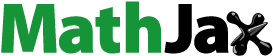
ABSTRACT
This study evaluates four satellite-based precipitation datasets with gauged rainfall observations at a daily and wet season time scales. Satellite Precipitation Estimators from Climate Hazards Group Infrared Precipitation Stations (CHIRPSv2), the Climate prediction center (CPC) morphing technique (CMORPH), the Integrated Multi-satellite Retrieval for GPM (IMERG-06) and Precipitation Estimation from Remotely Sensed Information using Artificial Neural Networks Cloud Classification System (PERSSIANN-CCS) were used. Categorical and continuous statistical techniques were applied to evaluate the detection and estimation ability of SPEs. Among the SPEs, CMORPH was superior, 0.90 and followed by IMERG-06, 0.82 in the Probability of detection (POD), Critical success index (CSI), 0.74 and 0.70 and Accuracy, 0.75 and 0.72 at wet season, respectively. The result of bias ratio showed underestimation for all SPEs at wet season and daily time scale. The comparison of SPEs in terms of RMSE indicated that IMERG-06 was better, 75.9 followed by CMORPH, 86.0. In terms of Relative bias (RBias), IMERG-06 was the second next to CHIRPSv2. On the other hand, IMERG-06 had better RMSE at JJAS and daily time scale. Overall, the findings of this evaluation study showed the capacity of IMERG-06 product could be reliable rainfall data sources for climate and hydrological analysis of central highlands of Abbay Basin.
Introduction
Examining the spatiotemporal dynamics of meteorological variables in the context of changing climate, particularly in countries where rain-fed agriculture is predominant, is vital to assess climate-induced variability and suggest viable adaptation strategies (Asfaw et al., Citation2018). Temporally and spatially direct, consistent and long-term ground-based observed rainfall data are generally accurate near the sensor location and important (Behrangi et al., Citation2011; Nguyen et al., Citation2018). However, the observed rainfall data are usually lacking of such quality and are subjected to missing data in almost all sub-Saharan Africa in general and Ethiopia in particular (Githungo et al., Citation2016; Kaspar et al., Citation2022; Kidd et al., Citation2012; Maggioni et al., Citation2016; Sisay et al., Citation2017). It may also be infeasible to install and maintain dense gauge-based stations in remote areas like mountains, deserts, forests and large water bodies (Dinku et al., Citation2018; Tapiador et al., Citation2012). That the availability of meteorological data gaps, especially in Southern and Eastern Africa, has hindered detailed assessments of the climate variability and change impact, challenging water resource management and hydrology applications (Bui et al., Citation2019; WMO, World Meteorological Organization, Citation2020).
Consequently, the application of remote sensing measurement techniques are preferred as it cover larger areas and provide rainfall data at high spatial and temporal scales with increasingly high resolution in areas where gauge-based rainfall measurements are impractical, sparse or nonexistent (Moges et al., Citation2022; Sahlaoui & Mordane, Citation2019). Although most SPEs had a good correlation with gauge-based observation since gauge information is integrated with the correction algorithm (Yilmaz et al., Citation2005), often lack direct evaluation at specific sites of interest or is plagued with uncertainties resulting from errors in measurement, sampling, retrieval algorithm, and bias correction processes (Hordofa et al., Citation2021; Wedajo et al., Citation2021). Further, local topography and climatic conditions can also affect the accuracy of SPE estimation (Bitew & Gebremichael, Citation2011). It means the performance of satellite-based dataset product capabilities varies in different climatic areas; there could be scale mismatches between area-averaged SPE data and point-like gauge-based measurements, which is the most critical drawback of SPE products, that it should be carefully evaluated and site-specific validation should be done before using satellite rainfall products for different applications (Belay et al., Citation2019).
Different studies have recommended SPEs for data scarce basin (Behrangi et al., Citation2011; Bitew & Gebremichael, Citation2011; Thiemig et al., Citation2013). However, there is no consensus regarding the “best” SPE product for different climatic regions. For instance, Ayehu et al. (Citation2018) reported that the performance of CHIRPS was very encouraging and significantly better than the other satellite rainfall products, TAMSAT and ARC2 over the Upper Blue Nile Basin (UBN). Similarly, Wedajo et al. (Citation2021) in their quantitative statistical index showed that the product of CHIRPS performed best in estimating and detecting rainfall events at monthly and annual timescales in Dhidhessa River Basin. Another study by Koukoula et al. (Citation2020) also showed CHIRPS exhibited the best performance in wet and dry basins and in obtaining the highest correlation when compared with TRMM in their study of evaluating the global water resources reanalysis products in the UBN. Furthermore, Taye et al. (Citation2020) confirmed that the performance of CHIRPSv2 for monthly rainfall estimates was better than MSWEPv2 with respect to ME and RMSE error metrics over the UBN basin. However, in another study by Belay et al. (Citation2019) who evaluated the application of multisource satellite rainfall products of CHIRPS in western margins of Ethiopian highlands, indicated that CHIRPS have shown a slight overestimation and underestimation of rainfall occurrence for the lowland and highland areas respectively. Similarly, a study by Rachdane et al. (Citation2022) did not select CHIRPS as the best estimator rather the GPM-F showed the most satisfactory results for all time scales and for spatial scales, than the CHIRPS and CCSCDR products at the monthly and annual scales, and by the GPM-E and GPM-L products at the daily scale. On the other hand, Hordofa et al. (Citation2021) who compared GPM-IMERG and CHIRPS, indicated that both estimators had a good agreement with the observed rainfall data at monthly and seasonal time scales but the CHIRPS products slightly outperformed the GPM-IMERG product.
Another study by Sahlu et al. (Citation2016) who evaluated the performance of high-resolution multi-satellite and reanalysis rainfall products over East Africa indicated that IMERG products have a better bias ratio and correlation coefficient, and showed a slight improvement in detecting rainfall events and avoiding false rainfall events on hourly and daily time scales compared to CMORPH. Similarly, a study by Retalis et al. (Citation2020) indicated a better performance of IMERG product than 3B43V7 with higher R-value, lower Bias values, and RBias values, and its better ability to adjust precipitation in comparison to GSMaP. While Ashenafi and Hailu (Citation2014) revealed that CMORPH has a potential to be used in water resource management and hydrologic prediction for ungagged catchments in the UBN even if it had the highest bias which is associated with the highest relative errors. Similarly, CMORPH was selected by Koukoula et al. (Citation2020) because of its NCRMSE and JULES values and best performance in the entire UBN basin. Furthermore, CMORPH was selected due to its best accuracy during wet season rainfall estimation. In terms of bias, POD, and CSI error metrics among TMPA, PERSIANN, ECMWF, ERA Interim reanalysis and MSWEP satellite-based rainfall products (Lakew et al., Citation2020; Sahlu et al., Citation2017). However, Hirpa et al. (Citation2010) indicated that the performance of CMORPH and PERSIANN depends on elevation; PERSIANN gives smaller precipitation estimates at higher elevations. Furthermore, these authors noted that PERSIANN provides considerably lower rainfall in comparison with CMORPH. It has been also confirmed by Sahlu et al. (Citation2017) that CMORPH products capture most of the rainy events better than TMPA and PERSIANN products. Furthermore, Derin et al. (Citation2016), and Hirpa et al. (Citation2010) justified that Infrared (IR-based) rainfall retrieval algorithms like CHIRPS have major limitations in complex topographic regions while Microwave (MW-based) rainfall retrieval algorithms like CMORPH are more physically based and free of cold surface of snow effects. In addition, Huffman et al. (Citation2020) also suggested that combining microwave and Infrared climate estimates can be a helpful approach that resulted in a high-resolution satellite precipitation algorithm.
