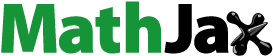
ABSTRACT
Relative soil water content (RSWC) is widely used to characterize the impact of water stress (WS) on vegetation. In bi-layer ecosystems, such as olive groves, this impact must be primarily estimated for the tree component, which, having greater rooting depth, responds more slowly to WS than understory grass. This complicates the application of methods for RSWC prediction, which must be properly adapted to consider the deeper soil layer influential on olive trees. The current study investigates the modification of a recently proposed RSWC simulation method based on a combination of meteorological and satellite-derived normalized difference vegetation index (NDVI) data. The application of the method to an olive grove in Central Italy requires the estimation of both weather and NDVI contributions affecting solely olive trees, which is carried out through the use of appropriate data processing techniques. The RSWC estimates obtained reasonably reproduce the ground RSWC observations referred to the 1 m soil layer, which are representative of the WS affecting olive trees (r2 = 0.795, RMSE = 0.15 and MBE = −0.09). The limits and prospects of this method are finally discussed with particular reference to the possible integration of the RSWC estimates within more complex ecosystem models.
Introduction
Soil water content (SWC) is an important environmental factor, the knowledge of which is fundamental for sustainable water management at different spatial and temporal scales. Soil water shortage, in fact, induces the activation of several eco-physiological mechanisms that reduce plant transpiration and photosynthesis and, consequently, development and growth (Chaves et al., Citation2002). The impact of water stress (WS) on vegetation is often characterized through the transformation of SWC into relative SWC (RSWC), which is obtained by normalizing the former between minimum and maximum values indicative of full soil water depletion and availability, respectively (Allen et al., Citation1998). Hence, RSWC is approximately equivalent to the soil WS coefficient (Ks) utilized by the Food and Agriculture Organization (FAO): when Ks is 1, no soil water deficit subsists, while when it is lower than 1, WS is on (Allen et al., Citation1998).
The operational estimation and/or modelling of RSWC over wide areas generally encounters several difficulties due to its high spatiotemporal variability (Vereecken et al., Citation2014). This is particularly the case for bi-layer ecosystems, such as olive groves, which are composed of a mixture of trees and grasses having different rooting depths. In fact, the water reserve in deep soil layers is both exhausted and replenished more slowly than near the surface, and vertical SWC variations can be additionally caused by other local factors, such as inputs from a shallow water table or lateral advection from adjacent water sources (Brocca et al., Citation2017). Thus, the actual RSWC influence on vegetation varies depending on the soil layer explored; in traditional olive groves, grass and tree root systems share the first soil layer, but the roots of herbaceous plants are generally confined to the first 0.2–0.3 m, while olive trees explore down to 0.8–1.0 m, depending on age, soil and management (Diaz-Espejo et al., Citation2012). In these cases, attention should be focused on the RSWC of the deeper layer, which directly controls the cultivation and management of olive trees (Arampatzis et al., Citation2018).
In a recent investigation, our research group has proposed and tested a modelling strategy that combines standard meteorological and optical remotely sensed data for the operational monitoring of RSWC (Gardin et al., Citation2021). The method is based on the weighting of two factors descriptive of meteorological WS which influence vegetation and bare soil for the respective cover fractions estimated remotely. This modelling strategy offers the advantage of being directly informative about the RSWC that affects local vegetation, which is usually the most relevant for operational agro-ecological applications. Consequently, the method is intrinsically suited to be adapted for estimating the RSWC affecting olive trees, which is the subject of the current research. In particular, the possibility of applying the RSWC estimation method in a by-layer ecosystem is investigated through the use of high spatial resolution normalised difference vegetation index (NDVI) imagery derived from the Sentinel-2 MultiSpectral Instrument sensor (S-2 MSI), which allows the analysis of a small olive grove situated within the urban park of Cascine (Firenze, Central Italy).
The paper is organized as follows: first, the study area and data are introduced, followed by a data processing section that describes the original RSWC estimation method and its adaptation to the current bi-layer ecosystem case. Next, the results obtained are presented and discussed in a final section that concludes with the main prospects of the study.
