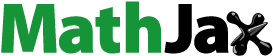
ABSTRACT
The oil spill is one of the most impactful sources of marine pollution on the ocean surface, detected by the SAR sensors as dark areas, regions with low backscatter values. Due to the complex mixture of hydrophobic hydrocarbons, mineral oil spills change the water surface tension dampening the capillary gravity waves and provoking a specular reflection. In this work, we associated the geochemical oil characteristics, such as density, viscosity, API, and molecular composition with the backscatter values for each oil spill case. We identified the relationship between the oil weathering processes, with the changes in the backscattering values of ocean oil spills. The method designed zonal sections over the oil spills detected in the SAR images, to extract the backscatter values for each pixel along the section. The lowest backscatter average was observed by the heavy oil spill in the Corsica Island study (−29,99 dB). The highest level of weathering had the highest backscatter averages. Damping rates ranged between 4,12 and 7,07 dB and the backscatter values may be related to low oil layer thickness. Furthermore, low wind speeds may have reduced the contrast between water and oil spills, resulting in low damping ratios in all events.
Introduction
Offshore oil spills represent a great source of pollution in the marine environment (Beyer et al., Citation2016; Nissanka & Yapa, Citation2018). The damage to this ecosystem is extensive and resistant, remaining for years in nature, with serious socioeconomic and environmental consequences (Li et al., Citation2016; Yekeen et al., Citation2020). Oil spill surveillance is an important part of the contingency plan (Jha et al., Citation2008; Mohammadiun et al., Citation2021) since remote sensing techniques allow mapping these events in peripheral areas with essential information to detect spills (Keramea et al., Citation2021; Shinga et al., Citation2013). Therefore, Synthetic Aperture Radar (SAR) data has been widely used for mapping ocean oil spills, since the detection process in the microwave spectral is independent of weather conditions and solar illumination (Chaturvedi et al., Citation2020; Huang et al., Citation2022).
Oil is a complex mixture of different organic compounds, mostly hydrocarbons and other components, such as sulphur, nitrogen, and oxygen (Fingas, Citation2011). The oil compositional percentages vary according to the geological processes of its formation, which gives them different characteristics and properties (Vafaie & Kivi, Citation2020). As mineral oil has hydrophobic constituents, it can persist on the ocean surface in the form of multiple layers (Alpers et al., Citation2017). When spilled into the sea, the mineral oil receives the weathering processes, such as evaporation, emulsification, biodegradation, dispersion, dissolution, and oxidation (Chaturvedi et al., Citation2020; Fingas, Citation2011). Which promote a series process by which the physical and chemical properties of oil change after spillage (Marta-Almeida et al., Citation2013). The presence of higher viscosity fluids in seawater alters the surface tension of the ocean and induces the attenuation of capillary waves and reduces backscattering (Ajadi et al., Citation2018; Alpers & Hühnerfuss, Citation1989; Alpers et al., Citation2017). Therefore, oil spills on the sea increase the water surface tension, reducing the sea surface roughness. The Bragg scattering suppression allows to recognize oil spills as low backscatter regions by the SAR sensors and presents high contrast to the clean, unaffected sea surface (Benelli & Garzelli, Citation1999; Espeseth et al., Citation2017; Naz et al., Citation2021; Skruners et al., Citation2014). The suppression of capillary waves presents on the ocean surface (same order of magnitude as the incident radar pulse) is induced by the presence of oil, which is less dense than seawater (Ermakov et al., Citation2018). This process reduces radar backscatter by making oil spill appear as dark regions in the SAR image (Zhang et al., Citation2017), while the ocean surface around the spill remains brighter, represented by higher Sigma 0 values (Naz et al., Citation2021; Xu et al., Citation2020).
According to Migliaccio et al. (Citation2011), oil spill observations in SAR images are physically possible under moderate wind conditions (∼3–12 m.s−1), since the slick dampens short gravity and capillary waves, surface roughness (Shen et al., Citation2019). The reducing backscatter is also provoked by other physical phenomena, so-called false positives, or look-alikes (Huang et al., Citation2022). As an example, we can cite biogenic blooms changes the radar Sigma-0 value by dampening small capillary waves in the oceanic microlayer, down to the upper 1000 micrometers of the water column (Jayasinghe et al., Citation2022). The same occurs in storm areas (associated or not with cyclogenesis), areas with low wind (velocity less than 3 m.s-1), convective cells, internal waves, upwelling zones, and ocean fronts are some of the factors that promote dark spots in SAR images (Alpers et al., Citation2017; Migliaccio et al., Citation2011).
Currently, the main studies of oceanic oil detection by SAR imaging focused on analyzing the backscatter signal or polarization, in a purely analytical way (Lentini et al., Citation2022). Manuscripts such as Zhang & Li (Citation2011) are references that spatially and statistically correlate the geochemical signatures of oil as density, viscosity, API degree and molecular composition; with radar signal backscatter values. The API degree is a parameter used to classify the type of oil or derivative and is related to the specific gravity of the oil (Chung et al., Citation2023). This parameter was established by the American Petroleum Institute and is directly related to the specific gravity (density) of the oil, used as an indicator of oil quality (Chedi et al., Citation2021; Guzmán-Osorio et al., Citation2020). Density is valuable information to represent the contribution of light and/or heavy compounds to petroleum fractions. In this parameter, oils composed of low molecular weight hydrocarbons have an API degree above 30, between 22 and 30 are classified as medium and below 22 are classified as heavy, with a predominance of heavy compounds, such as resins and asphaltenes (Pabón & de Souza Filho, Citation2019). The behavior of oil when spilled depends on its chemical composition and on the meteorological and oceanographic conditions, which impacts on weathering. When oil spills at sea, the oil slick tends to spread over the water surface forming thin layers of oil in lighter products (gasoline, diesel, and light oils), or thicker layers in heavy oils and Bunker C (Fingas, Citation2011).
