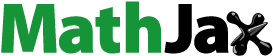
ABSTRACT
Rot in commercial timber reduces the value of the wood substantially and estimating the occurrence, severity, and volume of heartwood rot would be a useful tool in decision-making to minimize economic losses. Remotely sensed data has recently been used for mapping rot on a single-tree level, and although the results have been relatively poor, some potential has been shown. This study applied area-based approaches to predict rot occurrence, rot severity, and rot volume , at an area level. Ground reference data were collected from harvester operations in 2019–2021. Predictor variables were calculated from multi-temporal remotely sensed data together with environmental variables. Response variables from the harvester data and predictor variables from remotely sensed data were aggregated to grid cells and to forest stands. Random Forest models were built for the different combinations of response variables and predictor subsets, and validated with both random- and spatial cross-validation. The results showed that it was not possible to estimate rot occurrence and rot severity with the applied modeling procedure (pR2: 0.00–0.16), without spatially close training data. The better performance of rot volume models (pR2: 0.12–0.37) was mainly due to the correlation between timber volume and rot volume.
Introduction
Deciding whether to harvest timber from a forest or to allow the trees to continue to grow is one of the most important strategic decisions a forest manager must make. This decision typically relies on information regarding timber quality, volume increment, harvesting costs, and timber prices. It usually involves optimizing the net present value of different harvesting times. The optimal time for harvesting can be influenced by many factors, of which natural disturbances can have a significant effect. By incorporating the risk of disturbances in the decision process, the forest owner can adapt the forest management, especially the decision of when to harvest (Susaeta & Gong, Citation2019). Without information about these risks, erroneous decisions are made (Möykkynen & Pukkala, Citation2010), potentially leading to monetary losses for the forest owner.
Due to the effects of global warming, the probability of natural disturbances will likely increase particularly in coniferous forests in the boreal zone (Seidl et al., Citation2017). Among such disturbances are fungal decay pathogens, which can cause rot in living trees, resulting in reduced growth and decreased wood production. Studies conducted in Norway and Sweden found that 14–25% of Norway spruce (Picea abies (L.) Karst.) trees harvested in final felling were affected by rot (Huse et al., Citation1994; Noordermeer et al., Citationin press; Stenlid & Wästerlund, Citation1986; Thor et al., Citation2005). The national study of Huse et al. (Citation1994), in Norway, showed that most of the rot in Norway spruce, 71%, was caused by the fungus Heterobasidion parviporum Niemelä & Korhonen. The fungi spread through the air by basidiospores that can infect exposed fresh wood tissue on stumps or wounds, or by direct root contact (Garbelotto & Gonthier, Citation2013). It usually affects the lower and most valuable part of the tree stem, and the occurrence of rot in commercial timber substantially reduces its value. Economic losses in Norway alone are reported to be €18.5 million annually (Noordermeer et al., Citationin press).
Models for the prediction of rot occurrence based on empirical data have been developed in previous studies in Sweden (Thor et al., Citation2005), Finland (Mattila & Nuutinen, Citation2007), and Norway (Hylen & Granhus, Citation2018). These models predict the probability of rot at tree or stand level with explanatory variables describing climatic and edaphic conditions, forest structure, and development stages. The study of Hylen and Granhus (Citation2018) identified diameter at breast height (dbh), age, altitude, growing season temperature sum, and vegetation type as significant explanatory variables for the number of stems with rot observed at breast height. This model is, however, developed for use at national and regional scales, and its performance at an operational management level is currently unknown.
Wall-to-wall remotely sensed information has the potential to enable the mapping and monitoring of rot at an operational level (Lausch et al., Citation2016). In Norway spruce, rot has been shown to lead to defoliation (Žemaitis & Žemaitė, Citation2018), and close-range remote sensing has been used to detect differences in the crown condition in Scots pine affected by rot (Pitkänen et al., Citation2021). Recent research has also shown promising results in using remotely sensed data to detect attacks by bark beetles (Ips spp.) in Norway spruce (Abdullah et al., Citation2019; Bárta et al., Citation2021; Huo et al., Citation2021). These studies have shown that it is possible to detect differences in the spectral signatures of healthy and stressed trees, even in the early stages of an attack using satellite imagery.
Recent studies in Norway have used various sources of remotely sensed information coupled with ground reference data collected from cut-to-length harvesters to model and map rot on single-tree level (Allen et al., Citation2022a; Allen et al., Citation2022b; Dalponte et al., Citation2022a; Dalponte et al., Citation2022b) and management unit level (Räty et al., Citation2021). The work on single-tree level identified the classification of rot as a challenging task, with best results in terms of the kappa coefficient of 0.19–0.22 (Dalponte et al., Citation2022a) and 0.19–0.27 (Allen et al., Citation2022a). The relatively poor results were explained by the complexity of the problem and the fact that the visual signs of heartwood rot are not detectable to the human eye. Although there are differences in the spectral signatures of healthy and infected trees, there is not enough information to separate the two classes (Dalponte et al., Citation2022a). Furthermore, the single-tree approach has the drawback of not detecting all trees in the remotely sensed data (Dalponte et al., Citation2015; Kandare et al., Citation2017).
Hence, the area-based approach to forest inventory and modeling, whereby the response is modeled on a fixed area, is preferred to avoid systematic errors caused by undetected trees (Coomes et al., Citation2017). Räty et al. (Citation2021) applied the area-based approach to model the volume of butt rot at the grid cell level and aggregated the predictions to the management unit level. Explanatory information included both environmental and remotely sensed variables. Additionally, information from the harvester was included in the model to analyze this information as a theoretical case. This included variables such as the harvested timber volume, number of harvested stems, quadratic mean dbh of harvested stems of Norway spruce, the difference between the 90th and 10th percentile of the dbh distribution, and the proportion of harvested timber volume of Norway spruce. The study identified the variables describing the maturity of the forest, such as tree height, timber volume, and dbh, as the most important predictor variables.
Encouraged by the promising results in using multi-temporal remotely sensed data from nanosatellites (Allen et al., Citation2022a), the area-based approach used by Räty et al. (Citation2021) was in the present study expanded by incorporating multi-temporal remotely sensed data from other high-resolution optical sensors. The recent results on the mapping of beetle attacks using data from the Sentinel satellites (Abdullah et al., Citation2019; Bárta et al., Citation2021; Huo et al., Citation2021) indicated the importance of the data for the detection of stressed trees. The analysis also included bi-temporal airborne laser scanning (ALS) due to the possible difference in crown density between healthy and infected trees (Pitkänen et al., Citation2021; Žemaitis & Žemaitė, Citation2018), and the ability of ALS to measure tree crown density (Popescu, Citation2007). Bi-temporal ALS can also indicate site index and to an extent soil properties (Noordermeer et al., Citation2018). Furthermore, rot volume as a response variable was supplemented with proportional responses of rot.