Study area
The study olive grove (43.7854° N, 11.2183° E) is located in an experimental farm managed by the “Istituto Tecnico Agrario” of Florence, in the Cascine urban park (). This area is flat and close to the Arno River, at an altitude of about 45 m above sea level. The climate is Mediterranean, with mean annual rainfall of about 800 mm and mean annual temperature of about 15°C; rainfall is concentrated in autumn and spring, while summer is generally hot and dry. The soil is sandy-clay-loam (i.e. 52% sand, 24% silt and 24% clay) and has a depth over 2 m.
Figure 1. Geographical position of the study area (Cascine, Florence, Italy) (left) and S-2 NDVI MSI image of August 2021 showing in black the boundary of the study olive grove (right).

The olive grove extends over about 80 × 55 m2 and is surrounded by small agricultural fields grown with grasses and annual crops (mainly vegetables), vineyards and other tree crops. Olive trees are 5 × 5 m2 spaced, have a height of about 4 m and variable crown sizes (up to 4–5 m); they are rainfed and managed following local habits. The inter-tree areas are mostly covered by spontaneous herbaceous species, which are irregularly tilled; grass density and coverage are generally lower under the tree canopy and decrease during the summer dry period. At the end of March 2021, the olive grove was pruned with an intensity that was highly variable in space; the olive tree canopy reduction was notable in the south-eastern part of the grove (up to 80%) and almost null in the north-western part, inducing different olive tree crown sizes.
Study data
The study data consisted of two independent sets: the first, collected in situ, was used to compute the reference RSWC observations, while the second, estimated remotely, was used for RSWC prediction.
Ground data
Daily meteorological observations (i.e. minimum and maximum temperature, precipitation and solar radiation) were collected by means of a ground weather station placed near the olive grove. These measurements were taken during the whole study period, i.e. in 2021 and 2022.
Daily SWC measurements were taken within the olive grove by means of a Drill and Drop Sentek probe (Sentek Sensor Technologies, Stepney, Australia; https://sentektechnologies.com/products/soil-data-probes/drill-drop/). The instrument was placed approximately in the middle of the grove, at a distance of about 1.5 m from one of the olive trees, and collected data at 0.1 and 0.3 m depth from May 2021 to October 2022.
The fraction of photosynthetically active radiation absorbed (fAPAR) by the olive tree canopy was measured by means of an AccuPAR, LP-80 Ceptometer (Decagon Devices, Inc., Pullman, Washington, USA; https://www.metergroup.com/en/meter-environment/products/accupar-lp-80-canopy-interception-par-leaf-area-index). The measurements were collected along linear transects, regularly spaced (1 m), in 10 plots of 10 × 10 m2 (); the first four plots were sampled twice in 2021 (at the beginning of the growing season and in June), while the other seven plots (one in common with the former group) were sampled once in spring 2022.
Figure 2. GoogleEarth true color composites of the study olive grove taken in October 2020 (A), June 2021 (B), March 2022 (C) and May 2022 (D). The numbers in A indicate the position of ten ground plots in which ceptometer observations were taken; the red point indicates the position of the SWC probe (see text for details).

Interpolated and remotely sensed data
Gridded estimates of daily minimum and maximum air temperature and precipitation with a spatial resolution of 200 m were obtained by applying the DAYMET interpolation algorithm to the observations of adjacent ground stations (Thornton et al., Citation2000); the respective solar radiation estimates were derived from Meteosat Second Generation (MSG) images as proposed by Fibbi et al. (Citation2020).
10-m spatial resolution MSI images taken from the Sentinel-2 A and B satellites were downloaded in a pre-processed L2A format from the European Space Agency website (https://sentinel.esa.int/web/sentinel/sentinel-data-access). A maximum value composite (MVC) algorithm was applied to all MSI images covering the study site in 2021 and 2022 in order to retrieve 24 half-month NDVI images per year. These MVC images were subjected to two additional operations aimed at improving their quality, i.e.: 1) a spatial filtering which removed isolated pixels with anomalous NDVI values and 2) a temporal filtering based on an upper envelope algorithm that further reduced atmospheric disturbances (see Maselli et al., Citation2020).
In addition to the MSI imagery, four Google-Earth (GE; https://earth.google.com/) high-resolution RGB images of the study area were collected to characterise the CC of the olive grove during the two study years. These images were collected on 8th October 2020, 4th June 2021 (just after the pruning operation), 28th March 2022 and 11th May 2022 ().
