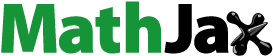
ABSTRACT
Soil moisture is a critical environmental variable that determines primary productivity and contributes to climatic processes. It is, therefore, important to forecast soil moisture to inform expectations of derivative outputs reliably. While forecasting soil moisture continues to advance, there is a need to extend it to different geoclimatic regions, including in sub-Saharan Africa, where livelihoods predominantly rely on subsistence agriculture. We used remotely sensed soil moisture data produced by the European Space Agency – Climate Change Initiative (ESA CCI). The data, which covered the period 1978 to 2019, were used to forecast monthly soil moisture in different agroecological zones and land cover types. The Seasonal Random Walk, Exponential Smoothing and Seasonal Autoregressive Integrated Moving Average (SARIMA) forecasting models were trained on 70% of the data (November 1978 – August 2007) and subsequently applied to a test dataset (September 2007 – December 2019). All models showed solid prediction accuracies for all agroecological zones (unbiased root mean square error, ubRMSE ≤ 0.05 m3 m−3) and land cover types (ubRMSE ≤ 0.04 m3 m−3). This was corroborated by similarities in season-adjusted anomalies between observed and forecasted soil moisture for nearly all agroecological zones and land cover types, with a correlation coefficient of r > 0.5 for most locations). The broad-scale interpretation of soil moisture forecasting can inform moisture availability and variability by regions; however, more research is encouraged to improve forecasting at spatially and temporally detailed levels to assist small-scale farming practices in the continent.
Introduction
Soil moisture is a crucial resource that serves as an immediate and main source of water for vegetation growth (Entekhabi et al., Citation2010; Petropoulos et al., Citation2015; Robock et al., Citation2000). In addition, it forms part of hydrological systems and thus controls the exchange of energy between the atmosphere and terrestrial ecosystems (Robinson et al., Citation2008; Seneviratne et al., Citation2010; Vereecken et al., Citation2008). Due to its vital ecosystem services and climate-moderating functions, soil moisture is recognized as an Essential Climate Variable that supports the goals of the United Nations Framework Convention on Climate Change (UNFCCC) and the Intergovernmental Panel on Climate Change (IPCC) (Bojinski et al., Citation2014). Understanding the amount and dynamics of soil moisture is vital to inform the determination of land capability for a specific purpose and to assist in the modelling of hydrological and energy cycles between land and the atmosphere (Agustí‐Panareda et al., Citation2010; Koster et al., Citation2009; Seneviratne et al., Citation2010). Traditional soil moisture monitoring method involves regular recording at strategically located sampling points (Robock et al., Citation2000). While field-based observations remain a reliable method for quantifying and monitoring soil moisture, they are limited to sampling locations (Seneviratne et al., Citation2010; Susha Lekshmi et al., Citation2014). Therefore, these methods fail to capture the spatially distributed nature of soil moisture caused by variations in landscape characteristics such as topography, vegetation composition, soil type and hydrology (Zhang et al., Citation2019). Moreover, field surveys involve substantial finance and labour to gather representative information from large spatial areas. On the other hand, remote sensing enables a synoptic observation of large spatial areas in a timely and cost-effective manner. Various space-borne sensors have been used to derive soil moisture-related information. Examples of widely used sensors to retrieve soil moisture globally include Soil Moisture Active Passive (SMAP), Advanced Microwave Scanning Radiometer (AMSR), Advanced Scatterometer (ASCAT), Soil Moisture and Ocean Salinity (SMOS) and Sentinel-1. Sabaghy et al. (Citation2018) provide an overview of the remote sensing systems and their inherent data interpretation principles for characterizing soil moisture.
Remotely sensed soil moisture data have been applied to different applications such as irrigation monitoring (e.g. Brocca et al., Citation2018), drought mapping (e.g. Liu et al., Citation2019), crop yield modelling (e.g. Gibon et al., Citation2018), agricultural risk monitoring (e.g. Champagne et al., Citation2015), vegetation status estimation (e.g. Boke-Olén et al., Citation2018) and runoff simulation (e.g. Liu et al., Citation2018), among others. The frequent data acquisition mode offered by remote sensing is ideal to characterize the time-series process of soil moisture. However, improving the accuracy of capturing the time-series process of soil moisture using remote sensing continues to be the focus of research (Fu et al., Citation2014; Konings et al., Citation2017; Loew & Schlenz, Citation2011; Zwieback et al., Citation2018). One of the improvements in soil moisture estimation is aligned with advances in spatial resolution of remotely sensed data (Babaeian et al., Citation2019; Korres et al., Citation2015). For example, Sadeghi et al. (Citation2017) introduced OPtical TRApezoid Model (OPTRAM) that exploits the relationship between the Normalized Difference Vegetation Index (NDVI) and shortwave infrared bands of remotely sensed data to estimate soil moisture. This model has two benefits: firstly, it captures the temporal dynamics of soil moisture through reliance on the physical evidence of NDVI, and secondly, it can provide a high level of spatial detail considering the prevalence of high-spatial-resolution optical data worldwide. However, such an approach remains limited to opportune atmospheric weather conditions that affect the quality of optical data. Therefore, remote sensing systems that use high-resolution radar sensors are the ideal alternative to optical remote sensing. In this regard, downscaling coarse-resolution data and advanced high-spatial resolution images such as Sentinel-1 is becoming an important source of data for mapping soil moisture from the local to regional scale (Huang et al., Citation2020; Ma et al., Citation2020; Meyer et al., Citation2021; Vergopolan et al., Citation2021).
Another important approach to improve soil moisture estimation is by factoring in land cover types and climatic scenarios (Baik et al., Citation2019; Celik et al., Citation2022; Dente et al., Citation2013; González-Zamora et al., Citation2019; Wang et al., Citation2018; Zohaib et al., Citation2017). These scenarios influence moisture holding capacity of soil through processes such as evapotranspiration, energy flux, infiltration and percolation, among others (Seneviratne et al., Citation2010). For example, Feng and Liu (Citation2015) showed the variation in the relationship between climatic variables (precipitation and temperature) and soil moisture for different land cover types in a humid environment in China. Their study, however, was limited to correlation analysis rather than a forecasting exercise. Wang et al. (Citation2018) performed a time-series analysis of soil moisture grouped by land cover types and noted variations of overall trends with land cover types. They also revealed the differential influence of precipitation and temperature on soil moisture variation. Zohaib et al. (Citation2017) studied the spatiotemporal pattern of root zone soil moisture (RZSM) stratified by major climatic zones in East Asia and reported RZSM trends being dependent on climatic zone. They also showed the temporal correlation of RZSM with climatic variables, including precipitation, skin (radiometric) temperature and actual evapotranspiration in each climatic zone.
While soil moisture estimation has improved significantly with remote sensing techniques, variation in uncertainty along land cover and climate gradients remains one area of focus for improvement (Dorigo et al., Citation2010; Kim et al., Citation2015; Pierdicca et al., Citation2015). In addition, the complex interplay of landscape factors controlling soil moisture characteristics (Di et al., Citation2019; Zwieback et al., Citation2018) suggests the need to explore soil moisture dynamics in different climatic and land cover setups. The above studies imply the need to investigate the time-series patterns of soil moisture in different environmental regions of the world, considering variations in climatic and landscape characteristics. In earlier work, Legates et al. (Citation2010) highlighted the complexity of soil moisture driven by the spatial variations in biogeographical and climatic factors and suggested more research efforts to improve soil moisture monitoring. One of the suggestions refers to the need for improving modelling performances in regional-level assessments. This recommendation, though it applies to a variety of surface models, serves well to address the challenges of soil moisture estimation. The second recommendation encourages for developing soil moisture forecasting capacity. This study, therefore, aims to forecast soil moisture amounts in sub-Saharan Africa at broad land cover and agroecological scales using monthly time-series data spanning nearly four decades. The forecasting exercise in this study uses aggregated data at broad management units (agroecological and land cover zones). This approach indeed conceals localized variations; however, it is helpful as a quick indicator of regional-scale soil moisture patterns (Sehler et al., Citation2019; Seneviratne et al., Citation2010) that, in turn, aid in the prediction of other derivatives such as primary productivity and drought occurrences (McNally et al., Citation2016; Milly et al., Citation2008).
Materials
AEZs and land cover types of sub-Saharan Africa (SSA)
An agroecological zone (AEZ) represents the agricultural potential of an area using its climatic characteristics and available natural resources (Fischer et al., Citation2000; Snapp, Citation2017). Agro-ecology incorporates the socio-economic values of land and the ecosystem services it offers to humans and the environment. Agroecological zones can be delineated at different spatial scales ranging from global to localized levels (Fischer et al., Citation2000). This study used the AEZs created specifically for SSA (IFPRI, Citation2015). Assessing soil moisture across AEZs is relevant since the zonation is determined by the growth period for crops, climate and altitude (Dudal, Citation1980; FAO, Citation1978). The climate and soil conditions, including the amount of soil moisture available for crop growth, fundamentally define the growth period. According to the latest zonation (IFPRI, Citation2015), nine broad AEZs are represented in SSA (). These include Arid, Semi-Arid, Tropical Cool Arid, Tropical Cool Semi-Arid, Sub-Tropical, Humid, Sub-Humid, Tropical Cool Humid and Tropical Cool Sub-Humid.
