP-UAV (medium quality, aggressive depth filtering).

Table 7. Site 1 ”young forest“– evaluation of the coverage.
Table 8. Site 2 ”old forest with rugged terrain“– evaluation of the coverage.
Table 9. Site 3 ”old forest“– evaluation of the coverage.
The differences are not so obvious in Sites 2 and 3. Although the tree crown density appears to be similar to that observed at Site 1, the reality is different – the trees are older and although their crowns are tall, the lower stores are empty. As is shown in , in Site 1, where the vegetation density is high, only 11% of the first reflections represented the terrain; the second (49%) and third (40%) returns dominated. In Sites 2 and 3, the proportion of first reflections is much higher (19% and 29%, respectively). The proportion of second returns is about the same in all sites (49%, 49%, 47%), but the number of third returns declines with the decreasing density of understory vegetation (40%, 32%, 24%). In view of the vegetation density, the LiDAR laser spot size (approx. 0.25 m × 0.03 m at 50 m distance) represents an advantage, highly increasing the chance that at least a part of each beam penetrates the vegetation cover down to the terrain.
Table 10. Evaluation of the multiple returns’ registration – the proportion of terrain points registered in the respective order of registration.
Direct comparison of DAP-UAV and LiDAR-UAV data
Results of the direct comparison of the data at all tested sites are shown in . Note that these comparisons were always performed in the entire area, i.e. in areas larger than those used for comparison with TLS data.
Table 11. Differences between DAP-UAV and lidar-UAV clouds.
We observed a good agreement in the mean elevation difference between LiDAR-UAV and DAP-UAV clouds in both sites with old trees Sites 2 and 3), with a mean difference of approx. 0.01 m, while in Site 1 with young forest, the mean difference was negative (approx. −0.02 m).
a) – c) show the elevation difference between DAP-UAV and LiDAR-UAV ground filtered models for individual sites, d) – f) show the slope. Comparison of these figures indicates that the magnitude of the differences is independent of the slope.
Discussion
The accuracy of DAP-UAV models is usually evaluated using residual errors on ground control points. Where the UAV is equipped with onboard RTK GNSS, residual errors can be calculated from the known accuracy of the UAV georeferencing during the flight. As shown in , residual errors in our study were below 0.02 m for GCPs and below 0.03 m for cameras. This is a good result – for comparison, Guerra-Hernández et al. (Citation2017) reported deviations for a fixed-wing UAV eBee flying at 170 m altitude to be approx. 5 cm; similar results were acquired also by Birdal et al. (Citation2017). Salach et al. (Citation2018) reported higher deviations of approx. 7 cm using a rotary UAV flying at an elevation of only 50 m; such a relatively high error compared to previous studies as well as to ours appears to indicate that the method used in their paper might be suboptimal.
Point clouds acquired photogrammetrically using the DAP-UAV (DJI Zenmuse P1) and LiDAR-UAV (DJI Zenmuse L1) differ in character as can be seen from (an area of about 5 × 5 m) and , (ground data profile).
Figure 15. Data representing an area of approx. 5 × 5 m – a) LiDAR-UAV data b) DAP-UAV data c) terrestrial scanning data.

Figure 16. A selected profile mapped by LiDAR-UAV (blue points), DAP-UAV (red) and reference TLS (green): a) actual profile data b) height differences from the reference dataset (TLS) c) profile location within Site 2.

In the left panel (a) shows LiDAR-UAV data that suffer from major noise but capture the full reality. This results from the principle of data acquisition in which any return is measured (even multiple returns from the same signal). The picture in the center (b) shows DAP-UAV data – the photogrammetric reconstruction is unable to capture thin branches and tree trunks at various degrees of occlusion.
The use of oblique imagery is, according to the literature, a great technique for improvement of DTMs (Nesbit & Hugenholtz, Citation2019; Teppati Losè et al., Citation2020). Oblique images may provide another set of images revealing terrain (for example, just below the tree crown) that is, when looking from a nadir direction, obscured by the tree crown. In this way, oblique imagery can significantly improve the coverage.