This study aimed to evaluate area-based approaches for estimating rot in Norway spruce trees using remotely sensed data. The specific objectives were to: (i) evaluate the use of multi-temporal remotely sensed data for modeling rot using the area-based approach, (ii) compare three modeling approaches; grid, stand, and grid-to-stand, (iii) assess the effect of using spatially close data, and (iv) evaluate the use of proportional model responses, percentage of trees with heartwood rot (occurrence) and percentage of rot volume to the total timber volume (severity), in addition to rot volume.
Materials and methods
Study area
The study area of 986 square kilometers was located in Etnedal, Nord-Aurdal, Sør-Aurdal, and Nordre Land municipalities in southeastern Norway (60°94”N,09°56”E, ). The area is characterized by steep terrain with an elevation of the harvested sites ranging from approximately 200 to 980 m above sea level. Norway spruce is the naturally dominant tree species in the area, especially on productive sites (i.e. sites with a high site index). Scots pine (Pinus sylvestris L.) and deciduous species, mainly birch (Betula pubescens Ehrh.), can be found in less productive sites or interspersed in spruce-dominated sites.
Harvester data
Ground reference data were collected from 46 harvesting operations using a single-grip Komatsu 931XC harvester equipped with a 230 H crane and a C144 harvester head, in the period August 2019 – November 2021. A total of 131,375 trees were harvested, 89% of which were Norway spruce, 6% Scots pine, and 5% deciduous, mainly birch. The harvester was fitted with a Septentrio AsteRx-U real-time kinematic Global Navigation Satellite System (GNSS) consisting of two antennas that logged positions and rotations at a rate of one second in the National Marine Electronics Association (NMEA) format. The locations of harvested trees were recorded with a planimetric accuracy of around 1 m using the positions and rotations obtained from the GNSS and the crane extension measured by sensor hardware (Noordermeer et al., Citation2021). The harvester production report files contained data on geographical coordinates, species and stem profile of harvested trees, and dimensions and assortments of individual logs.
In addition to their regular work related to harvesting and processing trees, the harvester operator visually assessed and recorded the presence or absence of heartwood rot on the butt ends of processed spruce logs. The harvester operator did not, however, assess rot on non-spruce logs.
Commercial height, total tree height, timber volume, and rot volume were computed for each tree. Commercial height was calculated as the height along the stem profile where the tree’s last log was bucked and total tree height was predicted using a taper model. For each log, the log volume was calculated from the stem profile recorded by the harvester. Timber volume was calculated using empirical single-tree volume functions that relied on total tree height and dbh as predictors (Braastad, Citation1966; Brantseg, Citation1967; Vestjordet, Citation1967), and rot volume was calculated in the following procedure: (i) logs for which the subsequent log (i.e. the next log higher up the tree stem) was also classified as having rot, the total volume of the log, calculated from the stem profile, was registered as rot and (ii) logs for which the subsequent log was classified as having no rot, the rot volume was calculated from the stem profile of the lower half of the log length.
A summary of the single-tree harvester data is shown in .
Table 1. Summary statistics (mean and range) of harvested trees.
Aggregation of harvester data
When conducting a forest inventory following the area-based approach, the inventory area is typically tessellated into grid cells of the exact size as the field sample plots. The use of harvester data as ground reference data provides substantial flexibility regarding plot and grid cell sizes, however, research results indicate that sizes between 200 and 400 m2 are most appropriate (Maltamo et al., Citation2019). Accordingly, and conforming to operational practices in Norwegian forest inventories, we used a grid cell size of 250 m2 when aggregating the harvester data. Thus, the study area was tessellated into grid cells and clipped by the boundary extent of harvested areas, creating a total of 10,304 cells. Cells with an area <100 m2 or with a spruce proportion < 70% were removed from the dataset. Furthermore, the harvester data were aggregated to the stands defined in the forest management plan. The stands were clipped by the boundary extent of harvested areas. A total of 562 stands were assigned harvester data. However, if the cells with aggregated harvester data were in sum less than 0.2 ha at stand level, the cells were discarded. After applying these filtering criteria, 3715 cells and 109 stands were used in the analysis.
For each grid cell, timber volume and three measures of rot were calculated: rot occurrence, rot severity, and rot volume. Rot occurrence was calculated as the percentage of trees with heartwood rot, rot severity as the percentage of rot volume relative to the total timber volume, and rot volume as the timber volume (m3/ha) with heartwood rot. Formulas used to obtain rot occurrence (eq. 4), rot severity (eq. 3), rot volume (eq. 2), and timber volume (eq. 1) on grid and stand level are shown below where and
are summed rot volume and timber volume for tree
,
is a binary identifier for rot (0 for trees with no rot, 1 for trees with rot) in tree
and
refers to the number of trees in the cell.
To calculate the dominant height (Hdom) for each grid cell, the number of trees to be used in the calculation (Ndom) was determined by dividing the area of the cell in square meters by 100, conforming to the criteria of the 100 largest trees per ha. Ndom was then rounded up to the nearest integer and this number was used to select the n trees with the largest dbh. Hdom was then calculated as the weighted mean of these selected trees, where all but the last tree were assigned a weight of 1, and the last tree was assigned the remainder obtained by subtracting Ndom from the rounded-down nearest integer of Ndom as its weight.
shows the statistics of the filtered and aggregated harvester data. The means of properties were quite similar for the different aggregation methods, while the variation was larger for the grid data. The correlation between the rot variables (rot occurrence, rot severity, rot volume) and timber volume was assessed (). Rot occurrence and rot severity both had a weak correlation with timber volume. For the grid and stand data, the correlation coefficients were respectively 0.2 and 0.3 for rot occurrence and 0.1 and 0 for rot severity. Rot volume had a moderate correlation with timber volume of 0.5 and 0.6 for the grid and stand data, respectively.
Table 2. Summary of aggregated harvester data at grid and stand level.
Remotely sensed and environmental data
Optical data
Optical satellite images were collected for 2017 and 2018 from Sentinel-2 and the Planet Dove constellation satellites (Planet Team, Citation2017). Sentinel-2 images have 10 bands with a spatial resolution of 10 m or 20 m while Dove images are of a higher resolution than Sentinel-2 at 3 m, but only contain four bands.
Monthly image composites were generated for Sentinel-2 and Dove. Sentinel-2 image composites were generated in Google Earth Engine, taking the median value of each pixel, considering only shadow-free and cloud-free ones. Analytic Ortho Dove images were collected, corrected to surface reflectance and harmonized to Sentinel-2 to reduce scene-to-scene variability (Kington & Collison, Citation2022) using Planet’s Data API. Composites for Dove were generated using the R packages terra (Hijmans, Citation2022) and RStoolbox (Leutner et al., Citation2022). Images were co-registered before generating composites by taking the median value of each pixel not affected by cloud, haze, shadow, and/or snow. Due to high cloud cover, some months contained cells with missing data. Months in which more than 10% of the cells had missing data were removed. Specifically, this included the months of April, July, September, and October of 2017 and April, September, and October of 2018.