We used the land cover map of the SSA produced as part of the Global Land Cover 2000 mapping project (). The map was created using multi-source remote sensing data, including SPOT VEGETATION, Japan Earth Resources Satellite (JERS-1) radar, European Remote Sensing (ERS) radar, Defence Meteorological Satellite Program (DMSP), Operational Linescan System (OLS) and digital elevation model (Bartholomé & Belward, Citation2005; Mayaux et al., Citation2003). Like the AEZs map, the land cover map had a spatial resolution of 1 km. Although this resolution is acknowledged as too coarse for Africa, where mixed land cover is common at localized scales, it provides a good indication of generic classes at broad spatial scales such as the one used in the present study. The map classifies SSA into six generic land cover types, five of which were used in the present study: agriculture, bare soil, forests, grasslands and woodlands/shrublands. The sixth land cover type representing water bodies was excluded from the analysis since it does not represent surface soil.
Soil moisture data
Soil moisture data covering the period November 1978 – December 2019 at a spatial resolution of ~25 km were obtained from the European Space Agency – Climate Change Initiative (ESA CCI, http://www.esa-soilmoisture-cci.org) and represented the latest version (version 04.2) in a series of releases (Dorigo et al., Citation2017; Gruber et al., Citation2017, Citation2019). The soil moisture coverage was derived by merging active and passive C-band microwave sensors at the global scale (Dorigo et al., Citation2015; Kidd and Haas, Citation2017). The data provide volumetric soil moisture for the top 2 cm of surface soil (Kidd and Haas, Citation2017). Validation studies have shown the ESA CCI soil moisture data’s accuracy compared to in situ observations (e.g. Dorigo et al., Citation2015; González-Zamora et al., Citation2019; McNally et al., Citation2016). Furthermore, studies have reported the product’s superiority over other remote-sensing-based soil moisture estimation methods (e.g. Jing et al., Citation2018; Qiu et al., Citation2016; Zeng et al., Citation2015). González-Zamora et al. (Citation2019) also showed the accuracy of the data across different environmental conditions in Spain. Although retrieval algorithms, sensor transitions and instrumental inconsistency may cause uncertainty (Su et al., Citation2016), the error level in soil moisture does not exceed the acceptable limit of 0.003 m3 m−3 yr−1 (Global Climate Observing System, Citation2011). In this study, we compared the data with in-situ observations taken at 22 sites collected from COSMOS, PBO H2O, AMMA-CATCH, CARBOAFRICA and DAHRA networks (https://ismn.geo.tuwien.ac.at/en/networks/) located along the central (northern part of SSA) and southern African region (Dorigo et al., Citation2011; Dorigo, Wagner et al., Citation2011). The comparison showed a strong correlation (Pearson’s correlation, r ≥ 0.61) across sites with r > 0.75 for 15 sites.
Individual daily soil moisture coverages were spatially incomplete due to orbital differences of the multiple satellites that acquired the data (Dorigo et al., Citation2015). As a result, each pixel location was quantified as an average of the days when there were records of the monthly soil moisture at each pixel location. We used the first month (November 1978) as a reference based on which the pixels of all the other months (until December 2019) needed to coincide spatially. In enforcing this matching, missing values were imputed for each month by using a combination of Bayesian kriging and regression available in ArcGIS Pro (ESRI® ArcGIS Pro, version 9.2). Generically, spatial interpolation estimates values at unknown locations using values from known locations by assuming that features closer to each other are more similar than those farther apart. Unlike other interpolation methods, kriging provides a measure of estimation uncertainty and spatial correlation of data (as opposed to input from the analyst) to assign weights to observations (Cressie, Citation1990; Goovaerts, Citation2019). We specifically chose the Bayesian kriging over the other variants of the interpolator as it uses a large number of simulations to optimize the kriging model parameters without the need for manual adjustment (Krivoruchko & Gribov, Citation2019). For the regression component, we used precipitation data obtained from WorlClim for the 1978–1980 period (Fick & Hijmans, Citation2017) and the Climate Hazards Infrared Precipitation with Stations (CHIRPS) for the 1981–2019 period (Funk et al., Citation2015). The combination of kriging (spatial autocorrelation) and regression that used precipitation as a predictor was preferred to limit the sole influence of each approach. Subsequently, the monthly soil moisture data were analyzed at the pixel-level to maintain localized information in a modelling exercise that included training and testing using holdout data. Validation of the imputation at known locations showed an R2 of 0.99 for each month. The resultant pixel-level model outputs were spatially averaged per AEZ and land cover type to answer the study’s objective. provides a summary of the methodology followed in the study.
Methods
We explored three statistical methods used to forecast soil moisture data. These included the Seasonal Random Walk, Seasonal Autoregressive Integrated Moving Average (SARIMA) and Exponential Smoothing models. Prior to implementing the models, we ascertained for data stationarity in which the mean, variance and autocorrelation are constant throughout the data. Subsequently, we divided the dataset into two parts: training and testing sets. The training set contained the first 70% of the time series (November 1978 – August 2007) and the remaining 30% (September 2007 – December 2019) was set aside for testing the three models. Details of the modelling exercises are given in the following sub-sections.
Random walk model
The Random Walk represents the most straightforward and basic approach to forecasting a time-series process on which the other two methods and many others are built (Fildes & Kourentzes, Citation2011; Green et al., Citation2009). It found prominence after applications in statistical physics, such as the Brownian motion (Kac, Citation1947; Weiss, Citation1983), and has since been widely used in zoology (Bearup & Petrovskii, Citation2015), ecology (Ahmed et al., Citation2021), climate (Yan & Wu, Citation2010) and hydrology (Yang & Liang, Citation2020), among others. Essentially, the simple Random Walk modelling traces the dynamics of a process by assuming each step taken at a given time to be unbiased and unrelated to the previous event (Lin & Segel, Citation1974). This assumption renders the approach largely independent of the temporal resolution of the time-series data (Bearup & Petrovskii, Citation2015). The model’s simplicity, coupled with its favorable accuracies, makes it popular in different fields. We applied a simple Random Walk in the present study to see how efficiently the model can capture monthly soil moisture dynamics. As such, the exploration will inform if the dynamics reflect random fluctuations at each time step. The Random Walk model can be described as follows:
where is soil moisture at time t,
is soil moisture at time t-1 and
is a Gaussian white noise series with mean zero and variance
.
A seasonal random walk is a counterpart to a random walk in the periodic domain. Typically, it is a non-stationary process and can be described by
with It is seasonally integrated with order one since its seasonal difference is the white noise process
. In this study, s = 12 since we are analyzing a monthly seasonal time series. Combining the non-seasonal random walk model (EquationEquation 1
(1)
(1) ) and the seasonal random walk model (EquationEquation 2
(2)
(2) ) results in the equation:
where is the value of the soil moisture from the same season in the previous year, and
is the value of the soil moisture from the previous season in the previous year. For example, for the monthly soil moisture data, the seasonal effect for October 2018 would be the seasonal effect for October 2017, plus some random mean-zero white noise process. This approach works well in our case as it accounts for the natural variation that happens over a 12-month period when using monthly soil moisture data. The seasonal random walk model was developed using the training sample dataset using the “forecast” package (Hyndman et al., Citation2021) in R 4.1.2 (R Core Team, Citation2021).
SARIMA model
SARIMA is a time-series model that considers the random nature and time dependence of variables under study. Research has shown the suitability of this model for time-series analysis of climate data, including soil moisture that is influenced by season-dependent patterns (e.g. Li et al., Citation2003). We applied the SARIMA model in the current study to capture the potential effect of climate seasonality on soil moisture. As stated in Shumway and Stoffer (Citation2016), a time-series , for t = 1, … , n, that follows a SARIMA model can be expressed as follows:
where is the seasonal autoregressive polynomial and
is the non-seasonal autoregressive polynomial. B is the backshift operator, while s represents the number of observations per season. The operator Brepresents a one-period lag in a time series. That is, given a time series
, the backshift operator B applied to
yields
(expresses as
). This enables us to investigate how changes in a variable at one point impact its values in the future. This principle can be extended to increase the lag time, such as
for two-time lags. As in the case of Random Walk modelling, we used s = 12 in this research. d and D are the differencing terms for the non-seasonal and seasonal orders, respectively.
is the constant term;
is seasonal moving average polynomial and
is the non-seasonal moving average polynomial. The model in EquationEquation 4
(4)
(4) can also be expressed using a standard ARIMA model with a seasonal component added to it as
model, where p and P are the autoregressive terms for the non-seasonal and seasonal orders, respectively. Similarly, q and Q represent the moving average terms for the non-seasonal and seasonal orders, respectively.