In this way, this manuscript selected seven case studies to analyze the radar backscatter values of different oil slicks, detected in distinct geographic regions, with different properties, associating them with their respective geochemical signature. The objective was to identify patterns in the physicochemical characteristics of different oil spills in response to backscatter, considering that the geochemical signatures of the oil and the degree of weathering must imply different responses to backscatter of the radar signal.
Material and methods
Data set
For this study, cases of oil spills detected by SAR sensors in oceanic regions were identified and selected, comprising different geochemical characteristics and meteorological and oceanographic conditions. lists per geographic region the geographical coordinates, the acquisition data, and the SAR sensor of each analyzed image. We selected these spill cases based on the availability of information in our database, supported by scientific articles that document the types of oil spilled in each situation.
Table 1. Images data with the oil spill location areas, acquisition date and satellite used in the study. Note: the data for Mexican Gulf case (2010) were obtained from the Envisat satellite. For the other cases, the data were from Sentinel-1.
The meteorological and oceanographic conditions are important to understand oil weathering, since the local wind, temperature and salinity change geochemical transformation and biodegradation.
In this study, we used SAR images operating in the C-band (Frequency between 8–4 GHz and wavelength between 3.8–7.5 cm), acquired by the satellites Sentinel-1 and Envisat to analyze the backscattering of the radar signal. The Envisat satellite was in operation between 2002 and 2012, providing data for global monitoring over oceanic regions, continents, and polar regions. The active sensor named ASAR (Advanced Synthetic Aperture Radar) on board the Envisat platform operated in the C-band. The Sentinel-1 mission, in operation since 2014, is composed of two satellites (Sentinel-1A and B) operating in the C Band, with different polarizations (HH+HV, VV+VH).
The SAR data downloaded from the Copernicus Open Access Hub portal (https://scihub.copernicus.eu/) was acquired in Single Look Complex (SLC) format, using the Interferometric Wide swath (IW) mode, with spatial resolution of 10 × 10 m, and image swath of 251.8 km.
Data were downloaded with the processing level 1, georeferenced, with satellite orbit and altitude provided in zero-Doppler tilted-range geometry. The IW acquisition system is the most used beam mode for monitoring oceanic regions, and the VV is considered the best polarization for oil identification on the sea surface, offering a better signal-to-noise ratio. The SAR data in the SLC format was pre-processed according to the following steps: application of orbit, radiometric correction, geometric correction, deburst, Multilooking, and conversion of data from linear values to decibels. The orbit application consists of updating the orbit state vectors for each scene through the metadata, providing accurate information about the satellite’s position and speed. Radiometric calibration provides correction values so that pixels can be related to the radar backscatter of the scene and was performed for each SAR data. The calculations were carried out after this stage. For all images, the backscattering was converted in decibels (dB) using a logarithmic transformation (Conceição et al., Citation2021).
Oil spill geochemical data
Mineral oil films are a chemical mixture, including alkanes (“paraffins”), cycloalkanes, and aromatic compounds (Alpers et al., Citation2017). The thickness of a mineral oil spill is greater than a monomolecular marine spill, which results in different viscoelastic properties and, therefore, different roughness attenuation on the sea surface waves (Sergievskaya et al., Citation2019). Thus, owing to the lack of detailed information on the geochemical characteristics of the oils analyzed in the SAR images, we use information from the database developed by the Medsilk-II program (available: http://www.medslik-ii.org). This database features API grade, viscosity, and solubility temperature for different oils from formation basins around the world. In , we present the technical information with the types of oil involved in the analyzed spill cases, in addition to the oil extraction fields and geochemical parameters (density, API degree, temperature and viscosity).
Table 2. Oil geochemistry data associated with the studied spills. Bunker-C type oils are derived from industrial processes, and it is not possible to classify the oil field referring to this type of oil.
The oil spilled near Corsica Island occurred after the collision of two ships (Ulysse – Virginia) and was classified as bunker-C fuel oil. According to the simulation performed by Liubartsevaa et al. (Citation2020), about 14% of the oil evaporated quickly, much of the oil formed stable water-in-oil emulsions and there was an increase in natural dispersion gradually over time, surpassing 70% on 16 October 2018. The Persian Gulf spills occurred in March 2017, near the Aboozar and Sirri oil fields in Iran, close to the United Arab Emirates frontier. The API degree of these oils is 26.96 and 30.90, respectively. In the Deepwater Horizon case in 2010, Light Louisiana Sweet crude has similar geochemical characteristics to oil spilled from the Macondo well (Wang et al., Citation2020).
An oil spill in the ocean involves a complex interaction between the oil, marine and atmospheric elements and natural degradation processes. Oil weathering rates are influenced by factors such as the type of oil and environmental conditions. After a spill, the oil spreads across the ocean surface, and the speed of this process is determined by the thickness and volume of the oil (Chaturvedi et al., Citation2020). Evaporation is essential in oil weathering, reducing the lighter aromatic hydrocarbons (Yim et al., Citation2011). In a few days, light crude oil can lose 75% of its initial mass, and medium crude oil can lose 40% (Wang et al., Citation2021). Emulsification is also fundamental, exacerbating contamination in marine ecosystems through the mixing of water and oil, as this process results in a significant increase in the oil’s water content (80% to 90%) and consequently exacerbates pollution in the environment (Ülker et al., Citation2022). The dispersion of the oil slick is fundamental in the early stages of the transformation, with the reaggregation of the droplets influenced by the hydraulic properties and characteristics of the oil, such as viscosity and waves (Wang et al., Citation2021).