For each of the monthly image composites from Sentinel-2 and Dove, vegetation indices (VIs) were computed. VIs were calculated using the RStoolbox R package (Leutner et al., Citation2022). In total, 28 VIs were computed from the Sentinel-2 composites and 16 from Dove (). VIs were computed for Sentinel-2 over seven months and Dove over eight months, resulting in a total of 196 and 128 computed variables, respectively.
Table 3. Overview of vegetation indices computed for Sentinel-2 (S2) and Planet Dove (Dove).
Airborne lasscanning data
Two sets of ALS data were prepared for the study area. The first set (T1) was collected in 2007 with a point density of 0.7 points per m2, while the second set (T2) consisted of data from several acquisitions covering different parts of the study area in the years 2013–2017. The point density in these acquisitions was either two or five points per m2.
ALS data were processed with the lidR package (Roussel & Auty, Citation2022) in R. Point cloud normalization was performed using a triangulated irregular network (TIN) created from echoes classified as ground. For each echo, the height was normalized by subtracting the interpolated height of the TIN.
For each grid cell, canopy height variables including percentiles at 10% intervals (H10, H20, … , H90) were calculated from laser echoes above a threshold of 2 m above the ground. In addition, the mean height (Hmean), standard deviation (Hsd), coefficient of variation (Hcv), kurtosis (Hkurt), and skewness (Hskewness) were calculated for echoes above the threshold of 2 m. Canopy density variables were calculated by first dividing the range between the 95% height percentile and the 2 m threshold into ten vertical layers of equal height. The proportion of echoes above each layer to the total number of echoes was calculated, resulting in ten canopy density metrics (D0, D1, … , D9). ALS metrics were calculated for T1 and T2, as well as the differences between corresponding T1 and T2 metrics. Since the collection of ALS data for T2 was spread over several years (2013–2017) these differences were calculated as the relative change per year. The ALS metrics are hereafter referred to as ALS variables.
Environmental data
Forest management plans are typically revised every 10–15 years for the majority of the productive forest area in Norway. The plans provide stand-level information and are usually produced using a combination of remotely sensed information and field surveys. We collected two variables obtained from manual photo interpretation, i.e. site index and age, from the forest management plan covering the study area. Some grid cells intersected multiple stands, in which case the values of the stand with the largest overlap were selected.
A water table model referred to as the depth to water (DTW) is available at a national level (Hoffmann et al., Citation2022). The mean value in centimeters from the soil surface to the water table was extracted for each grid cell. Furthermore, ALS data from T1 were used to create a digital terrain model (DTM) with one-meter resolution using a TIN. The R package Terra (Hijmans, Citation2022) was then used to calculate slope, aspect, terrain ruggedness index (TRI), topographic position index (TPI), roughness, and flow direction from the DTM. In addition, the height above sea level was extracted from the DTM, and mean values were extracted for each cell.
Modeling and validation
The complete dataset contained all VIs computed from Sentinel-2 and Dove in the years 2017 and 2018 (324 variables), ALS variables from T2 and the ALS difference variables (48 variables), environmental variables (8 variables), and variables collected from the forest management plan (2 variables). The variables were divided into four different subsets of predictor variables: all variables (all), only ALS variables (als), only VIs variables (optical), and only environmental variables (env).
Three approaches to modeling were used for the aggregated data. In the grid and stand approach, predictor and response variables (rot occurrence, rot severity, rot volume, and timber volume) aggregated to the respective levels were used for training and validating models. The last approach was a grid-to-stand approach where the models were first trained at grid cell-level. CV predictions and observed values at grid cell-level were then averaged to the respective stands weighted by the area of the grid cells to create estimates at stand-level. These stand-level values were then used to validate the model.
A model was created for each combination of the four response variables and the four subsets of predictor variables, each model was fitted with and without outlier removal for grid data, totaling 32 models for grid-level and 16 models for stand-level. All models’ performance was assessed with random cross-validation (CV) and spatial CV. An overview of the data sources and modeling steps is shown in .
Regression method
The random forest algorithm has become a popular and widely used algorithm in both classification and regression problems (Biau & Scornet, Citation2016). The algorithm presented by Breiman (Citation2001) can handle datasets with a large number of variables, non-linearity, and at the same time having few parameters that require tuning. Several random forest implementations are available, of which we used one of the R package ranger (Wright & Ziegler, Citation2017) for computational efficiency.
Preliminary modeling using the R package tuneRanger (Probst et al., Citation2019) showed that hyperparameter tuning had little effect on model performance. Therefore, the hyperparameters were fixed to their default values: Number of trees to 500, minimum node size to five, and mtry to the largest integer value, not greater than or equal to the square root of the number of predictor variables. Impurity importance was used for estimating variable importance measures. Variable importance measures were averaged across the folds used in the validation and then scaled according to the greatest value on a scale from 0 to 1.
Outlier removal
To improve model robustness, outlier removal was attempted to remove grid cells where harvester data and ALS data did not match. Outlier removal was only applied to the grid dataset as the inaccuracy of harvester data would have little effect on the stand dataset. A linear model with Hdom as the response and the H90 ALS variable from T2 as the predictor was fitted to the harvester grid data. Observations with a Cook’s distance higher than 0.5 of the mean Cook’s distance were flagged as outliers. These observations were removed from the training data but not from the validation data. Modeling was performed with and without outlier removal.
Validation
Two validation methods were used for assessing model performance. The first method was random k-fold CV, by which observations were randomly assigned to folds. The second validation method was spatial clustering CV, by which spatially close samples were assigned to the same folds. Clusters based on the distances between observations were created with k-means clustering. The k in the k-fold CV was set to 10 for all datasets. For the spatial CV, the average distance between folds was 13.0 km for the grid approach and 11.4 km for the stand approach, with minimum and maximum distances of 1.4 km and 28.0 km for grid, and 0.5 km and 24.1 km for stand.
Machine learning models applied on spatially dependent datasets have been shown to overestimate performance when random CV is used compared to spatial CV (Meyer et al., Citation2018, Citation2019). By including both random and spatial CV we can assess the effects of spatially close training data.
The results were evaluated using root mean squared error (RMSE), relative root mean squared error (RMSE%), pseudo coefficient of determination (pR2) calculated as the squared correlation between observed and predicted values, and mean difference (MD). Additionally, a fuzzy set evaluation, as proposed by Gopal and Woodcock (Citation1994), was used on a scale from five to one to complement the preceding metrics. The fuzzy set score from five to one gives a percentage-wise distribution of the deviation between the predicted value and the observed value, corresponding to ≤ 10%, ≤20%, ≤30%, ≤40%, and > 40%, respectively. This can be helpful in operational forest management by assessing the model performances in terms of deviation from the true value within a range, eg., 0–20%. For each response variable, a null-model was created. Null-models for rot severity and rot occurrence used the average value of the response as a constant estimate, while rot volume was estimated from true timber volume and average rot severity.