We used the Hyndman-Khandakar algorithm to fit the best SARIMA model for the training sample set (Hyndman & Khandakar, Citation2008; Hyndman et al., Citation2021). The algorithm was applied in R 4.1.2 (R Core Team, Citation2021) using the “auto.arima” function to search for the optimal p, d, q, P, D and Q values (Hyndman et al., Citation2021) and the corrected Akaike Information Criterion (AICc) (Hurvich & Tsai, Citation1989) was used to help choose the most suitable model. The algorithm uses maximum likelihood estimation (MLE), Kwiatkowski Phillips Schmidt Shin (KPSS) stationarity testing (Kwiatkowski et al., Citation1992) and AICc to determine the best SARIMA model. The following formula is used to calculate the AICc:
where k is the number of parameters, and n represents the sample size.
Exponential smoothing model
Unlike a simple moving average time-series modelling, Exponential Smoothing apportions differential weighting to past observations when forecasting the future (Hyndman & Athanasopoulos, Citation2018). Specifically, the most recent observation carries the greatest weight that decreases exponentially for the older observations. This approach can be used to forecast climatic variables that often follow a temporal sequence of events (e.g. Diodato et al., Citation2019; Papacharalampous et al., Citation2018). Therefore, it is justified to hypothesize that the amount of soil moisture at a given time reflects the amount of the most recent past, while the similarity decreases to the point of insignificance with time. A time-series (for t = 1, …, n) can be decomposed into trend, seasonal and irregular (or noise) components as follows:
where and
are the trend, seasonal and irregular components of the observed time-series, respectively. For a model defined as
,
can be expressed as a weighted average of
and
:
where is the weight (smoothing constant).
EquationEquation 7(7)
(7) is a simple (or single) Exponential Smoothing model commonly used to forecast a series when there is no trend. In the presence of a trend in a series, EquationEquation 7
(7)
(7) can be modified as follows:
where the second update for the trend can be defined as
EquationEquations 8(8)
(8) and Equation9
(9)
(9) together are known as the Holt-Winters model (Mills, Citation2019). We can incorporate seasonality further in the series by updating the Holt-Winters framework as follows:
where the seasonal updating equation is defined as
The Holt-Winters exponential smoothing model was fitted for the training sample dataset using the “forecast” package (Hyndman et al., Citation2021) in R 4.1.2 (R Core Team, Citation2021). Like in the SARIMA modelling, the exponential smoothing model uses MLE to estimate the values of the smoothing parameters (such as α, β and γ).
Accuracy assessment
The present study developed the models to forecast soil moisture using the training dataset (November 1978 – August 2007) of the data. Rolling window estimation was applied to read the data over time in all the modelling approaches (Seasonal Random Walk, SARIMA and Exponential Smoothing). The rolling window was preferred to the fixed window method since it accommodates parameter instability expected to occur with time (Balcilar et al., Citation2014; Clark & McCracken, Citation2009). The trained models were subsequently applied to a 30% holdout validation dataset (September 2007 – December 2019). Since the validation dataset does not participate in model development, it provides an unbiased indication of model performances. We, therefore, used the validation data to evaluate the qualities of the models using the unbiased root mean square error (ubRMSE) that is widely used in time-series forecasting efforts. The ubRMSE, is recommended for assessing soil moisture evolution since it corrects for extreme biases in the mean or amplitude of estimations that are otherwise unaccounted for in RMSE (Entekhabi et al., Citation2010; Rao et al., Citation2022). The ubRMSE can therefore be computed from RMSE as in EquationEquation 12.(12)
(12)
for and
where and
are the forecasted and observed values, respectively. We also used correlation coefficient, r, to compare the degree of similarity between predicted and observed soil moisture data (Dorigo et al., Citation2015; Entekhabi et al., Citation2010). The standard correlation statistic is affected by severe soil moisture fluctuations caused by seasonal variations (Liu et al., Citation2012). We, therefore, added a correlation analysis comparing anomalies of observed and forecasted values commonly used in time-series of soil moisture (Dorigo et al., Citation2010, Citation2015; Entekhabi et al., Citation2010). An anomaly interprets soil moisture at a given time against one representing a longer-term pattern. Therefore, it is computed as the difference between the soil moisture at a given month and the average soil moisture computed from a moving window of months, including that month’s temporally averaged soil moisture (Dorigo et al., Citation2010, Citation2015). We used a temporal average of soil moisture using a three-month moving window by assuming 4-seasons-per-year scenario.
Results
Forecast accuracy by agroecological zone
Pixel-level ubRMSE of monthly soil moisture forecasting for September 2007 – December 2019 (148 months) is shown in . Generally, there was a certain level of agreement in terms of accuracy among the three forecasting approaches. The pixel-level averages were then aggregated by AEZs (). The ubRMSE never exceeded 0.05 m3 m−3, with the humid zones showing comparable forecasting errors compared to the drier aridic zones. A relative comparison of the modelling approaches showed SARIMA and Exponential Smoothing models to yield lower estimation errors than the Seasonal Random Walk approach in each AEZ.
Figure 3. Pixel-level unbiased RMSE (ubRMSE) of monthly soil moisture forecasting using three modelling approaches implemented on test datasets (September 2007 – December 2019) of SSA.

Figure 4. Unbiased RMSE (ubRMSE) of monthly soil moisture forecasting using three modelling approaches implemented on test datasets (September 2007 – December 2019) per agroecological zone of SSA.

presents the time-series soil moisture patterns for the nine agroecological zones. In general, the three models were able to forecast soil moisture well in most agroecological zones. Agroecological zones with relatively weakly forecast temporal dynamics include Sub-Tropical, Tropical Cool Humid and, to a lesser extent, Arid regions. It is important to note that these specific agroecological zones show erratic variability of soil moisture dynamics that fluctuate at different (short- and long-term) intervals. Although the three approaches were quite successful in forecasting locally variable fluctuations, the Seasonal Random Walk method tended to over- and under-estimate in the extreme fluctuation cases in most of the AEZs.
Figure 5. Monthly soil moisture forecasted using three modelling approaches implemented on test datasets (September 2007 – December 2019) per AEZ of SSA.

The aggregation of the pixel-level anomaly correlations by AEZ is summarized in . More than 50% of the samples within each of the humid AEZs showed good agreement (r > 0.5) between the anomalies of the observed and modelled soil moisture using the three forecasting approaches (). A similar finding was obtained for Arid and Semi-Arid zones within the aridic AEZs. The mean correlations between anomalies revealed the comparability of accuracies across AEZs, with r exceeding 0.6 using the three modelling approaches ().
Figure 6. Correlations of anomalies between observed and forecast soil moisture using three modelling approaches implemented on test datasets (September 2007 – December 2019) per AEZ of SSA. (a) the proportion of samples (pixels) with a correlation greater than 0.5 within each AEZ; (b) mean correlation.

Forecast accuracy by land cover type
The overall soil moisture forecasting errors per land cover type were low with ubRMSE never exceeding 0.04 m3 m−3 (). Similar to the observation in the estimation per AEZ, the SARIMA and Exponential Smoothing models proved to be the best models in nearly all land cover types, though marginally. The time-series patterns shown in reveal detailed estimation accuracies obtained using the three models per land cover type. For example, forecasting was highly accurate in agricultural, forest, woodland and bare soil land cover types for nearly the entire time period of September 2007 to December 2019, irrespective of the model type. In contrast, the weakest soil moisture forecasting accuracy was observed for grassland. It is also important to note the similarities of forecasting among the three approaches, such as those observed in Bare soil, Forest and Woodland during the last few months of the forecast period (). A comparison of the soil moisture estimations and observed values based on anomalies is shown in . The number of samples that had r > 0.5 between observed and estimated soil moisture exceeded 50% of all samples within each land cover type with the agriculture except in bareland in which only one modelling approach (exponential smoothing) yielded such a result (). The mean correlation between observed and estimated soil moisture anomalies within each land cover zone exceeded 0.7 (), indicating the stability of estimation across the season. This stability is similar to what was observed in the results comparing AEZs.
Figure 7. Unbiased RMSE (ubRMSE) of monthly soil moisture forecasting using four modelling approaches implemented on test datasets (September 2007 – December 2019) per land cover type of SSA.

Figure 8. Monthly soil moisture forecasted using three modelling approaches implemented on test datasets (September 2007 – December 2019) per land cover type of SSA.

Figure 9. Correlations of anomalies between observed and forecast soil moisture using three modelling approaches implemented on test datasets (September 2007 – December 2019) per land cover type of SSA. (a) proportion of samples (pixels) with a correlation greater than 0.5 within each land cover type; (b) mean correlation.