We have observed an interesting phenomenon. It is generally believed that terrain acquisition by DAP is problematic as the canopy obscures the ground and DAP is unable to penetrate it. This is typically overcome by DAP acquisition during the leaf-off period, but even so, the leafless branches obscure the terrain to a major extent (Moudrý et al., Citation2019). Our model, however, provided almost “ground filtered” data (see ). This was likely due to the fact that on the day of image acquisition, it was slightly windy (2–6 m/s), which caused the branches to move slightly, which prevented their inclusion in the SfM models as the same pixels are not in the same position on adjacent photos and the software fails to identify them.
Data from the terrestrial scanner P40 are also shown as a reference as in view of its accuracy and level of detail, this method represents the most accurate depiction of reality.
compares representations of a selected profile acquired by the three technologies used in this paper. It is clear that LiDAR-UAV data are more scattered than those acquired by DAP-UAV, which are very close to the reference TLS data. shows the same not in absolute numbers but as differences from the reference dataset.
The accuracy of data acquired by DAP-UAV (SfM) and LiDAR-UAV (separately) has been investigated many times; the accuracy of photogrammetric methods in elevation is, under favourable conditions, typically 1–2× ground sampling distance (GSD) generally (e.g. Santise et al., Citation2014; Štroner, Urban, Seidl, et al., Citation2021). In our experiment, the mean GSD for the DAP-UAV cloud was 0.008 m, implying the expected elevation accuracy of 8–16 mm. However, such expectation (especially as the reference method is TLS with extremely detailed ground coverage and high accuracy) would be unrealistic as the ground is covered by dry leaves, which makes it extremely uneven.
In view of this, the elevation accuracy (RMSEZ) acquired by SfM with the reference terrestrial scanning (Leica P40) of 0.02 m can be still considered excellent. The quality of georeferencing is reflected in the systematic component of this error (7 mm to 16 mm in our sites). On the other hand, the random component is practically identical for all sites and calculation variants, ranging between 12 mm and 14 mm.
The accuracy of Zenmuse L1 (LiDAR-UAV)-derived data is slightly worse, with RMSEZ ranging between 44mm and 65 mm, which is, however, still relatively good, especially considering the effects of the fallen leaves on the ground (see the comparison with the literature below). The systematic component of the error is similar to that reported for Zenmuse P1 (DAP-UAV), namely 22 mm, 15 mm and −17 mm for individual sites, respectively; the random component described by standard deviations is 38 mm, 48 mm and 63 mm, respectively. This random component of the error should be perceived (considering the character of the data that can be seen in ) as a point layer with 95% of points lying within approx. 2× standard deviation in each direction (upward and downward), i.e. within approx. 240 mm from the determined point in the worst case. This is difficult to use in practice – it is possible to generate a mesh (TIN) from such data, but the model is too “bristly” (). The logical solution to this problem is to perform rasterization; we used the Rasterize function in CloudCompare, which calculated the mean elevation of all points for a set grid cell size and such a calculated elevation was “placed” into the center of the cell. This approach significantly reduces the random error to approx. 20 mm, with the systematic component remaining practically unaffected.
The coverage quality is a very important parameter; optimally, of course, a 100% coverage is desirable, but it is difficult to achieve in view of the obstacles, such as trees, branches, shrubs or other occlusions (e.g. due to terrain characteristics, such as overhangs). In our experiment, the emphasis was put on the effect of vegetation and for this reason, Sites 1 and 3 were on a relatively flat terrain. Site 2 was on a rugged terrain to be able to better evaluate the effect of georeferencing error; still, this terrain contained no sharp edges, etc. As expected, LiDAR-UAV data (0.1 m × 0.1 m cell size) provide a better coverage, almost 100% (99.3%−99.9%). DAP-UAV data show much lower coverage, especially in Site 1 with young forest (with the best value without data filtering and high-quality processing with 0.1 m × 0.1 m cells being 80.7%, with aggressive filtering and same settings only 57.5%). When medium quality was used, the results were even worse – namely, 62.1% and 44.6% (no filtering and aggressive filtering, respectively). Better results were achieved on Site 2 – the best result was 95.3% for high-quality processing without filtering; the same settings yielded 92.1% on Site 3. The remaining results were proportionally worse without any major swings, just like the use of the 0.2 m × 0.2 m cells (showing higher coverage but the same trends). Comparisons of LiDAR-UAV and DAP-UAV systems have been published in the literature. Liao et al. (Citation2021) compared the digital surface model (DSM) acquired by a LiDAR-UAV system (SZT-R250 sensor onboard a DJI Matrice 600 Pro UAV, flight altitude 100 m) with a DSM acquired by SfM using DJI Phantom 4 Pro (flight altitude 200 m). The comparison was performed separately for three areas of different types (forest, wasteland, and bare land). The RMSDs between LiDAR-UAV and DAP-UAV were 1.4 m, 0.08 m, and 0.69 m, respectively. In view of the LiDAR-UAV system’s declared accuracy of 0.02 m (standard deviation in elevation) and the GSD of the photogrammetric method of about 0.06 m, these results are obviously suboptimal.