Formulas for RMSE, RMSE%, pR2, and MD are shown in formulas 5–8 where and
is the observed and predicted values of the response variable for cell
,
is the observed mean of the response variable and
is the number of cells.
Results
Performance in terms of pR2 are shown in for all models. Random CV led to higher performances compared to the spatial CV for all approaches. The drop in performance, from random- to spatial CV, was reduced using ALS variables as predictors for timber volume- and rot volume models.
The effect of outlier removal depended on the validation method. Results using the random CV, showed reduced performance for every model evaluated. Applying the spatial CV, the effect of outlier removal was small. However, it improved the results for some rot volume and rot severity models.
Performance metrics for the best-performing models for each response and modeling approach are shown in . Of the rot responses, the rot volume models achieved the highest pR2 values for all approaches with 0.12, 0.14, and 0.37 and RMSE-values of 22.4, 9.9, and 11.4 using spatial CV for grid-, stand- and grid-to-stand approach, respectively. The RMSEs for the grid approach were clearly larger than the other two, while the difference between stand and grid-to-stand was smaller. The performance of rot occurrence and rot severity models was low for all approaches. The pR2 of the rot severity models for all approaches ranged from 0.00 to 0.01 with spatial CV. Rot occurrence models performed better for the grid and grid-to-stand approach with pR2 of 0.06 and 0.16, and RMSE-values of 15.0 and 7.7, respectively, while the stand approach had pR2 of 0.03 and RMSE of 6.6.
Table 4. Performance metrics for best-performing models.
The predicted versus observed values for spatial CV of the best-performing models are shown in . Timber volume models performed well, although they underpredicted observations with large volumes. The predictions for rot models were centered around the mean, underestimating small values and overestimating large ones.
Variable importance plots are shown in . The best-performing models for rot occurrence and rot volume models are dependent on ALS variables. The variable Hsd and height percentiles are assigned high importance. The importance of ALS variables is lower for rot severity models and is dependent on the approach. The grid approach assigned high importance to environmental and optical variables, while the stand approach only selected environmental variables.
The timber volume models showed substantially better performance than any of the rot models, with pR2-values of 0.62, 0.66, and 0.80 for grid-, stand- and grid-to-stand approaches, respectively, using spatial CV. The drop in performance between validation methods was small compared to any of the rot measures when ALS variables were included as predictors. Height percentiles and Hmean from the ALS variables scored high on variable importance for models.
The fuzzy set evaluation scores show that, using spatial CV, 65% of the timber volume predictions were within ± 30% of the true value on grid level and 85% at stand level using the grid-to-stand aggregation approach. For rot occurrence, rot severity, and rot volume the comparable values were 26%, 22%, and 18% at grid level, respectively, and 47%, 41%, and 34% at the stand level.
Comparing the results from the null-models in with the best regression models (), the RMSEs were reduced by all regression models using random CV, with the exception of rot volume models. For rot occurrence and rot severity the RMSEs were reduced by 11% and 5% respectively on grid level and 8% and 9% on stand level. The difference in RMSEs was small on grid level (0%) but 14% lower on stand level for rot volume. For spatial CV, the RMSE were reduced with 2% for rot occurrence at grid cell level, while for rot severity and rot volume the RMSEs increased with 3% and 11%. At the stand level, there was no difference for rot occurrence while the RMSEs of rot severity and rot volume increased with 5% and 22% respectively.
Table 5. Null-model results. Average values of response used for prediction.
Discussion
By using an area-based approach combined with multi-temporal data from high-resolution optical sensors, we were not able to build a robust model for the estimation of rot. With spatial CV the relative responses performed poorly with pR2 of rot severity ranging from 0.00 to 0.01 and rot occurrence ranging from 0.03 to 0.16. Rot volume models performed better than the relative responses with pR2 ranging from 0.12 to 0.37.
The poor performance of the relative measures of rot may have been caused by a lack of predictive power of the used predictor variables. The classification of rot at single-tree level has been shown to be challenging when using multi-temporal imagery (Dalponte et al., Citation2022b) and hyperspectral imagery (Allen et al., Citation2022a; Allen et al., Citation2022b; Dalponte et al., Citation2022a). Žemaitis and Žemaitė (Citation2018) found significant differences in crown defoliation between trees with and without rot damage, but noted that almost all of the morphological indicators were non-specific. Thus, despite the existence of detectable differences between trees with and without rot, other stressors may hinder our ability to detect these differences using remote sensing. The effect of such stressors may be augmented in the present study, which was conducted at the spatial scale of grid cells and stands as opposed to smaller individual trees in the mentioned studies.
Although rot volume was the best-performing response of rot, its correlation with timber volume may cause it to be misleading. In practice, estimating rot volume involves two parts: estimating timber volume and estimating rot severity (i.e. the extent to which the timber is damaged by rot). The poor performance of the rot severity models indicated that our rot volume models were mainly driven by volume estimation rather than the estimation of rot. In this study, both rot volume and rot occurrence models shared the high importance of height percentiles with timber volume models, further highlighting the link with timber volume. Contrary to Räty et al. (Citation2021) however, our rot volume models did not outperform the null-models for rot volume.
Interestingly, ALS variables such as Hsd and Hkurt were assigned high importance for both rot occurrence, rot severity, and rot volume, but not for the timber volume models. Räty et al. (Citation2021) also found high importance of Hvar for their rot volume models. Hsd can be associated with stand density, stand variation, or used for identifying thinning, although the low performance of spatial CV makes it likely to be a result of spatial overfit. Rot severity had the weakest correlation with timber volume and was assigned high importance to environmental, optical, and non-volume-related ALS variables.
Compared to rot occurrence or rot severity, rot volume is less useful for making decisions on forest management due to the correlation to timber volume. If the models cannot differentiate between stands with similar volumes, they also cannot assist in prioritizing. Comparing the null-models and the rot volume models shows that using the true volume and the average rot severity result in a pR2 of 0.41 compared to a rot volume model which resulted in a pR2 of 0.37. We therefore suggest that future studies should, in addition to rot volume, include rot occurrence or rot severity, as both are suitable alternatives that are useful in decision-making.
Dalponte et al. (Citation2022b) found that classification accuracy increased when only individual tree crowns with an area greater than 9 m2 were included instead of all individual tree crowns. This was in part explained by the more representative spectral information, avoiding a mixed spectral signature from multiple trees within a satellite pixel. Aggregating harvester data to larger units such as grid cells or stands could result in a loss of representativeness of the data, especially for larger units. Since the effect of rot on the vigor of a tree is quite low at the individual tree level, aggregating data to large units may obscure any response present at the tree level. When predicting rot at the stand level, factors that explain the risk of rot may be more important than detecting the presence of rot. This would explain the shift from predominantly optical variables to exclusively environmental variables when predicting rot severity using the grid- and stand approach, respectively. The stronger correlation with timber volume may obscure this effect for rot occurrence.