Discussion
Forecasting accuracies at aggregated AEZs and land cover types
Monitoring and forecasting soil moisture should be essential for hydrological, agricultural and environmental management efforts. The need for soil moisture prediction cannot be overemphasized for SSA where subsistent agriculture predominates (Bjornlund et al., Citation2020) and biodiversity loss threatens environmental sustainability (Mohammed, Citation2020; Scheren et al., Citation2021; UNEP-WCMC, Citation2016). In the present study, we modelled monthly soil moisture dynamics of SSA using ~40 years of remotely sensed data at the pixel level and aggregated the results at regional scales (agroecological and land cover zones). Soil moisture estimation models that were calibrated using the rolling window forecasting method and implemented on independent test datasets showed the reliability of generalizing soil moisture in broad agroecological zones. The forecasting can be considered accurate in most humid zones, while it was still encouraging for the aridic zones (). The high prediction accuracies in humid zones can be attributed to relatively dense vegetation that improves moisture-holding capacity (Feng, Citation2016). It is essential to highlight moderate underestimations for high soil moisture values in humid zones, such as between September 2016 and December 2019 (). Estimation uncertainty occurs when soil moisture amounts are high such as after dry (or relatively low moisture) periods. Almagbile et al. (Citation2019) found similar results in which lower estimation accuracies were observed in the wet than in the drier season within a humid study area. It is also important to recognise the potential limitation of all the models in handling major fluctuations that deviate significantly from long-term trends, although this argument must be explored with better statistical evidence. Still, these underpredictions (as opposed to overprediction) can be considered conservative estimations that prepare communities, particularly farmers, to be prudent in their expectations of soil moisture content. Such underpredictions are not expected in the drier (aridic) zones; this can be linked to the fact that the soil moisture in those regions is generally low. Therefore, any underprediction observed for certain times in the Tropical Cool, Arid and Sub-Tropical AEZs can be considered as deviations from long-term patterns. This can be ascertained by the fact that the underpredictions for the two specific AEZs were noted when soil moisture was above 0.15 m3 m−3.
Soil moisture information by land cover type should be interpreted carefully since the land cover type can obscure soil except in bare and agricultural lands during the off-season. However, knowledge of soil moisture per vegetation type remains relevant for regional or localized spatial scales (Feng, Citation2016). In the present study, soil moisture prediction was achieved at high accuracies within all land cover types and forecasting approaches (). The predictions were successful in forecasting even in highly variable scenarios observed in Bare soil, where soil moisture amount and range were the lowest (0.07–0.11 m3 m−3). The high forecasting accuracy in agricultural areas can be linked to the relatively predictable seasonal patterns or the management efforts by farmers to maintain cropping patterns such as routine irrigation applications, minimum tillage practices and mulching that likely guarantee consistent soil moisture over the years (Fér et al., Citation2020; García-Moreno et al., Citation2013). The high prediction accuracies in Forest and Woodland land cover types can be explained by the long-term sustenance of vegetation structure (as opposed to short-term harvesting of crops), which tends to stabilize soil moisture dynamics (Feng & Liu, Citation2015). It is important to note the low forecasting accuracy in forest and to a lesser extent in woodland zones especially at high values. This could be attributed to the higher complexity of vegetation forms than the land cover designation indicates. The LCCS classification used to produce the map classifies an area as forest cover if the tree cover >15% while the rest can be covered by woody savanna and savanna covers (Bartholomé & Belward, Citation2005). Similarly, woodlands consist of woody plants interspersed by savannas (Bartholomé & Belward, Citation2005). Such heterogenous land cover composition can complicate the soil moisture dynamics, making the forecasting accuracy relatively uncertain, especially when the soil moisture values are high. The high uncertainty of soil moisture estimation in forests compared to other land cover types such as crop lands was also shown by Celik et al. (Citation2022). The high level of soil moisture variability over time in grassland was the most difficult to reproduce (), although the mean ubRMSE was still encouraging at less than 0.04 m3 m−3 and comparable to the other land cover types (). Forecast uncertainty in grassland can be explained by high moisture variability within localized spatial scales (von Randow et al., Citation2012), and thus the broad-scale averaging used in our study could have contributed to the weakness of accuracy in this land cover type. In addition, the low soil moisture holding/retention capacity of grasslands (Chen et al., Citation2019) can add to a rapid temporal variability that may not have been captured well by the monthly scale analysis in our study.
Furthermore, the impacts of extreme weather episodes were neglected in our study. Herbst et al. (Citation2008) suggested that the impact of such episodes on soil moisture could be more significant than that of land cover type. This impact can most certainly be realized in the face of the increasing variability and unpredictability of the climate the world is experiencing (Green et al., Citation2019). In contrast, the impact of land cover on soil moisture outweighs that of weather factors under climatic conditions that fall within long-term patterns (Feng & Liu, Citation2015).
Effect of land cover dynamics on forecasting accuracy
Although not a stated objective, assessing the effect of land cover dynamics on soil moisture forecasting accuracy is worthwhile. By focusing on the period that was used for testing the forecasting models (2007–2019), we used Global Land Cover data updated in 2009 () and 2014 () to assess the forecasting accuracies. The maps clearly show shifts in land cover types with, for example, an increase in agriculture in 2014 compared with the year 2009. Similarly, a substantial increase in woodland/shrubland is observed, replacing grassland, particularly in the southern part of the continent. The land cover shifts are reflected in soil moisture variations in the 2 years, with significant differences observed in agriculture and woodland/shrubland land cover types (). In the agriculture zone, soil moisture remained stable across 2014 compared to 2009, while the opposite can be noted in the woodland zone. However, the forecast accuracies were high in all land cover types for both years despite the soil moisture dynamics. This is attributed to the fact that the forecasting exercise was applied at pixel level and thus the accuracy is determined at such level. Subsequent spatial aggregations are therefore unlikely to influence the forecast accuracies irrespective of potential land cover dynamism.
Way forward for improved soil moisture forecasting
One of the limitations of our study is the use of coarse spatial scale remote sensing data (25 km × 25 km) and the further aggregation to regional spatial zones. Such data obscure local variations that would have been captured using higher spatial resolution data. The spatial aggregation is relevant to provide a generic outlook on land cover types and ecological zones, although such zones are temporally and spatially dynamic (FAO, Citation2017). One practical solution in this regard is to use more detailed land cover classes and AEZ than those used in the present study. The problem concerning the spatial resolution of remote sensing data is solved by combining low-resolution data with high-resolution data (Babaeian et al., Citation2019; Korres et al., Citation2015; Sadeghi et al., Citation2017; Song et al., Citation2019). For example, Song et al. (Citation2019) integrated the 25 km soil moisture data derived from Advanced Microwave Scanning Radiometer-Earth Observing System-2 (AMSR-2) with NDVI and land surface temperature obtained from the Moderate Resolution Imaging Spectroradiometer (MODIS) to produce 1 km scale soil moisture data. A similar data fusion was adopted by Zhao et al. (Citation2021) to downscale the ESA CCI soil moisture data to 1 km scale. Such an approach can be expanded by including landscape features as covariates to downscale soil moisture data (Gaur & Mohanty, Citation2019; Zhao et al., Citation2018). For instance, Zhao et al. (Citation2018) combined MODIS derived optical data, vegetation indices, temperature and topographic data to downscale the 36 km Soil Moisture Active and Passive (SMAP) soil moisture data to 1 km scale. Another remote sensing system that allows for soil moisture mapping at all weather conditions are radar data. The increasing availability of high-spatial resolution radar data provides an ideal opportunity for spatially detailed mapping purposes (Cui et al., Citation2022; Zeyliger et al., Citation2022). High-spatial resolution forecasting is a key missing factor in the SSA context where agricultural fields in particular are small and fragmented (Giller et al., Citation2021).
We attempted to show the insensitivity of soil moisture forecasting to land cover dynamics if the estimation is implemented at the pixel level (). However, land cover type and its dynamics remain crucial variables that influence soil moisture content and, as such, can improve forecasting accuracy. Although certain landscape variables such as topography are considered time-invariant, their interactions with other non-constant biophysical and climatic characteristics (Bogena et al., Citation2010; Qiu et al., Citation2001) make them valid temporal inputs in soil moisture forecasting. Understanding land cover dynamics trends can therefore be considered in the forecasting process, however, this should be handled cautiously as the expected land cover change may not occur.
Adding biophysical variables such as topography and vegetation characteristics can improve soil moisture forecasting accuracy (Chen et al., Citation2019; Fathololoumi et al., Citation2021; Lawrence & Hornberger, Citation2007; Liang et al., Citation2017; Qiu et al., Citation2001; Vereecken et al., Citation2008; Yu et al., Citation2018). For example, Liang et al. (Citation2017) compared soil moisture content across topographic gradient and found a higher moisture content in a gully followed by a valley-head slope and side slope. Their study also showed the importance of factoring in soil porosity, hydraulic conductivity, vegetation density and basal area in quantifying soil moisture content. Similarly, Fathololoumi et al. (Citation2021) demonstrated the response of soil moisture contents to environmental and biophysical variations at different spatial scales, indicating the value of including such characteristics in soil moisture forecasting. It is also beneficial to incorporate resource utilization cultures in the forecasting process. For example, cropping practices such as crop type and tillage methods impact soil moisture retention capability by changing soil structures and, subsequently, capillaries that serve as a conduit for water movement (Gabriel et al., Citation2017; Hatfield et al., Citation2001). While including several influencing factors improve the accuracy of soil moisture forecasting, the complex interactions of those factors must be well understood to make robust conclusions and interpretations (Chen et al., Citation2019; Fathololoumi et al., Citation2021).