Salach et al. (Citation2018) compared DTMs created from data acquired by a LiDAR-UAV system YellowScan Surveyor (using Velodyne laser sensor VLP-16, manufacturer-declared subdecimeter accuracy) and DAP-UAV using the Sony Alpha a6000 camera (24 Mpix), both mounted on a Hawk Moth quadrocopter. Testing was performed in a riverbank area covered by high mixed vegetation, agricultural plants, roads, bushes, and trees. The flight altitude was 50 m above the terrain, camera GSD was approx. 0.02 m. Accuracy was evaluated on GNSS RTK-total station georeferenced points (standard deviation of approx. 0.03 m). The elevation RMSE was in the best case, i.e. low vegetation of 0–0.2 m, 0.14 m for DAP-UAV and 0.11 m for the LiDAR-UAV system. For comparison with our results, it is more appropriate to use results achieved for higher vegetation cover of >0.6 m with RMSEs of 0.36 m and 0.11 m, respectively.
The DTM estimation in a lowland deciduous forest was examined in (Jurjević et al., Citation2021), among other methods, also by LiDAR-UAV and DAP-UAV; as a reference, terrestrial measurements by total station with an accuracy of <0.01 m were used. The achieved RMSEs were 0.14 m for DAP-UAV and 0.09 m for LiDAR-UAV, respectively.
The accuracy of DTM under leaf-off conditions on teak plantations using DAP-UAV was investigated by (Aguilar et al., Citation2019), who, similarly to us, used TLS as a reference (FARO Focus 3D X-330 instrument). The achieved elevation accuracy was characterized by a mean deviation of −0.03 m and RMSE of 0.12 m; similarly, the elevation RMSE in Slovak forests ranged between 0.08 and 0.2 m (Tomaštík et al., Citation2017) or even 0.08 m (Kršák et al., Citation2016). In (Lovitt et al., Citation2017), the accuracy ranged between 0.15 m and 1.1 m; however, it is not the aim of this paper to discuss all such studies as there are many indeed. However, we can still add, for comparison, the elevation accuracy of DAP-UAV on a vegetation-free terrain, with (for example) (James & Robson, Citation2012) reporting an RMSE of 0.07 m or (Harwin & Lucieer, Citation2012) an RMSE of 0.06 m. Similarly, we can mention the result of LiDAR-UAV system testing with vertical RMSE of 0.04 m (Kucharczyk et al., Citation2017) where, however, a significantly larger and more accurate system Riegl VUX-1 UAV was employed (with the declared accuracy of 10 mm distance measurement from the flight height of 60 m above ground level (AGL).
In general, the DJI P1 elevation accuracy is assumed to be approx. 1–2 × GSD (which would be 8–16 mm in our case); however, this is valid for flat ground. When considering accuracy on the terrain covered with dry leaves and low vegetation (as was the case in our study), the 1–2 × GSD obviously cannot be expected to be relevant. Still, comparing our results to those of the studies mentioned above reporting the RMSDs for the elevation component of the DTM of 0.1 m or worse, we can still rank our results with the worst RMSEZ detected in the difficult terrain of the rugged Site 2 (20 mm) as excellent. Our measurements using the DJI L1 system (LiDAR-UAV) yielded an elevation accuracy RMSEZ of 0.065 m (worst case, Site 3). Even this value is better than the best results reported in the literature. Using rasterization to a 0.1 m grid square, the RMSE dropped further to a max. of 0.04 m (Site 1).