Oil dissolution is the process by which oil enters the aqueous phase. Due to limited solubility, the less predictable components of oil vanish faster than they degrade (Wang et al., Citation2020). Photooxidation is a potentially significant process in the degradation of spilled crude oil in the sea, primarily affecting the aromatic compounds in the oil (Ward et al., Citation2018). Photooxidation products tend to be highly water-soluble and bioavailable, potentially enhancing biodegradation. Furthermore, specific components can absorb light, leading to the generation of reactive oxygen species that facilitate additional oxidation.
SAR data processing
The Normalized Radar Cross Section (NRCS) is a measure of a target’s reflectivity, which shows the interaction of electromagnetic energy with the target, and how it spreads the signal back to the radar instrument (Latini et al., Citation2016). The gray level in SAR images is related to the normalized cross-section of the radar (NRCS or σ0), which shows the strength of the backscattered radar signal (Alpers et al., Citation2017). The σ0 value is usually expressed in decibel (dB) and changes significantly with the incidence angle, radar wavelength and polarization (Nunziata et al., Citation2018).
At intermediate angles (between 20° and 60°): the Bragg scattering is predominant (Latini et al., Citation2016). According to the Bragg scattering theory, the NRCS is proportional to the spectral energy density of the Bragg waves, where kb is the surface wave which follows the Bragg resonance condition, k0 is the radar wave number, and θ is the incidence angle (Gade et al., Citation1998):
As described by Alpers et al. (Citation2017), the reduction of the radar backscatter on the sea surface due to the presence of oil spills shows significant variation according to the oil type, time after the spill, vertical oil spill distribution, wind speed, and direction. These factors modify the sea surface tension, changing the oceanic mixing layer and causing the specular reflection of the radar signal.
To obtain the backscatter values of the radar signal, zonal sections were made over the Sigma 0 data values in areas with samples of mineral films on the sea surface. This step aims to verify the backscatter values variation within the oil films. The steps comprise: (I) Selection of SAR images with oil spills; (II) Pre-processing of SAR images; (III) Creation of transverse sections using a shapefile file format; (IV) Creation of a mask to distinguish the central portions and the edges; (V) we test possible improvements in the acquisition of the backscatter signals with the application of the filters: Lee, Frost, Median and IDAN filters to remove speckle noise. In this study, four filters were tested, in which the loss of backscatter values and the smoothing of the radar signal along the patch were observed. Therefore, as the result of applying the filters reduced the difference in values between the edge and the center, they were not used in the analyses.
The backscattering values extracted for each oil slick per transversal section, designed at the center and near the oil slick edges, were recorded and then associated with the weathering process. shows the SAR images selected with the zonal sections designed. The edges and center parts were separated in red and pink, respectively. The section in yellow corresponds to the backscatter profile in each image, showing the backscatter behavior along the oil slick. The lines were positioned according to the distribution and shape of the oil slick, separating the edge and center parts.
Damping ratio cross section
The NRCS ratios between water and oil provide information on the dielectric properties of the surface layer and, for thick spots, indicate the damping rate through the information contained in the ocean spectral density per Bragg wavelength (Minchew et al., Citation2012). In the present study, the damping rate will calculate from the ratio between the pixel value for the clean sea surface and for the oiled sea surface, at the same angle of incidence. According to Gade et al. (Citation1998), the damping ratio for microwave backscattering at a given JK polarization(HH or VV) is given by:
This water/oil ratio is a function of the Bragg coefficients denoted by (F (R)) and the ratio of the wave spectra to the Bragg wave number in clean water for the analyzed spot. Wwater(Kb) e Woil(Kb) is the spectral density of the 2-D wavenumber of ocean surface roughness on a clean surface and on a slick, respectively. The extraction of the damping rate values will be conducted in the central part and in the edges of the stain, looking for differences in the backscatter (water/oil) ratios, because of the weathering processes of the spilled oil. Sampling points were collected in the SAR images, over the area covered by oil (in the edge and central portions) and in the free portion of the spill, all with the same angle of incidence, in which the backscattering values will be extracted.
We tried to find results that agree with Gade et al. (Citation1998) and verify that the damping rates decrease with increasing wind speed and increasing turbulence in the ocean mix layer. Therefore, they must be considered in the analysis.
Wind data
Wind is a key factor in dampening radar signal backscattering. Wind changes the marine surface roughness and affects the specular backscattering inherent in SAR. Wind data, for each region, was extracted using the CMOD-5 algorithm (C-band scatterer as a function of the ocean geophysical model). This CMOD-5 wind recovery model was originally developed for radar C-band and VV polarization (Shuming et al., Citation2010).
The algorithm provides an empirical relationship between the C-band backscatter and wind vector in the neutral ocean region, equivalent to 10 meters high (neutral surface wind), with dependency of the incidence angle. This data was detected by the ERS-2 and ASCAT spatial origin scatterers (Hersbach, Citation2010). The wind speed and direction data are shown in . To extract wind speed data we use the Ground Range Detected (GRD) images with a resolution corresponding to the altitude mean range value at average height orbit, while in IW mode the pixel size (Range x Azimuth) corresponds to 10 × 10 m and 5 × 1 in numbers of looks. In general, the wind speed values ranged between 0,9 and 5,3 m/s. Therefore, with this interval, the result differences suffered a low variation by the wind action.
Table 3. Wind speed values for each spill case analyzed.
Results and discussion
Backscattering behavior within the oil slicks
The results presented in comprise the backscatter values in dB extracted from the Sentinel-1 and Envisat-ASAR satellites using the zonal sections as a reference, with the incidence angles, and the API grade of each spilled oil. We seek to find relationship patterns between the backscatter signals and the oil geochemical signature, as previously proposed by Sato et al. (Citation1978) and Wismann et al. (Citation1998).
Table 4. Mean, maximum, minimum, and standard deviation values of backscattering extracted from zonal sections applied to the patches.