Conclusion
The present study applied three modelling methods (SARIMA, Exponential Smoothing and Random Walk) to estimate monthly soil moisture data using only antecedent soil moisture records as inputs. Although there were slight variations with SARIMA and the Exponential Smoothing performing better in terms of error, the methods generally agreed in forecast accuracies. This indicates that soil moisture forecasting may not be influenced significantly if well-established methods such as those used in this study are applied. All the models were able to forecast soil moisture using test data set (September 2007 – December 2019) at high accuracy levels at broad spatial scales, namely AEZ and land cover types of SSA. Relatively modest variations in estimation accuracy can be expected across AEZ, with the more humid and arid zones having the best accuracy compared to the other zones. Soil moisture forecasting by land cover type showed the best accuracies in Agriculture, Forest, Woodland and Bare soil, while estimations in most grassland areas were still encouraging. Season-normalized anomalies between observed and forecasted soil moisture were strongly correlated (), confirming the forecasting accuracies even with soil moisture fluctuations expected with seasonal changes. The broad spatial scale averaging adopted in the study provides a synoptic guide of soil moisture scenarios that can be used as inputs for other applications such as crop yield forecasting and drought prediction.
Author contributions
Solomon G. Tesfamichael: Conceptualization and writing original draft; Yegnanew A. Shiferaw: Data analysis; Tsehaie Woldai: Supervision and editing.
Acknowledgments
The University of Johannesburg (South Africa) provided the necessary financial and material support to undertake the study. We thank Adrian Snyman, Linux Systems Administrator, from Technology Architecture and Planning at the University of Johannesburg, on guiding as with cluster computation used to analyze our data. We would also like to thank the two anonymous reviewers whose comments resulted in the current improved version of the paper.
Disclosure statement
The authors declare that they have no known competing financial interests or personal relationships that could have appeared to influence the work reported in this paper.
References
- Agustí‐Panareda, A., Balsamo, G., & Beljaars, A. 2010. Impact of improved soil moisture on the ECMWF precipitation forecast in West Africa. Geophysical Research Letters, 37, L20808. doi.https://doi.org/10.1029/2010GL044748. 20
- Ahmed, D. A., Benhamou, S., Bonsall, M. B., & Petrovskii, S. V. (2021). Three-dimensional Random Walk models of individual animal movement and their application to trap counts modelling. Journal of Theoretical Biology, 524, 110728. https://doi.org/10.1016/j.jtbi.2021.110728
- Almagbile, A., Zeitoun, M., Hazaymeh, K., Sammour, H. A., & Sababha, N. 2019. Statistical analysis of estimated and observed soil moisture in sub-humid climate in north-western Jordan. Environmental Monitoring and Assessment, 191(96). https://doi.org/10.1007/s10661-019-7230-9.
- Babaeian, E., Sidike, P., Newcomb, M. S., Maimaitijiang, M., White, S. A., Demieville, J., Ward, R. W., Sadeghi, M., LeBauer, D. S., Jones, S. B., Sagan, V., & Tuller, M. (2019). A new optical remote sensing technique for high-resolution mapping of soil moisture. Frontiers in Big Data, 2, 37. https://doi.org/10.3389/fdata.2019.00037
- Baik, J., Zohaib, M., Kim, U., Aadil, M., & Choi, M. (2019). Agricultural drought assessment based on multiple soil moisture products. Journal of Arid Environments, 167, 43–568. https://doi.org/10.1016/j.jaridenv.2019.04.007
- Balcilar, M., van Eyden, R., Inglesi-Lotz, R., & Gupta, R. (2014). Time-varying linkages between tourism receipts and economic growth in South Africa. Applied Economics, 46(36), 4381–4398. https://doi.org/10.1080/00036846.2014.957445
- Bartholomé, E., & Belward, A. S. (2005). GLC2000: A new approach to global land cover mapping from Earth observation data. International Journal of Remote Sensing, 26(9), 1959–1977. https://doi.org/10.1080/01431160412331291297
- Bearup, D., & Petrovskii, S. (2015). On time scale invariance of Random Walks in confined space. Journal of Theoretical Biology, 367, 230–245. https://doi.org/10.1016/j.jtbi.2014.11.027
- Bjornlund, V., Bjornlund, H., & Van Rooyen, A. F. 2020. Why agricultural production in sub-Saharan Africa remains low compared to the rest of the world – a historical perspective. International Journal of Water Resources Development, 36(sup1) S20–S53. https://doi.org/10.1080/07900627.2020.1739512
- Bogena, H. R., Herbst, M., Huisman, J. A., Rosenbaum, U., Weuthen, A., & Vereecken, H. (2010). Potential of wireless sensor networks for measuring soil water content variability. Vadose Zone Journal, 9(4), 1002. https://doi.org/10.2136/vzj2009.0173
- Bojinski, S., Verstraete, M., Peterson, T. C., Richter, C., Simmons, A., & Zemp, M. (2014). The Concept of essential climate variables in support of climate Research, applications, and policy. Bulletin of the American Meteorological Society, 95(9), 1431–1443. https://doi.org/10.1175/BAMS-D-13-00047.1
- Boke-Olén, N., Ardo, J., Eklundh, L., Holst, T., Lehsten, V., & Bond-Lamberty, B. (2018). Remotely sensed soil moisture to estimate savannah NDVI. PLoS ONE, 13(7), e0200328. https://doi.org/10.1371/journal.pone.0200328
- Brocca, L., Tarpanelli, A., Filippucci, P., Dorigo, W., Zaussinger, F., Gruber, A., & Fernández-Prieto, D. (2018). How much water is used for irrigation? A new approach exploiting coarse resolution satellite soil moisture products. International Journal of Applied Earth Observation and Geoinformation, 73, 752–766. https://doi.org/10.1016/j.jag.2018.08.023
- Celik, M. F., Isik, M. S., Yuzugullu, O., Fajraoui, N., & Erten, E. 2022. Soil moisture prediction from remote sensing images coupled with climate, soil texture and topography via Deep Learning. Remote Sensing, 14, 5584. https://doi.org/10.3390/rs14215584. 21
- Champagne, C., Davidson, A., Cherneski, P., L’Heureux, J., & Hadwen, T. (2015). Monitoring agricultural risk in Canada using L-band passive microwave soil moisture from SMOS. Journal of Hydrometeorology, 16(1), 5–18. https://doi.org/10.1175/JHM-D-14-0039.1
- Chen, Y., Xia, J., Zhao, X., & Zhuge, Y. (2019). Soil moisture ecological characteristics of typical shrub and grass vegetation on Shell Island in the Yellow River Delta, China. Geoderma, 348, 45–53. https://doi.org/10.1016/j.geoderma.2019.04.011
- Clark, T. E., & McCracken, M. W. (2009). Improving forecast accuracy by combining recursive and rolling forecasts. International Economic Review, 50(2), 363–395. https://doi.org/10.1111/j.1468-2354.2009.00533.x
- Cressie, N. A. C. (1990). The origins of kriging. Mathematical Geology, 22(3), 239–252. https://doi.org/10.1007/BF00889887
- Cui, H., Jiang, L., Paloscia, S., Santi, E., Pettinato, S., Wang, J., Fang, X., & Liao, W. 2022. The potential of ALOS-2 and Sentinel-1 radar data for soil moisture retrieval with high spatial resolution over agroforestry areas, China. IEEE Transactions on Geoscience & Remote Sensing, 60, 4402617.https://doi.org/10.1109/TGRS.2021.3082805
- Dente, L., Vekerdy, Z., de Jeu, R., & Su, Z. (2013). Seasonality and autocorrelation of satellite-derived soil moisture products. International Journal of Remote Sensing, 34(9–10), 3231–3247. https://doi.org/10.1080/01431161.2012.716923
- Diodato, N., de Guenni, L., Garcia, M., & Bellocchi, G. 2019. Decadal oscillation in the predictability of Palmer drought severity index in California. Climate, 7, (1), 6. https://doi.org/10.3390/cli7010006
- Di, C., Wang, T., Istanbulluoglu, E., Jayawardena, A. W., Li, S., & Chen, X. (2019). Deterministic chaotic dynamics in soil moisture across Nebraska. Journal of Hydrology, 578, 124048. https://doi.org/10.1016/j.jhydrol.2019.124048
- Dorigo, W. A., Gruber, A., de Jeu, R. A. M., Wagner, W., Stacke, T., Loew, A., Albergel, C., Brocca, L., Chung, D., Parinussa, R. M., & Kidd, R. (2015). Evaluation of the ESA CCI soil moisture product using ground-based observations. Remote Sensing of Environment, 162, 380–395. https://doi.org/10.1016/j.rse.2014.07.023
- Dorigo, W., Scipal, K., Parinussa, R. M., Liu, Y. Y., Wagner, W., De Jeu, R. A., & Naeimi, V. 2010. Error characterisation of global active and passive microwave soil moisture datasets. Hydrology and Earth System Sciences, 14, 2605–2616. (12) https://doi.org/10.5194/hess-14-2605-2010
- Dorigo, W., van Oevelen, P., Wagner, W., Drusch, M., Mecklenburg, S., Robock, A., & Jackson, T. 2011. A new international network for in situ soil moisture data. Eos Transactions American Geophysical Union, 92, 141–142. (17) https://doi.org/10.1029/2011EO170001
- Dorigo, W., Wagner, W., Albergel, C., Albrecht, F., Balsamo, G., Brocca, L., Chung, D., Ertl, M., Forkel, M., Gruber, A., Haas, E., Hamer, P. D., Hirschi, M., Ikonen, J., de Jeu, R., Kidd, R., Lahoz, W., Liu, Y. Y. … Smolander, T.… 2017. ESA CCI soil moisture for improved Earth system understanding: State-of-the art and future directions. Remote Sensing of Environment, 203, 185–215. https://doi.org/10.1016/j.rse.2017.07.001
- Dorigo, W. A., Wagner, W., Hohensinn, R., Hahn, S., Paulik, C., van Oevelen, M., Robock, S., Drusch, P., Mecklenburg, A., & Jackson, T. 2011. The international soil moisture Network: A data hosting facility for global in situ soil moisture measurements. Hydrology and Earth System Sciences, 15, 1675–1698. (5) https://doi.org/10.5194/hess-15-1675-2011
- Dudal, R. 1980. Soil-related constraints to agricultural development in the tropics. In Priorities for alleviating soil-related constraints to food production in the tropics. International Rice Research Institute, pp. 23–37.