Though the SPEs should be carefully evaluated and calibrated before using the products for any application, they can be a promising alternative precipitation data sources for climatic applications, and assessment of flood susubtability (Ruidas et al., Citation2022), especially for areas where ground-based observations are limited. However, existing satellite-based rainfall estimations are subjected to systematic biases. Previous studies indicated that estimating and detecting the performance of SPEs depend on spatial and temporal conditions (Belay et al., Citation2019; Lakew et al., Citation2020). Further, SPEs possess uncertainties resulting from errors in measurement, sampling, retrieval algorithm, and bias correction processes (Dinku et al., Citation2010; Gebremichael et al., Citation2014; Tong et al., Citation2014). Local topography and climatic conditions can also affect the accuracy of SPE estimation (Bitew & Gebremichael, Citation2011). To the best of our knowledge, the rainfall detection and estimation capability of SPEs (CHIRPs, CMORPH, IMERG-06, and PERSIANN-CCS) were not evaluated and compared for the Central Highlands of Abbay Basin, Ethiopia. Hence, this research was aimed to give a new insights to wards using SPEs, as the best alternative sources of rainfall data, in areas where guage based stations are limited/absent like our study area, which as far as we know, will be the first approach to compare the detection and estimation performance of SPEs and select the best one for further research work. Therefore, the key objective of this study was to examine the rainfall detection and estimation performance of the SPEs: CHIRPSv2, CMORPH, IMERG-06, and PERSIANN-CCS against gauge-based observations over the central highlands of Abbay (Upper Blue Nile) Basin, Ethiopia. Hence, the best-performed ones will be used as a climate data source in areas where there is sparse/absence of meteorological stations, to study the climate system, agricultural planning, water resource management, drought assessment, and flood management and forecasting.
Materials and methods
Description of the study area
The study was conducted in the domain area of the central highlands of Abbay Basin, located between 10°16′ to 11°54′ Latitude and 36°31′ to 37°57′ Longitude (). The study area in the central highlands of Abbay Basin covers an area of approximately 31,152 km2. The source of the River Abbay (Blue Nile) is commonly known as Gish Abbay which is found in the study area. It is from this area that the river flows northward as the Gilgel Abbay into Lake Tana (Oestigaard & Gedef, Citation2011). The topographical feature of the central highlands of Abbay Basin is a rugged mountainous area and the altitude ranges approximately between 1400 and 4090 m asl (Getahun & Shefine, Citation2015). The climate system of the central highlands of Abbay basin, Ethiopia is a monomodal rainfall pattern and is mainly governed by the global rain-bearing system, particularly the Inter-tropical Convergence Zone (ITCZ). It follows the position of the sun relative to Earth and the associated atmospheric circulation, in conjunction with the country’s complex topography (Seleshi & Zanke, Citation2004). The main rainfall season of the area occurred during kiremt season (JJAS) (Segele et al., Citation2015), when the Inter-tropical Front, the zone of convergence of winds move towards the north of the country (Tadesse, Citation1994). During this time the moist winds from the middle part of the Atlantic Ocean, often referred to as Equatorial Westerly, are down towards this area of low atmospheric pressure and provide rain (Kassahun, Citation1987). Thus, most of the western and central highlands capture more rainfall than the rest of the basin (Korecha & Barnston, Citation2007). Kiremt season contributed about 74% of rainfall for annual budget (Korecha & Sorteberg, Citation2013).
Figure 1. Location and altitude map of central highlands of Abbay Basin, West Gojjam Zone, Ethiopia.

The basin has a mean annual rainfall ranging between 810 mm and 2030 mm. Western and south-eastern parts of the central highlands of Abbay basin received lower annual rainfall, i.e. less than 1200 mm, whereas the northern part of the basin has received greater than 1400 mm up to 2025 mm per year. The mean annual maximum and minimum temperatures in the basin vary between 13°C to 33°C and −0.5°C to 18°C, respectively. Temperature is higher in the western parts with a maximum of 26°C to 33°C and a minimum of 12°C to18°C. Potential Evapotranspiration (PET) in the area is generally between 1070 mm and 2010 mm per year. PET is higher (>1700 mm per year) in relatively lowland areas and along the rivers where high temperature is observed. The highlands of the basin show lower PET, less than 1400 mm per year (Awulachew et al., Citation2009).
The land use and cover types in the study domain are forest, scrublands, grasslands, cultivated land, rural settlement and farmland, urban settlements, plantation, water body, and croplands (Getahun & Shefine, Citation2015). The dominant land use in the study domain is dominated by agricultural and agro-pastoral lands. Small parts of the sub-basin were used as pastoral land. Agro-Ecological Zone of the study basin is characterized by tepid to cool moist/sub-humid mid-highlands, and cold to very cold moist sub-afro-alpine to afro-alpine in parts of the highlands and in the eastern, southeastern and southern parts of the basin being hot to warm moist/sub-humid lowlands (Awulachew et al., Citation2009). The dominant soils in the basin are Alisols and Cambisols with the occurrence of Leptosols, Vertisols, Luvisols, Nitosols, and Acrisols. The geology of the sub-basin is mainly dominated by basalt, with the southeastern lowlands dominated by sandstone. There are also Coluvium and Alluvium deposits. The total population of the basin is 7,778,726 people (Yilma & Bekele Awulachew, Citation2009).
Data sources
Observed data
Daily rainfall data from 1991 to 2020 were obtained from 18 meteorological stations of the National Meteorological Agency (NMA), Ethiopia. However, after a quality control process and considering that the rainfall station with missing data, less than 10% or 15% (WMO, Citation2017) is considered for this study, accordingly 15 stations were selected out of 18 (). Missing data of the study stations ranged between 1.2% and 12.8% (). The Gauged rainfall data is used as a reference to evaluate the measuring accuracy of SPEs. Most stations have better continuous data with little missing data for the study period. To determine a common period for all the gauge-based stations, some missing data were calculated. The arithmetic average method, a simulation of daily data from existing using the Markov chain simulation model (INSTAT + v3.36 software) which is a stochastic model describing a sequence of possible events in which the probability of each event depends only on the state attained in the previous event was employed (Stern et al., Citation2006).
Table 1. Geographical coordinates and mean rainfall of the selected station used for this study.
Satellite precipitation estimators (SPEs) data set
The satellite products used in the analysis are commonly used in operational and research activities focusing on water resource planning, design, planning agricultural activities, and decision-making in the central highlands of Abbay River basin. This study evaluated four SPEs at a daily time scale (). The satellite precipitation data estimators were Climate Hazards center Infra-Red Precipitation with Station data (CHIRPSv2), the Climate prediction center (CPC) morphing technique (CMORPH), the Integrated Multi-satellite Retrieval for GPM (IMERG-06), and the Precipitation Estimation from Remotely Sensed Information using Artificial Neural Networks (PERSIANN-CCS). SPEs data were carefully inspected for quality and completeness. Further, data homogeneity test was done by using RAINBOW-a software package for hydro-metrological frequency analysis and testing the homogeneity of climate data sets (Raes et al., Citation2006).
Table 2. Summary of the satellite rainfall products used in this study.
CHIRPS is a 30+ year quasi-global rainfall data set. Spanning 50°S-50°N (and all longitudes), starting in 1981 to the near present, CHIRPS incorporates 0.05° resolution satellite imagery with in-situ station data to create a gridded rainfall time series for trend analysis and seasonal drought monitoring (Funk et al., Citation2015). The data were accessed through (https://data.chc.ucsb.edu/products/CHIRPS-2.0/africa_daily) link.
