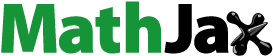
ABSTRACT
Numerous studies have evaluated the application of Remote Sensing (RS) techniques for mapping actual evapotranspiration (ETa) using Vegetation-Index-based (VI-based) and surface energy balance methods (SEB). SEB models computationally require a large effort for application. VI-based methods are fast and easy to apply and could therefore potentially be applied at high resolution; however, the accuracy of VI-based methods in comparison to SEB-based models remains unclear. We tested the ETa computed with the modified 2-band Enhanced Vegetation Index (METEVI2) implemented in the Google Earth Engine – for mapping croplands’ water use dynamics in the Lower Colorado River Basin. We compared METEVI2 with the well-established RS-based products of OpenET (Ensemble, eeMETRIC, SSEBop, SIMS, PT_JPL, DisALEXI and geeSEBAL). METEVI2 was then evaluated with measured ETa from four wheat fields (2017–2018). Results indicated that the monthly ETa variations for METEVI2 and OpenET models were comparable, though of varying magnitudes. On average, METEVI2 had the lowest difference rate from the average observed ETa with 17 mm underestimation, while SIMS had the highest difference rate (82 mm). Findings show that METEVI2 is a cost-effective ETa mapping tool in drylands to track crop water use. Future studies should test METEVI2’s applicability to croplands in more humid regions.
Introduction
Water shortages in the arid and semi-arid regions have led an increasing number of countries to face serious water deficiencies (Peña-Arancibia et al., Citation2016) and restricted agricultural production. Globally, irrigation consumes more than 70% of freshwater (Peña-Arancibia et al., Citation2016); nevertheless, the spatial distribution and water use of many important irrigated areas are uncertain (Peña-Arancibia et al., Citation2014). Water availability is crucial in agricultural areas located in semiarid regions where regular irrigation is essential to complete the crops’ growth. Since crops in semiarid regions grow during the driest months, irrigation scheduling is critical (Bhattacharya, Citation2019).
Estimating crop evapotranspiration (ETc) is essential for monitoring irrigation performance (Poudel et al., Citation2021), particularly in areas with limited water availability (Jamshidi et al., Citation2019; Zare et al., Citation2021). Actual ET (ETa) is a key climate variable in linking water, carbon and energy cycles (Fisher et al., Citation2017; Pan et al., Citation2020), and its direct measurement through conventional techniques such as lysimeter and eddy covariance at the large scale is not feasible. This explains why there are few studies with a sufficient number of ETa measurement devices that capture the spatial variability of ETa (El Ghandour et al., Citation2019). For instance, the global network of eddy flux towers dataset (FLUXNET2015) provides data from 212 sites around the globe (Pastorello et al., Citation2020) with most towers located in the United States and Europe and few towers in croplands across semiarid and arid regions. To address this limitation, various approaches have been developed in recent years to estimate ETa at a large scale. When in situ ETa measurements are unavailable, crop models can be used to estimate ETa under various crop and soil moisture conditions (Allen, Citation2000). Many difficulties arise when attempting to apply crop models at regional scales including availability and quality of essential model input data such as cropping calendars or soil information (Biggs et al., Citation2015).
The use of precision agricultural techniques for field-scale monitoring and mapping of crop phenology, crop water use and crop production has been expanded through the application of technologies such as Earth observation (Barasa et al., Citation2021). The utilization of remote sensing (RS) has the potential to enhance the precision of ETa estimation and, consequently, facilitate agricultural water management (Wanniarachchi & Sarukkalige, Citation2022). This is primarily attributed to the advantageous aspect of RS data, which offers high spatial resolution, enabling detailed monitoring and analysis of agricultural landscapes (Jamshidi et al., Citation2021; Niyogi et al., Citation2020). ETa estimated by RS has been used to study drought (Senay et al., Citation2022), improve hydrological models and applications (Dile et al., Citation2020; Roy et al., Citation2017), monitor water consumption dynamics (Senay et al., Citation2017) and irrigation performance and water productivity (Ayyad et al., Citation2019; Poudel et al., Citation2021), quantify groundwater use by crops in irrigated areas (Nhamo et al., Citation2020), estimate the water balance (Falalakis & Gemitzi, Citation2020) and groundwater recharge (Githui et al., Citation2012). Within past decades, advances in Earth observation have contributed to improving the quantification of ETa arranging across fields, through continental, and global scales (Guerschman et al., Citation2022; Jaafar et al., Citation2022; Senay et al., Citation2022).
Conventional ways of processing RS data include downloading data and processing them, which are challenging and require terabytes of storage space and processing systems (Amani et al., Citation2020). A cloud-based platform, such as Google Earth Engine (GEE), effectively tackles the challenges associated with processing large volumes of data, enabling long-term monitoring of environmental parameters without the requirement to download and locally manage data (Amani et al., Citation2020). Moreover, GEE enhances computational speed and facilitates result sharing, thereby making it possible to achieve a precise and efficient estimation of ETa across extensive geographical regions. The GEE’s capacity to process and analyze extensive collections of satellite imagery made it an efficient tool for crop monitoring purposes as used in different research (Mhawej & Faour, Citation2020; Naghdyzadegan Jahromi et al., Citation2023; Senay et al., Citation2022).
Different RS-based approaches to estimate ETa include surface energy balance (SEB) and vegetation index (VI) methods (Calera et al., Citation2017; González-Dugo & Mateos, Citation2008). A thermal band obtained from satellites is used in SEB equations to estimate ETa. SEB models solve the energy balance equation to calculate ETa using a range of empirical and physically based models, input data and assumptions (Sishodia et al., Citation2020). Some of the widely used SEB models include the Surface Energy Balance Algorithm for Land (SEBAL) (Bastiaanssen et al., Citation1998), Mapping ETa at High Resolution using Internalized Calibration (METRIC) (Allen et al., Citation2007) and the Operational Simplified Surface Energy Balance (SSEBop) model by Senay et al. (Citation2013). SEB models require a relatively large effort for parameterization and application. Anchor pixel selection with extreme temperature and vegetation conditions by the user can constrain these models’ ability to estimate ETa during periods when vegetation activity is not at its peak (Jaafar et al., Citation2022). In contrast, VI-based approaches are quick and simple to implement, and hence have the potential to be used at high resolution and large extent using optical bands (Glenn et al., Citation2010; Ramón-Reinozo et al., Citation2019). To apply a VI-based method, a widely used approach to calculate ETa is based on the Food and Agriculture Organization (FAO) method for estimating ETc, in which reference evapotranspiration (ETo) is multiplied by coefficients representing crop characteristics and water stress condition (Kc and Ks respectively) (Allen, Citation1998; González-Dugo & Mateos, Citation2008). ETo defines the influence of weather on the ETa rate of the reference crop (grass), and Kc and Ks scale ETo to ETa (Allen, Citation1998; Pereira et al., Citation2021). VI is assumed as a proxy of the product of Kc and Ks capturing the actual crop growth condition and its spatial variability (Abbasi et al., Citation2021). Although several VI-based algorithms have been presented for specific irrigation districts, no single ET-VI method has been rigorously evaluated over a wide range of irrigation districts. Moreover, the accuracy of this method in comparison to various SEB models is yet unclear.