- Entekhabi, D., Reichle, R. H., Koster, R. D., & Crow, W. T. (2010). Performance metrics for soil moisture retrievals and application requirements. Journal of Hydrometeorology, 11(3), 832–840. https://doi.org/10.1175/2010JHM1223.1
- FAO. 1978. Report on agroecological zones. Volume 1: methodology and results for Africa. Food and Agriculture Organization,
- FAO. 2017. Climate Change Predictions in Sub-Saharan Africa: Impacts and adaptations. Food and Agriculture Organization of the United Nations. Retrieved October 13, 2021 https://www.fao.org/3/i7040e/i7040e.pdf
- Fathololoumi, S., Vaezi, A. R., Firozjaei, M. K., & Biswas, A. 2021. Quantifying the effect of surface heterogeneity on soil moisture across regions and surface characteristic. Journal of Hydrology, 126132. https://doi.org/10.1016/j.jhydrol.2021.126132. 596
- Feng, H. 2016. Individual contributions of climate and vegetation change to soil moisture trends across multiple spatial scales. Scientific Reports, 6(1) https://doi.org/10.1038/srep32782
- Feng, H., & Liu, Y. (2015). Combined effects of precipitation and air temperature on soil moisture in different land covers in a humid basin. Journal of Hydrology, 531, 1129–1140. https://doi.org/10.1016/j.jhydrol.2015.11.016
- Fér, M., Kodešová, R., Hroníková, S., & Nikodem, A. (2020). The effect of 12-year ecological farming on the soil hydraulic properties and repellency index. Biologia, 75(6), 799–807. https://doi.org/10.2478/s11756-019-00373-1
- Fick, S. E., & Hijmans, R. J. 2017. WorldClim 2: New 1-km spatial resolution climate surfaces for global land areas. International Journal of Climatology, 37, 4302–4315. (12) https://doi.org/10.1002/joc.5086
- Fildes, R., & Kourentzes, N. (2011). Validation and forecasting accuracy in models of climate change. International Journal of Forecasting, 27(4), 968–995. https://doi.org/10.1016/j.ijforecast.2011.03.008
- Fischer, G., van Velthuizen, H., & Nachtergaele, F. O. 2000. Global agroecological zones assessment: Methodology and results. International Institute for Applied Systems Analysis (IIASA) and Food and Agriculture Organization of the United Nations (UN–FAO). 338 pp. https://pure.iiasa.ac.at/id/eprint/6182/1/IR-00-064.pdf.
- Funk, C., Peterson, P., Landsfeld, M., Pedreros, D., Verdin, J., Shukla, S., Husak, G., Rowland, J., Harrison, L., Hoell, A., & Michaelsen, J. 2015. The climate Hazards Infrared precipitation with Stations—a new environmental record for monitoring extremes. Scientific Data. 2 1 https://doi.org/10.1038/sdata.2015.66
- Fu, X., Yu, Z., Luo, L., Lu, H., Liu, D., Ju, Q., Yang, T., Xu, F., Gu, H., Yang, C., Chen, J., & Wang, T. 2014. Investigating soil moisture sensitivity to precipitation and evapotranspiration errors using SiB2 model and Ensemble Kalman Filter. Stochastic Environmental Research and Risk Assessment, 28, 681–693. 3 https://doi.org/10.1007/s00477-013-0781-3
- Gabriel, J. L., Quemada, M., Martín-Lammerding, D., & Vanclooster, M. 2017. Assessing the cover crop effect on soil hydraulic properties by inverse modelling in a 10-year field trial. Hydrology and Earth System Sciences – Discussions. https://doi.org/10.5194/hess-2017-643.
- García-Moreno, J., Gordillo-Rivero, Á. J., Zavala, L. M., Jordán, A., & Pereira, P. (2013). Mulch application in fruit orchards increases the persistence of soil water repellency during a 15-years period. Soil and Tillage Research, 130, 62–68. https://doi.org/10.1016/j.still.2013.02.004
- Gaur, N., & Mohanty, B. P. (2019). A nomograph to incorporate geophysical heterogeneity in soil moisture downscaling. Water Resources Research, 55(1), 34–54. https://doi.org/10.1029/2018WR023513
- Gibon, F., Pellarin, T., Román-Cascón, C., Alhassane, A., Traoré, S., Kerr, Y., Lo Seen, D., & Baron, C. (2018). Millet yield estimates in the Sahel using satellite derived soil moisture time series. Agricultural and Forest Meteorology, 262, 100–109. https://doi.org/10.1016/j.agrformet.2018.07.001
- Giller, K. E., Delaune, T., Silva, J. V., van Wijk, M., Hammond, J., Descheemaeker, K., van de Ven, G., Schut, A. G. T., Taulya, G., Chikowo, R., & Andersson, J. A. 2021. Small farms and development in sub-Saharan Africa: farming for food, for income or for lack of better options? Food Security, 13, 1431–1454. 6 https://doi.org/10.1007/s12571-021-01209-0
- Global Climate Observing System. 2011. Systematic observation requirements for satellite-based data products for climate: The supplemental details to the satellite-based component of the “Implementation plan for the global observing system for climate in support of the UNFCCC (2010 update, GCOS-154)”. https://www.wmo.int/pages/prog/gcos/documents/SatelliteSupplement2011Update.pdf
- González-Zamora, Á., Sánchez, N., Pablos, M., & Martínez-Fernández, J. (2019). CCI soil moisture assessment with SMOS soil moisture and in situ data under different environmental conditions and spatial scales in Spain. Remote Sensing of Environment, 225, 469–482. https://doi.org/10.1016/j.rse.2018.02.010
- Goovaerts, P. 2019. Kriging interpolation. The Geographic information Science & Technology Body of Knowledge (4th Quarter 2019 edition), John P. Wilson (ed.). https://doi.org/10.22224/gistbok/2019.4.4.
- Green, K. C., Armstrong, J. S., & Soon, W. (2009). Validity of climate change forecasting for public policy decision making. International Journal of Forecasting, 25(4), 826–832. https://doi.org/10.1016/j.ijforecast.2009.05.011
- Green, J. K., Seneviratne, S. I., Berg, A. M., Findell, K. L., Hagemann, S., Lawrence, D. M., & Gentine, P. (2019). Large influence of soil moisture on long-term terrestrial carbon uptake. Nature, 565(7740), 476–479. https://doi.org/10.1038/s41586-018-0848-x
- Gruber, A., Dorigo, W. A., Crow, W., & Wagner, W. (2017). Triple collocation-based merging of satellite soil moisture retrievals. IEEE Transactions on Geoscience and Remote Sensing 55(12), 6780–6792.
- Gruber, A., Scanlon, T., van der Schalie, R., Wagner, W., & Dorigo, W. (2019). Evolution of the ESA CCI soil moisture climate data records and their underlying merging methodology. Earth System Science Data, 11(2), 717–739. https://doi.org/10.5194/essd-11-717-2019
- Hatfield, J. L., Sauer, T. J., & Prueger, J. H. (2001). Managing soils to achieve greater water use efficiency: A review. Agronomy Journal, 93(2), 271–280. https://doi.org/10.2134/agronj2001.932271x
- Herbst, M., Rosier, P. T. W., McNeil, D. D., Harding, R. H., & Gowing, D. J. 2008. Seasonal variability of interception evaporation from the canopy of a mixed deciduous forest. Agricultural and Forest Meteorology, 148, 1655–1667. (11) https://doi.org/10.1016/j.agrformet.2008.05.011
- Huang, J., Desai, A. R., Zhu, J., Hartemink, A. E., Stoy, P. C., Loheide, S. P. I., Bogena, H. R., Zhang, Y., Zhang, Z., & Arriaga, F. (2020). Retrieving heterogeneous surface soil moisture at 100 m across the globe via fusion of remote sensing and land surface parameters. Frontiers in Water, 2, 578367. https://doi.org/10.3389/frwa.2020.578367
- Hurvich, C. M., & Tsai, C. L. (1989). Regression and time series model selection in small samples. Biometrika, 76(2), 297–307. https://doi.org/10.1093/biomet/76.2.297
- Hyndman, R. J., & Athanasopoulos, G. 2018. Forecasting: Principles and practice. 2nd edition. OTexts,
- Hyndman, R., Athanasopoulos, G., Bergmeir, C., Caceres, G., Chhay, L., O’Hara-Wild, M., Petropoulos, F., Razbash, S., Wang, E., & Yasmeen, F. 2021. Forecast: Forecasting Functions for Time Series and Linear models. R Package Version 8.21. Retrieved February 25, 2021 https://pkg.robjhyndman.com/forecast/
- Hyndman, R. J., & Khandakar, Y. 2008. Automatic time series forecasting: The forecast package for R. Journal of Statistical Software, 26, 1–22. (3) https://doi.org/10.18637/jss.v027.i03
- IFPRI. 2015. Agroecological zones for Africa South of the Sahara. HarvestChoice and International Food Policy Research Institute. Harvard Dataverse, V3. https://doi.org/10.7910/DVN/M7XIUB.