Data processing
Computation of reference RSWC for grass and trees
Reference daily RSWC observations were obtained from the available SWC measurements collected at the study site by applying the method described in Chiesi et al. (Citation2018). Specifically, daily meteorological observations were combined with the SWC measurements collected at depths of 0.1 and 0.3 m to compute the reference RSWC of grass and trees, respectively. In the latter case, the RSWC calculation method had to be forced to consider an effective soil depth (i.e. the rooting depth from which plants can absorb water) of 1 m; this was obtained by driving the bucket model, which computes the soil water balance using this depth in place of that found automatically.
Estimation of RSWC for the olive grove
The prediction of daily RSWC for the study olive grove was performed by processing the interpolated and remotely sensed data through the algorithm described in Gardin et al. (Citation2021). Within this formulation vegetation greenness is considered to be responsive to the soil water conditions cumulated over a sufficient time period and is used to modulate the intensity of meteorological water deficit computed on the basis of precipitation and potential evapotranspiration (PET) estimates. The method therefore predicts the daily the RSWC of day i (RSWCi) by weighting the meteorological water effective on vegetation and soil for the respective cover fractions as:
where FVC is the fractional vegetation cover and AW is the WS scalar, both referred to day i. Half-month FVC values were derived from the NDVI averages of the 10 selected plots through the linear equation of Gutman and Ignatov (Citation1998) and were interpolated on a daily time step. The daily AW was obtained from the described estimates of PET and precipitation (Prec) through:
where PET was estimated from mean air temperature and solar radiation using the algorithm of Jensen and Haise (Citation1963), the two summations were computed over 45 days as proposed by Battista et al. (Citation2016), and AW was bounded to 1 (no WS).
In areas with heterogeneous vegetation cover, the method is mainly driven by the high spatial resolution NDVI imagery used (Gardin et al., Citation2021), and therefore currently provides RSWC estimates having 10 m resolution.
Estimation of RSWC for grass and trees
The prediction of RSWC for the two components of the olive grove, understory grass and olive trees, was based on the separate estimation of the meteorological and remotely sensed information relevant for the two vegetation types. Separate AW scalars were therefore computed over 30 and 60 days for grass and trees, respectively, following the logic exposed in Maselli et al. (Citation2014). Next, the prediction of the half-month NDVI values of the two ecosystem components was performed by the method proposed by Maselli (Citation2001), which is based on the application of linear regression analysis to tree canopy cover (CC) observations (independent variable) and NDVI data (dependent variable). The regression equation found is then extrapolated to 0 and 1 CC for predicting the NDVI of grass and trees.
The estimation of olive tree CC was performed by visual analysis of the plots selected in the olive grove. This operation was applied to all available GE images, and the CC estimates were then temporally interpolated considering slight CC increments during the two growing seasons examined. The CC estimates obtained were assessed versus the ceptometer measurements taken in the 10 ground plots during 2021 and 2022. To this aim, the fAPAR averages of the 15 collected samples were converted into respective values of tree CC following Chiesi et al. (Citation2022).
The application of the regression analyses to all half-month NDVI images yielded relevant estimates for grass and trees, which were converted into the respective FVC values through the same linear equation used above and interpolated into a daily time step. EquationEquations 1(1)
(1) -Equation2
(2)
(2) were then fed with these FVC values and with the interpolated meteorological data cumulated over 30 and 60 days for grass and trees, respectively (Maselli et al., Citation2014).
Accuracy assessment
In all cases, the accuracy of the estimates was assessed versus the ground measurements using standard statistics, i.e. the coefficient of determination (r2), the root mean square error (RMSE) and the mean bias error (MBE).
Results
The annual evolution of mean air temperature and rainfall collected in 2021 and 2022 at the study site is shown in . Mean temperature follows the typical pattern of a Mediterranean area, with relatively low values in winter and clear maxima in summer; during the latter season there are rainfall minima, leading to marked dryness. Some differences are visible between the two years: 2021 was slightly cooler and wetter than 2022, especially during spring and early summer. From May to August, the mean air temperature was 25.4°C and 27.1°C in 2021 and 2022, respectively, while rainfall was almost null from late spring to mid-summer 2022 (from 1st May to August 15th only 11.8 mm versus 40 mm in 2021). The weather estimates obtained by interpolating independent weather datasets faithfully reproduce these measurements; the determination coefficient is higher than 0.89 both for air temperature and rainfall and the MBEs are lower than 0.1°C and −0.2 mm, respectively.