As a first attempt to improve and extend evaluations, Abbasi et al. (Citation2021) developed and applied an ET-VI method, ET-EVI2, in GEE for a 20-year study in a large irrigation district, the Zayandeh-Rud River Basin (ZRB), for analyzing crop water use. This approach could generate monthly ETa maps at large scales with minimal input requirements. The results of this study have been encouraging, but a systematic evaluation of the accuracy of the method was hampered by a lack of ground observations and independent estimates obtained with other RS-based methods. This product (ET-EVI2), originally built for a watershed level in a dry region (Abbasi et al., Citation2021), encouraged us to expand the extent of the model and test it on a different region. Therefore, in a lack of a large-scale VI-based ETa product with a medium resolution (30 m), for the first time, the reliability and transferability of ET-EVI2 were evaluated in mapping the water use dynamics of croplands over arid and semi-arid regions.
To introduce a large-scale ETa product and improve modeling and mapping water use dynamics of arid and semi-arid croplands, this study modifies and tests the ET-EVI2 across the Lower Colorado River Basin (LCRB) and evaluates it systematically against commonly used RS products of OpenET and ground-based observations obtained in several wheat fields in the study region. OpenET provides open access RS-based information on ETa over agricultural areas using multiple ETa models across the western United States (Melton et al. Citation2021). In this research, we pursue to answer: a) how does the modified ET-EVI2 (referred to hereinafter as METEVI2) perform compared to OpenET models at monthly and seasonal scales?, b) are there a systematic seasonal and monthly over- or underestimation in METEVI2 and OpenET models?, and c) how well do monthly METEVI2 and OpenET perform in comparison to wheat fields’ ETa?
Material and methods
Study area
The Colorado River (CR) is southwestern North America’s largest and most important river (Stevens et al., Citation2020) and is a strictly regulated transboundary river (Sullivan et al., Citation2019). The CR basin () is divided into two basins of upper Colorado (Colorado, New Mexico, Utah and Wyoming) and lower Colorado (Arizona, California and Nevada) for water delivery and management purposes (Sullivan et al., Citation2019). The Upper CR basin (UCRB), which exports water, has an area of 283,384 km2, and the water-receiving LCRB is 344,440 km2. Groundwater supplies approximately half of the river’s baseflow (Stevens et al., Citation2020). The lower CR flows 570 km from Hoover Dam to the southern International Boundary with Mexico (Nagler et al., Citation2009). To keep the land suitable for farming, more than 90% of the basin’s pasture and cropland receive additional irrigation water. CR basin has continental and arid weather (Stevens et al., Citation2020). The UCRB uses less irrigation water than the LCRB due to shorter growing seasons and cooler climates. LCRB and Mexican fields receive about four times as much water as UCRB fields (Cohen et al., Citation2013). Reduced runoff, earlier snowmelt, and more severe droughts are anticipated due to climate change in the CR (Garrick et al., Citation2014). Despite the arid climate, regions in the west that use CR water have shown to be agriculturally productive, producing a variety of crops like alfalfa, hay, wheat, cotton and lettuce. Currently, 78% of the CR’s water is used for agriculture. Due to the presence of larger cities and agricultural activities as well as the LCRB’s more arid climate, water demand has historically been higher there (The State of the Rockies Project Citation2011–12 Research Team, 2012). Our study focuses on the adjacent croplands from Hoover to Morelos Dam ().
Figure 1. Study area; a) Colorado river basin (shapefile of the CRB was downloaded from https://coloradoriverbasin-lincolninstitute.hub.arcgis.com/, last accessed: February 2022); b) croplands located in the LCRB; c) a zoom window of random polygons.

METEVI2 calculation
The ET-EVI2 model was developed in the GEE platform for agricultural areas in the ZRB using Landsat imagery (Abbasi et al., Citation2021):
with ETo initially calculated by using the Global Crop Water Model (GCWM) and applying the FAO-56 Penman – Monteith method forced by hourly ERA5 reanalysis data as climate input. GCWM is executed in daily time steps and produces ETo with a spatial resolution of about 8 km (Meza et al., Citation2021). Performance assessment of the ET-EVI2 across croplands in the ZRB (Abbasi et al., Citation2021, Citation2023) confirmed that this index tended to overestimate ETa in agricultural areas. Therefore, by implementing FAO-56’s Kc principles (Allen, Citation2000), we modified the original scaling factor “1.65” by considering a maximum of 1.1 for the crop coefficient Kc while before a maximum Kc of 1.2 was used. Based on FAO-56, for most crops, Kc values peak at 1.1. Also, according to FAO-56 (table 12, FAO-56 report (Allen et al., Citation1998)), only about four crops exhibit a Kc mid of 1.2, while the majority of crops have a Kc mid ranging from 1 to 1.15. It is important to note that these values were derived under optimal, non-water-stressed and well-irrigated conditions. However, in reality, maintaining such conditions for all crops and fields during the growing season may not be feasible. As a result, it is possible that Kc mid may not attain its maximum value as reported in FAO-56 due to the influence of varying environmental conditions and potential water stress on crops.
Consequently, the new scaling factor was calculated as:
and the index METEVI2 was computed subsequently as:
To compare the METEVI2 and MEVI2 with OpenET data and in situ measurements (), monthly ETa estimates were extracted. GEE was used to implement the model and calculate monthly METEVI2 and MEVI2. We were able to develop METEVI2 and MEVI2 with a 30 m resolution over large areas using cloud computing rather than traditional desktop hardware constraints. As a result, METEVI2 was calculated monthly across various regions from 1990 to 2021. It is worth noting that after downloading the METEVI2 layers from GEE, missing monthly METEVI2 layers were filled in with average values from the preceding and following months’ METEVI2 layers.
OpenET dataset description
OpenET (https://openetdata.org/about/, last access: October 2022) provides ETa across agricultural areas from multiple models for the western United States and also calculates a single “ensemble value” from all these models. All models currently use Landsat imagery to produce ETa estimates at a spatial resolution of 30 m. The majority of the models that make up the OpenET ensemble are based on full or simplified implementations of the SEB approach: 1) eeMETRIC (Mapping Evapotranspiration at high Resolution with Internalized Calibration (Allen et al., Citation2007; Ayse et al., Citation2011); 2) geeSEBAL (Surface Energy Balance Algorithm for Land developed within the GEE environment) (Laipelt et al., Citation2021) which is based on the original algorithms introduced by (Bastiaanssen et al., Citation1998); 3) DisALEXI (The Disaggregated Atmosphere – Land Exchange Inverse (Anderson et al., Citation2018, Citation2012); 4) PT_JPL (The Priestley – Taylor evapotranspiration (Fisher et al., Citation2008); 5) SIMS (Satellite Irrigation Management Support (Pereira et al., Citation2020)) relies on surface reflectance data and crop type information to compute ETa as a function of canopy density using a Kc approach for agricultural lands; 6) The Operational Simplified Surface Energy Balance (SSEBop) model is an SEB model (Senay et al., Citation2013, Citation2017); 7) Ensemble is calculated from an ensemble of the preceding six models. OpenET products are available from 2017 to 2022. Due to strong overall agreement with the flux towers’ ETa for all OpenET models (Melton et al. Citation2021), we used OpenET estimates to evaluate our METEVI2 model in arid and semi-arid regions.