- Jing, W., Song, J., & Zhao, X. 2018. A comparison of ECV and SMOS soil moisture products based on OzNet monitoring Network. Remote Sensing, 10(5), 5. https://doi.org/10.3390/rs10050703
- Kac, M. (1947). Random Walk and the theory of Brownian motion. The American Mathematical Monthly, 54(7P1), 369–391. https://doi.org/10.1080/00029890.1947.11990189
- Kidd, R., & Haas, E. (2017). ESA Climate Change Initiative Phase II Soil Moisture. Soil Moisture ECV Product User Guide (PUG). Version 3.3. Earth Observation Data Centre for Water Resources Monitoring (EODC) GmbH and TU. Wien, GeoVille, ETH Zürich: TRANSMISSIVITY.
- Kim, S., Liu, Y. Y., Johnson, F. M., Parinussa, R. M., & Sharma, A. (2015). A global comparison of alternate AMSR2 soil moisture products: Why do they differ? Remote Sensing of Environment, 161, 43–62. https://doi.org/10.1016/j.rse.2015.02.002
- Konings, A. G., Piles, M., Das, N., & Entekhabi, D. (2017). L-band vegetation optical depth and effective scattering albedo estimation from SMAP. Remote Sensing of Environment, 198, 460–470. https://doi.org/10.1016/j.rse.2017.06.037
- Korres, W., Reichenau, T. G., Fiener, P., Koyama, C. N., Bogena, H. R., Cornelissen, T., Baatz, R., Herbst, M., Diekkrüger, B., Vereecken, H., & Schneider, K. (2015). Spatio-temporal soil moisture patterns – a meta-analysis using plot to catchment scale data. Journal of Hydrology, 520, 326–341. https://doi.org/10.1016/j.jhydrol.2014.11.042
- Koster, R. D., Guo, Z., Yang, R., Dirmeyer, P. A., Mitchell, K., & Puma, M. J. (2009). On the nature of soil moisture in land surface models. Journal of Climate, 22(16), 4322–4335. https://doi.org/10.1175/2009JCLI2832.1
- Krivoruchko, K., & Gribov, A. (2019). Evaluation of empirical Bayesian kriging. Spatial Statistics, 32, 100368. https://doi.org/10.1016/j.spasta.2019.100368
- Kwiatkowski, D., Phillips, P. C. B., Schmidt, P., & Shin, Y. (1992). Testing the null hypothesis of stationarity against the alternative of a unit root. Journal of Econometrics, 54(1–3), 159–178. https://doi.org/10.1016/0304-4076(92)90104-Y
- Lawrence, J. E., & Hornberger, G. M. 2007. Soil moisture variability across climate zones. Geophysical Research Letters, 34 (20), L20402. https://doi.org/10.1029/2007GL031382
- Legates, D. R., Mahmood, R., Levia, D. F., DeLiberty, T. L., Quiring, S. M., Houser, C., & Nelson, F. E. 2010. Soil moisture: A central and unifying theme in physical geography. Progress in Physical Geography, 35, 65–86. https://doi.org/10.1177/0309133310386514. (1)
- Liang, W.-L., Li, S.-L., & Hung, F.-X. (2017). Analysis of the contributions of topographic, soil, and vegetation features on the spatial distributions of surface soil moisture in a steep natural forested headwater catchment. Hydrological Processes, 31(22), 3796–3809. https://doi.org/10.1002/hyp.11290
- Li, Y., Campbell, E. P., Haswell, D., Sneeuwjagt, R. J., & Venables, W. N. (2003). Statistical forecasting of soil dryness index in the southwest of Western Australia. Forest Ecology and Management, 183(1–3), 147–157. https://doi.org/10.1016/S0378-1127(03)00103-8
- Lin, C. C., & Segel, L. A. 1974. Mathematics applied to deterministic problems in the natural sciences. Macmillan,
- Liu, Y. Y., Dorigo, W. A., Parinussa, R. M., de Jeu, R. A. M., Wagner, W., McCabe, M. F., Evans, J. P., & van Dijk, A. I. J. M. (2012). Trend-preserving blending of passive and active microwave soil moisture retrievals. Remote Sensing of Environment, 123, 280–297. https://doi.org/10.1016/j.rse.2012.03.014
- Liu, Y. W., Liu, Y. B., & Wang, W. (2019). Inter-comparison of satellite-retrieved and global land data Assimilation System-simulated soil moisture datasets for global drought analysis. Remote Sensing of Environment, 220, 1–18. https://doi.org/10.1016/j.rse.2018.10.026
- Liu, Y., Wang, W., & Liu, Y. 2018. ESA CCI soil moisture assimilation in SWAT for improved hydrological simulation in Upper Huai River Basin. Advances in Meteorology, 2018. 1–13 https://doi.org/10.1155/2018/7301314
- Loew, A., & Schlenz, F. (2011). A dynamic approach for evaluating coarse scale satellite soil moisture products. Hydrology and Earth System Sciences, 15(1), 75–90. https://doi.org/10.5194/hess-15-75-2011
- Ma, C., Li, X., & McCabe, M. F. 2020. Retrieval of high-resolution soil moisture through combination of Sentinel-1 and Sentinel-2 data. Remote Sensing, 12(14): 2303. https://doi.org/10.3390/rs12142303.
- Mayaux, P., Bartholomé, E., Massart, M., Van Cutsem, C., Cabral, A., Nonguierma, A., & Diallo, O. 2003. A land cover map of Africa. EUR 20665 EN, European Commission,
- McNally, A., Shukla, S., Arsenault, K. R. C., Wang, S., Peters-Lidard, C. D., & Verdin, J. P. (2016). Evaluating ESA CCI soil moisture in East Africa. International Journal of Applied Earth Observation and Geoinformation, 48, 96–109. https://doi.org/10.1016/j.jag.2016.01.001
- Meyer, R., Zhang, W., Kragh, S. J., Andreasen, M., Jensen, K. H., Fensholt, R., Stisen, S., & Looms, M. C. 2021. Exploring the combined use of SMAP and Sentinel-1 data for downscaling soil moisture beyond the 1 km scale. Hydrology and Earth System Sciences Discussion. https://doi.org/10.5194/hess-2021-508.
- Mills, T. C. 2019. Applied time series analysis: A practical guide to modelling and forecasting. Elsevier Science,
- Milly, P. C. D., Betancourt, J., Falkenmark, M., Hirsch, R. M., Kundzewicz, Z. W., Lettenmaier, D. P., & Stouffer, R. J. (2008). Stationarity is dead: Whither water management? Science, 319(5863), 573–574. https://doi.org/10.1126/science.1151915
- Mohammed, J. (2020). Challenges in implementing biodiversity policy in sub-Saharan Africa region. American Journal of Biological and Environmental Statistics, 6(2), 24–30. https://doi.org/10.11648/j.ajbes.20200602.12
- Papacharalampous, G. A., Tyralis, H., & Koutsoyiannis, D. (2018). Predictability of monthly temperature and precipitation using automatic time series forecasting methods. Acta Geophysica, 66(4), 807–831. https://doi.org/10.1007/s11600-018-0120-7
- Petropoulos, G. P., Ireland, G., & Barrett, B. 2015. Surface soil moisture retrievals from remote sensing: current status, products & future trends. Physics and Chemistry of the Earth, 83–84, 36–56. https://doi.org/10.1016/j.pce.2015.02.009
- Pierdicca, N., Fascetti, F., Pulvirenti, L., Crapolicchio, R., & Muñoz-Sabater, J. (2015). Analysis of ASCAT, SMOS, in-situ and land model soil moisture as a regionalized variable over Europe and North Africa. Remote Sensing of Environment, 170, 280–289. https://doi.org/10.1016/j.rse.2015.09.005
- Qiu, Y., Fu, B., Wang, J., & Chen, L. (2001). Soil moisture variation in relation to topography and land use in a hillslope catchment of the Loess Plateau, China. Journal of Hydrology, 240(3–4), 243–263. https://doi.org/10.1016/S0022-1694(00)00362-0
- Qiu, J., Gao, Q., Wang, S., & Su, Z. (2016). Comparison of temporal trends from multiple soil moisture data sets and precipitation: the implication of irrigation on regional soil moisture trend. International Journal of Applied Earth Observation and Geoinformation, 48, 17–27. https://doi.org/10.1016/j.jag.2015.11.012
- Rao, P., Wang, Y., Wang, F., Liu, Y., Wang, X., & Wang, Z. 2022. Daily soil moisture mapping at 1 km resolution based on SMAP data for desertification areas in northern China. Earth System Science Data, 14, 3053–3073. https://doi.org/10.5194/essd-14-3053-2022. 7
- R Core Team. 2021. R: A language and environment for statistical computing. R Foundation for Statistical Computing, https://www.R-project.org/.