In general, the results show that denser oils provoke a lower backscattering of the radar signal, then heavy oils promote a greater reduction in radar backscatter. Since oil slicks modify the ocean surface tension, these differences can be related to physicochemical aspects, due to different geochemical signatures of the analyzed oils. These results agree with those found by Fingas (Citation2018), who state that heavy fuel oils presented lower backscattering coefficients than light oils.
Among the analyzed images, the lowest backscattering average was found for the Corsica Island spill (−29.99) on October 08, with heavy oil. Then for the spills on the boundary of Iran and the United Arab Emirates, Sirri field (−29.34 dB) and in the Mexican Gulf (−28.75 dB). These backscatter values may be associated with low wind speed, thus promoting an intensity of specular backscattering effect (). On the other hand, for the spills in Corsica Island and Deepwater Horizon (Mexican Gulf), the radar backscatter values were higher, −22.23 dB and −18.63 dB, respectively. Analyzing the results, the oil detected in Corsica Island on the 8 October with −29.99 dB underwent weathering processes, which were perceptible through the increase of the backscattering values (−22.23 dB) for the same oil spill in Corsica Island on the 9 October. In the Deepwater Horizon study case, in addition to the low oil density, the oil plume underwent water emulsification until it reaches the surface, emerging from a 1.7 km of depth. Its backscattering value was the highest (−18.63 dB), coherent with the oil emulsification process that drags seawater within the slick (Migliaccio et al., Citation2011).
The changing of backscatter signal strength in a mineral film depends on the geochemical properties of the oil, as well as the wind conditions. The low-viscosity oils spread faster than high-viscosity oils. This process is rarely uniform, and its solubility is influenced by the stress of wind, waves, currents, and tidal currents. The relationship is that the interaction of stronger forces generates a faster process of spreading low-viscosity oils (Fingas, Citation2011). In spills from the Aboozar (Iran) and Al Khajfi (Kuwait) fields, we observed different backscattered signal intensities on oil slicks, despite the oils having similar densities and API grade of 26.96 and 26.4, respectively (). In these images, the backscatter values found were −26.20 dB for Iran and −24.05 dB for Kuwait, however, the oil viscosity values are different (36.6 for Iran and 9.8 for Kuwait, ). Therefore, this backscattered power difference can be related to the oil spilled viscosity into the ocean, so the higher viscosity generates a greater specular backscatter.
Figure 2. Backscatter values extracted from each SAR image and their respective densities in g/cm3. The figure shows the backscatter with the density of the oils in the cases.

Figure 3. Backscatter values were extracted from each SAR image along with their respective viscosity values. The figure illustrates the relationship between backscatter and the viscosity of each oil. Notably, Bunker-C oil exhibits a significantly higher viscosity compared to the others.

In general, an oil film spilled on the marine surface spreads quickly. The edges of the oil slick are usually thinner than the center of the slick at this stage, resembling a “fried egg”. After about a day of propagation, this effect diminishes (Gong et al., Citation2014). This small variation in oil hydrocarbon concentration promotes a difference in the thickness of the mineral film. Therefore, the variation of the mineral film layer in the central and edge areas of an oil spill can promote the difference in the roughness of the sea surface across the entire oil-affected region. This results in variation in the intensity of the specular backscatter.
Thus, the lower backscattering coefficients are concentrated in the center of the oil slick, relative to the edges of the oil slicks, as seen in . This variation in backscatter intensity can be associated with the oil concentration inside the oil slick and weathering. Mineral oil films reduce sea surface tension and wind friction, so they dampen capillary and short-gravity sea waves, which control radar backscatter (Meng et al., Citation2021). This is due to the difference in the viscoelastic properties and density of water and oil. Weathering processes alter the properties of the oil spill, especially the viscosity and density of the spilled oil (Naz et al., Citation2021). Consequently, this implies a change in the smoothing effect of the roughness of the sea surface, which in turn affects the damping of radar backscatter. Weathering tends to act, more intensely, in the beginning, through volatile and lower molecular weight elements, increasing the concentration of the oil’s heavier fractions. Evaporation promotes the reduction of lighter aromatic hydrocarbons, whose molecules consist of less than 15 carbon atoms (Yim et al., Citation2011). The modifications in the composition of the oil slicks alter their interaction with seawater and, consequently, affect the signal strength of the backscattered radar.
Damping ratio values
The damping ratio provides the backscatter values information for free water and water with oil stains, for the same incidence angle (Eq. 2). This parameter measures the ratio of the wave’s spectral energy on the ocean surface, on clean and oil-covered, as a function of the Bragg wave vector (Rix et al., Citation2000).
These ratio values were calculated for all analyzed test cases, according to , with damping rates that ranged between 4,12 and 7,07 dB. These variations in the damping ratio are attributed to changes in oil concentration, thickness, dielectric properties, and spectral density variation of ocean waves not captured by the average for any water/oil ratio (Minchew et al., Citation2012; Quigley et al., Citation2020). In this study, the damping rate was affected by oil weathering. The measurement of the energy fraction of spectral waves on sea surfaces is influenced by the dielectric properties of oil slicks, even for thick oil spills or those with a thickness much smaller than the depth of penetration of the radar. Furthermore, the dielectric properties of an oil spill can change over time as the slick is exposed to various weathering processes such as evaporation and emulsification with water (Skruners et al., Citation2015).
Figure 5. Damping ratio values extracted from each SAR image, their respective incidence angles, and the damping values found. The figure shows the damping ratio with incidence angle.

The incidence angle for extracted points (areas with and without oil stains) changed between 31° and 43°. We observe that there was no increase in the damping ratio as the incidence angle increased (). According to Wismann et al. (Citation1998) the angle of incidence does not affect the damping ratio, which was also observed by Johnson and Croswell (Citation1982) to identify no significant changes in the Ku-band damping ratio for incidence angles up to 40 degrees. These findings may support the idea that the geochemical characteristics of the oil are affecting the signal damping. We believe that this small variation in damping rate values in these studied cases may be associated with the angle of incidence of the oil slick, since the parameters that influence roughness, in this case, wind speed, are similar for oil spills analyzed.