Therefore, for the needs of DTM production, both methods (DAP-UAV and LiDAR-UAV) are highly suitable and sufficient in terms of accuracy. However, from the perspective of the coverage of the terrain under the trees, DJI L1 (LiDAR-UAV) is clearly superior, especially in Site 1 (young forest), achieving almost 100% coverage in practically all sites, which is much better than the result derived from DAP-UAV imagery.
Our results are better than those reported in the literature. In many of the aforementioned studies, the manufacturer-declared nominal accuracies have been grossly exceeded. It would be, of course, unwise to expect that the manufacturer-declared values would be met in all cases; still, their exceeding by an order of magnitude is highly suspicious. We believe that the accuracy of our results has been positively influenced by:
Terrestrial georeferencing performed with high accuracy, which allowed high-quality georeferencing for all compared measurements (in the magnitude of several millimeters);
Meticulous point cloud georeferencing, i.e. the sufficient number of the terrestrial ground control points marked by well visible black-and-white paper targets despite using the onboard GNSS RTK (Štroner et al., Citation2020; Nesbit et al., Citation2022). For LiDAR-UAV measurements, we used the method employing highly reflective GCPs, which can be identified in the cloud regardless of the possible colour shift (Štroner et al., 2021a);
From the perspective of flight strategy, the combination of nadir and oblique images leads both to the improvement in the model robustness and to a reduction in the proportion of the obstructed areas (Štroner et al., 2021c; Nesbit et al., Citation2022) and (Taddia et al., Citation2020);
Terrain filtering is also important as high-quality removal of the vegetation is absolutely crucial for being able to compare terrain models acquired by deep penetrating LiDAR-UAV data to DAP-UAV data. The poor results of previous studies might have been partially due to the failure to perceive the importance of this step (Wang and Koo Citation2022; Štroner et al., 2021b).
Conclusions
Results obtained in our experiment are in all cases at or below the lower limit of the results reported in the literature. To be able to determine the effect of grown vegetation on the accuracy of the resulting DTMs, we chose locations with a minimum amount of grass or low vegetation, which would render the proper evaluation difficult or even impossible).
We have shown that the presence of branches, twigs or tree trunks has no effect on the accuracy in the case of DTMs acquired using LiDAR-UAV (DJI Zenmuse L1) or DAP-UAV (DJI Zenmuse P1). At the same time, however, due to the nature of the LiDAR-UAV data from the DJI Zenmuse L1 system, some form of rasterization is recommended for further processing of such data. In our case, simply averaging the data within a square cell provided significant smoothening of the LiDAR data for a cell size as small as 0.1 m while at the same time, increasing the accuracy/reducing the RMSEZ from 0.07 m to <0.04 m.
However, where the DTM coverage is concerned, the situation is different. Here, the LiDAR-UAV system provides much better results (almost full coverage), with DAP-UAV coverage declining with increasing vegetation density. The increase in the filtering aggressiveness and reduction of the resolution during calculation also have a detrimental effect on the coverage. In Site 1 with very dense vegetation, DAP-UAV data obtained using high-quality calculation without filtering achieved a coverage (if grid cells with 0.1 m side are considered) of 80.7%, compared to the medium calculation quality with aggressive filtering, which was markedly worse.
We have also investigated the significance of registering multiple returns when using LiDAR-UAV. The fact that only 11% of the terrain points at Site 1 with the densest vegetation were represented by first returns demonstrates the high importance of the multiple returns (or the last return) registration.
It is, however, necessary to note that our measurements were performed under leaf-off conditions, and the above statements and conclusions cannot be applied to leaf-on conditions.
Author contributions
Conceptualization, MŠ and RU; methodology, MŠ and RU; data acquisition, TK and JB; data processing, MŠ and RU; writing – original draft preparation, MŠ; writing – review and editing, RU; visualization, MŠ; supervision, RU; funding acquisition, RU. All authors have read and agreed to the published version of the manuscript.