Spatial overfitting was a significant issue for all models estimating rot, as indicated by the drop in performance with spatial CV. Similarly, Räty et al. (Citation2021) reported a drop in performance from a pR2 of 0.60 to 0.37 for rot volume models when using spatial CV. They also found high variable importance measures for the coordinates of stands. However, we observed a substantial improvement in model performance for random CV of all rot responses when using the grid-to-stand approach, and the performance was better than the stand approach. This improvement could be attributed to the use of spatially close training data, which could potentially be implemented at the operational management level, as local data from harvesters with accurate positioning systems are becoming more widely available.
To our knowledge, the only comparative study is that of Räty et al. (Citation2021), which used an approach similar to our stand approach to predict rot volume. They reported values of RMSE, RMSE%, and pR2 of 15.6, 65.4, and 0.37, respectively, for their spatial CV. The results were similar to ours, and their increased RMSE and pR2 may partially be explained by the larger spread in rot volume and rot severity. Based on their variable importance plots, it is unlikely that their model would be able to describe rot severity better, as important variables are related to the volume or position of stands. The study of Räty et al. (Citation2021) contains observations covering a broader geographical area of Norway than the present study. The high importance of stand position might indicate that their model can describe differences in rot between regions but not on a local scale.
Although the study area in this study is quite large, it is limited in the range of environmental variables compared to the study of Hylen and Granhus (Citation2018) who identified growing season temperature sum, altitude, and age as important predictor variables. In the present study, data was only collected from mature and harvested stands, and the models might not apply to younger or older forests.
The accuracies of the volume models, with values of RMSE of 38% and 22% at grid- and stand level, respectively, were found to be similar or somewhat smaller in comparison to earlier studies using ALS and harvester data for estimating timber volumes. For instance, Hauglin et al. (Citation2018) reported a relative RMSE of 20% and 32% at high and medium productivity sites, respectively, using a grid cell size of 400 m2. In comparison, Maltamo et al. (Citation2019) reported a relative RMSE of 33% at a grid cell size of 200 m2 and only 9% at stand level.
The results presented in this study may be partially affected by errors in the harvester data, as discussed by Hauglin et al. (Citation2018). The mentioned study noted that errors in tree positions could result in co-registration errors between harvester and ALS data. This impact may be expected to be greater at grid cell level due to the smaller area and number of trees compared to the stand level. Trees that were not harvested and therefore not registered in the data, such as retention trees, could lead to an underestimation of the actual volume. Additionally, some trees may be too large for the harvester head to process, and therefore manually felled and thus missing in the harvester data. However, the small effect of the outlier removal indicated that errors in the harvester data had little impact on the models. Moreover, the point density of the ALS acquisitions varied between two and five echoes per m2, and the time of acquisition ranged from two to eight years before harvest. Previous studies have shown that the use of different sensors and pulse densities could significantly affect the height, density, and intensity variables calculated from ALS data, however, the impact on the precision forest attributes, such as volume and tree height has been shown to be minor (Næsset, Citation2009; Ørka et al., Citation2010). While Noordermeer et al. (Citation2022) found no significant issues when using data from multiple ALS acquisitions to model timber volume, it remains unclear how potential sensor effects could impact the utility of ALS data for modeling rot properties. However, using multiple ALS acquisitions could add additional noise to the variables used for modeling rot properties, and a more uniform dataset may therefore provide better results. Furthermore, an eight-year time difference could have significant effects due to changes in both timber volume and rot.
Constellations of nanosatellites, such as Planet Dove provide imagery of high spatial and temporal resolutions but suffers in geometric and radiometric lower quality compared to Sentinel-2 (Frazier & Hemingway, Citation2021). Radiometric inconsistencies between images, geometric errors, and unusable data missed by the provided mask, e.g. undetected clouds, will all introduce noise in the data. The applied harmonization tool, the co-registration of images, and the aggregation of pixels to a higher level (grid or stand) should reduce noise introduced from these sources. Lower image quality, wide overlapping bands of the Dove Classic sensor (Collison et al., Citation2021), and the lack of bands can explain the weaker performance of Dove imagery compared to Sentinel-2. Using newer generations of Planet’s Dove satellites and improved pre-processing methods of imagery could be applied in future studies to improve the performance.
Forest management activities can have large effects on the infection risk and spread of H. parviporum (Garbelotto & Gonthier, Citation2013; Pukkala et al., Citation2005), and the fungus can remain active in infected stumps for decades (Greig & Pratt, Citation1976). Thus, records of management history, or techniques to derive previous activities, could be a viable route for future studies to improve the performance of rot models.
Conclusions
In this study, we evaluated area-based methods for estimating rot in Norway spruce trees using remotely sensed data. Models for rot volume, rot severity, rot occurrence, and timber volume were developed using stem rot data collected by a cut-to-length harvester and multi-temporal remotely sensed data from the Sentinel-2 and Planet Dove constellation satellites and ALS. The models’ performance for timber volume was consistent with previous studies. However, for rot responses, the models showed relatively poor performance, with RMSEs of 91%, 112%, and 119% at the grid cell level, for rot occurrence, rot severity, and rot volume, respectively. At the stand level, the best results using spatial CV were 46%, 51%, and 65% RMSE. The only comparative study known to us by Räty et al. (Citation2021) reported an RMSE of 65% for rot volume using a similar approach.
The variable importance analysis showed that ALS was the most important source of information for both rot occurrence and volume. For rot severity at the grid cell level, the models also included optical data from the two satellite sources and environmental information. At the stand level, the models were dominated by environmental variables.
Including spatially close data in training improved the performance of models. The results showed reduced accuracy when predicting new areas and improved when observations in nearby stands or within stands were allowed in the training data.
Compared to estimates based solely on the average values of rot, the models only slightly improved the estimates, and only for rot volume. This effect can be explained by the positive correlation between timber volume and rot volume, and the fact that ALS data has proven to be a valuable data source for timber volume estimation. For responses less correlated to timber volume, such as rot severity and occurrence, the results indicated that the models were less useful.
Author contributions
Conceptualization: E.H., J.W., M.D., T.G., H.O.; Methodology: J.W., M.D., T.G., H.O.; Formal analysis: E.H., J.W.; Investigation: E.H., J.W.; Data Curation: J.W., M.D., T.G., L.N., H.O.; Writing – original draft: E.H., J.W.; Writing – review & editing: E.H., J.W., M.D., T.G., L.N., H.O.; Visualization: J.W.; Supervision: T.G., H.O.; Project administration: T.G., H.O.; Funding acquisition: T.G., H.O.
Acknowledgments
We would like to thank Valdres Skog AS for collecting and sharing the harvester data and Planet Labs Inc. for providing the images used in this study under the “Education and Research Program”. Furthermore, we are grateful for the constructive and valuable comments from three anonymous reviewers on previous versions of this study.
Data availability statement
The data that support the findings of this study are owned by Valdres Skog AS. Restrictions apply to the availability of these data, which were used under license for this study. Data are available upon reasonable request from the corresponding author, [H.O.Ø.], with the permission of Valdres Skog AS.
Disclosure statement
No potential conflict of interest was reported by the authors.