The Climate Prediction Center (CPC) Morphing Technique (CMORPH) provides half-hourly and 8 km grid size of relatively high-quality precipitation estimates derived from passive microwave satellite scans are propagated by motion vectors derived from geostationary satellite infrared data and produces global precipitation (Joyce et al., Citation2004). The dataset estimates the precipitation that has been derived using low orbital satellite-based passive microwave observation from different sources. The rainfall estimate data is extracted from a grid box gauge observation is found. Originally, the data were a 30-minute time scale and it was aggregated to a daily time step for analysis (), https://climatedataguide.ucar.edu/climate-data, accessed 2 April 2022. The PMW-derived precipitation estimates that are presently used in CMORPH are generated from observations obtained from the NOAA polar-orbiting operational meteorological satellites, such as the U.S. Defense Meteorological Satellite Program (DMSP) satellites, and from the Tropical Rainfall Measuring Mission https://www.ncei.noaa.gov/products/climate-data-records/precipitation-cmorph satellite, accessed on 5 April 2022.
The Integrated Multi-satellite Retrieval for GPM (IMERG-06) product combines precipitation observations using infrared and microwave sensors from a constellation of partner satellites, united by the GPM Core Observatory, to provide near real-time half-hourly precipitation estimates at 0.1 degree resolution for the entire globe; https://gpm.nasa.gov/data/imerg accessed 2 April 2022. This algorithm is intended to inter-calibrate, merge, and interpolate “all” satellite microwave precipitation estimates, together with microwave calibrated infrared (IR) satellite estimates, precipitation gauge analyses, and potentially other precipitation estimators at fine time and space scales for the TRMM and GPM eras over the entire globe (Huffman et al., Citation2020; Sharma et al., Citation2020). The product Integrated Multi-satellitE Retrieval, IMERG-06 final daily time step is extracted for the study domain.
The Precipitation Estimation from Remotely Sensed Information using Artificial Neural Networks (PERSIANN) family includes: PERSIANN, PERSIANN-CCS, and PERSIANN-CDR. The products are accessible through several web-based interfaces: CHRS iRain (http://irain.eng.uci.edu), Data Portal 30 (http://chrsdata.eng.uci.edu), and RainSphere (http://rainsphere.eng.uci.edu). These web-based interfaces provide different visualization, analysis, and download capabilities (Nguyen et al., Citation2019).
PERSIANN-Cloud Classification System is a real-time global high-resolution (0.04° × 0.04°) satellite precipitation product developed by the center for Hydrometeorology and Remote Sensing (CHRS) at the University of California, Irvine (UCI). PERSIANN-CCS enables the categorization of cloud patch features based on cloud height, areal extent, and variability of texture estimated from satellite imagery. At the heart of PERSIANN-CCS is the variable threshold cloud segmentation algorithm. In contrast to the traditional constant threshold approach, the variable threshold enables the identification and separation of individual patches of clouds. The individual patches can then be classified based on texture, geometric properties, dynamic evolution, and cloud top height. These classifications help in assigning rainfall values to pixels in each cloud based on a specific curve describing the relationship between rain rate and brightness temperature (Hong et al., Citation2007). Currently, SPEs of CHIRPSv2, CMORPH, IMERG-06, and PERSIANN-CCS were evaluated against gauge-based data obtained from the global data sets and national meteorology agency.
Methods of data analysis
Categorical analysis
The categorical technique is an assessment system of satellite estimation using a contingency table that reflects the frequency of “Yes” and “No” of the satellite estimation (Sharifi et al., Citation2016). Four categorical techniques were employed to summarize and analyze the daily and wet season rainfall data. Such as Probability of detection (POD), False alarm ratio (FAR), Critical Success Index (CSI), and Accuracy (fraction correct) have been applied.
A dichotomous estimate says, “Yes, an event will happen”, or “No, the event will not happen”. Based on , a set of statistical measurements were calculated using the daily precipitation data set. POD responds to the question of what fraction of the observed “Yes” events were correctly estimated. FAR deals with the question of what fraction of the estimated “Yes” events did not occur. CSI answers the question of how well the estimated “Yes” corresponds to the observed “Yes” events. Accuracy measures the fraction of correct estimates. The ideal value of POD, CSI, and Accuracy is 1. Whereas the best value of FAR is 0 (Khan et al., Citation2010). These capability metrics are calculated as follows:
Table 3. Contingency table to evaluate precipitation occurrence by satellite products.
Where, is the number of rainfall events correctly detected, b is the number of rainfall events falsely detected by the satellite, c is the number of rainfall events missed, and d events with no rainfall are correctly detected.
Continuous validation statistics
Various statistical analysis techniques were employed to evaluate the rainfall estimation performance of SPEs with the rain gauge precipitation data. Such as, bias ratio, Relative/probability Bias, Mean Absolute Error (MAE), Root Mean Square Error (RMSE), and Correlation Coefficient (CC) indices were applied.
The bias ratio is an error metric measuring the systematic error component of the data set. Bias indicates how well the mean of the satellite rainfall corresponds to the mean of rain-gauge rainfall and can be either less than or greater than 1. Values less than and greater than 1indicates underestimation and overestimation by satellite, respectively, where a perfect score is 1. Relative Bias (RBias) describes the systematic bias of satellite-based precipitation and the magnitude of the bias expressed in the percentile. The mean absolute error (MAE) is used to represent the average magnitude of the error. The root mean square error (RMSE), which gives greater weight to the larger errors relative to MAE, is used to measure the average error magnitude. It indicates the absolute fit of the SPE to the rain-gauge data and provides an average SPEs prediction error. The range of RMSE is between 0 to infinity, where closer the score is to 0 the better performing the SPE is. The correlation coefficient (CC) is used to assess the agreement between satellite and rain gauge observations. The range of CC is in between −1 and + 1. A correlation value of 1 indicates a perfect positive fit and the reverse is true for −1 value. If there is no linear correlation or weak linear correlation, CC is close to zero. The above error metrics are calculated as follows (equations 5–9):
where Si is the satellite estimate; Gi is the rain gauge rainfall; and
are the mean of satellite and gauge rainfall, respectively.
Results and discussions
Annual and wet season rainfall pattern
The spatial patterns of mean annual and wet season rainfall of the study area were evaluated visually. The gauged based mean annual and wet season rainfalls were used as a reference to compare with CHIRPSv2, CMORPH, IMERG-06, and PERSIANN-CCS datasets (). The long-term (1991 to 2020) areal mean annual rainfall obtained from the gauge-based stations was 1497 mm, whereas from CHIRPSv2 was 1436 mm. However, the mean annual rainfall estimation from CMORPH (1998–2020), IMERG-06 (2001–2020), and PERSIANN-CCS (2003–2020) were about 1258 mm, 1370 mm and 1123 mm rainfall, respectively (). As indicated by Ibrahim et al. (Citation2022), in his study on Performance evaluation of satellite-based rainfall estimates for hydrological modeling over Bilate river basin, Ethiopia, the mean wet season rainfall of SPEs of the present study was compared with the gauged data. The results showed that about 1140 mm of rainfall was recorded from the gauge, on the other hand, the SPEs estimated about 1007 mm (CHIRPSv2), 964 mm (CMORPH), 1002 mm (IMERG-06), and 798 mm (PERSIANN-CCS) (). The results from the areal mean annual and wet season rainfall of the SPEs indicated that CHIRPSv2 estimated slightly better than the other three SPEs for the period examined followed by IMERG-06 whereas CMORPH and PERSIANN-CCS did not perform well.
Figure 2. Spatial distribution of mean annual Gauge, CHIRPSv2, CMORPH, IMERG-06 and PERSIANN-CCS and JJAS Gauge, CHIRPSv2, CMORPH, IMERG-06 and PERSIANN-CCS rainfall data (1998–2020).