Acknowledgments
We would also like to thank Ing. Ondřej Kočí from the company Hrdlička spol. s r.o., for piloting the flights and supplying us with the data.
Disclosure statement
No potential conflict of interest was reported by the authors.
The data that support the findings of this study are available from the corresponding author [RU] upon reasonable request.
Additional information
Funding
References
- Aguilar, F. J., Rivas, J. R., Nemmaoui, A., Peñalver, A., & Aguilar, M. A. (2019). UAV-based digital terrain model generation under leaf-off conditions to support teak plantations inventories in tropical dry forests. A case of the coastal region of ecuador. Sensors, 19(8), 1934. https://doi.org/10.3390/s19081934
- Almeida, A., Gonçalves, F., Silva, G., Souza, R., Treuhaft, R., Santos, W., Loureiro, D., & Fernandes, M. (2020). Estimating structure and biomass of a secondary Atlantic forest in Brazil using Fourier transforms of vertical profiles derived from UAV photogrammetry point clouds. Remote Sensing, 12(12), 3560. https://doi.org/10.3390/rs12213560
- Balsi, M., Esposito, S., Fallavollita, P., & Nardinocchi, C. (2018). Single-tree detection in high-density LiDAR data from UAV-based survey. European Journal of Remote Sensing, 51(1), 679–20. https://doi.org/10.1080/22797254.2018.1474722
- Birdal, A. C., Avdan, U., & Türk, T. (2017). Estimating tree heights with images from an unmanned aerial vehicle. Geomatics, Natural Hazards and Risk, 8(2), 1144–1156. https://doi.org/10.1080/19475705.2017.1300608
- Braun, J., Braunová, H., Suk, T., Michal, O., Pěťovský, P., & Kurič, I. (2021). Structural and geometrical vegetation filtering-case study on mining area point cloud acquired by UAV LiDAR. Acta Montanistica Slovaca, 26(4), 661–674. ISSN 1335-1788. https://doi.org/10.46544/AMS.v26i4.06
- Brodu, N., & Lague, D. (2012). 3D terrestrial LiDAR data classification of complex natural scenes using a multi-scale dimensionality criterion: Applications in geomorphology. Isprs Journal of Photogrammetry and Remote Sensing, 68, 121–134. 2012. https://doi.org/10.1016/j.isprsjprs.2012.01.006
- Emtehani, S., Jetten, V., van Westen, C., & Shrestha, D. P. Quantifying Sediment Deposition Volume in Vegetated Areas with UAV Data. (2021). Remote Sensing, 13(12), 2391. 2021. https://doi.org/10.3390/rs13122391
- Enwright, N. M., Kranenburg, C. J., Patton, B. A., Dalyander, P. S., Brown, J. A., Piazza, S. C., & Cheney, W. C. (2021). Developing bare-earth digital elevation models from structure-from-motion data on barrier islands. Isprs Journal of Photogrammetry and Remote Sensing, 180, 269. 2021. https://doi.org/10.1016/j.isprsjprs.2021.08.014
- Fraštia, M., Liščák, P., Žilka, A., Pauditš, P., Bobáľ, P., Hronček, S., Sipina, S., Ihring, P., & Marčiš, M. Mapping of debris flows by the morphometric analysis of DTM: A case study of the Vrátna dolina Valley, Slovakia. (2019). Geografický časopis - Geographical Journal, 71(2), 101–120. ISSN 0016-7193. https://doi.org/10.31577/geogrcas.2019.71.2.06
- Goodbody, T. R. H., Coops, N. C., Hermosilla, T., Tompalski, P., & Pelletier, G. (2018). Vegetation 525 phenology driving error variation in digital aerial photogrammetrically derived Terrain 526 models. Remote Sensing, 10(10), 1554. https://doi.org/10.3390/rs10101554
- Graham, A., Coops, N. C., Wilcox, M., & Plowright, A. (2019). Evaluation of ground surface models derived from unmanned aerial systems with digital aerial photogrammetry in a disturbed conifer forest. Remote Sensing, 11(1), 84. https://doi.org/10.3390/rs11010084
- Guerra-Hernández, J., Cosenza, D. N., Rodriguez, L. C. E., Silva, M., Tomé, M., Díaz-Varela, R. A., & González-Ferreiro, E. (2018). Comparison of ALS-and UAV (SfM)-derived high-density point clouds for individual tree detection in Eucalyptus plantations. International Journal of Remote Sensing, 39(15–16), 5211–5235. https://doi.org/10.1080/01431161.2018.1486519