The behavior of these measurements agrees with Wismann et al. (Citation1998) studies, which states that the damping ratio increases with the Bragg wave number, oil viscosity and oil layer thickness. This behavior of damping ratios was also found by Sergievskaya et al. (Citation2019) and this relationship shows the damping ratio with the gradual increase in incidence angle. However, incidence angles close to the geochemical signature of light oils have a higher Damping ratio than heavy oils.
The contrast in sea-oil backscatter intensity was low for all spills. The maximum wind speeds in the spills ranged from 2,2 to 5,3 m/s. As a result, we did not have enough contrast in the surface roughness between the free and stained areas, thus decreasing the contrast of backscatter values. According to Zhang et al. (Citation2018), the increase in wind speed makes the ocean surface NRCS larger. In such a way that the signal-to-noise ratio becomes greater and the damping ratios measurable.
In general, it was observed that light oils have a lower damping rate than heavy oils (). This behavior is expected for light and heavy oils, but for the heavy oil from Corsica Island (09-10-18), the influence of weathering was significant so that the value of the damping rate was similar to that of light oils.
Figure 6. Relation of the API degree and damping ratio values. Triangles are API grade 12 spills (heavy oils) and circles (light oils).

The damping ratio values are influenced by the oil dielectric constant, which in turn is affected by the thickness of the stain on the surface, oil composition intensity of weathering processes, and the rate of emulsification in the oil. The C-band radar signal, despite providing mainly information about the surface, penetrates a few millimeters into the stain and provides information on the thickness. Lower values of the damping ratio may be related to compositional variation due to weathering.
Corsica Island – study case
The effect of the weathering on the backscatter values detected by the SAR sensors is evident for the oil spill near the islands of Corsica (). In the 24-h interval during the oil spill image acquisition over the Corsica Islands, the mean backscatter values showed an increase of 7.76 dB (from −29.99 dB to −22.23 dB), a relative discrepancy of 23%. As the type of oil spilled near the Corsica Islands (Bunker-C) has lower levels of volatile elements (about 14%), than other oils analyzed, the oil evaporated quickly (Liubartsevaa et al., Citation2020). Furthermore, heavy oil or residue can lose up to 10% in mass in the first few days after a spill (American Society of Civil Engineers, 1996). In this case, the weathering process changed the oil spill composition. Thus, the backscatter tends to increase, that is, to be less negative, as seen in the data below (−22.23 dB), leading to an increase in backscattered energy. This large difference in backscatter values over this period is due to the difference in the angle of incidence between the images. On the other hand, the damping rate between the oils was different. Knowing that the angle of incidence does not change the damping rate, according to the conclusions of Wismann et al. (Citation1998), the action of weathering modified the characteristics of the slicks, consequently contributing to the reduction (rather than increase) of specular backscattering in the SAR image (October 9) compared to the previous day (October 8) in 2018.
Figure 7. Boxplot diagram of core and edge backscattering values (dB) of Corsica Island oil spill case for 8 and 9 of October 2018.

When comparing the oil backscatter values close to the Corsica Islands, the relative discrepancy was greater near to the edge in relation to the spill core (). We expect that the outer regions are more subject to the action of weathering. The evaporation, emulsification, and dispersion caused by wind-driven sea surface generated higher rates at the edges than at the spill core. This is due to the variation in thickness and composition of the oil spill. Therefore, this change generates a geochemical signature that occurs from the edge to the center, changing the intensity of the backscattering. This process presents a small variation of relative discrepancy (only 2%), between the external and internal values of the stain.
Conclusions
Radar remote sensing proves to be an important tool. It allows an understanding of the degree of influence of the geochemical signature of the oils on the backscatter values detected by SAR sensors. The limited technical information regarding the spills studied in this manuscript makes more in-depth conclusions difficult. Our goal was to extend the idea of controlled studies such as Chedi et al. (Citation2021). Geochemical analysis of the oil can reveal how the increase in mean NRCS values shows a positive correlation is crucial to understanding the behavior of oil in the marine environment. Our results showed that meteorological oceanographic conditions affect the radar backscatter intensely, as observed on Corsica Island. However, it was possible to see differences between light and heavy oils. Our results confirm that the higher the API grade, the smaller the sea surface attenuation, and the higher will be the backscattering values over oil spills in SAR images. On the other hand, the lower the API, the denser the oil slicks, the higher the sea surface attenuation, and the lower the backscattering values.
Our analyses show that near the edges of the patches, oil slicks had higher backscatter values than in the central portions, where the oil is more impacted by weathering processes. This pattern is associated with a higher concentration of oil in the core of the spill and a greater effect of weathering on the edges. The events that occurred on the island of Corsica and on the Deepwater Horizon (Gulf of Mexico) showed that oil weathering is strongly associated with an increase in the backscattered signal. The damping ratio values confirmed the role of weathering in modifying the radar signal backscatter.
Author contributions
Conceptualization, M.R.A.C., L.F.F.M.; methodology, M.R.A.C., L.F.F.M.; software execution, M.R.A.C., L.F.F.M.; writing – original draft preparation, M.R.A.C., L.F.F.M.; writing – review and editing, M.R.A.C., L.F.F.M., supervision, L.F.F.M., C.A.D.L., A.T.C.L. all authors have read and agreed to the published version of the manuscript.
Acknowledgments
We appreciate comments and suggestions from the anonymous reviewers that helped improve the quality and presentation of the manuscript.
Disclosure statement
No potential conflict of interest was reported by the author(s).