Extracting OpenET and METEVI2 in LCRB
The shapefile of agricultural fields located in the LCRB was obtained from the U.S. Bureau of Reclamation. To compare OpenET with METEVI2 at the field scale using QGIS (QGIS.org,Citation2021), we randomly selected 70 polygons to extract ETa products for each field. Among these selected polygons, 57 polygons () were included, while non-crop polygons such as orchards and dates were excluded. Different crops such as alfalfa, cotton, wheat, cabbage, etc. are cultivated in this region. ETa and Kc rates estimated from different RS-based models were then extracted in each field from 2017 to 2021. The annual average ETa (mm) in each field was calculated to determine the crop water consumption in volumetric units (m3) using the corresponding field area, as shown below:
where ETa (m) is the annual averaged RS-based ETa for a given field, and Area (m2) is the area of the corresponding field.
Observed ETa and Kc values
In situ data collected and published by French et al. (Citation2020) were utilized to compare the performance of RS-based ETa and Kc products. They investigated the capacity of RS-based VIs to estimate ETa of wheat over 7 sites between 2016 and 2019 in Yuma and Maricopa, Arizona, USA, by using Sentinel 2 and Venus satellites. ETa was measured using eddy covariance stations in each field over the same growing season. In their study, daily values of the single Kc were calculated for sites by dividing the observed daily ETa by daily ETo. Linear FAO-56 Kc curves were derived by visually fitting the Kc data to the initial, mid-season and late-season growth stages. ETa values in this study were reported as total ETa for the whole growing season. In this study, four durum wheat fields (2017–2018) located in our study area () were chosen to compare RS-based ETa and Kc values with reported values. FAO-Kc values were reported as graphs at a daily scale, while RS-based Kc values were at a monthly scale; therefore, we interpolated FAO-56 Kc values and averaged daily values to monthly to find equivalent monthly FAO-56 Kc values and compare them with RS-based estimates. We excluded December (wheat 3) and January (wheat 4) in the calculation of monthly Kc curves because sowing dates for these sites occurred in the middle and at the end of the months, respectively.
Table 1. Wheat fields’ planting and harvesting dates extracted from French et al. (Citation2020).
Model performance evaluation
The following metrics were used to evaluate the METEVI2 and MEVI2 performances: 1) a correlation matrix to determine the relationship between different OpenET models and METEVI2 estimates in each field; 2) intercomparison of estimated ETa rates using monthly and seasonal graphs; 3) intercomparison of the different models using average volume (m3) of estimated ETa in each field; 4) evaluating of RS-based ETa and Kc rates using observed values.
Results
METEVI2 and OpenET products intercomparison
Monthly graphs of each field were used to illustrate the inter-annual variability of ETa estimates (Appendix 1). Due to a large number of monthly graphs, only the average ETa of RS-ETs across all fields was presented in ); the monthly figures can be found in Appendix (1). At a 30 m resolution, METEVI2 and OpenET showed the in-field ETa variations. This temporal behavior of the majority of RS-based ETa models agreed and was similar across the majority of the fields. In most fields, METEVI2’s monthly graphs agreed with OpenET products. ) demonstrates that METEVI2’s estimates were in close agreement with eeMETRIC. METEVI2 had slightly higher values during the peak growing season. Boxplots of the seasonal average of RS-ET across all fields () demonstrate that METEVI2, followed by eeMETRIC, estimated ETa higher than other RS-ETs in the spring. In summer, eeMETRIC had the highest values and then METEVI2. METEVI2 was the second RS-ET with high ETa estimates in other seasons. geeSEBAL and DisALEXI underestimated ETa in fall and winter, respectively. Small boxplots of geeSEBAL and DisALEXI in winter, spring, and fall revealed that ETa varies less than that of other RS-ETs. A correlation matrix was used to summarize the correlations and get a snapshot of the relationship between METEVI2 and OpenET products across 57 fields (). As can be seen, there was a strong correlation between METEVI2 and OpenET models. The strongest relationship was between METEVI2 and SIMS (from 0.9 to 0.99) followed by the Ensemble, while the lowest is between geeSEBAL and METEVI2 (from 0.5 to 0.96). No systematic monthly and seasonally over- or underestimation were seen in METEVI2 when compared to OpenET models.
Figure 3. a) Average ETa of each RS-ET across all fields; b) boxplots of average of seasonal RS-ETs.

demonstrates boxplots of the deviation of OpenET products from METEVI2 (ratio of individual OpenET models to METEVI2). Also, to compare the volume of water lost through ETa, we multiplied the annual RS-based ETa products in each field by the corresponding area and then calculated the average ETa volume (m3) for each model separately across all fields (). Negative values in show the differences between average METEVI2 and average OpenET models. eeMETRIC and SIMS had the lowest differences (2.6 m3 and 9.1 m3 respectively), while geeSEBAL and DisALEXI had the highest differences (22.3 m3 and 24.3 m3 respectively). DisALEXI and geeSEBAL also showed the highest deviation from METEVI2 (). eeMETRIC followed by SIMS presented the lowest deviation from METEVI2. The median value of eeMETRIC is near one showing the lower difference between estimations from eeMETRIC and METEVI2. All models’ median values except eeMETRIC were lower than one showing that these models estimate ETa lower than METEVI2. The results showed that METEVI2 and eeMETRIC had the highest estimations of consumed water. The length of boxplots for SIMS and PT_JPL showed the lowest variability in the values of SIMS and PT_JPL. illustrates a zoomed-in view of the monthly METEVI2 map at field scale in the LCRB.
Figure 5. a) Relative deviation of OpenET products from METEVI2 (ratio of individual OpenET models to METEVI2). The blue horizontal line shows value one; b) the average volume of ETa (m3) over all fields, to see the differences better y-axis starts from 100 (*1000) m3; c) a close-up view of METEVI2 map at field scale in the LCRB for July 2021.

Evaluation of RS-based ETa estimates against observed ETa
To assess the performance of METEVI2 against OpenET models, ETa estimates were compared with observed ETa of wheat for the growing season of 2017–2018 ( and ). OpenET models demonstrated a clear over- or underestimation in all wheat fields compared to the field data called wheat ETa. Ensemble, SIMS, PT_JPL, DisALEXI, and geeSEBAL underestimated ETa, while eeMETRIC and SSEBop overestimated ETa in all fields. METEVI2 underestimated ETa in two fields and overestimated ETa in other fields.