Figure 3. Daily mean air temperature and rainfall collected at the study area by the ground weather station during 2021 and 2022.

The RSWC evolutions observed during the study period (May 2021 – October 2022) are shown in . Evident drops were recorded during the summer period due to water shortage, which, as previously noted, is longer and more intense in 2022. The RSWC drops of grass are generally more marked and irregular than those of trees; the RSWC of grass falls down to about 0.15 in 2021 and 0.10 in 2022; the RSWC difference between the 2 years is higher for the olive trees, with summer minima reaching about 0.6 and 0.4 in 2021 and 2022, respectively.
Figure 4. Daily reference RSWC derived from the available in situ measurements for grass and olive trees during 2021 and 2022.

The olive tree CC estimates obtained by visual interpretation of the available GE images are moderately in accordance with the measurements obtained by means of the ceptometer. The scatter plot of shows that the former reproduce the ground observations quite correctly, inducing only a marginal underestimation (r2 = 0.536, RMSE = 0.103 and MBE = −0.018).
Figure 5. Olive tree CC estimated (visually) versus observed (by ceptometer) with 1:1 dotted line: 8 points (4 per image) are from 2021 and 7 from 2022 (see text for details) (** = highly significant correlation, P < 0.01).

The regression analyses performed between these CC estimates and the half-month NDVI data allow the prediction of separate multitemporal NDVI and FVC values for grass and trees; the latter are shown in together with the average values for the entire olive grove. These last estimates show the typical seasonal trend of Mediterranean ecosystems, with clear FVC maxima in winter-spring followed by a summer minimum consequent on water limitation. These seasonal FVC variations are almost exclusively attributable to the grass component of the olive grove, which is strongly responsive to summer dryness; the FVC of olive trees is instead constantly high during 2021 and only slightly decreases in summer 2022.
Figure 6. Daily FVC estimated from S-2 MSI NDVI data for the olive grove, grass and olive trees during 2021 and 2022.Daily RSWC estimated by equation 1 for the olive grove, grass and olive trees during 2021 and 2022..

The composition of the AW and FVC estimates through Equationequation 1(1)
(1) yields the RSWC estimates shown in for the entire olive grove, grass and trees. The accuracy statistics descriptive of the comparisons between these estimates and the measurements of are reported in . The estimates referred to the two ecosystem components reproduce the ground observations faithfully. In particular, the errors obtained after separating the FVC contributions of trees and grass are markedly reduced for both components: with respect to the reference RSWC descriptive of the 1 m layer the best statistics are obtained for the tree estimates (), while with respect to the RSWC of the shallower layer the lowest errors are obtained for the grass estimates ().
Figure 7. Daily RSWC estimated by equation 1 for the olive grove, grass and trees during 2021 and 2022.

Table 1. Summary statistics obtained by comparing the daily RSWC estimates to the respective reference observations for trees (i.e. 1 m rooting depth, A) and grass (i.e. 0.2 m rooting depth, B) (** = highly significant correlation, P < 0.01).
Discussion and conclusions
Olive tree is a drought-tolerant species that has an adapting strategy based on both the avoidance of WS emergence and the capability to tolerate drought (Villalobos et al., Citation2012). The mechanisms at the basis of this strategy are related to leaf morphological characteristics, the efficient function of water transport via xylem and the regulation of transpiration through the effective control of stomata apparatus (Brito et al., Citation2019). Extreme WS conditions, however, can also negatively affect this kind of trees and have a high impact on all ecophysiological processes, especially in view of the ongoing climate change (Brilli et al., Citation2016; Maselli et al., Citation2012).
This situation enhances the importance of correctly monitoring the impact of WS on olive tree conditions and functions, which can be theoretically carried out by the use of remotely sensed data. Such use, however, is complicated by the bilayer nature of olive groves, within which the tree and grass components are differently responsive to water shortage. The different behaviours are mostly caused by the diversified ecological strategies adopted by the two vegetation types and specifically by their different rooting depths, which induce different accesses to water resources. While, in fact, grass roots are usually confined to more superficial soil layers that are rapidly depleted during WS periods, the greater depths reached by tree roots allow the access to larger water reserves (Schenk & Jackson, Citation2002).