Additional information
Funding
References
- Ajadi, O. A., Meyer, F. J., Tello, M., & Ruello, G. (2018). Oil spill detection in synthetic aperture radar images using Lipschitz-regularity and multiscale techniques. IEEE Journal of Selected Topics in Applied Earth Observations and Remote Sensing, 11(7), 2389–13. https://doi.org/10.1109/JSTARS.2018.2827996
- Alpers, W., Holtb, B., & Zzengc, K. (2017). Oil spill detection by imaging radars: Challenges and pitfalls. Remote Sensing of Environment, 201, 133–147. https://doi.org/10.1016/j.rse.2017.09.002
- Alpers, W., & Hühnerfuss, H. (1989). The damping of ocean waves by surface films: A new look at an old problem. Journal of Geophysical Research, 94(C5), 6251–6265. https://doi.org/10.1029/JC094iC05p06251
- Benelli, G., & Garzelli, A. (1999). Oil-spills detection in SAR images by fractal dimension estimation. In Geoscience and Remote Sensing Symposium, 28 June 1999–02 July 1999, Online Publication (Vol. 1, pp. 218–220). IEEE 1999 International Geoscience and Remote Sensing Symposium. IGARSS'99 (Cat. No.99CH36293).
- Beyer, J., Trannum, H. C., Bakke, T., Hodson, P. V., & Collier, T. K. (2016). Environmental effects of the Deepwater Horizon oil spill: A review. Marine Pollution Bulletin, 110(1), 28–51. https://doi.org/10.1016/j.marpolbul.2016.06.027
- Chaturvedi, S. K., Banerjee, S., & Lele, S. (2020). An assessment of oil spill detection using Sentinel 1 SAR-C images. Journal of Ocean Engineering and Science, 5(2), 166–135. https://doi.org/10.1016/j.joes.2019.09.004
- Chedi, I. Y., Amitrano, D., & Guida, R.2021 Influence on radar back-scatter of oil spreading and evaporation in marine oil spills. In EUSAR 2021; 13th European Conference on Synthetic Aperture Radar, 29 March 2021–01 April 2021, Online Conference (pp. 1–6). VDE.
- Chung, S., Loh, A., Jennings, C. M., Sosnowski, K., Ha, S. Y., Yim, U. H., & Yoon, J. Y. (2023). Capillary flow velocity profile analysis on paper-based microfluidic chips for screening oil types using machine learning. Journal of Hazardous Materials, 447, 130806. https://doi.org/10.1016/j.jhazmat.2023.130806
- Conceição, M. R. A., Mendonça, L. F. F., Lentini, C. A. D., Lima, A. T. C., Lopes, J. M., Vasconcelos, R. N., Gouveia, M. B., & Porsani, M. J. (2021). SAR oil spill detection System through random forest classifiers. Remote Sensing, 13(11), 2044. https://doi.org/10.3390/rs13112044
- Ermakov, S. A., Sergievskaya, I. A., Da Silva, J. C., Kapustin, I. A., Shomina, O. V., Kupaev, A. V., & Molkov, A. A. (2018). Remote sensing of organic films on the water surface using dual co-polarized ship-based X-/C-/S-band radar and TerraSAR-X. Remote Sensing, 10(7), 1097. https://doi.org/10.3390/rs10071097
- Espeseth, M. M., Skrunes, S., Jones, C. E., Brekke, C., Holt, B., & Doulgeris, A. P. (2017). Analysis of evolving oil spills in full-polarimetric and hybrid-polarity SAR. IEEE Transactions on Geoscience and Remote Sensing, 55(7), 4190–4210. https://doi.org/10.1109/TGRS.2017.2690001
- Fingas, M. (2011). Introduction to oil chemistry and properties. In M. FINGAS. (ed.), Oil spill science and technology: Prevention, response, and cleanup (1st ed., Vol. 3, pp. 51–59). Elsevier. https://doi.org/10.1016/B978-1-85617-943-0.10003-6
- Fingas, M. (2018). The challenges of remotely measuring oil slick thickness. Remote Sensing, 10(2), 319. https://doi.org/10.3390/rs10020319
- Gade, M., Alpers, W., Hühnerfuss, H., Masuko, H., & Kobayashi, T. (1998). Imaging of biogenic and anthropogenic ocean surface films by the multifrequency/multipolarization SIR‐C/X‐SAR. Journal of Geophysical Research: Oceans, 103(C9), 18851–18866. https://doi.org/10.1029/97JC01915
- Gong, Y., Zhao, X., Cai, Z., O’reilly, S. E., Hao, X., & Zhao, D. (2014). A review of oil, dispersed oil and sediment interactions in the aquatic environment: Influence on the fate, transport and remediation of oil spills. Marine Pollution Bulletin, 79(1–2), 16–33. https://doi.org/10.1016/j.marpolbul.2013.12.024
- Guzmán-Osorio, F. J., Adams, R. H., Domínguez-Rodríguez, V. I., Lobato-García, C. E., Guerrero-Peña, A., Barajas-Hernández, J. R., & Baltierra-Trejo, E. (2020). Alternative method for determining API degrees of petroleum in contaminated soil by FTIR. Egyptian Journal of Petroleum, 29(1), 39–44. https://doi.org/10.1016/j.ejpe.2019.10.002