Figure 6. Wheat and RS-based ETa in four fields. Values on the bars show the difference between wheat ETa and OpenET models. To see the differences better y-axis starts from 300 mm.

Table 2. Wheat ETa reported by French et al. (Citation2020) and RS-based ETa (mm).
reports the estimated and observed ETa rates in each field and also the average values of all fields. The average ETa of METEVI2 had the lowest difference rate from observed values (17 mm underestimation), while the highest difference rate was estimated by SIMS (82 mm underestimation). In all four fields, eeMETRIC and SSEBop overestimated ETa by an average of 8% (47 mm and 50 mm accordingly) and METEVI2 underestimated ETa by an average of 3% (17 mm). The total RS-based ETa rates in all fields were within 2 to 18% of the observed ETa and, on average, within 3 to 13%. The point-based comparison versus observed ETa data revealed that METEVI2 accuracy varies by field, although on average, the METEVI2 performs better.
Evaluation of RS-based Kc estimates
Averages of all estimated and observed Kc values are illustrated in . presents Kc curves of RS-based and observed Kc values during the growing seasons of four wheat fields. SSEBop and eeMETRIC overestimated Kc values during the mid and end seasons (), while other models, particularly MEVI2, underestimated Kc values in all fields. geeSEBAL could not capture the Kc values during the end stage of crop growing season () and had the highest differences with FAO-Kc during all growth stages. shows that all Kc estimates were lower than FAO-Kc values during the initial stage. DisALEXI and SIMS had the lowest difference with FAO-Kc during the end stage with 0.06 (. While in all fields, MEVI2 has lower values compared to the observed FAO-Kc values, estimated METEVI2 rates are close to or higher than that of observed ETa. The reason must be the GCWM product (Abbasi et al., Citation2021) that was used to calculate METEVI2.
Figure 7. a) Average of wheat FAO-Kc extracted from French et al. (Citation2020) vs. RS-based Kc of all wheat fields; b) wheat FAO-Kc extracted from French et al. (Citation2020) vs. RS-based Kc of each wheat field, background colors show different crop growth stage, i.e. blue: initial stage, green: middle stage, yellow: end-stages.

Discussion
We compared our modified VI-based products (METEVI2 and MEVI2) to commonly used RS products of OpenET (from 2017 to 2021) in the LCRB to introduce a large-scale VI-based ETa product with medium resolution (30 m) which can map water use dynamics of arid and semi-arid croplands. Numerous studies have highlighted the significance of having an ETa product that minimizes the bias between estimated and actual water consumption for proper climate-smart agricultural water management (Adamides, Citation2020; Jaafar et al., Citation2022; Lipper et al., Citation2014). Satellite-derived ETa maps have been actively incorporated into water resource management programs (Melton et al. Citation2021) by mapping, monitoring and forecasting the spatial variability of croplands’ water use (Schauer & Senay, Citation2019).
Our product, METEVI2, provides a time series of crop ETa in different arid and semi-arid regions over time (Abbasi et al., Citation2021). Prior studies of crop water use utilizing VI-based techniques have been site-specific studies with a constrained temporal and spatial scope and static harvested area (Johnson & Trout, Citation2012; Reyes-González et al., Citation2018). These studies employ Kc values for specific crops, assuming ideal water availability (Schauer & Senay, Citation2019). On average, the METEVI2 method displayed the least discrepancy from the observed values for ETa, with an underestimation of only 17 mm. However, a field-level analysis comparing METEVI2 to the observed ETa data showed that the accuracy of METEVI2 varied by field, but overall, it performed better than other methods. Studies conducted in various biomes have also reported a strong correlation between VIs and ETa (French et al., Citation2020; Nagler et al., Citation2013; Nouri et al., Citation2020). In a study carried out by Glenn et al. (Citation2010), it was found that the difference between measured ETa and empirical methods can have an RMSE of up to 30% of mean ETa, across different biomes. In a previous study, a comparative analysis of different ET-VI products was conducted, including the non-modified version of METEVI2 (ET-EVI2) and three NDVI-based ETa methods (ET-NDVI*, ET-NDVI*scaled and ET-NDVIKc) across the ZRB. After conducting the analysis and comparing it with in situ data, we found that ET-EVI2 outperformed the other three methods in terms of accuracy, ease of parametrization and applicability across different regions. ET-EVI2 exhibited strong performance in arid and semi-arid regions, indicating its suitability as a reliable tool for estimating ETa in these areas (Abbasi et al., Citation2023). In another study, Nouri et al. (Citation2020) examined the suitability of three VIs, namely EVI, EVI2, and NDVI*, for estimating ETa in urban green areas of Adelaide, Australia. The research employed three sensors with varying spatial resolutions to compare the performance of different satellite-based ET-VI products with in situ data. The findings of the study indicated that ETa estimated using EVI2 displayed good performance across all three sensors and exhibited the strongest correlation with in situ measurements (Nouri et al., Citation2020). Although like other RS-based ETa products, performance assessment and validation of METEVI2 are challenging, having access to several experimental fields with detailed measured ETa data and OpenET may shed some light on the model’s performance behavior. OpenET models were evaluated for accuracy using in situ ETa data from 139 flux tower sites. Results indicated an excellent agreement between the OpenET models and the flux tower ETa data (Melton et al. Citation2021).
The good agreement between our product, OpenET, and observational data was promising (). We prepared the correlogram of the average monthly RS-based ETa of 57 fields; it demonstrated a good agreement between the METEVI2 and OpenET models (). Although the models were established with different assumptions and theories, they all provided a good agreement.
Figure 8. Correlogram between the METEVI2 and OpenET models (the correlogram represents the correlations for all pairs of variables.).

Accuracy assessment was conducted between observed ETa and Kc and individual RS-based models in four wheat fields. Evaluation of METEVI2 and OpenET models showed that METEVI2 estimations followed by Ensemble, eeMETRIC, and SSEBop were the closest to the wheat ETa measurements. eeMETRIC and SSEBop tended to overestimate while SIMS, PT_JPL, DisALEXI, and geeSEBAL underestimated ETa values. All individual OpenET models showed a distinct over- or underestimation compared to observed ETa values.
During the initial stage, the RS-based Kc estimates (MEVI2 and OpenET) were observed to be lower than the FAO-Kc values. Although MEVI2 values are lower than FAO-Kc values reported for all fields, estimated METEVI2 rates are comparable to or higher than observed ETa values. The reason can be the GCWM ETo product (Abbasi et al., Citation2021) which was used to estimate METEVI2. The gridded ETo used in each RS-based ETa model may also affect the calculated ETa. Given that transpiration predominates over soil evaporation when vegetation cover is high (Biggs et al., Citation2016; French et al., Citation2020), VI-based approaches may perform as well as or better than temperature-based methods; the greatest discrepancies between these methods occur when vegetation cover is low (e.g. initial growing stage) and land surface wetness is high (Biggs et al., Citation2016). The underestimation of soil evaporation could be the cause of the disparity between measured and modeled ETa. However, the available ground data were insufficient to thoroughly determine the causes of these differences.