This expectation is supported by the RSWC observations currently collected in situ, which were obtained by normalizing the available SWC measurements through a procedure fully described in Chiesi et al. (Citation2018). The RSWC that affects the olive trees responds more slowly and with lower intensity to summer water shortages with respect to the RSWC impacting understorey grass. These findings are confirmed by the first sap flow observations that are being collected from some selected trees in the olive grove starting from June 2022 (data not shown).
Remote sensing estimation of WS impact on olive trees must address this issue, which is the subject of the current research. In particular, the investigation was aimed at adapting a previously presented semi-empirical method for RSWC prediction whose main properties are fully discussed in Gardin et al. (Citation2021), together with relevant limitations. The method combines meteorological descriptors of WS with estimates of green FVC derived from NDVI data and is intrinsically suitable to assess the response of existing vegetation to water shortage, i.e. the RSWC effect on the respective rooting depth. This property allows a proficient adaptation of the method to the present case once the meteorological and NDVI contributions relative to the two plant types coexisting in the olive grove are properly estimated.
The estimation of meteorological WS scalars for different biome types is straightforward when, as in the current case, spatially extended weather datasets are available. Previous studies have, in fact, demonstrated that the impact of meteorology on different vegetation types can be approximated by using different accumulation times in the calculation of WS conditions (Maselli et al., Citation2014).
The estimation of FVC values, referred uniquely to the two ecosystem components, is instead a more critical issue (Xiao & Moody, Citation2005). The use of S-2 MSI NDVI data, in fact, has been recently confirmed to fit the need for detecting the FVC of olive groves (Kamenova & Dimitrov, Citation2021), but the production of separate NDVI estimates for understory grass and olive trees is more problematic. As reported also by Burchard Levine et al. (Citation2021), the 10-m spatial resolution pixels are not sufficient to properly separate these two contributions, which requires the determination of the respective CC.
This issue has been currently addressed by applying a procedure based on the linear regression of olive grove NDVI values versus tree CC estimates. The latter have been obtained by photointerpretation of commonly available GE images, which allows a cost-effective operational application of the method. However, the accuracy yielded by this operation is variable depending on the quality of the images as well as on the ability of the photo-interpreters. In the case of olive groves, these issues are exacerbated by the irregular shapes of the tree canopies, which may be consequent on the different pruning intensities applied. The use of a single high spatial resolution image per season can therefore suffice for estimating the olive tree CC when no external disturbance is applied, but it is insufficient in the case of pruning (as observed for 2021). Thus, the number of needed GE images is mainly dependent on the natural or human induced disturbances that determine temporal CC variations.
The relatively good quality of the CC estimates currently obtained was demonstrated by comparison with multitemporal ceptometer measurements of fAPAR, which are, however, rarely available. Alternative methods to yield olive tree CC estimates can be represented by the automatic processing of the same optical imagery or of airborne high spatial resolution LiDAR datasets (Maselli et al., Citation2012). The latter are actually more informative on tree CC values but are also more expensive to acquire and therefore taken less frequently, which would create problems when facing the mentioned cases of olive groves showing temporal variations of tree CC. In the case of relatively stable olive groves, the availability of spatially extended CC estimates would also allow us to predict the NDVI/FVC spatial variability of both vegetation layers through a technique based on geographically weighted regression analyses (Maselli, Citation2001).
The possibly inaccurate estimation of olive tree CC is obviously influent on the multitemporal NDVI/FVC values obtained by linear regression analyses. While a direct assessment of these estimates was not currently possible, the FVC values found are generally plausible for both understorey grass and olive trees. The former, in fact, are usually lower and more variable than those of the evergreen trees during the Mediterranean growing season (March–October). In particular, the FVC of grass is more readily sensitive to WS, as was clearly apparent from ground surveys conducted in the olive grove during July–August 2021 and 2022.