- Hersbach, H. (2010). Comparison of C-band scatterometer CMOD5. Equivalent neutral winds with ECMWF. Journal of Atmospheric and Oceanic Technology, 27(4), 4, 721–736. https://doi.org/10.1175/2009JTECHO698.1
- Huang, X., Zhang, B., Perrie, W., Lu, Y., & Wang, C. (2022). A novel deep learning method for marine oil spill detection from satellite synthetic aperture radar imagery. Marine Pollution Bulletin, 179, 113666. https://doi.org/10.1016/j.marpolbul.2022.113666
- Jayasinghe, A., Elliott, S., Gibson, G. A., & Vandemark, D. (2022). The role of phytoplankton biomacromolecules in controlling ocean surface roughness. Atmosphere, 13(12), 2101. https://doi.org/10.3390/atmos13122101
- Jha, M. N., Levy, J., & Gao, Y. (2008). Advances in Remote Sensing for oil spill disaster management: State-of-the-art sensors Technology for oil spill surveillance. Sensors, 8(1), 236–255. https://doi.org/10.3390/s8010236
- Johnson, J. W., & Croswell, W. F. (1982). Characteristics of 13.9 GHz radar scattering from oil films on the sea surface. Radio Science, 17(3), 611–617. https://doi.org/10.1029/RS017i003p00611
- Keramea, P., Spanoudaki, K., Zodiatis, G., Gikas, G., & Sylaios, G. (2021). Oil spill modeling: A critical review on current trends, perspectives, and challenges. Journal of Marine Science and Engineering, 9(2), 181. https://doi.org/10.3390/jmse9020181
- Latini, D., Del Frate, E., & Jones, C. F. (2016). Multi-frequency and polarimetric quantitative analysis of the Gulf of Mexico oil spill event comparing different SAR systems. Remote Sensing of Environment, 183, 26–42. https://doi.org/10.1016/j.rse.2016.05.014
- Lentini, C. A. D., Mendonça, L. F. F., Conceição, M. R. A., Lima, A. T., Vasconcelos, R. N., & Porsani, M. J. (2022). Comparison between oil spill images and look-alikes: An evaluation of SAR-derived observations of the 2019 oil spill incident along Brazilian waters. Anais da Academia Brasileira de Ciências, 94(suppl 2), 94. https://doi.org/10.1590/0001-3765202220211207
- Li, P., Cai, Q., Lin, W., Chen, B., & Zhang, B. (2016). Offshore oil spill response practices and emerging challenges. Marine Pollution Bulletin, 110(1), 6–27. https://doi.org/10.1016/j.marpolbul.2016.06.020
- Liubartsevaa, S., Smaouib, M., Coppinic, G., Gonzales, G., Leccic, R., Cretìc, S., & Federico, I. (2020). Model-based reconstruction of the Ulysse-Virginia oil spill, October–November 2018. Marine Pollution Bulletin, 154, 154. https://doi.org/10.1016/j.marpolbul.2020.111002
- Marta-Almeida, M., Ruiz-Villarreal, M., Pereira, J., Otero, P., Cirano, M., Zhang, X., & Hetland, R. D. (2013). Hetland efficient tools for marine operational forecast and oil spill tracking. Marine Pollution Bulletin, 71(1–2), 139–151. https://doi.org/10.1016/j.marpolbul.2013.03.022
- Meng, T., Yang, X., Chen, K. S., Nunziata, F., Xie, D., & Buono, A. (2021). Radar backscattering over sea surface oil emulsions: Simulation and observation. IEEE Transactions on Geoscience and Remote Sensing, 60, 1–14. https://doi.org/10.1109/TGRS.2021.3073369
- Migliaccio, M., Nunziata, F., Montuori, A., Li, X., & Pichel, W. G. (2011). Multi-frequency polarimetric SAR processing chain to observe oil fields in the Gulf of Mexico. IEEE- Transactions on Geoscience and Remote Sensing, 44(12), 4729–4737. https://doi.org/10.1109/TGRS.2011.2158828
- Minchew, B., Jones, C. E., & Holt, B. (2012). Polarimetric analysis of backscatter from the Deepwater Horizon oil spill using L-band synthetic aperture radar. IEEE Transactions on Geoscience & Remote Sensing, 50(10), 3812–3830. https://doi.org/10.1109/TGRS.2012.2185804
- Mohammadiun, S., Hu, G., Gharahbagh, A. A., Li, J., Hewage, K., & Sadiq, R. (2021). Intelligent computational techniques in marine oil spill management: A critical review. Journal of Hazardous Materials, 419, 126425. https://doi.org/10.1016/j.jhazmat.2021.126425
- Naz, S., Iqbal, M. F., Mahmood, I., & Allam, M. (2021). Marine oil spill detection using synthetic aperture radar over indian ocean. Marine Pollution Bulletin, 162, 111921. https://doi.org/10.1016/j.marpolbul.2020.111921
- Nissanka, I. D., & Yapa, P. D. (2018). Calculation of oil droplet size distribution in ocean oil spills: A review. Marine Pollution Bulletin, 135, 723–734. https://doi.org/10.1016/j.marpolbul.2018.07.048
- Nunziata, F., Buono, A., & Migliaccio, M. (2018). COSMO-SkyMed synthetic aperture radar data to observe the deepwater horizon oil spill. Sustainability, 10(10), 3599.