Visual examination of shows the distribution pattern of mean annual and wet season rainfall exhibited a decreasing tendency from the northwestern to the southeastern part of the study area. The spatial pattern in shows that all SPEs have a relatively constant rainfall pattern. This finding is in line with the result of Hordofa et al. (Citation2021) and Sahlu et al. (Citation2016), who stated IMERG & CMORPH had consistent rainfall patterns in Ziway Lake Basin and UBN basin, respectively. The present finding also indicated that both the mean annual and wet season rainfall distributions of SPEs have similar patterns. JJAS are the main rainfall contributor, about 74% of rainfall for the annual budget, of the study area. The finding of present study is inline with a previous study by (Gummadi et al., Citation2018), who stated that the June to September season contributed 74% of the annual rainfall for most parts of Ethiopia. This season is the main season for farmers to perform their livelihood activities, depending on rainfed agriculture (Gebreegziabher et al., Citation2016). On the other hand, high altitude area rainfall is under estimated by all SPEs for both mean annual and wet season rainfall of the study area ().
Seasonal rainfall pattern
Monthly rainfall analysis was done using common time coverage for all satellite datasets (2003–2020). The seasonal analysis results showed that all products perform better in October, November, December, and January (ONDJ), where little rain had been recorded in all stations. However, CHIRPSv2 performs better during February, March, April, and May (FMAM) and annual rainfall estimation than the other examined data sources (). A similar result was reported by Wedajo et al. (Citation2021) who evaluated multiple satellite rainfall products for Dhidhessa River (DRB), Ethiopia, and found CHIRPSv2 had best matched with the observed data. Contrary to this, the study by Ji et al. (Citation2022) indicated that CMORPH and GPM (IMERG-06) fit well with the annual observation, in evaluating multiple satellite precipitation products and their potential utilities in the Yarlung Zangbo River basin. On the other hand, the main rainfall season (JJAS) evaluation of the present study result indicated IMERG-06 was better and superior to the others while PERSIANN-CCS was found to underestimate and overestimate the rainfall during JJAS and FMAM seasons, respectively (). A similar result was reported by Rachdane et al. (Citation2022) who compared a high-resolution satellite precipitation products in sub-saharan Morocco, and found GPM-F (IMERG-06) showed the most satisfactory result for all time scales and for both spatial scales, followed by CHIRPS products at monthly and annual scales. Further, the study by Kumar et al. (Citation2022) also confirmed that CHIRPS was competent in terms of matching the precipitation magnitude to ground observations in data-sparse Himalayan terrain.
Rainfall detection capability
For the daily time scale, the value of POD was 0.77 for CMORPH and 0.73 for IMERG-06. Whereas the values of FAR were 0.33 for CMORPH and 0.31 for IMERG-06 (). During the wet season, CMORPH had a POD of 0.90 and 0.20 FAR, and IMERG-06 had a value of 0.82 POD and 0.18 FAR (). The result indicates that CMORPH performed better than all SPEs in detecting rainy events while IMERG-06 was found slightly better than CMORPH in avoiding detecting false rainfall events. Previous studies by Ashenafi and Hailu (Citation2014), Sahlu et al. (Citation2016), Sahlu et al. (Citation2017), and Kumar et al. (Citation2022) confirmed that CMORGH was superior in rainfall event detection. CHIRPSv2 and PERSIANN-CCS exhibit a good performance in avoiding detecting false rainfall events. In addition, a research by Chen et al. (Citation2020) also confirmed that CMORPH performs better than those designed to achieve the most temporally homogeneous record, PERSIANN-CDR and CHIRPS, who evaluates eight satellite-based precipitation datasets in streamflow simulations on a monsoon-climate watershed in China.
Table 4. Comparison of daily rainfall (≥1 mm) for satellite datasets using categorical metrics.
The results of CSI () illustrated that CMORPH, IMERG-06, PERSIANN-CCS, and CHIRPSv2 have 0.74, 0.70, 0.67 and 0.57, respectively. This indicated that the estimation of CMORPH product has well corresponded to the gauge-based estimation of rainfall at the wet season time scale. Next to CMORPH, IMERG-06 had better capability than the remaining SPEs to estimate rainfall against gauge-based observation at JJAS. In a relative term, the poor estimation agreement has been shown by PERSIANN-CCS. The statistics of accuracy in the wet season time scale for CMORPH; was 0.75 followed by IMERG-06 recorded 0.72. While PERSIANN-CCS showed better performance than CMORPH to measure the fraction of correct estimates or accuracy at the daily time scale.
The POD and FAR of rainfall products are the result of several factors, including the calibrated relationship between sensor reflectivity and estimated rain rate, and the sampling error depending on the timing of satellite overpasses (Serrat-Capdevila et al., Citation2016). CMORPH outperformed in POD, CSI, and Accuracy, while CHIRPSv2 performed the lowest detection, especially at the wet season. Furthermore, CMORPH has a relatively high FAR, whereas PERSIANN-CCS has a great capacity to avoid such kind of errors among the four SPEs next to CHRIPSv2 for the daily and wet season of the study area. The rainfall detection capability of the four SPEs in the study area is better for the wet season compared to the daily time scale ().
A number of studies (Dandridge et al., Citation2019; de Brito et al., Citation2022; Gebremicael et al., Citation2019; Gummadi et al., Citation2022; Hordofa et al., Citation2021; Malede et al., Citation2022; Taye et al., Citation2020) indicated the superior performance of CHIRPS on monthly and wet season estimation than CMORPH, CPC, and GSMaP; ARC2, REFV2, TAMSATv3; IMERG, IMERG-L, IMERG-F, and PERSIANN-CDR in terms of low bias, MAE and RMSE. In addition, the study of Bayissa et al. (Citation2017) reported an excellent score of bias and mean error (close to one) for CHIRPS at decadal, monthly, and seasonal time scales in their study at the UBN basin. Further, Wedajo et al. (Citation2021) also reported better rainfall estimation by CHIRPS compared to IMERG and TAMSAT3 and 3B42/3 products for the Dhidhessa River Basin of Ethiopia. Similarly, Reda et al. (Citation2021) indicated that the CHIRPS data set showed higher agreement with gauge observations over Ethiopia’s Upper Tekeze River basin. On the other hand, Ayehu et al. (Citation2018) reported an overestimation of the frequency of rainfall occurrence of decadal rainfall by CHIRPSv2 in the UBN basin. All the above studies are in line with the current study in terms of low FAR statistical measures but not with bias, MAE, and RMSE. The discrepancy in findings between the current and the previous studies might be due to differences in topography, vegetation cover, climatic conditions, and selected performance indicator (Kumar et al., Citation2022). Evaluation results of different SPEs may not be the same in all places in different time scales, for example, the evaluation result made by Perera et al. (Citation2022) and Hartke and Wright (Citation2022), indicated that IMERG product was the best alternative of SPEs while PERSIANN-CDR showed the worst performance in detecting and predicting rainfall among PERSIANN, PERSIANN-CCS, IMERG, TRMM-342, and TRMM-3B42RT in terms of POD, FAR, and CSI. Similarly, the study by Retalis et al. (Citation2020) and Retalis et al. (Citation2022), showed that IMERG product had better ability than 3B43V7 and GSMap with higher R, lower Bias, and RBias values. According to the same source, IMERG-06; rainfall data can be surrogate in the limited and absence of ground-based observed rainfall data. However, it has to be clear that these products possess significant errors which cannot be ignored when using SPEs in various applications. Conversely, the study by (de Brito et al., Citation2021) reported PERSIANN-CDR could detect the events better than CHIRPS in Piranhas River basin, in northeastern Brazil.