- Guerra-Hernández, J., González-Ferreiro, E., Monleón, V. J., Faias, S. P., Tomé, M., & Díaz-Varela, R. A. (2017). Use of multi-temporal UAV-derived imagery for estimating individual tree growth in Pinus Pinea stands. Forests, 8(8), 300. https://doi.org/10.3390/f8080300
- Hartley, R. J. L., Leonardo, E. M., Massam, P., Watt, M. S., Estarija, H. J., Wright, L., Melia, N., & Pearse, G. D. An Assessment of High-Density UAV Point Clouds for the Measurement of Young Forestry Trials. (2020). Remote Sensing, 12(24), 4039. 2020. https://doi.org/10.3390/rs12244039
- Harwin, S., & Lucieer, A. Assessing the Accuracy of Georeferenced Point Clouds Produced via Multi-View Stereopsis from Unmanned Aerial Vehicle (UAV) Imagery. (2012). Remote Sensing, 4(6), 1573–1599. 2012. https://doi.org/10.3390/rs4061573
- James, M. R., & Robson, S. Straightforward reconstruction of 3D surfaces and topography with a camera: Accuracy and geoscience application. (2012). Journal of Geophysical Research: Earth Surface, 117(F3), F03017. 2012. https://doi.org/10.1029/2011JF002289
- Jensen, J. L. R., & Mathews, A. J. (2016). Assessment of image-based point cloud products to generate a bare earth surface and estimate canopy heights in a woodland ecosystem. Remote Sensing, 8(1), 50. https://doi.org/10.3390/rs8010050
- Jon, J., Koska, B., & Pospíšil, J. (2013). Autonomous Airship Equipped by Multi-Sensor Mapping Platform. The International Archives of the Photogrammetry, Remote Sensing and Spatial Information Sciences, XL-5/W1(W1), 119–124. https://doi.org/10.5194/isprsarchives-XL-5-W1-119-2013
- Jurjević, L., Gašparović, M., Liang, X., & Balenović, I. Assessment of CloseRange remote sensing methods for DTM estimation in a lowland deciduous forest. (2021). Remote Sensing, 13(11), 2063. 2021. https://doi.org/10.3390/rs13112063
- Kachamba, D. J., Ørka, H. O., Næsset, E., Eid, T., & Gobakken, T. (2017). Influence of plot size on efficiency of biomass estimates in inventories of dry tropical forests assisted by photogrammetric data from an unmanned aircraft system. Remote Sensing, 9(6), 610. https://doi.org/10.3390/rs9060610
- Kršák, B., Blišťan, P., Pauliková, A., Puškárová, P., Kovanič, Ľ., Palková, J., & Zelizňaková, V. (2016). Use of low-cost UAV photogrammetry to analyze the accuracy of a digital elevation model in a case study. Measurement, 91, 276–287. 2016. https://doi.org/10.1016/j.measurement.2016.05.028
- Kucharczyk, M., Hugenholtz, C. H., & Zou, X. (2017). UAV–LIDAR accuracy in vegetated terrain. Journal of Unmanned Vehicle Systems, 6(4), 212–234. https://doi.org/10.1139/juvs-2017-0030
- Kuželka, K., & Surový, P. (2018). Automatic detection and quantification of wild game crop damage using an unmanned aerial vehicle (UAV) equipped with an optical sensor payload: A case study in wheat. European Journal of Remote Sensing, 51(1), 241–250. https://doi.org/10.1080/22797254.2017.1419442
- Leal-Alves, D. C., Weschenfelder, J., Albuquerque, M. D., Espinoza, J. M. D. A., Ferreira-Cravo, M., & Almeida, L. P. M. D. Digital elevation model generation using UAV-SfM photogrammetry techniques to map sea-level rise scenarios at Cassino Beach, Brazil. (2020). SN Applied Sciences, 2(12), 2181. 2020. https://doi.org/10.1007/s42452-020-03936-z
- Liao, J., Zhou, J., & Yang, W. Comparing LiDAR and SfM digital surface models for three land cover types. (2021). Open Geosciences, 13(1), 497–504. 2021. https://doi.org/10.1515/geo-2020-0257
- Lovitt, J., Rahman, M. M., & McDermid, G. J. Assessing the Value of UAV Photogrammetry for Characterizing Terrain in Complex Peatlands. (2017). Remote Sensing, 9(7), 715. 2017. https://doi.org/10.3390/rs9070715
- Moravec, D., Komárek, J., Kumhálová, J., Kroulík, M., Prošek, J., & Klápště, P. (2017). Digital elevation models as predictors of yield: Comparison of an UAV and other elevation data sources. Agronomy Research, 15(1), 249–255.