- Pabón, R. E. C., & de Souza Filho, C. R. (2019). Crude oil spectral signatures and empirical models to derive API gravity. Fuel, 237, 1119–1131. https://doi.org/10.1016/j.fuel.2018.09.098
- Quigley, C., Brekke, C., & Eltoft, T. (2020). Retrieval of marine surface slick dielectric properties from radarsat-2 data via a polarimetric two-Scale model. IEEE Transactions on Geoscience and Remote Sensing, 58(7), 5162–5178. https://doi.org/10.1109/TGRS.2020.2973724
- Rix, G. J., Lai, C. G., & Spang, A. W., Jr. (2000). In situ measurement of damping ratio using surface waves. Journal of Geotechnical and Geoenvironmental Engineering, 126(5), 472–480. https://doi.org/10.1061/(ASCE)1090-0241(2000)126:5(472)
- Sato, T., Suzuki, Y., Kashiwagi, H., Nanjo, M., & Kakui, Y. (1978). Laser radar for remote detection of oil spills. Applied Optics, 17(23), 3798–3803. https://doi.org/10.1364/AO.17.003798
- Sergievskaya, I., Ermakov, S., Lazareva, T., & Guo, J. (2019). Damping of surface waves due to crude oil/oil emulsion films on water. Marine Pollution Bulletin, 146, 206–214. https://doi.org/10.1016/j.marpolbul.2019.06.018
- Shen, H., Perrie, W., & Wu, Y. (2019). Wind drag in oil spilled ocean surface and its impact on wind-driven circulation. Anthropocene Coasts, 2(1), 244–260. https://doi.org/10.1139/anc-2018-0019
- Shinga, S., Vespe, M., & Trieschmann, O. (2013). Automatic synthetic aperture radar based oil spill detection and performance estimation via a semi-automatic operational service benchmark. Marine Pollution Bulletin, 73(1), 199–209. https://doi.org/10.1016/j.marpolbul.2013.05.022
- Shuming, L., Ziwei, L. I., Xiaofeng, Y., William, P. G., Yang, Y., Zheng, Q., & Xiaofeng, L. I. (2010). Atmospheric frontal gravity waves observed in satellite SAR images of the Bohai sea and Huanghai sea. Acta Oceanologica Sinica, 29(5), 35–43. https://doi.org/10.1007/s13131-010-0061-8
- Skruners, S., Brekke, C., Eltoft, T., & Kudryavtsev, V. (2014). Comparing near-coincident C-and X-band SAR acquisitions of marine oil spills. IEEE Transactions on Geoscience and Remote Sensing, 53(4), 1958–1975. https://doi.org/10.1109/TGRS.2014.2351417
- Skruners, S., Brekke, C., Eltoft, T., & Kudryavtsev, V. (2015). Comparing near-coincident C- and X-Band SAR Acquisitions of marine oil spills. IEEE Transactions on Geoscience and Remote Sensing, 53(4), 1958–1975. https://doi.org/10.1109/TGRS.2014.2351417
- Ülker, D., Burak, S., Balas, L., & Çağlar, N. (2022). Mathematical modelling of oil spill weathering processes for contingency planning in Izmit Bay. Regional Studies in Marine Science, 50, 102155. https://doi.org/10.1016/j.rsma.2021.102155
- Vafaie, A., & Kivi, I. R. (2020). An investigation on the effect of thermal maturity and rock composition on the mechanical behavior of carbonaceous shale formations. Marine and Petroleum Geology, 116, 104315. https://doi.org/10.1016/j.marpetgeo.2020.104315
- Wang, Z., An, C., Lee, K., Owens, E., Chen, Z., Boufadel, M., Taylor, E., & Feng, Q. (2021). Factors influencing the fate of oil spilled on shorelines: A review. Environmental Chemistry Letters, 19(2), 1611–1628. https://doi.org/10.1007/s10311-020-01097-4
- Wang, Q., Leonce, B., Meredith, E. S., Adegboyega, N. F., Lu, K., Hockday, W. C., & Liu, W. (2020). Elucidating the formation pathway of photo-generated asphaltenes from light Louisiana sweet crude oil after exposure to natural sunlight in the Gulf of Mexico. Organic Geochemistry, 150. https://doi.org/10.1016/j.orggeochem.2020.104126
- Ward, C. P., Sharpless, C. M., Valentine, D. L., French McCay, D. P., Aeppli, C., White, H. K., Rodgers, R. P., Gosselin, K. M., Nelson, R. K., & Reddy, C. M. (2018). Partial photochemical oxidation was a dominant fate of Deepwater Horizon surface oil. Environmental Science & Technology, 52(4), 1797–1805. https://doi.org/10.1021/acs.est.7b05948
- Wismann, V., Gade, M., Alpers, W., & Hühnerfuss, W. H. (1998). Radar signatures of marine mineral oil spills measured by an airborne multi-frequency radar. International Journal of Remote Sensing, 19(18), 3607–3623. https://doi.org/10.1080/014311698213849
- Xu, J., Wang, H., Cui, C., Zhao, B., & Li, B. (2020). Oil spill monitoring of shipborne radar image features using SVM and local adaptive threshold. Algorithms, 13(3), 69. https://doi.org/10.3390/a13030069
- Yekeen, S. T., Balogun, A. L., & Yusof, K. B. W. (2020). A novel deep learning instance segmentation model for automated marine oil spill detection. ISPRS Journal of Photogrammetry and Remote Sensing, 167, 190–200. https://doi.org/10.1016/j.isprsjprs.2020.07.011
- Yim, U. H., Ha, S. Y., An, J. G., Won, J. H., Han, G. M., Hong, S. H., Kim, M., Jung, J. H., & Shim, W. J. (2011). Fingerprint and weathering characteristics of stranded oils after the Hebei Spirit oil spill. Journal of Hazardous Materials, 197, 60–69. https://doi.org/10.1016/j.jhazmat.2011.09.055
- Zhang, K., Huang, J., Mansaray, L. R., Guo, Q., & Wang, X. (2018). Developing a subswath-based wind speed retrieval model for Sentinel-1 VH-polarized SAR data over the ocean surface. IEEE Transactions on Geoscience and Remote Sensing, 57(3), 1561–1572. https://doi.org/10.1109/TGRS.2018.2867438
- Zhang F. F., and Li, S. L. (2011). A study on oil spill identification based on backscattering of SAR data. 5th International Conference on Bioinformatics and Biomedical Engineering, 10–12 May 2011, Wuhan (Vol.1, pp. 4). https://doi.org/10.1109/icbbe.2011.5780769
- Zhang, Y., Li, Y., Liang, X. S., & Tsou, J. (2017). Comparison of oil spill classifications using fully and compact polarimetric SAR images. Applied Sciences, 7(2), 193. https://doi.org/10.3390/app7020193