Performance of the satellite estimates
For evaluating the estimation performance of SPEs, daily rainfall data has been used. The performance of CHIRPSv2, CMORPH, IMERG-06, and PERSIANN-CCS has been evaluated quantitatively against the reference rain gauge observation data using the statistical error metrics of CC, bias, RBias, MAE, and RMSE. The results from CC statistics showed that all SPEs performed well (). The mean value of CC for CHIRPSv2, CMORPH, IMERG-06, and PERSIANN-CCS indicated a better relationship with daily and wet season observed rainfall data. All SPEs were equivalent at the daily time scale (r ∼0.8) except PERSIANN-CCS. Whereas CHIRPSv2 and CMORPH performed well with a high CC value at the wet season (r = 0.58) compared to IMERG-06, r = 0.52; and PERSIANN-CCS, r = 0.43. As presented in the scatterplot (), the value of R-square (R2) was approximately equivalent in the case of CHIRPSv2 and IMERG-06, whereas CMORPH and PERSIANN-CCS had, R2 of 0.67 and 0.60, respectively. This indicated that CHIRPSv2 and IMERG-06 have a better relationship with gauge rainfall data, whereas CMORPH and PERSIANN-CCS underestimated the gauge rainfall. Whereas the mean value of r was nearly equivalent to the perfect score in CHIRPSv2, CMORPH, and IMERG-06; this indicates that the presence of a linear relationship between the estimated and observed rainfall measurements. In relative terms, CHIRPSv2, IMERG-06 and, CMORPH showed a better performance in the study area ().
Figure 4. Scatterplots for monthly rainfall data for CHIRPSv2, CMORPH, IMERG-06, and PERSIANN-CCS against gauge rainfall data in central highlands of Abbay Basin.

Table 5. Statistical measures of daily and JJAS rainfall amount in the central highlands of Abbay Basin.
The bias ratio result of IMERG-06 (0.90) at daily and CHIRPSv2 (0.87) at JJAS time scale indicated that relatively both have estimated the rainfall perfectly. PERSIANN-CCS scored (0.68) at JJAS and (0.73) at daily time scale and this indicates the product underestimated rainfall in both cases. CMORPH (∼0.82) at the JJAS and daily time scale and IMERG-06 (0.86) at JJAS and CHIRPSv2 (0.87) at daily time scale and this indicated they slightly underestimated the observed rainfall ().
The result from RBias showed that the spread of error of satellite products in percent at daily time scale is in the range from 9 to 26, while at JJAS time scale the values found in the range from −31 to −12 with CHIRPSv2 and IMERG-06 yielded a better estimation at JJAS (12% and 13%) and at daily time scale (10% and 9%), respectively. In general, this index showed that all SPEs underestimated the observed rainfall at both time scales over the study area. In relative terms, IMERG-06 and CHIRPSv2 have better estimation performance than the others in both time scales ().
RMSE indicates the random error component of SPEs. As well illustrated in , RMSE values of CHIRPSv2, PERSIANN-CCS, CMORPH, and IMERG-06 are 99.0, 89.7, 86.0, and 75.9 for JJAS, respectively. In terms of RMSE, all SPEs had a lower error on a daily time scale than wet season (JJAS). The results showed the presence of a lower level magnitude of estimation error between SPEs and rain gauge, and the greater central tendency for IMERG-06 for both time scales. In addition, IMERG-06 provides better values of RMSE for both daily (50.3 mm) and wet season (75.9 mm) rainfall and MAE (7.7 mm) for daily time scale followed by CHIRPSv2 (12.5 mm). On the other hand CHIRPSv2 scored the highest error magnitude of rainfall estimation for daily and wet season rainfall. Overall, IMERG-06 was found to be superior to the rest SPEs with respect to the important performance assessment of error parameters in estimating the rainfall of the study area. A similar study by Wang and Qiaoyan (Citation2022) compared multi-satellite precipitation data from global precipitation measurement mission and tropical rainfall measurement mission datasets: seasonal and diurnal cycles, and found that the IMERG-06 product provides a high resolution in estimating the precipitation. Another study by Jiang et al. (Citation2021) also reported that GPM IMERG Final can be seen as a substitute for measured data when elevation is below 4000 m. Besides the studies by Moazami and Najafi (Citation2021), Rachdane et al. (Citation2022), Hordofa et al. (Citation2021) and Retalis et al. (Citation2022) confirmed that IMEG-06 had the potential to complement ground-based observation. In support of this, the study by Gebremichael et al. (Citation2014) reported that CMORPH suffers from a large positive bias at the lowland plain site of UBN. Another study by Hirpa et al. (Citation2010) also indicated that PERSIANN consistently underestimates extreme wet events in comparison with 3B42RT and CMORPH over very complex terrain in Ethiopia.
Conclusions
This study evaluates four high-resolution SPEs (CHIRPSv2, CMORPH, IMERG-06, and PERSIANN-CCS) to identify the best product in terms of detection and estimation performance of rainfall for the period from 1991 to 2020 in the central highlands of Abbay Basin. Where categorical and continuous, statistical indices were applied for assessment.
The results of the study showed that all SPEs considered in the study are able to capture the daily and wet season rainfall of the study area, even though statistical errors or overestimation and underestimation were observed. Especially, satellite data sets that used IR frequency (CHIRPSv2) in the categorical indices such as POD, CSI, accuracy but they scored better FAR values. The reverse is true for SPEs that used Microwave (CMORPH). Whereas the datasets used, the integration of IR and MW (IMERG-06) perform the optimum result in both statistical indices, i.e. categorical and continuous analysis techniques. However, PERSIANN-CCS which used IR satellite imagery showed poor performance in all aspects.
Even though CMORPH is superior in rainy day detection, it has high bias error and False Alarm Rate (FAR). On the contrary, CHIRPSv2 has better total rainfall estimation, relatively low bias ratio, and FAR but it has high error metrics; RBias, RMSE, and low rainy day detection compared with the other three SPEs.
Each dataset has both weaknesses and strengths. Some of them underestimate and others overestimate rainfall. This indicates that accurate estimation of SPEs remains a challenge. Such inaccuracy may be rooted in the inadequate number of gauges provided by the Global Precipitation Climatology Centre and used for bias correction in satellite products or the non-uniform beam filling problem for remote sensing instruments (Sharifi et al., Citation2016). Overall, while the IMERG-06 rainfall dataset showed better performance over CHIRPSv2, CMORPH, and PERSIANN-CCS datasets, all datasets can be used when gauge-based rainfall data are not available.
This study was initiated to indicate the possibility of having alternative data sources in areas where meteorological stations are not established and/or sparsely distributed and filling missing data. From the result of this study, the best-performed SPE product has been selected and could be used as a reliable rainfall data source for researchers, practitioners, developmental planners, stakeholders, decision-makers, and other interested groups for climate analysis; drought assessment and monitoring; hydrological analysis, and water resource management, flood forecasting and other related activities in the central highlands of Abbay Basin of Ethiopia.
Future studies should focus on containing more stations in the area having different topography, vegetation, cover and climatic conditions of the area to see the detection and estimation performance of SPEs in various altitudinal areas and to generalize the conclusions drawn from the study.
Availability of data statement
The data used for analysis for the current study are available up on the corresponding author and National Meteorological Station Agency (NMA) on reasonable request.
Acknowledgments
The authors acknowledge the National Meteorology Agency of Ethiopia (NMA) for providing the study with long-term gauge-based daily rainfall data. We are grateful to Bahir Dar University for providing the first author a study leave.
Disclosure statement
No potential conflict of interest was reported by the authors.
References
- https://www.ncei.noaa.gov/data/cmorph-high-resolution-global-precipitation-estimates/access/30min/8km/
- https://gpm.nasa.gov/data/imerg
- https://data.chc.ucsb.edu/products/CHIRPS-2.0/africa_daily
- Asfaw, A., Simane, B., Hassen, A., & Bantider, A. (2018). Variability and time series trend analysis of rainfall and temperature in northcentral Ethiopia: A case study in woleka sub-basin. Weather and Climate Extremes, 19, 29–14. https://doi.org/10.1016/j.wace.2017.12.002
- Ashenafi, Y., & Hailu, D. (2014). Assessment of the use of remotely sensed rainfall products for runoff simulation in the Upper Blue Nile basin of Ethiopia. Zede Journal, 31, 1–9.