The efficiency of the methodology applied to separately analyse the water available for the two olive grove components is also indirectly supported by the final assessment of the RSWC estimates versus the available ground observations. RSWC is, in fact, correctly predicted for the different soil layers explored by both grass and trees. In the former case, the RSWC estimates reproduce the higher temporal variability as well as the lower summer levels of the grass observations, which are due to the thinner layer explored. As fully discussed in Gardin et al. (Citation2021), these properties derive from the more temporally variable AW and lower FVC values, which are combined to yield the grass RSWC estimates. Correspondingly, the RSWC estimates of olive trees well reproduce the main features of the respective observations both in intensity and timing, which is a fundamental prerequisite for the correct prediction of the main olive functions and processes, and particularly olive fruit yield.
A logical extension of the current study could therefore be aimed at investigating the possible integration of the obtained RSWC estimates within modelling frameworks, which simulate these functions and processes. Process-based models, in fact, usually simulate water balance by considering the SWC distribution at different soil depths, which allows to account for the water availability and uptake in a multi-layer ecosystem (Moriondo et al., Citation2019). The competition for water uptake, due to the aboveground vegetation cover (olive tree and grass), is therefore an important factor that should be considered during the simulation of olive tree growth and fruit yield (Pardini et al., Citation2002). Water availability and uptake, however, may vary spatially in olive groves because of multiple environmental factors (e.g. terrain and soil characteristics, understorey cover, etc.). If no integration with remote sensing data is performed, crop growth models are rarely able to reproduce the within-field SWC variability. This situation prevents accounting for the influences of WS on plant growth and productivity under different SWC conditions, thus eventually limiting the estimation accuracy of models at sub-field scale (Leolini, Bregaglio, et al., Citation2022).
Satellite data assimilation with detailed spatial and temporal resolutions can therefore reduce the uncertainty of the simulation during the growing season by improving vegetation monitoring (Leolini, Bregaglio, et al., Citation2022,b). More specifically, the currently proposed formulation of RSWC with NDVI-derived FVC can be useful to account for the spatial variability of soil water deficit and plant WS effects. Within this field of investigation, the use of different vegetation indices could also be considered with the purpose of improving RSWC prediction (Leolini, Moriondo, et al., Citation2022).
Acknowledgments
The authors wish to thank the “Istituto Tecnico Agrario” (ITAS) of Florence, for offering the experimental site. The authors are also grateful to the two EuJRS anonymous reviewers for their helpful comments on the first draft of the manuscript.
Disclosure statement
No potential conflict of interest was reported by the authors.
Data availability statement
Meteorological data are available at https://www.lamma.rete.toscana.it/meteo and the land use map is available at https://land.copernicus.eu/pan-european/corine-land-cover. Sentinel-2 MSI imagery is available at https://sentinel.esa.int/web/sentinel/sentinel-data-access and GoogleEarth images are available at https://earth.google.com/.
Correction Statement
This article has been corrected with minor changes. These changes do not impact the academic content of the article.
Additional information
Funding
References
- Allen, R. G., Pereira, L. S., Raes, D., & Smith, M. (1998). Crop evapotranspiration: Guidelines for computing crop requirements. FAO Irrigation and Drainage Paper No. 5. FAO: https://www.fao.org/3/x0490e/x0490e00.htm