- Robinson, D. A., Campbell, C. S., Hopmans, J. W., Hornbuckle, B. K., Jones, S. B., Knight, R., Ogden, F., Selker, J., & Wendroth, O. (2008). Soil moisture measurement for ecological and hydrological watershed-scale observatories: A review. Vadose Zone Journal, 7(1), 358–389. https://doi.org/10.2136/vzj2007.0143
- Robock, A., Vinnikov, K. Y., Srinivasan, G., Entin, J. K., Hollinger, S. E., Speranskaya, N. A., Liu, S., & Namkhai, A. (2000). The global soil moisture data bank. Bulletin of the American Meteorological Society, 81(6), 1281–1299. https://doi.org/10.1175/1520-0477(2000)081<1281:TGSMDB>2.3.CO;2
- Sabaghy, S., Walker, J. P., Renzullo, L. J., & Jackson, T. J. (2018). Spatially enhanced passive microwave derived soil moisture: Capabilities and opportunities. Remote Sensing of Environment, 209, 551–580. https://doi.org/10.1016/j.rse.2018.02.065
- Sadeghi, M., Babaeian, E., Tuller, M., & Jones, S. B. (2017). The optical trapezoid model: A novel approach to remote sensing of soil moisture applied to Sentinel-2 and Landsat-8 observations. Remote Sensing of Environment, 198, 52–68. https://doi.org/10.1016/j.rse.2017.05.041
- Scheren, P., Tyrrell, P., Brehony, P., Allan, J. R., Thorn, J. P. R., Chinho, T., Katerere, Y., Ushie, V., & Worden, J. S. 2021. Defining pathways towards African ecological futures. Sustainability, 13, 8894. https://doi.org/10.3390/su13168894. 16
- Sehler, R., Li, J., Reager, J. T., & Ye, H. (2019). Investigating relationship between soil moisture and precipitation globally using remote sensing observations. Journal of Contemporary Water Research & Education, 168(1), 106–118. https://doi.org/10.1111/j.1936-704X.2019.03324.x
- Seneviratne, S. I., Corti, T., Davin, E. L., Hirschi, M., Jaeger, E. B., Lehner, I., Orlowsky, B., & Teuling, A. J. (2010). Investigating soil moisture–climate interactions in a changing climate: A review. Earth-Science Reviews, 99(3–4), 125–161. https://doi.org/10.1016/j.earscirev.2010.02.004
- Shumway, R. H., & Stoffer, D. S. 2016. Time series analysis and its applications: With R examples. 4th edition. Springer, https://doi.org/10.1007/978-3-319-52452-8
- Snapp, S. 2017. Agroecology: Principles and practice (chapter 2). In Agricultural systems: Agroecology and rural innovation for development, 2nd edition (Eds. S. Snapp & B.). Pound Elsevier Inc. https://doi.org/10.1016/B978-0-12-802070-8.00002-5. pp. 33–72.
- Song, P., Huang, J., & Mansaray, L. R. (2019). An improved surface soil moisture downscaling approach over cloudy areas based on geographically weighted regression. Agricultural and Forest Meteorology, 275, 146–158. https://doi.org/10.1016/j.agrformet.2019.05.022
- Su, C.-H., Ryu, D., Dorigo, W., Zwieback, S., Gruber, A., Albergel, C., Reichle, R. H., & Wagner, W. 2016. Homogeneity of a global multisatellite soil moisture climate data record. Geophysical Research Letter, 43, 11245–11252. (21) https://doi.org/10.1002/2016GL070458
- Susha Lekshmi, S. U., Singh, D. N., & Baghini, M. S. 2014. A critical review of soil moisture measurement. Measurement, 54, 92–105. https://doi.org/10.1016/j.measurement.2014.04.007
- UNEP-WCMC. 2016. The state of biodiversity in Africa: A mid-term review of progress towards the Aichi biodiversity Targets,
- Vereecken, H., Huisman, J. A., Bogena, H., Vanderborght, J., Vrugt, J. A., & Hopmans, J. W. (2008). On the value of soil moisture measurements in vadose zone hydrology: A review. Water Resources Research, 44(4), 253–270. https://doi.org/10.1029/2008WR006829
- Vergopolan, N., Chaney, N. W., Pan, M., Pan, M., Sheffield, J., Beck, H. E., Ferguson, C. R., Torres-Rojas, L., Sadri, S., & Wood, E. F. 2021. SMAP-HydroBlocks, a 30-m satellite-based soil moisture dataset for the conterminous US. Scientific Data, 8, 264. https://doi.org/10.1038/s41597-021-01050-2. (1)
- von Randow, R. C. S., von Randow, C., Hutjes, R. W. A., Tomasella, J., & Kruijt, B. (2012). Evapotranspiration of deforested areas in central and southwestern Amazonia. Theoretical and Applied Climatology, 109(1–2), 205–220. https://doi.org/10.1007/s00704-011-0570-1
- Wang, Y., Yang, J., Chen, Y., Wang, A., & De Maeyer, P. 2018. The spatiotemporal response of soil moisture to precipitation and temperature changes in an arid region, China. Remote Sensing, 10(3), 468. https://doi.org/10.3390/rs10030468
- Weiss, G. H. 1983. Random Walks and their applications: Widely used as mathematical models, Random Walks play an important role in several areas of physics, chemistry, and biology. American Scientist, 71(1), 65–71. https://www.jstor.org/stable/27851819
- Yang, F., & Liang, D. 2020. Random Walk simulation of non-conservative pollutant transport in shallow water flows. Environmental Modelling and Software, 134, 104870. https://doi.org/10.1016/j.envsoft.2020.104870
- Yan, S., & Wu, G. (2010). Application of Random Walk model to fit temperature in 46 gamma world cities from 1901 to 1998. Natural Science, 2(12), 1425–1431. https://doi.org/10.4236/ns.2010.212174
- Yu, B., Liu, G., Liu, Q., Wang, X., Feng, J., & Huang, C. 2018. Soil moisture variations at different topographic domains and land use types in the semi-arid Loess Plateau, China. Catena, 165, 125–132. https://doi.org/10.1016/j.catena.2018.01.020
- Zeng, J., Li, Z., Chen, Q., Bi, H., Qiu, J., & Zou, P. (2015). Evaluation of remotely sensed and reanalysis soil moisture products over the Tibetan Plateau using in-situ observations. Remote Sensing of Environment, 163, 91–110. https://doi.org/10.1016/j.rse.2015.03.008
- Zeyliger, A. M., Muzalevskiy, K. V., Zinchenko, E. V., & Ermolaeva, O. S. (2022). Field test of the surface soil moisture mapping using Sentinel-1 radar data. Science of the Total Environment, 807, 151121. https://doi.org/10.1016/j.scitotenv.2021.151121
- Zhang, K., Wang, Q., Chao, L., Ye, J., Li, Z., Yu, Z., Yang, T., & Ju, Q. (2019). Ground observation-based analysis of soil moisture spatiotemporal variability across a humid to semi-humid transitional zone in China. Journal of Hydrology, 574, 903–914. https://doi.org/10.1016/j.jhydrol.2019.04.087
- Zhao, W., Sánchez, N., Lu, H., & Li, A. (2018). A spatial downscaling approach for the SMAP passive surface soil moisture product using random forest regression. Journal of Hydrology, 563, 1009–1024. https://doi.org/10.1016/j.jhydrol.2018.06.081
- Zhao, W., Wen, F., Wang, Q., Sanchez, N., & Piles, M. 2021. Seamless downscaling of the ESA CCI soil moisture data at the daily scale with MODIS land products. Journal of Hydrology, 126930. https://doi.org/10.1016/j.jhydrol.2021.126930. 603
- Zohaib, M., Kim, H., & Choi, M. (2017). Evaluating the patterns of spatiotemporal trends of root zone soil moisture in major climate regions in East Asia. Journal of Geophysical Research: Atmospheres, 122(15), 7705–7722. https://doi.org/10.1002/2016JD026379
- Zwieback, S., Colliander, A., Cosh, M. H., Martínez-Fernández, J., McNairn, H., Starks, P. J., Thibeault, M., & Berg, A. (2018). Estimating time-dependent vegetation biases in the SMAP soil moisture product. Hydrology and Earth System Sciences, 22(8), 4473–4489. https://doi.org/10.5194/hess-22-4473-2018