- Awulachew, S. B., McCartney, M., Steenhuis, T. S., & Ahmed, A. A. (2009). A review of hydrology, sediment and water resource use in the Blue Nile Basin, 131, 81.
- Ayehu, G. T., Tadesse, T., Gessesse, B., & Dinku, T. (2018). Validation of new satellite rainfall products over the Upper Blue Nile Basin, Ethiopia. Atmospheric Measurement Techniques, 11(4), 1921–1936. https://doi.org/10.5194/amt-11-1921-2018
- Bayissa, Y., Tadesse, T., Demisse, G., & Shiferaw, A. (2017). Evaluation of satellite-based rainfall estimates and application to monitor meteorological drought for the Upper Blue Nile Basin, Ethiopia. Remote Sensing, 9(7), 669. https://doi.org/10.3390/rs9070669
- Behrangi, A., Khakbaz, B., Chun Jaw, T., AghaKouchak, A., Hsu, K., & Sorooshian, S. (2011). Hydrologic evaluation of satellite precipitation products over a mid-size basin. Journal of Hydrology, 397(3–4), 225–237. https://doi.org/10.1016/j.jhydrol.2010.11.043
- Belay, A. S., Almaw Fenta, A., Yenehun, A., Nigate, F., Tilahun, S. A., Moges, M. M., Dessie, M., Adgo, E., Nyssen, J., Chen, M., Griensven, A. V., & Walraevens, K. (2019). Evaluation and application of multi-source satellite rainfall product CHIRPS to assess spatio-temporal rainfall variability on data-sparse western margins of Ethiopian highlands. Remote Sensing, 11(22), 2688. https://doi.org/10.3390/rs11222688
- Bitew, M. M., & Gebremichael, M. (2011). Evaluation of satellite rainfall products through hydrologic simulation in a fully distributed hydrologic model. Water Resources Research, 47(6). https://doi.org/10.1029/2010WR009917
- Bui, H. T., Ishidaira, H., & Shaowei, N. (2019). Evaluation of the use of global satellite–gauge and satellite-only precipitation products in stream flow simulations. Applied Water Science, 9(3), 1–15. https://doi.org/10.1007/s13201-019-0931-y
- Chen, J., Ziyi, L., Li, L., Wang, J., Wenyan, Q., Chong-Yu, X., & Kim, J.-S. (2020). Evaluation of multi-satellite precipitation datasets and their error propagation in hydrological modeling in a monsoon-prone region. Remote Sensing, 12(21), 3550. https://doi.org/10.3390/rs12213550
- Dandridge, C., Lakshmi, V., Bolten, J., & Srinivasan, R. (2019). Evaluation of satellite-based rainfall estimates in the lower Mekong river basin (southeast asia). Remote Sensing, 11(22), 2709. https://doi.org/10.3390/rs11222709
- de Brito, C. S., da Silva, R. M., Augusto Guimarães Santos, C., Moura Brasil Neto, R., & Hugo Rabelo Coelho, V. (2021). Monitoring meteorological drought in a semiarid region using two long-term satellite-estimated rainfall datasets: A case study of the Piranhas River basin, northeastern Brazil. Atmospheric Research, 250, 105380. https://doi.org/10.1016/j.atmosres.2020.105380
- de Brito, C. S., da Silva, R. M., Augusto Guimarães Santos, C., Moura Brasil Neto, R., & Hugo Rabelo Coelho, V. (2022). Long-term basin-scale comparison of two high-resolution satellite-based remote sensing datasets for assessing rainfall and erosivity in a basin in the Brazilian semiarid region. Theoretical and Applied Climatology, 147(3–4), 1049–1064. https://doi.org/10.1007/s00704-021-03857-w
- Derin, Y., Anagnostou, E., Berne, A., Borga, M., Boudevillain, B., Buytaert, W., Chang, C.-H., Delrieu, G., Hong, Y., Chia Hsu, Y., Lavado-Casimiro, W., Manz, B., Moges, S., Nikolopoulos, E. I., Sahlu, D., Salerno, F., Rodríguez-Sánchez, J.-P., Vergara, H. J., & Yilmaz, K. K. (2016). Multiregional satellite precipitation products evaluation over complex terrain. Journal of Hydrometeorology, 17(6), 1817–1836. https://doi.org/10.1175/JHM-D-15-0197.1
- Dinku, T., Funk, C., Peterson, P., Maidment, R., Tadesse, T., Gadain, H., & Ceccato, P. (2018). Validation of the CHIRPS satellite rainfall estimates over eastern Africa. Quarterly Journal of the Royal Meteorological Society, 144(S1), 292–312. https://doi.org/10.1002/qj.3244
- Dinku, T., Ruiz, F., Connor, S. J., & Ceccato, P. (2010). Validation and intercomparison of satellite rainfall estimates over Colombia. Journal of Applied Meteorology and Climatology, 49(5), 1004–1014. https://doi.org/10.1175/2009JAMC2260.1
- Funk, C., Peterson, P., Landsfeld, M., Pedreros, D., Verdin, J., Shukla, S., Husak, G., Rowland, J., Harrison, L., Hoell, A., & Michaelsen, J. (2015). The climate hazards infrared precipitation with stations—a new environmental record for monitoring extremes. Scientific Data, 2(1), 1–21. https://doi.org/10.1038/sdata.2015.66
- Gebreegziabher, S. B., Yimer, S. A., & Bjune, G. A. (2016). Qualitative assessment of challenges in tuberculosis control in West Gojjam Zone, Northwest Ethiopia: Health Workers’ and tuberculosis control program Coordinators’ perspectives. Tuberculosis Research and Treatment, 2016, 1–8. https://doi.org/10.1155/2016/2036234
- Gebremicael, T. G., Mohamed, Y. A., van der Zaag, P., Gebremedhin, A., Gebremeskel, G., Yazew, E., & Kifle, M. (2019). Evaluation of multiple satellite rainfall products over the rugged topography of the Tekeze-Atbara basin in Ethiopia. International Journal of Remote Sensing, 40(11), 4326–4345. https://doi.org/10.1080/01431161.2018.1562585
- Gebremichael, M., Bitew, M. M., Hirpa, F. A., & Tesfay, G. N. (2014). Accuracy of satellite rainfall estimates in the B lue N ile B asin: L owland plain versus highland mountain. Water Resources Research, 50(11), 8775–8790. https://doi.org/10.1002/2013WR014500
- Getahun, Y. S., & Shefine, B. G. (2015). Analysis of climate variability (ENSO) and vegetation dynamics in Gojjam, Ethiopia. Journal of Earth Science & Climatic Change, 6(10), 320.