- Arampatzis, G., Hatzigiannakis, E., Pisinaras, V., Kourgialas, N., Psarras, G., Kinigopoulou, V., Panagopoulos, A., & Koubouris, G. (2018). Soil water content and olive tree yield responses to soil management, irrigation, and precipitation in a hilly Mediterranean area. Journal of Water and Climate Change, 9(4), 672–10. https://doi.org/10.2166/wcc.2018.224
- Battista, P., Chiesi, M., Rapi, B., Romani, M., Cantini, C., Giovannelli, A., Cocozza, C., Tognetti, R., & Maselli, F. (2016). Integration of ground and multi-resolution satellite data for predicting the water balance of a Mediterranean two-layer agro-ecosystem. Remote Sensing, 8(9), 731. https://doi.org/10.3390/rs8090731
- Brilli, L., Gioli, B., Toscano, P., Moriondo, M., Zaldei, A., Cantini, C., Ferrise, R., & Bindi, M. (2016). Rainfall regimes control C-exchange of Mediterranean olive orchard. Agriculture, Ecosystem & Environment, 233, 147–157. https://doi.org/10.1016/j.agee.2016.09.006
- Brito, C., Dinis, L. -T., Moutinho-Pereira, J., & Correia, C. M. (2019). Drought stress effects and olive tree acclimation under a changing climate. Plants, 8(7), 232. https://doi.org/10.3390/plants8070232
- Brocca, L., Ciabatta, L., Massari, C., Camici, S., & Tarpanelli, A. (2017). Soil moisture for hydrological applications: Open questions and new opportunities. Water, 9(2), 140. https://doi.org/10.3390/w9020140
- Burchard Levine, V., Nieto, H., Riano, D., Migliavacca, M., El-Madany, T. S., Guzinski, R., Carrara, A., & Martín, M. P. (2021). The effect of pixel heterogeneity for remote sensing based retrievals of evapotranspiration in a semi-arid tree-grass ecosystem. Remote Sensing of Environment, 260, 112440. https://doi.org/10.1016/j.rse.2021.112440
- Chaves, M. M., Pereira, J. S., Maroco, J., Rodrigues, M. L., Ricardo, C. P. P., Osòrio, M. L., Carvalho, I., Faria, T., & Pinheiro, C. (2002). How plants cope with water stress in the field? Photosynthesis and growth. Annals of Botany, 89(7), 907–916. https://doi.org/10.1093/aob/mcf105
- Chiesi, M., Battista, P., Fibbi, L., Gardin, L., Pieri, M., Rapi, B., Romani, M., & Maselli, F. (2018). A semi-empirical method to estimate actual evapotranspiration in Mediterranean environments. Advances in Meteorology, 2018, 9792609. https://doi.org/10.1155/2018/9792609
- Chiesi, M., Costafreda-Aumedes, S., Argenti, G., Battista, P., Fibbi, L., Leolini, L., Moriondo, M., Rapi, B., Sabatini, F., & Maselli, F. (2022). Estimating the GPP of olive trees with variable canopy cover by the use of S-2 MSI NDVI images. European Journal of Agronomy, 141, 126618. https://doi.org/10.1016/j.eja.2022.126618
- Diaz-Espejo, A., Buckley, T. N., Sperry, J. S., Cuevas, M. V., de Cires, A., Elsayed-Farag, S., Martin-Palomo, M. J., Muriel, J. L., Perez-Martin, V., Rodriguez-Dominguez, C. M., Rubio-Casal, A. E., Torres-Ruiz, J. M., & Fernández, J. E. (2012). Steps toward an improvement in process-based models of water use by fruit trees: A case study in olive. Agricultural Water Management, 114, 37–49. https://doi.org/10.1016/j.agwat.2012.06.027
- Fibbi, L., Maselli, F., & Pieri, M. (2020). Improved estimation of global solar radiation over rugged terrains by the disaggregation of satellite applications facility on land surface analysis data (LSA SAF). Meteorological Applications, 27(4), e1940. https://doi.org/10.1002/met.1940
- Gardin, L., Chiesi, M., Fibbi, L., Angeli, L., Rapi, B., Battista, P., & Maselli, F. (2021). Simulation of soil water content through the combination of meteorological and satellite data. Geoderma, 393, 115003. https://doi.org/10.1016/j.geoderma.2021.115003
- Gutman, G., & Ignatov, A. (1998). The derivation of the green vegetation fraction from NOAA/AVHRR data for use in numerical weather prediction models. International Journal of Remote Sensing, 19(8), 1533–1543. https://doi.org/10.1080/014311698215333
- Jensen, M. E., & Haise, H. R. (1963). Estimating evapotranspiration from solar radiation. Journal of Irrigation and Drainage Division, 89(4), 15–41. https://doi.org/10.1061/JRCEA4.0000287