- Moudrý, V., Gdulová, K., Fogl, M., Klápště, P., Urban, R., Komárek, J., Moudrá, L., Štroner, M., Barták, V., & Solský, M. (2019). Comparison of leaf-off and leaf-on combined UAV imagery and airborne LiDAR for assessment of a post-mining site terrain and vegetation structure: Prospects for monitoring hazards and restoration success. Applied Geography, 104, 32–41. https://doi.org/10.1016/j.apgeog.2019.02.002
- Moudrý, V., Moudrá, L., Barták, V., Bejček, V., Gdulová, K., Hendrychová, M., Moravec, D., Musil, P., Rocchini, D., Šťastný, K., Volf, O. & Šálek, M. (2021). The role of the vegetation structure, primary productivity and senescence derived from airborne LiDAR and hyperspectral data for birds diversity and rarity on a restored site. Landscape and Urban Planning, 210, 104064. https://doi.org/10.1016/j.landurbplan.2021.104064
- Nesbit, P. R., Hubbard, S. M., & Hugenholtz, C. H. Direct Georeferencing UAV-SfM in High-Relief Topography: Accuracy Assessment and Alternative Ground Control Strategies along Steep Inaccessible Rock Slopes. (2022). Remote Sensing, 14(3), 490. 2022. https://doi.org/10.3390/rs14030490
- Nesbit, P. R., & Hugenholtz, C. H. Enhancing UAV–SfM 3D Model Accuracy in High-Relief Landscapes by Incorporating Oblique Images. (2019). Remote Sensing, 11(3), 239. 2019. https://doi.org/10.3390/rs11030239
- Nikolakopoulos, K., Kavoura, K., Depountis, N., Kyriou, A., Argyropoulos, N., Koukouvelas, I., & Sabatakakis, N. (2017). Preliminary results from active landslide monitoring using multidisciplinary surveys. European Journal of Remote Sensing, 50(1), 280–299. https://doi.org/10.1080/22797254.2017.1324741
- Piras, M., Taddia, G., Forno, M. G., Gattiglio, M., Aicardi, I., Dabove, P., Lo Russo, S., & Lingua, A. (2017). Detailed geological mapping in mountain areas using an unmanned aerial vehicle: Application to the Rodoretto Valley. NW Italian Alps, Geomatics, Natural Hazards and Risk, 8(1), 137–149. https://doi.org/10.1080/19475705.2016.1225228
- Prata, G. A., Broadbent, E. N., de Almeida, D. R. A., St. Peter, J., Drake, J., Medley, P., Corte, A. P. D., Vogel, J., Sharma, A., Silva, C. A., Zambrano, A. M. A., Valbuena, R., & Wilkinson, B. Single-Pass UAV-Borne GatorEye LiDAR Sampling as a Rapid Assessment Method for Surveying Forest Structure. (2020). Remote Sensing, 12(24), 4111. 2020. https://doi.org/10.3390/rs12244111
- Resop, J. P., Lehmann, L., & Hession, W. C. Drone laser scanning for modeling riverscape topography and vegetation: Comparison with traditional aerial LiDAR. (2019). Drones, 3(2), 35. 2019. https://doi.org/10.3390/drones3020035
- Rybansky, M. Determination of Forest Structure from Remote Sensing Data for Modeling the Navigation of Rescue Vehicles. (2022). Applied Sciences, 12(8), 3939. 2022. https://doi.org/10.3390/app12083939
- Salach, A., Bakuła, K., Pilarska, M., Ostrowski, W., Górski, K., & Kurczyński, Z. Accuracy Assessment of Point Clouds from LiDAR and Dense Image Matching Acquired Using the UAV Platform for DTM Creation. (2018). ISPRS International Journal of Geo-Information, 7(9), 342. 2018. https://doi.org/10.3390/ijgi7090342