Additional information
Funding
References
- Abdullah, H., Skidmore, A. K., Darvishzadeh, R., Heurich, M., Pettorelli, N., & Disney, M. (2019). Sentinel-2 accurately maps green-attack stage of European spruce bark beetle (Ips typographus L.) compared with Landsat-8. Remote Sensing in Ecology and Conservation, 5(1), 87–16. https://doi.org/10.1002/rse2.93
- Allen, B., Dalponte, M., Hietala, A., Ørka, H. O., Næsset, E., & Gobakken, T. (2022a). Detection of root, butt, and stem rot presence in Norway spruce with hyperspectral imagery. Silva Fennica, 56(2). https://doi.org/10.14214/sf.10606
- Allen, B., Dalponte, M., Ørka, H. O., Næsset, E., Puliti, S., Astrup, R., & Gobakken, T. (2022b). UAV-based hyperspectral imagery for detection of root, butt, and stem rot in Norway Spruce. Remote Sensing, 14(15), 16. https://doi.org/10.3390/rs14153830
- Baret, F., & Guyot, G. (1991). Potentials and limits of vegetation indices for LAI and APAR assessment. Remote Sensing of Environment, 35(2), 161–173. https://doi.org/10.1016/0034-4257(91)90009-U
- Barnes, E. M., Clarke, T. R., Richards, S. E., Colaizzi, P. D., Haberland, J., Kostrzewski, M., Waller, P., Choi, C., Riley, E., Thompson, T., Lascano, R. J., Li, H., Moran, M. S. (2000). Coincident detection of crop water stress, nitrogen status and canopy density using ground based multispectral data. Proceedings of the Fifth International Conference on Precision Agriculture, Bloomington, MN, USA, 1619, 6.
- Bárta, V., Lukeš, P., & Homolová, L. (2021). Early detection of bark beetle infestation in Norway spruce forests of Central Europe using Sentinel-2. International Journal of Applied Earth Observation and Geoinformation, 100, 102335. https://doi.org/10.1016/j.jag.2021.102335
- Biau, G., & Scornet, E. (2016). A random forest guided tour. Test, 25(2), 197–227. https://doi.org/10.1007/s11749-016-0481-7
- Birth, G. S., & McVey, G. R. (1968). Measuring the color of growing turf with a reflectance spectrophotometer 1. Agronomy Journal, 60(6), 640–643. https://doi.org/10.2134/agronj1968.00021962006000060016x
- Braastad, H. (1966). Volume tables for birch. Meddelelser Fra Det Norske Skogforsøksvesen, 21, 265–365. https://hdl.handle.net/11250/2988596
- Brantseg, A. (1967). Volume functions and tables for scots pine South Norway. Meddelelser Fra Det Norske Skogforsøksvesen, 22, 695–739. https://hdl.handle.net/11250/2988611
- Breiman, L. (2001). Random forests. Machine Learning, 45(1), 5–32. https://doi.org/10.1023/A:1010933404324
- Camps-Valls, G., Campos-Taberner, M., Moreno-Martínez, Á., Walther, S., Duveiller, G., Cescatti, A., Mahecha, M. D., Muñoz-Marí, J., García-Haro, F. J., Guanter, L., Jung, M., Gamon, J. A., Reichstein, M., & Running, S. W. (2021). A unified vegetation index for quantifying the terrestrial biosphere. Science Advances, 7(9). https://doi.org/10.1126/sciadv.abc7447
- Collison, A., Jumpasut, A., & Bourne, H. (2021). On-Orbit Radiometric Calibration of the planet satellite fleet. https://assets.planet.com/docs/radiometric_calibration_white_paper.pdf
- Coomes, D. A., Dalponte, M., Jucker, T., Asner, G. P., Banin, L. F., Burslem, D. F. R. P., Lewis, S. L., Nilus, R., Phillips, O. L., Phua, M.-H., & Qie, L. (2017). Area-based vs tree-centric approaches to mapping forest carbon in Southeast Asian forests from airborne laser scanning data. Remote Sensing of Environment, 194, 77–88. https://doi.org/10.1016/j.rse.2017.03.017
- Dalponte, M., Kallio, A. J. I., Ørka, H. O., Næsset, E., & Gobakken, T. (2022a). Wood decay detection in Norway spruce forests based on airborne hyperspectral and ALS data. Remote Sensing, 14(8), 15. https://doi.org/10.3390/rs14081892
- Dalponte, M., Reyes, F., Kandare, K., & Gianelle, D. (2015). Delineation of individual tree crowns from ALS and hyperspectral data: A comparison among four methods. European Journal of Remote Sensing, 48(1), 365–382. https://doi.org/10.5721/EuJRS20154821
- Dalponte, M., Solano-Correa, Y. T., Ørka, H. O., Gobakken, T., & Næsset, E. (2022b). Detection of heartwood rot in Norway spruce trees with lidar and multi-temporal satellite data. International Journal of Applied Earth Observation and Geoinformation, 109, 11. https://doi.org/10.1016/j.jag.2022.102790
- Dash, J., & Curran, P. J. (2004). The MERIS terrestrial chlorophyll index. International Journal of Remote Sensing, 25(23), 5403–5413. https://doi.org/10.1080/0143116042000274015
- Deering, D. W. (1975, October 6).Measuring forage production of grazing units from Landsat MSS data. Proceedings of 10th International Symposium on Remote Sensing of Environment, Ann Arbor, MI, USA, 1975, 1169–1178.