- Githungo, W., Otengi, S., Wakhungu, J., & Masibayi, E. (2016). Infilling monthly rain gauge data gaps with satellite estimates for Asal of Kenya. Hydrology, 3(4), 40. https://doi.org/10.3390/hydrology3040040
- Gummadi, S., Dinku, T., Shirsath, P. B., & Kadiyala, M. D. M. (2022). Evaluation of multiple satellite precipitation products for rainfed maize production systems over Vietnam. Scientific Reports, 12(1), 1–18. https://doi.org/10.1038/s41598-021-04380-8
- Gummadi, S., Rao, K. P. C., Seid, J., Gizachew Legesse, M. K., Takele, R., Amede, T., & Whitbread, A. (2018). Spatio-temporal variability and trends of precipitation and extreme rainfall events in Ethiopia in 1980–2010. Theoretical and Applied Climatology, 134(3), 1315–1328. https://doi.org/10.1007/s00704-017-2340-1
- Hartke, S. H., & Wright, D. B. (2022). Where can IMERG provide a better precipitation estimate than interpolated Gauge data? Remote Sensing, 14(21), 5563. https://doi.org/10.3390/rs14215563
- Hirpa, F. A., Gebremichael, M., & Hopson, T. (2010). Evaluation of high-resolution satellite precipitation products over very complex terrain in Ethiopia. Journal of Applied Meteorology and Climatology, 49(5), 1044–1051. https://doi.org/10.1175/2009JAMC2298.1
- Hong, Y., Gochis, D., Cheng, J.-T., Hsu, K.-L., & Sorooshian, S. (2007). Evaluation of PERSIANN-CCS rainfall measurement using the NAME event rain gauge network. Journal of Hydrometeorology, 8(3), 469–482. https://doi.org/10.1175/JHM574.1
- Hordofa, A. T., Tolessa Leta, O., Alamirew, T., Serre Kawo, N., & Demissie Chukalla, A. (2021). Performance evaluation and comparison of satellite-derived rainfall datasets over the Ziway lake basin, Ethiopia. Climate, 9(7), 113. https://doi.org/10.3390/cli9070113
- Huffman, G. J., Bolvin, D. T., Braithwaite, D., Hsu, K.-L., Joyce, R. J., Kidd, C., Nelkin, E. J., Sorooshian, S., Stocker, E. F., Tan, J., Wolff, D. B., & Xie, P. (2020). Integrated multi-satellite retrievals for the global precipitation measurement (GPM) mission (IMERG). In Satellite precipitation measurement (pp. 343–353). Springer International Publishing. https://doi.org/10.1007/978-3-030-24568-9_19
- Ibrahim, A. H., Daniel Molla, D., & Kumar Lohani, T. (2022). Performance evaluation of satellite-based rainfall estimates for hydrological modeling over Bilate river basin, Ethiopia. World Journal of Engineering (Ahead-Of-Print). https://doi.org/10.1108/WJE-03-2022-0106
- Jiang, X., Liu, Y., Yongxiang, W., Wang, G., Zhang, X., Meng, Q., Pengfei, G., & Liu, T. (2021). Evaluation of the performance of multi-source precipitation data in Southwest China. Water, 13(22), 3200. https://doi.org/10.3390/w13223200
- Ji, H., Peng, D., Gu, Y., Liang, Y., & Luo, X. (2022). Evaluation of multiple satellite precipitation products and their potential utilities in the Yarlung Zangbo River Basin. Scientific Reports 12 (1):13334.
- Joyce, R. J., Janowiak, J. E., Arkin, P. A., & Xie, P. (2004). CMORPH: A method that produces global precipitation estimates from passive microwave and infrared data at high spatial and temporal resolution. Journal of Hydrometeorology, 5(3), 487–503. https://doi.org/10.1175/1525-7541(2004)005<0487:CAMTPG>2.0.CO;2
- Kaspar, F., Andersson, A., Ziese, M., & Hollmann, R. (2022). Contributions to the improvement of climate data availability and quality for sub-saharan Africa. Frontiers in Climate, 3, 815043. https://doi.org/10.3389/fclim.2021.815043
- Kassahun, B. 1987. “Weather systems over Ethiopia.” Proc. First Tech. Conf. on Meteorological Research in Eastern and Southern Africa, Nairobi, Kenya, UCAR.
- Khan, S. I., Hong, Y., Wang, J., Yilmaz, K. K., Gourley, J. J., Adler, R. F., Robert Brakenridge, G., Policelli, F., Habib, S., & Irwin, D. (2010). Satellite remote sensing and hydrologic modeling for flood inundation mapping in Lake Victoria basin: Implications for hydrologic prediction in ungauged basins. IEEE Transactions on Geoscience and Remote Sensing, 49(1), 85–95. https://doi.org/10.1109/TGRS.2010.2057513
- Kidd, C., Bauer, P., Turk, J., Huffman, G. J., Joyce, R., Hsu, K.-L., & Braithwaite, D. (2012). Intercomparison of high-resolution precipitation products over northwest Europe. Journal of Hydrometeorology, 13(1), 67–83. https://doi.org/10.1175/JHM-D-11-042.1
- Korecha, D., & Barnston, A. G. (2007). Predictability of June–September rainfall in Ethiopia. Monthly Weather Review, 135(2), 628–650. https://doi.org/10.1175/MWR3304.1
- Korecha, D., & Sorteberg, A. (2013). Validation of operational seasonal rainfall forecast in Ethiopia. Water Resources Research, 49(11), 7681–7697. https://doi.org/10.1002/2013WR013760
- Koukoula, M., Nikolopoulos, E. I., Dokou, Z., & Anagnostou, E. N. (2020). Evaluation of global water resources reanalysis products in the upper Blue Nile River Basin. Journal of Hydrometeorology, 21(5), 935–952. https://doi.org/10.1175/JHM-D-19-0233.1
- Kumar, S., Amarnath, G., Ghosh, S., Park, E., Baghel, T., Wang, J., Pramanik, M., & Belbase, D. (2022). Assessing the performance of the satellite-based precipitation products (SPP) in the data-sparse Himalayan Terrain. Remote Sensing, 14(19), 4810. https://doi.org/10.3390/rs14194810
- Lakew, H. B., Ayalew Moges, S., & Hailu Asfaw, D. (2020). Hydrological performance evaluation of multiple satellite precipitation products in the upper Blue Nile basin, Ethiopia. Journal of Hydrology: Regional Studies, 27, 100664. https://doi.org/10.1016/j.ejrh.2020.100664
- Maggioni, V., Meyers, P. C., & Robinson, M. D. (2016). A review of merged high-resolution satellite precipitation product accuracy during the tropical rainfall measuring mission (TRMM) era. Journal of Hydrometeorology, 17(4), 1101–1117. https://doi.org/10.1175/JHM-D-15-0190.1
- Malede, D. A., Alamirew Agumassie, T., Rotich Kosgei, J., Pham, Q. B., & Andualem, T. G. (2022). Evaluation of satellite rainfall estimates in a rugged topographical basin over south Gojjam Basin, Ethiopia. Journal of the Indian Society of Remote Sensing, 50(7), 1333–1346. https://doi.org/10.1007/s12524-022-01530-x
- Moazami, S., & Najafi, M. R. (2021). A comprehensive evaluation of GPM-IMERG V06 and MRMS with hourly ground-based precipitation observations across Canada. Journal of Hydrology, 594, 125929. https://doi.org/10.1016/j.jhydrol.2020.125929
- Moges, D. M., Kmoch, A., & Uuemaa, E. (2022). Application of satellite and reanalysis precipitation products for hydrological modeling in the data-scarce Porijõgi catchment, Estonia. Journal of Hydrology: Regional Studies, 41, 101070. https://doi.org/10.1016/j.ejrh.2022.101070
- Nguyen, P., Ombadi, M., Sorooshian, S., Hsu, K., AghaKouchak, A., Braithwaite, D., Ashouri, H., & Rose Thorstensen, A. (2018). The PERSIANN family of global satellite precipitation data: A review and evaluation of products. Hydrology and Earth System Sciences, 22(11), 5801–5816. https://doi.org/10.5194/hess-22-5801-2018
- Nguyen, P., Shearer, E. J., Tran, H., Ombadi, M., Hayatbini, N., Palacios, T., Huynh, P., Braithwaite, D., Updegraff, G., Hsu, K., Kuligowski, B., Logan, W. S., & Sorooshian, S. (2019). The CHRS data portal, an easily accessible public repository for PERSIANN global satellite precipitation data. Scientific Data, 6(1), 1–10. https://doi.org/10.1038/sdata.2018.296
- Oestigaard, T., & Gedef, A. F. (2011). Gish Abay: The source of the Blue Nile. Water and Society, 153, 27–38. https://doi.org/10.2495/WS110031
- Perera, H., Fernando, S., Gunathilake, M. B., Sirisena, T. A. J. G., & Rathnayake, U. (2022). Evaluation of satellite rainfall products over the Mahaweli River Basin in Sri Lanka. Advances in Meteorology, 2022, 1–20. https://doi.org/10.1155/2022/1926854
- Rachdane, M., Mahdi El Khalki, E., Elmehdi Saidi, M., Nehmadou, M., Ahbari, A., & Tramblay, Y. (2022). Comparison of high-resolution satellite precipitation products in sub-saharan morocco. Water, 14(20), 3336. https://doi.org/10.3390/w14203336
- Raes, D., Willems, P., & Gbaguidi, F. 2006. “RAINBOW–A software package for hydrometeorological frequency analysis and testing the homogeneity of historical data sets.” Proceedings of the 4th International Workshop on Sustainable management of marginal drylands. Islamabad, Pakistan.