- Santise, M., Fornari, M., Forlani, G., & Roncella, R. (2014). Evaluation of DEM generation accuracy from UAS imagery. The International Archives of the Photogrammetry, Remote Sensing and Spatial Information Sciences, XL-5, 529–536. 2014, XL-. https://doi.org/10.5194/isprsarchives-XL-5-529-2014
- Simpson, J., Smith, T., & Wooster, M. (2017). Assessment of errors caused by forest vegetation structure in airborne LiDAR-derived DTMs. Remote Sensing, 9(11), 1101. https://doi.org/10.3390/rs9111101
- Štroner, M., Urban, R., Lidmila, M., Kolář, V., & Křemen, T. Vegetation Filtering of a Steep Rugged Terrain: The Performance of Standard Algorithms and a Newly Proposed Workflow on an Example of a Railway Ledge. (2021). Remote Sensing, 13(15), 3050. 2021. https://doi.org/10.3390/rs13153050
- Štroner, M., Urban, R., & Línková, L. A New Method for UAV LiDAR Precision Testing Used for the Evaluation of an Affordable DJI ZENMUSE L1 Scanner. (2021). Remote Sensing, 13(23), 4811. 2021. https://doi.org/10.3390/rs13234811
- Štroner, M., Urban, R., Reindl, T., Seidl, J., & Brouček, J. Evaluation of the georeferencing accuracy of a photogrammetric model using a quadrocopter with onboard GNSS RTK. (2020). Sensors, 20(8), 2318. 2020. https://doi.org/10.3390/s20082318
- Štroner, M., Urban, R., Seidl, J., Reindl, T., & Brouček, J. Photogrammetry Using UAV-Mounted GNSS RTK: Georeferencing Strategies without GCPs. (2021). Remote Sensing, 13(7), 1336. 2021. https://doi.org/10.3390/rs13071336
- Taddia, Y., Pellegrinelli, A., Corbau, C., Franchi, G., Staver, L. W., Stevenson, J. C., & Nardin, W. High-Resolution Monitoring of Tidal Systems Using UAV: A Case Study on Poplar Island, MD (USA). (2021). Remote Sensing, 13(7), 1364. 2021. https://doi.org/10.3390/rs13071364
- Taddia, Y., Stecchi, F., & Pellegrinelli, A. Coastal mapping using DJI phantom 4 RTK in post-processing kinematic mode. (2020). Drones, 4(2), 9. 2020. https://doi.org/10.3390/drones4020009
- Teppati Losè, L., Chiabrando, F., & Giulio Tonolo, F. Boosting the Timeliness of UAV Large Scale Mapping. Direct Georeferencing Approaches: Operational Strategies and Best Practices. (2020). ISPRS International Journal of Geo-Information, 9(10), 578. 2020. https://doi.org/10.3390/ijgi9100578
- Tomaštík, J., Mokroš, M., Saloň, Š., Chudý, F., & Tunák, D. Accuracy of photogrammetric UAV-based point clouds under conditions of partially-open forest canopy. (2017). Forests, 8(5), 151. 2017. https://doi.org/10.3390/f8050151
- Wang, Y., & Koo, K. -Y. Vegetation removal on 3D point cloud reconstruction of cut-slopes using U-net. (2022). Applied Sciences, 12(1), 395. 2022. https://doi.org/10.3390/app12010395
- Zhang, W., Qi, J., Wan, P., Wang, H., Xie, D., Wang, X., & Yan, G. An easy-to-use airborne LiDAR data filtering method based on cloth simulation. (2016). Remote Sensing, 8(6), 501. 2016. https://doi.org/10.3390/rs8060501