- Frazier, A. E., & Hemingway, B. L. (2021). A technical review of planet smallsat data: practical considerations for processing and using PlanetScope imagery. Remote Sensing, 13(19), 3930. https://doi.org/10.3390/rs13193930
- Gao, B.-C. (1996). NDWI—A normalized difference water index for remote sensing of vegetation liquid water from space. Remote Sensing of Environment, 58(3), 257–266. https://doi.org/10.1016/S0034-42579600067-3
- Garbelotto, M., & Gonthier, P. (2013). Biology, epidemiology, and control of Heterobasidion species worldwide. Annual Review of Phytopathology, 51(1), 39–59. https://doi.org/10.1146/annurev-phyto-082712-102225
- Gitelson, A. A., Kaufman, Y. J., & Merzlyak, M. N. (1996). Use of a green channel in remote sensing of global vegetation from EOS-MODIS. Remote Sensing of Environment, 58(3), 289–298. https://doi.org/10.1016/S0034-42579600072-7
- Gitelson, A., & Merzlyak, M. N. (1994). Quantitative estimation of chlorophyll-a using reflectance spectra: Experiments with autumn chestnut and maple leaves. Journal of Photochemistry and Photobiology B, Biology, 22(3), 247–252. https://doi.org/10.1016/1011-13449306963-4
- Gitelson, A. A., Viña, A., Arkebauer, T. J., Rundquist, D. C., Keydan, G., & Leavitt, B. (2003). Remote estimation of leaf area index and green leaf biomass in maize canopies. Geophysical Research Letters, 30(5). https://doi.org/10.1029/2002gl016450
- Gopal, S., & Woodcock, C. (1994). Theory and methods for accuracy assessment of thematic maps using fuzzy sets. Photogrammetric Engineering and Remote Sensing, 60(2), 181–188. https://www.asprs.org/wp-content/uploads/pers/1994journal/feb/1994_feb_181-188.pdf
- Greig, B. J. W., & Pratt, J. E. (1976). Some observations on the longevity of Fomes annosus in conifer stumps. Forest Pathology, 6(4), 250–253. https://doi.org/10.1111/j.1439-0329.1976.tb00533.x
- Guyot, G., & Baret, F. (1988). Utilisation de la haute resolution spectrale pour suivre l’etat des couverts vegetaux. Spectral Signatures of Objects in Remote. https://adsabs.harvard.edu/full/1988ESASP.287.279G
- Hauglin, M., Hansen, E., Sørngård, E., Næsset, E., & Gobakken, T. (2018). Utilizing accurately positioned harvester data: Modelling forest volume with airborne laser scanning. Canadian Journal of Forest Research, 48(8), 913–922. https://doi.org/10.1139/cjfr-2017-0467
- Hijmans, R. J. (2022). Terra: spatial data analysis (Version 1.6-17) [Computer software]. https://CRAN.R-project.org/package=terra
- Hoffmann, S., Schönauer, M., Heppelmann, J., Asikainen, A., Cacot, E., Eberhard, B., Hasenauer, H., Ivanovs, J., Jaeger, D., Lazdins, A., Mohtashami, S., Moskalik, T., Nordfjell, T., Stereńczak, K., Talbot, B., Uusitalo, J., Vuillermoz, M., & Astrup, R. (2022). Trafficability prediction using depth-to-water maps: the status of application in Northern and Central European Forestry. Current Forestry Reports, 8(1), 55–71. https://doi.org/10.1007/s40725-021-00153-8
- Huete, A. R. (1988). A soil-adjusted vegetation index (SAVI). Remote Sensing of Environment, 25(3), 295–309. https://doi.org/10.1016/0034-42578890106-X
- Huo, L., Persson, H. J., & Lindberg, E. (2021). Early detection of forest stress from European spruce bark beetle attack, and a new vegetation index: Normalized distance red & SWIR (NDRS). Remote Sensing of Environment, 255, 112240. https://doi.org/10.1016/j.rse.2020.112240
- Huse, K. J., Solheim, H., & Venn, K. (1994). Råte i gran registrert på stubber etter hogst vinteren 1992 [Stump inventory of root and butt rots in Norway spruce cut in 1992]. Rapp Skogforsk, 23, 1–26. http://hdl.handle.net/11250/2560332
- Hylen, G., & Granhus, A. (2018). A probability model for root and butt rot in Picea abies derived from Norwegian national forest inventory data. Scandinavian Journal of Forest Research, 33(7), 657–667. https://doi.org/10.1080/02827581.2018.1487074
- Kandare, K., Dalponte, M., Ørka, H. O., Frizzera, L., & Næsset, E. (2017). Prediction of species-specific volume using different inventory approaches by fusing airborne laser scanning and hyperspectral data. Remote Sensing, 9(5), 19. https://doi.org/10.3390/rs9050400
- Kington, J., & Collison, A. (2022). Scene level normalization and harmonization of planet dove imagery. Planet Labs. https://assets.planet.com/docs/scene_level_normalization_of_planet_dove_imagery.pdf
- Lausch, A., Erasmi, S., King, D. J., Magdon, P., & Heurich, M. (2016). Understanding forest health with remote sensing -Part I—a review of spectral traits, processes and remote-sensing characteristics. Remote Sensing, 8(12), 1029. https://doi.org/10.3390/rs8121029
- Leutner, B., Horning, N., & Schwalb-Willmann, J. (2022). RStoolbox: tools for remote sensing data analysis (Version 0.3.0) [Computer software]. https://CRAN.R-project.org/package=RStoolbox
- Lozano-Garcia, D. F., Fernandez, R. N., & Johannsen, C. J. (1991). Assessment of regional biomass-soil relationships using vegetation indexes. IEEE Transactions on Geoscience and Remote Sensing: A Publication of the IEEE Geoscience and Remote Sensing Society, 29(2), 331–339. https://doi.org/10.1109/36.73676
- Lymburner, L., Beggs, P. J., & Jacobson, C. R. (2000). Estimation of canopy-average surface-specific leaf area using Landsat TM data. Photogrammetric engineering & remote sensing, 66. http://www.asprs.org/wp-content/uploads/pers/2000journal/february/2000_feb_183-191.pdf
- Maltamo, M., Hauglin, M., Næsset, E., & Gobakken, T. (2019). Estimating stand level stem diameter distribution utilizing harvester data and airborne laser scanning. Silva Fennica, 53(3), 19. https://doi.org/10.14214/sf.10075
- Marsett, R. C., Qi, J., Heilman, P., Biedenbender, S. H., Carolyn Watson, M., Amer, S., Weltz, M., Goodrich, D., & Marsett, R. (2006). Remote sensing for grassland management in the arid southwest. Rangeland Ecology & Management, 59(5), 530–540. https://doi.org/10.2111/05-201R.1
- Mattila, U., & Nuutinen, T. (2007). Assessing the incidence of butt rot in Norway spruce in southern Finland. Silva Fennica, 41(1), 29–43. https://doi.org/10.14214/sf.473
- McFeeters, S. K. (1996). The use of the Normalized Difference Water Index (NDWI) in the delineation of open water features. International Journal of Remote Sensing, 17(7), 1425–1432. https://doi.org/10.1080/01431169608948714
- Meyer, H., Reudenbach, C., Hengl, T., Katurji, M., & Nauss, T. (2018). Improving performance of spatio-temporal machine learning models using forward feature selection and target-oriented validation. Environmental Modelling & Software, 101, 1–9. https://doi.org/10.1016/j.envsoft.2017.12.001
- Meyer, H., Reudenbach, C., Wöllauer, S., & Nauss, T. (2019). Importance of spatial predictor variable selection in machine learning applications – Moving from data reproduction to spatial prediction. Ecological Modelling, 411, 11. https://doi.org/10.1016/j.ecolmodel.2019.108815
- Möykkynen, T., & Pukkala, T. (2010). Optimizing the management of Norway spruce and Scots pine mixtures on a site infected by Heterobasidion coll. Scandinavian Journal of Forest Research, 25(2), 127–137. https://doi.org/10.1080/02827581003667322
- Næsset, E. (2009). Effects of different sensors, flying altitudes, and pulse repetition frequencies on forest canopy metrics and biophysical stand properties derived from small-footprint airborne laser data. Remote Sensing of Environment, 113(1), 148–159. https://doi.org/10.1016/j.rse.2008.09.001
- Nagler, P. L., Daughtry, C. S. T., & Goward, S. N. (2000). Plant Litter and Soil Reflectance. Remote Sensing of Environment, 71(2), 207–215. https://doi.org/10.1016/S0034-42579900082-6
- Noordermeer, L., Bollandsås, O. M., Gobakken, T., & Næsset, E. (2018). Direct and indirect site index determination for Norway spruce and Scots pine using bitemporal airborne laser scanner data. Forest Ecology and Management, 428, 104–114. https://doi.org/10.1016/j.foreco.2018.06.041
- Noordermeer, L., Korpunen, H., Berg, S., Gobakken, T., & Astrup, R. (In press). Economic losses caused by butt rot in Norway spruce trees in Norway. Scandinavian Journal of Forest Research.