Different factors, such as structural complexity, model assumptions, parameterizations and the availability and reliability of data, have an impact on how well a model performs. A portion of the uncertainty in monthly estimates by different approaches is brought on by the rarity of clear satellite imagery during cloudy days (Senay et al., Citation2022). VIs change more slowly than the surface moisture conditions reflected in the land surface temperature, and the sampling frequency might be less of an issue. However, the slower response to moisture conditions can also work against VI methods. VI methods typically require less setup and coding effort than energy balance algorithms. In natural and agricultural ecosystems, VI approaches are less effective at identifying the beginning of stress conditions (Anderson et al., Citation2012; French et al., Citation2020). Moreover, due to the scarcity of reference observations, global ETa quantification has long been accompanied by significant uncertainties (Mueller et al., Citation2011). Despite yielding promising results when compared to other well-established ETa models, the METEVI2 model requires validation using more field data. Finding field data in dry and semi-arid regions was difficult; as a result, we lacked solid measurements against which to test our findings. In addition, METEVI2’s potential and limitations in different regions can be better determined by further comparison with other well-established models such as WaPOR (FAO, Citation2020). The accuracy of ETa estimates derived from SEB models can also be impacted by the timing of thermal imagery acquisition. This is especially true when there are significant changes in vegetation conditions throughout the day, resulting in fluctuations in land surface temperature and subsequent variations in ETa values (Jaafar et al., Citation2022). Also, OpenET models have some uncertainties in ETa estimation. For instance, ETa is underestimated in several arid and semi-arid grassland regions of the western United States in the current version of ALEXI which impacts the DisALEXI ETa values during the disaggregation of the ETa (https://openetdata.org/known-issues/, last access, October 2022). geeSEBAL tends to produce lower ETa estimates in desert regions and arid or semiarid climates, particularly over sparse vegetation areas. Moreover, the processing of the SSEBop model does not employ any additional cloud buffering methods. Because of insufficient cloud masking, model estimations can lead to extremely high ETa outliers (https://openetdata.org/known-issues/, last access October 2022).
The integration of the METEVI2 model into the GEE platform was one of the study’s primary goals. Like some other RS-based ETa estimation methods, the computational power of GEE and the availability of datasets on the GEE’s platform greatly facilitated running METEVI2. Knowing that developing a model is a continual process, our model has been improved since its first release (Abbasi et al., Citation2021; Nagler et al., Citation2013); we will keep working on the model to upgrade it to a global product and enhance its performance with better validation data.
Summary and conclusion
This research assessed the applicability of our model, METEVI2, which originally was developed for a watershed basin in Iran, and its performance in a dry region in LCRB in the United States. This is an exploratory step to introduce METEVI2 as a global ETa product to map and monitor cropland water consumption. Also, it underlines the value of the historical Landsat series and the ability of the METEVI2 model in capturing the spatiotemporal variability of agricultural water needs. We compared METEVI2 and MEVI2 to RS-based products of OpenET (from 2017 to 2021) in the LCRB with a medium resolution (30 m) that can map water use dynamics of arid and semi-arid croplands. To model ETa throughout dry and semi-arid regions, we implemented the METEVI2 model in the GEE cloud computing interface. The performance of METEVI2 was also tested against the observed ETa of wheat for the growing season of 2017–2018. The METEVI2 rates were comparable to ETa estimated by OpenET methods and observed ETa values and showed almost a similar monthly ETa pattern with varying magnitudes. Besides model strengths, the calculation of the soil-evaporation component was determined to be the drawback of the VI-based model, which is also the case for the SEB models. Overall, the aggregated results indicate that METEVI2 can be utilized interchangeably due to its acceptable performance in comparison to ground measurements and OpenET products. This promising outcome encourages us to pursue the goal of an automated and user-friendly global ETa application tool to fill the gap in the global ETa product. With more validation data and the incorporation of soil evaporation components, our METEVI2 product can be improved. After the application of this method over different regions, our product will be expanded to cover the arid and semi-arid regions of the whole globe. Future studies should quantify the applicability of this technique to various crops under various soil moisture stress conditions.
Acknowledgments
Our special thanks to the financial support (Ph.D. scholarship) given by the German Academic Exchange Service (DAAD). We would like to gratefully acknowledge NASA and the USGS for providing the open-access satellite data (Landsat and MODIS), Google for the GEE platform, the Bureau of Reclamation at the Boulder City office, the U.S. Department of Agriculture, Agricultural Research Service, for provisional data provided by Douglas J. Hunsaker and Andrew N. French. We are also immensely grateful to our colleagues at the University of Arizona and USGS for their scientific input and assistance. Any use of trade, firm or product names is for descriptive purposes only and does not imply endorsement by the U.S. Government.
Disclosure statement
No potential conflict of interest was reported by the author(s).
Data availability statement
Part of the data that support the findings of this study is available from the corresponding author upon reasonable request.