- Kamenova, I., & Dimitrov, P. (2021). Evaluation of Sentinel-2 vegetation indices for prediction of LAI, fAPAR and fCover of winter wheat in Bulgaria. European Journal of Remote Sensing, 54(S1), 89–108. https://doi.org/10.1080/22797254.2020.1839359
- Leolini, L., Bregaglio, S., Ginaldi, F., Costafreda-Aumedes, S., DiGennaro, S. F., Matese, A., Maselli, F., Caruso, G., Palai, G., Bajocco, S., Bindi, M., & Moriondo, M. (2022). Use of remote sensing-derived fPAR data in a grapevine simulation model for estimating vine biomass accumulation and yield variability at sub-field level. Precision Agriculture, 24(2), 1–22. https://doi.org/10.1007/s11119-022-09970-8
- Leolini, L., Moriondo, M., Rossi, R., Bellini, E., Brilli, L., López-Bernal, Á., Santos, J. A., Fraga, H., Bindi, M., Dibari, C., & Costafreda-Aumedes, S. (2022). Use of Sentinel-2 derived vegetation indices for estimating fPAR in olive groves. Agronomy, 12(7), 1540. https://doi.org/10.3390/agronomy12071540
- Maselli, F. (2001). Definition of spatially variable spectral endmembers by locally calibrated multivariate regression analyses. Remote Sensing of Environment, 75(1), 29–38. https://doi.org/10.1016/S0034-4257(00)00153-X
- Maselli, F., Angeli, L., Battista, P., Fibbi, L., Gardin, L., Magno, R., Rapi, B., & Chiesi, M. (2020). Evaluation of Terra/Aqua MODIS and Sentinel-2 MSI NDVI data for predicting actual evapotranspiration in Mediterranean regions. International Journal of Remote Sensing, 41(14), 5186–5205. https://doi.org/10.1080/01431161.2020.1731000
- Maselli, F., Chiesi, M., Brilli, L., & Moriondo, M. (2012). Simulation of olive fruit yield in Tuscany through the integration of remote sensing and ground data. Ecological Modelling, 244, 1–12. https://doi.org/10.1016/j.ecolmodel.2012.06.028
- Maselli, F., Papale, D., Chiesi, M., Matteucci, G., Angeli, L., Raschi, A., & Seufert, G. (2014). Operational monitoring of daily evapotranspiration by the combination of MODIS NDVI and ground meteorological data: Application and validation in Central Italy. Remote Sensing of Environment, 152, 279–290. https://doi.org/10.1016/j.rse.2014.06.021
- Moriondo, M., Leolini, L., Brilli, L., Dibari, C., Tognetti, R., Giovannelli, A., Rapi, B., Battista, P., Caruso, G., Gucci, R., Argenti, G., Raschi, A., Centritto, M., Cantini, C., & Bindi, M. (2019). A simple model simulating development and growth of an olive grove. European Journal of Agronomy, 105, 129–145. https://doi.org/10.1016/j.eja.2019.02.002
- Pardini, A., Faiello, C., Longhi, F., Mancuso, S., & Snowball, R. (2002). Cover crop species and their management in vineyards and olive groves. Advances in Horticultural Science, 16(225–234), 22. https://doi.org/10.1400/14122
- Schenk, H. J., & Jackson, R. B. (2002). Rooting depths, lateral root spreads and below-ground/above-ground allometries of plants in water-limited ecosystems. The Journal of Ecology, 90(3), 480–494. https://doi.org/10.1046/j.1365-2745.2002.00682.x
- Thornton, P. E., Hasenauer, H., & White, M. A. (2000). Simultaneous estimation of daily solar radiation and humidity from observed temperature and precipitation: An application over complex terrain in Austria. Agricultural and Forest Meteorology, 104(4), 255–271. https://doi.org/10.1016/S0168-1923(00)00170-2
- Vereecken, H., Huisman, J. A., Pachepsky, Y., Montzka, C., van der Kruk, J., Bogena, H., Weihermüller, L., Herbst, M., Martinez, G., & Vanderborght, J. (2014). On the spatio-temporal dynamics of soil moisture at the field scale. Journal of Hidrology, 516, 76–96. https://doi.org/10.1016/j.jhydrol.2013.11.061
- Villalobos, F. J., Perez-Priego, O., Testi, L., Morales, A., & Orgaz, F. (2012). Effects of water supply on carbon and water exchange of olive trees. European Journal of Agronomy, 40, 1–7. https://doi.org/10.1016/j.eja.2012.02.004
- Xiao, J., & Moody, A. (2005). A comparison of methods for estimating fractional green vegetation cover within a desert-to-upland transition zone in central New Mexico, USA. Remote Sensing of Environment, 98(2–3), 237–250. https://doi.org/10.1016/j.rse.2005.07.011