- Noordermeer, L., Næsset, E., & Gobakken, T. (2022). Effects of harvester positioning errors on merchantable timber volume predicted and estimated from airborne laser scanner data in mature Norway spruce forests. Silva Fennica, 56(1). https://doi.org/10.14214/sf.10608
- Noordermeer, L., Sørngård, E., Astrup, R., Næsset, E., & Gobakken, T. (2021). Coupling a differential global navigation satellite system to a cut-to-length harvester operating system enables precise positioning of harvested trees. International Journal of Forest Engineering, 32(2), 119–127. https://doi.org/10.1080/14942119.2021.1899686
- Ørka, H. O., Næsset, E., & Bollandsås, O. M. (2010). Effects of different sensors and leaf-on and leaf-off canopy conditions on echo distributions and individual tree properties derived from airborne laser scanning. Remote Sensing of Environment, 114(7), 1445–1461. https://doi.org/10.1016/j.rse.2010.01.024
- Perry, C. R., & Lautenschlager, L. F. (1984). Functional equivalence of spectral vegetation indices. Remote Sensing of Environment, 14(1–3), 169–182. https://doi.org/10.1016/0034-4257(84)90013-0
- Pinty, B., & Verstraete, M. M. (1992). GEMI: A non-linear index to monitor global vegetation from satellites. Vegetatio, 101(1), 15–20. https://doi.org/10.1007/BF00031911
- Pitkänen, T. P., Piri, T., Lehtonen, A., & Peltoniemi, M. (2021). Detecting structural changes induced by Heterobasidion root rot on Scots pines using terrestrial laser scanning. Forest Ecology and Management, 492, 119239. https://doi.org/10.1016/j.foreco.2021.119239
- Planet Team. (2017). Planet Application Program Interface: In Space for Life on Earth. Planet. https://api.planet.com
- Popescu, S. C. (2007). Estimating biomass of individual pine trees using airborne lidar. Biomass and Bioenergy, 31(9), 646–655. https://doi.org/10.1016/j.biombioe.2007.06.022
- Probst, P., Wright, M. N., & Boulesteix, A.-L. (2019). Hyperparameters and tuning strategies for random forest. Data Mining and Knowledge Discovery, 9(3), e1301. https://doi.org/10.1002/widm.1301
- Pukkala, T., Möykkynen, T., Thor, M., Rönnberg, J., & Stenlid, J. (2005). Modeling infection and spread of Heterobasidion annosum in even-aged Fennoscandian conifer stands. Journal Canadien de La Recherche Forestiere, 35(1), 74–84. https://doi.org/10.1139/x04-150
- Qi, J., Chehbouni, A., Huete, A. R., Kerr, Y. H., & Sorooshian, S. (1994). A modified soil adjusted vegetation index. Remote Sensing of Environment, 48(2), 119–126. https://doi.org/10.1016/0034-42579490134-1
- Räty, J., Breidenbach, J., Hauglin, M., & Astrup, R. (2021). Prediction of butt rot volume in Norway spruce forest stands using harvester, remotely sensed and environmental data. International Journal of Applied Earth Observation and Geoinformation, 105, 102624. https://doi.org/10.1016/j.jag.2021.102624
- Richardson, A. J., & Wiegand, C. L. (1977). Distinguishing vegetation from soil background information. Photogrammetric Engineering and Remote Sensing, 43(12), 1541–1552. https://www.asprs.org/wp-content/uploads/pers/1977journal/dec/1977_dec_1541-1552.pdf
- Rouse, J. W., Haas, R. H., Schell, J. A., Deering, D. W. (1974). Monitoring vegetation systems in the Great Plains with ERTS. NASA Special Publication, 351(1), 309. https://ntrs.nasa.gov/citations/19740022614
- Roussel, J.-R., & Auty, D. (2022). lidR: airborne lidar data manipulation and visualization for forestry applications (Version 4.0.1) [Computer software]. https://cran.r-project.org/package=lidR
- Seidl, R., Thom, D., Kautz, M., Martin Benito, D., Peltoniemi, M., Vacchiano, G., Wild, J., Ascoli, D., Petr, M., Honkaniemi, J., Lexer, M. J., Trotsiuk, V., Mairota, P., Svoboda, M., Fabrika, M., Nagel, T. A., & Reyer, C. P. O. (2017). Forest disturbances under climate change. Nature Climate Change, 7(6), 395–402. https://doi.org/10.1038/nclimate3303
- Stenlid, J., & Wästerlund, I. (1986). Estimating the frequency of stem rot in Picea abies using an increment borer. Scandinavian Journal of Forest Research, 1(1–4), 303–308. https://doi.org/10.1080/02827588609382421
- Susaeta, A., & Gong, P. (2019). Optimal harvest strategy for even‐aged stands with price uncertainty and risk of natural disturbances. Natural Resource Modeling, 32(3), 25. https://doi.org/10.1111/nrm.12211
- Thiam, A. K. (1998). Geographic information systems and remote sensing methods for assessing and monitoring land degradation in the Sahel region: The case of southern Mauritania. https://search.proquest.com/openview/484bdf270c849a8303198121f2efe075/1?pq-origsite=gscholar&cbl=18750&diss=y
- Thor, M., Ståhl, G., & Stenlid, J. (2005). Modelling root rot incidence in Sweden using tree, site and stand variables. Scandinavian Journal of Forest Research, 20(2), 165–176. https://doi.org/10.1080/02827580510008347
- Vestjordet. (1967). Functions and tables for volume of standing trees. Norway spruce. Meddelelser Fra Det Norske Skogforsøksvesen, 22, 545–574. https://hdl.handle.net/11250/2988611
- Wright, M. N., & Ziegler, A. (2017). Ranger: a fast implementation of random forests for high dimensional data in C++ and R. Journal of Statistical Software, 77(1), 1–17. https://doi.org/10.18637/jss.v077.i01
- Xu, H. (2006). Modification of normalised difference water index (NDWI) to enhance open water features in remotely sensed imagery. International Journal of Remote Sensing, 27(14), 3025–3033. https://doi.org/10.1080/01431160600589179
- Žemaitis, P., & Žemaitė, I. (2018). Does butt rot affect the crown condition of Norway spruce trees? Trees, 32(2), 489–495. https://doi.org/10.1007/s00468-017-1645-0