- Reda, K. W., Liu, X., Tang, Q., & Gebretsadkan Gebremicael, T. (2021). Evaluation of global gridded precipitation and temperature datasets against gauged observations over the Upper Tekeze River Basin, Ethiopia. Journal of Meteorological Research, 35(4), 673–689. https://doi.org/10.1007/s13351-021-0199-7
- Retalis, A., Katsanos, D., Michaelides, S., & Tymvios, F. (2022). Evaluation of high-resolution satellite precipitation data over the Mediterranean Region. In Precipitation science (pp. 159–175). Elsevier. https://doi.org/10.1016/B978-0-12-822973-6.00017-2
- Retalis, A.s, Katsanos, D., Tymvios, F., and Michaelides, S. (2020). Comparison of GPM IMERG and TRMM 3B43 Products over Cyprus. Remote Sensing, 12(19), 3212. https://doi.org/10.3390/rs12193212
- Ruidas, D., Chakrabortty, R., Reza Md Towfiqul Islam, A., Saha, A., & Chandra Pal, S. (2022). A novel hybrid of meta-optimization approach for flash flood-susceptibility assessment in a monsoon-dominated watershed, Eastern India. Environmental Earth Sciences, 81(5), 145. https://doi.org/10.1007/s12665-022-10269-0
- Sahlaoui, Z., & Mordane, S. (2019). Radar rainfall estimation in Morocco: Quality control and gauge adjustment. Hydrology, 6(2), 41. https://doi.org/10.3390/hydrology6020041
- Sahlu, D., Moges, S. A., Nikolopoulos, E. I., Anagnostou, E. N., & Hailu, D. (2017). Evaluation of high-resolution multisatellite and reanalysis rainfall products over East Africa. Advances in Meteorology, 2017, 1–14. https://doi.org/10.1155/2017/4957960
- Sahlu, D., Nikolopoulos, E. I., Moges, S. A., Anagnostou, E. N., & Hailu, D. (2016). First evaluation of the day-1 IMERG over the upper Blue Nile basin. Journal of Hydrometeorology, 17(11), 2875–2882. https://doi.org/10.1175/JHM-D-15-0230.1
- Segele, Z. T., Richman, M. B., Leslie, L. M., & Lamb, P. J. (2015). Seasonal-to-interannual variability of ethiopia/horn of Africa monsoon. Part II: Statistical multimodel ensemble rainfall predictions. Journal of Climate, 28(9), 3511–3536. https://doi.org/10.1175/JCLI-D-14-00476.1
- Seleshi, Y., & Zanke, U. (2004). Recent changes in rainfall and rainy days in Ethiopia. International Journal of Climatology: A Journal of the Royal Meteorological Society, 24(8), 973–983. https://doi.org/10.1002/joc.1052
- Serrat-Capdevila, A., Merino, M., Valdes, J. B., & Durcik, M. (2016). Evaluation of the performance of three satellite precipitation products over Africa. Remote Sensing, 8(10), 836. https://doi.org/10.3390/rs8100836
- Sharifi, E., Steinacker, R., & Saghafian, B. (2016). Assessment of GPM-IMERG and other precipitation products against gauge data under different topographic and climatic conditions in Iran: Preliminary results. Remote Sensing, 8(2), 135. https://doi.org/10.3390/rs8020135
- Sharma, S., Khadka, N., Hamal, K., Shrestha, D., Talchabhadel, R., & Chen, Y. (2020). How accurately can satellite products (TMPA and IMERG) detect precipitation patterns, extremities, and drought across the Nepalese Himalaya? Earth & Space Science, 7(8), e2020EA001315. https://doi.org/10.1029/2020EA001315
- Sisay, K., Thurnher, C., & Hasenauer, H. (2017). Daily climate data for the Amhara region in Northwestern Ethiopia. International Journal of Climatology, 37(6), 2797–2808. https://doi.org/10.1002/joc.4880
- Stern, R., Rijks, D., Dale, I., & Knock, J. (2006). Instat climatic guide. Statistical Services Centre. Reading University.
- Tadesse, T. 1994. “The influence of the Arabian Sea storms/depressions over the Ethiopian weather.” Proc. Int. Conf. on Monsoon Variability and Prediction. Geneva, Switzerland. WCRP-84 and WMO Tech. Doc. 619. World Meteorological Organization: 228–236.
- Tapiador, F. J., Turk, F. J., Petersen, W., Hou, A. Y., García-Ortega, E., Machado, L. A., Angelis, C. F., Salio, P., Kidd, C., & Huffman, G. J. (2012). Global precipitation measurement: Methods, datasets and applications. Atmospheric Research, 104, 70–97. https://doi.org/10.1016/j.atmosres.2011.10.021
- Taye, M., Sahlu, D., Zaitchik, B. F., & Neka, M. (2020). Evaluation of satellite rainfall estimates for meteorological drought analysis over the upper Blue Nile basin, Ethiopia. Geosciences, 10(9), 352. https://doi.org/10.3390/geosciences10090352
- Thiemig, V., Rojas, R., Zambrano-Bigiarini, M., & De Roo, A. (2013). Hydrological evaluation of satellite-based rainfall estimates over the volta and baro-akobo basin. Journal of Hydrology, 499, 324–338. https://doi.org/10.1016/j.jhydrol.2013.07.012
- Tong, K., Fengge, S., Yang, D., & Hao, Z. (2014). Evaluation of satellite precipitation retrievals and their potential utilities in hydrologic modeling over the Tibetan Plateau. Journal of Hydrology, 519, 423–437. https://doi.org/10.1016/j.jhydrol.2014.07.044
- Wang, Y., & Qiaoyan, W. (2022). Comparison of multi-Satellite precipitation data from the global precipitation measurement mission and tropical rainfall measurement mission datasets: Seasonal and diurnal cycles. Advances in Meteorology, 2022, 1–20. https://doi.org/10.1155/2022/6404243
- Wedajo, G. K., Kebede Muleta, M., & Gessesse Awoke, B. (2021). Performance evaluation of multiple satellite rainfall products for Dhidhessa River Basin (DRB), Ethiopia. Atmospheric Measurement Techniques, 14(3), 2299–2316. https://doi.org/10.5194/amt-14-2299-2021
- WMO, World Meteorological Organization. (2017). WMO guidelines on the calculation of climate normals. World Meteorological Organization.
- WMO, World Meteorological Organization. (2020). State of climate in Africa 2019, World Meteorological Organization, WMO–No. 1275.
- Yilma, A. D., & Bekele Awulachew, S. (2009). Characterization and Atlas of the Blue Nile Basin and its Sub basins.
- Yilmaz, K. K., Hogue, T. S., Hsu, K.-L., Sorooshian, S., Gupta, H. V., & Wagener, T. (2005). Intercomparison of rain gauge, radar, and satellite-based precipitation estimates with emphasis on hydrologic forecasting. Journal of Hydrometeorology, 6(4), 497–517. https://doi.org/10.1175/JHM431.1