Additional information
Funding
References
- Abbasi, N., Nouri, H., Didan, K., Barreto-Muñoz, A., Chavoshi Borujeni, S., & Opp, C., P. Nagler, P., Thenkabail, S., and Siebert, S. (2023). Mapping vegetation index-derived actual evapotranspiration across croplands using the Google Earth Engine platform. Remote Sensing, 15(4), 1017. https://doi.org/10.3390/rs15041017
- Abbasi, N., Nouri, H., Didan, K., Barreto-Muñoz, A., Chavoshi Borujeni, S., Salemi, H., Opp, C., Siebert, S., & Nagler, P. (2021). Estimating actual evapotranspiration over croplands using vegetation index methods and dynamic harvested area. Remote Sensing, 13(24), 5167. https://doi.org/10.3390/rs13245167
- Adamides, G. (2020). A review of climate-smart agriculture applications in cyprus. Atmosphere, 11(9), 898. https://doi.org/10.3390/atmos11090898
- Allen, R. (2000). Using the FAO-56 dual crop coefficient method over an irrigated region as part of an evapotranspiration intercomparison study. Journal of Hydrology, 229(1–2), 27–22. https://doi.org/10.1016/s0022-1694(99)00194-8
- Allen, R. G., Pereira, L. S., Raes, D., & Smith, M. (1998). Crop evapotranspiration: Guidelines for computing crop water requirements. Of FAO Irrigation and Drainage Paper, 56, 0254–5284. https://www.fao.org/3/x0490e/x0490e00.htm
- Allen, R. G., Tasumi, M., & Trezza, R. (2007). Satellite-based energy balance for mapping evapotranspiration with internalized calibration (metric)‐-model. Journal of Irrigation & Drainage Engineering, 133(4), 380–394. https://doi.org/10.1061/(ASCE)0733-9437(2007)133:4(380)
- Amani, M., Ghorbanian, A., Ahmadi, S. A., Kakooei, M., Moghimi, A., Mirmazloumi, S. M., Moghaddam, S. H. A., Mahdavi, S., Ghahremanloo, M., Parsian, S., Wu, Q., & Brisco, B. (2020). Google Earth Engine cloud computing platform for remote sensing big data applications: A comprehensive review. IEEE Journal of Selected Topics in Applied Earth Observations and Remote Sensing, 13, 5326–5350. https://doi.org/10.1109/JSTARS.2020.3021052
- Anderson, M. C., Allen, R. G., Morse, A., & Kustas, W. P. (2012). Use of Landsat thermal imagery in monitoring evapotranspiration and managing water resources. Remote Sensing of Environment, 122, 50–65. https://doi.org/10.1016/j.rse.2011.08.025
- Anderson, M., Gao, F., Knipper, K., Hain, C., Dulaney, W., Baldocchi, D., Eichelmann, E., Hemes, K., Yang, Y., Medellin-Azuara, J., & Kustas, W. (2018). Field-scale assessment of land and water use change over the California Delta using remote sensing. Remote Sensing, 10(6), 889. https://doi.org/10.3390/rs10060889
- Anderson, M. C., Kustas, W. P., Alfieri, J. G., Gao, F., Hain, C., Prueger, J. H., Evett, S., Colaizzi, P., Howell, T., & Chávez, J. L. (2012). Mapping daily evapotranspiration at Landsat spatial scales during the BEAREX’08 field campaign. Advances in Water Resources, 50, 162–177. https://doi.org/10.1016/j.advwatres.2012.06.005
- Ayyad, S., Al Zayed, I. S., van Ha, T. T., & Ribbe, L. (2019). The performance of satellite-based actual evapotranspiration products and the assessment of irrigation efficiency in egypt. Water, 11(9), 1913. https://doi.org/10.3390/w11091913
- Barasa, P. M., Botai, C. M., Botai, J. O., & Mabhaudhi, T. (2021). A review of climate-smart agriculture research and applications in Africa. Agronomy, 11(6), 1255. https://doi.org/10.3390/agronomy11061255
- Bastiaanssen, W. G. M., Menenti, M., Feddes, R. A., & Holtslag, A. A. M. (1998). A remote sensing surface energy balance algorithm for land (SEBAL): 1. Formulation. Journal of Hydrology, 212-213, 198–212. https://doi.org/10.1016/s0022-1694(98)00253-4
- Bhattacharya, A. (2019). Water-use efficiency under changing climatic conditions. In changing climate and resource use efficiency in plants (pp. 111–180). Amsterdam, The Netherlands: Elsevier. https://doi.org/10.1016/B978-0-12-816209-5.00003-9
- Biggs, T. W., Marshall, M., & Messina, A. (2016). Mapping daily and seasonal evapotranspiration from irrigated crops using global climate grids and satellite imagery: Automation and methods comparison. Water Resources Research, 52(9), 7311–7326. https://doi.org/10.1002/2016WR019107
- Biggs, T. W., Petropoulos, G. P., Velpuri, N. M., Marshall, M., Glenn, E. P., Nagler, P. L., & Messina, A. 2015. Remote sensing of actual evapotranspiration from cropland. Remote sensing handbook: Remote sensing of water resources, disasters, and urban studies, Prasad S. Thenkabail. CRC Press. Available online at http://pubs.er.usgs.gov/publication/70176490.
- Calera, A., Campos, I., Osann, A., D’Urso, G., & Menenti, M. (2017). Remote sensing for crop water management: From ET modelling to services for the end users. Sensors (Basel, Switzerland), 17(5), 1104. https://doi.org/10.3390/s17051104
- Cohen, M., Christian-Smith, J., & Berggren, J. (2013). Water to supply the land irrigated agriculture in the colorado river basin. Pacific Institute.
- Dile, Y. T., Ayana, E. K., Worqlul, A. W., Xie, H., Srinivasan, R., Lefore, N., You, L., & Clarke, N. (2020). Evaluating satellite-based evapotranspiration estimates for hydrological applications in data-scarce regions: A case in Ethiopia. The Science of the Total Environment, 743, 140702. https://doi.org/10.1016/j.scitotenv.2020.140702
- El Ghandour, F.-E., Alfieri, S. M., Houali, Y., Habib, A., Akdim, N., Labbassi, K., & Menenti, M. (2019). Detecting the response of irrigation water management to climate by remote sensing monitoring of evapotranspiration. Water, 11(10), 2045. https://doi.org/10.3390/w11102045
- Falalakis, G., & Gemitzi, A. (2020). A simple method for water balance estimation based on the empirical method and remotely sensed evapotranspiration estimates. Journal of Hydroinformatics, 22(2), 440–451. https://doi.org/10.2166/hydro.2020.182
- FAO. (2020) . Wapor database methodology.
- Fisher, J. B., Melton, F., Middleton, E., Hain, C., Anderson, M., Allen, R., McCabe, M. F., Hook, S., Baldocchi, D., Townsend, P. A., Kilic, A., Tu, K., Miralles, D. D., Perret, J., Lagouarde, J.-P., Waliser, D., Purdy, A. J., French, A. … Stephens, G. (2017). The future of evapotranspiration: Global requirements for ecosystem functioning, carbon and climate feedbacks, agricultural management, and water resources. Water Resources Research, 53(4), 2618–2626. https://doi.org/10.1002/2016WR020175
- Fisher, J. B., Tu, K. P., & Baldocchi, D. D. (2008). Global estimates of the land–atmosphere water flux based on monthly AVHRR and ISLSCP-II data, validated at 16 FLUXNET sites. Remote Sensing of Environment, 112(3), 901–919. https://doi.org/10.1016/j.rse.2007.06.025
- French, A. N., Hunsaker, D. J., Sanchez, C. A., Saber, M., Gonzalez, J. R., & Anderson, R. (2020). Satellite-based NDVI crop coefficients and evapotranspiration with eddy covariance validation for multiple durum wheat fields in the US southwest. Agricultural Water Management, 239, 106266. https://doi.org/10.1016/j.agwat.2020.106266
- Garrick, D., de Stefano, L., Pittock, J., & Connell, D. (2014). Transboundary water management in federal political systems: A story of three semi-arid rivers (pp. 343–353). https://doi.org/10.1007/978-3-319-07548-8_22
- Githui, F., Selle, B., & Thayalakumaran, T. (2012). Recharge estimation using remotely sensed evapotranspiration in an irrigated catchment in southeast Australia. Hydrological Processes, 26(9), 1379–1389. https://doi.org/10.1002/hyp.8274
- Glenn, E. P., Nagler, P. L., & Huete, A. R. (2010). Vegetation index methods for estimating evapotranspiration by remote sensing. Surveys in Geophysics, 31(6), 531–555. https://doi.org/10.1007/s10712-010-9102-2
- González-Dugo, M. P., & Mateos, L. (2008). Spectral vegetation indices for benchmarking water productivity of irrigated cotton and sugarbeet crops. Agricultural Water Management, 95(1), 48–58. https://doi.org/10.1016/j.agwat.2007.09.001
- Guerschman, J. P., McVicar, T. R., Vleeshower, J., van Niel, T. G., Peña-Arancibia, J. L., & Chen, Y. (2022). Estimating actual evapotranspiration at field-to-continent scales by calibrating the CMRSET algorithm with MODIS, VIIRS, Landsat and Sentinel-2 data. Journal of Hydrology, 605, 127318. https://doi.org/10.1016/j.jhydrol.2021.127318
- Irmak, A., Ratcliffe, I., Ranade, P., Hubbard, K. G., Singh, R. K., Kamble, B., & Kjaersgaard, J. (2011). Estimation of land surface evapotranspiration with a satellite remote sensing procedure. Great Plains Research, 21(1), 73–88. http://www.jstor.org/stable/23779969
- Jaafar, H., Mourad, R., & Schull, M. (2022). A global 30-m ET model (HSEB) using harmonized Landsat and Sentinel-2, MODIS and VIIRS: Comparison to ECOSTRESS ET and LST. Remote Sensing of Environment, 274, 112995. https://doi.org/10.1016/j.rse.2022.112995
- Jamshidi, S., Zand-Parsa, S., & Niyogi, D. (2021). Assessing crop water stress index of citrus using in-situ measurements, Landsat, and Sentinel-2 data. International Journal of Remote Sensing, 42(5), 1893–1916. https://doi.org/10.1080/01431161.2020.1846224
- Jamshidi, S., Zand-Parsa, S., Pakparvar, M., & Niyogi, D. (2019). Evaluation of evapotranspiration over a semiarid region using multiresolution data sources. Journal of Hydrometeorology, 20(5), 947–964. https://doi.org/10.1175/JHM-D-18-0082.1
- Johnson, L. F., & Trout, T. J. (2012). Satellite NDVI assisted monitoring of vegetable crop evapotranspiration in California’s San Joaquin Valley. Remote Sensing, 4(2), 439–455. https://doi.org/10.3390/rs4020439
- Laipelt, L., Henrique Bloedow Kayser, R., Santos Fleischmann, A., Ruhoff, A., Bastiaanssen, W., Erickson, T. A., & Melton, F. (2021). Long-term monitoring of evapotranspiration using the SEBAL algorithm and Google Earth Engine cloud computing. ISPRS Journal of Photogrammetry and Remote Sensing, 178, 81–96. https://doi.org/10.1016/j.isprsjprs.2021.05.018
- Lipper, L., Thornton, P., Campbell, B. M., Baedeker, T., Braimoh, A., Bwalya, M., Caron, P., Cattaneo, A., Garrity, D., Henry, K., Hottle, R., Jackson, L., Jarvis, A., Kossam, F., Mann, W., McCarthy, N., Meybeck, A., Neufeldt, H. … Tibu, A. (2014). Climate-smart agriculture for food security. Nature Climate Change, 4(12), 1068–1072. https://doi.org/10.1038/NCLIMATE2437
- Melton, F. S., Huntington, J., Grimm, R., Herring, J., Hall, M., Rollison, D., Erickson, T., Allen, R., Anderson, M., Fisher, J. B. et al. (2021). Openet: Filling a critical data gap in water management for the western united states, JAWRA Journal of the American Water Resources Association. https://doi.org/10.1111/1752-1688.12956
- Meza, I., Eyshi Rezaei, E., Siebert, S., Ghazaryan, G., Nouri, H., Dubovyk, O., Gerdener, H., Herbert, C., Kusche, J., Popat, E., Rhyner, J., Jordaan, A., Walz, Y., & Hagenlocher, M. (2021). Drought risk for agricultural systems in South Africa: Drivers, spatial patterns, and implications for drought risk management. The Science of the Total Environment, 799, 149505. https://doi.org/10.1016/j.scitotenv.2021.149505
- Mhawej, M., & Faour, G. (2020). Open-source Google Earth Engine 30-m evapotranspiration rates retrieval: The SEBALIGEE system. Environmental Modelling & Software, 133, 104845. https://doi.org/10.1016/j.envsoft.2020.104845
- Mueller, B., Seneviratne, S. I., Jimenez, C., Corti, T., Hirschi, M., Balsamo, G., Ciais, P., Dirmeyer, P., Fisher, J. B., Guo, Z., Jung, M., Maignan, F., McCabe, M. F., Reichle, R., Reichstein, M., Rodell, M., Sheffield, J., Teuling, A. J. … Wood, E. F. (2011). Evaluation of global observations-based evapotranspiration datasets and IPCC AR4 simulations. Geophysical Research Letters, 38(6), n/a–n/a. https://doi.org/10.1029/2010GL046230
- Naghdyzadegan Jahromi, M., Zand-Parsa, S., Razzaghi, F., Jamshidi, S., Didari, S., Doosthosseini, A., & Pourghasemi, H. R. (2023). Developing machine learning models for wheat yield prediction using ground-based data, satellite-based actual evapotranspiration and vegetation indices. The European Journal of Agronomy, 146, 126820. https://doi.org/10.1016/j.eja.2023.126820
- Nagler, P., Glenn, E., Nguyen, U., Scott, R., & Doody, T. (2013). Estimating riparian and agricultural actual evapotranspiration by reference evapotranspiration and MODIS enhanced vegetation index. Remote Sensing, 5(8), 3849–3871. https://doi.org/10.3390/rs5083849
- Nagler, P., Morino, K., Murray, R. S., Osterberg, J., & Glenn, E. (2009). An empirical algorithm for estimating agricultural and riparian evapotranspiration using MODIS enhanced vegetation index and ground measurements of ET. I. Description of method. Remote Sensing, 1(4), 1273–1297. https://doi.org/10.3390/rs1041273
- Nhamo, L., Ebrahim, G. Y., Mabhaudhi, T., Mpandeli, S., Magombeyi, M., Chitakira, M., Magidi, J., & Sibanda, M. (2020). An assessment of groundwater use in irrigated agriculture using multi-spectral remote sensing. Physics and Chemistry of the Earth, Parts A/B/C, 115, 102810. https://doi.org/10.1016/j.pce.2019.102810
- Niyogi, D., Jamshidi, S., Smith, D., & Kellner, O. (2020). Evapotranspiration climatology of Indiana using in situ and remotely sensed products. Journal of Applied Meteorology and Climatology, 59(12), 2093–2111. https://doi.org/10.1175/JAMC-D-20-0024.1
- Nouri, H., Nagler, P., Chavoshi Borujeni, S., Barreto Munez, A., Alaghmand, S., Noori, B., Galindo, A., & Didan, K. (2020). Effect of spatial resolution of satellite images on estimating the greenness and evapotranspiration of urban green spaces. Hydrological Processes, 34(15), 3183–3199. https://doi.org/10.1002/hyp.13790
- Pan, S., Pan, N., Tian, H., Friedlingstein, P., Sitch, S., Shi, H., Arora, V. K., Haverd, V., Jain, A. K., Kato, E., Lienert, S., Lombardozzi, D., Nabel, J. E. M. S., Ottlé, C., Poulter, B., Zaehle, S., & Running, S. W. (2020). Evaluation of global terrestrial evapotranspiration using state-of-the-art approaches in remote sensing, machine learning and land surface modeling. Hydrology and Earth System Sciences, 24(3), 1485–1509. https://doi.org/10.5194/hess-24-1485-2020
- Pastorello, G., Trotta, C., Canfora, E., Chu, H., Christianson, D., Cheah, Y.-W., Poindexter, C., Chen, J., Elbashandy, A., Humphrey, M., Isaac, P., Polidori, D., Reichstein, M., Ribeca, A., van Ingen, C., Vuichard, N., Zhang, L., Amiro, B. … Torn, M. (2020). The FLUXNET2015 dataset and the ONEFlux processing pipeline for eddy covariance data. Scientific Data, 7(1), 225. https://doi.org/10.1038/s41597-020-0534-3
- Peña-Arancibia, J. L., Mainuddin, M., Kirby, J. M., Chiew, F. H. S., McVicar, T. R., & Vaze, J. (2016). Assessing irrigated agriculture’s surface water and groundwater consumption by combining satellite remote sensing and hydrologic modelling. The Science of the Total Environment, 542(Pt A), 372–382. https://doi.org/10.1016/j.scitotenv.2015.10.086
- Peña-Arancibia, J. L., McVicar, T. R., Paydar, Z., Li, L., Guerschman, J. P., Donohue, R. J., Dutta, D., Podger, G. M., van Dijk, A. I., & Chiew, F. H. (2014). Dynamic identification of summer cropping irrigated areas in a large basin experiencing extreme climatic variability. Remote Sensing of Environment, 154, 139–152. https://doi.org/10.1016/j.rse.2014.08.016
- Pereira, L. S., Paredes, P., Hunsaker, D. J., López-Urrea, R., & Mohammadi Shad, Z. (2021). Standard single and basal crop coefficients for field crops. Updates and advances to the FAO56 crop water requirements method. Agricultural Water Management, 243, 106466. https://doi.org/10.1016/j.agwat.2020.106466
- Pereira, L. S., Paredes, P., Melton, F., Johnson, L., Wang, T., López-Urrea, R., Cancela, J. J., & Allen, R. G. (2020). Prediction of crop coefficients from fraction of ground cover and height. Background and validation using ground and remote sensing data. Agricultural Water Management, 241, 106197. https://doi.org/10.1016/j.agwat.2020.106197
- Poudel, U., Stephen, H., & Ahmad, S. (2021). Evaluating irrigation performance and water productivity using EEFlux ET and NDVI. Sustainability, 13(14), 7967. https://doi.org/10.3390/su13147967
- QGIS. (2021). QGIS geographic information system. QGIS Association. http://www.qgis.org
- Ramón-Reinozo, M., Ballari, D., Cabrera, J. J., Crespo, P., & Carrillo-Rojas, G. (2019). Altitudinal and temporal evapotranspiration dynamics via remote sensing and vegetation index-based modelling over a scarce-monitored, high-altitudinal Andean páramo ecosystem of Southern Ecuador. Environmental Earth Sciences, 78(11). https://doi.org/10.1007/s12665-019-8337-6
- Reyes-González, A., Kjaersgaard, J., Trooien, T., Hay, C., & Ahiablame, L. (2018). Estimation of crop evapotranspiration using satellite remote sensing-based vegetation index. Advances in Meteorology, 2018, 1–12. https://doi.org/10.1155/2018/4525021
- Roy, T., Gupta, H. V., Serrat-Capdevila, A., & Valdes, J. B. (2017). Using satellite-based evapotranspiration estimates to improve the structure of a simple conceptual rainfall–runoff model. Hydrology and Earth System Sciences, 21(2), 879–896. https://doi.org/10.5194/hess-21-879-2017
- Schauer, M., & Senay, G. B. (2019). Characterizing crop water use dynamics in the Central Valley of California using Landsat-derived evapotranspiration. Remote Sensing, 11(15), 1782. https://doi.org/10.3390/rs11151782
- Senay, G. B., Bohms, S., Singh, R. K., Gowda, P. H., Velpuri, N. M., Alemu, H., & Verdin, J. P. (2013). Operational evapotranspiration mapping using remote sensing and weather datasets: A new parameterization for the sseb approach. JAWRA Journal of the American Water Resources Association, 49(3), 577–591. https://doi.org/10.1111/jawr.12057
- Senay, G. B., Friedrichs, M., Morton, C., Parrish, G. E., Schauer, M., Khand, K., Kagone, S., Boiko, O., & Huntington, J. (2022). Mapping actual evapotranspiration using Landsat for the conterminous United States: Google Earth Engine implementation and assessment of the SSEBop model. Remote Sensing of Environment, 275, 113011. https://doi.org/10.1016/j.rse.2022.113011
- Senay, G. B., Schauer, M., Friedrichs, M., Velpuri, N. M., & Singh, R. K. (2017). Satellite-based water use dynamics using historical Landsat data (1984–2014) in the southwestern United States. Remote Sensing of Environment, 202, 98–112. https://doi.org/10.1016/j.rse.2017.05.005
- Sishodia, R. P., Ray, R. L., & Singh, S. K. (2020). Applications of remote sensing in precision agriculture: A review. Remote Sensing, 12(19), 3136. https://doi.org/10.3390/rs12193136
- The State of the Rockies Project 2011-12 Research Team. 2012. The Colorado River basin: An overview. https://www.coloradocollege.edu/dotAsset/e57e7c73-2983-477b-a05d-de0ba0b87a00.pdf.
- Stevens, L. E., Jenness, J., & Ledbetter, J. D. (2020). Springs and springs-dependent taxa of the Colorado River basin, southwestern North America: Geography, ecology and human impacts. Water, 12(5), 1501. https://doi.org/10.3390/w12051501
- Sullivan, A., White, D. D., & Hanemann, M. (2019). Designing collaborative governance: Insights from the drought contingency planning process for the lower Colorado River basin. Environmental Science & Policy, 91, 39–49. https://doi.org/10.1016/j.envsci.2018.10.011
- Wanniarachchi, S., & Sarukkalige, R. (2022). A review on evapotranspiration estimation in agricultural water management: Past, present, and future. Hydrology, 9(7), 123. https://doi.org/10.3390/hydrology9070123
- Zare, M., Pakparvar, M., Jamshidi, S., Bazrafshan, O., & Ghahari, G. (2021). Optimizing the runoff estimation with HEC-HMS model using spatial evapotranspiration by the SEBS model. Water Resources Management, 35(8), 2633–2648. https://doi.org/10.1007/s11269-021-02855-x
Appendices