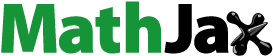
ABSTRACT
Many freely available Digital Elevation Models (DEM) have increasingly been used worldwide due to the difficulty in acquiring accurate elevation data in some regions, emphasizing the need to investigate their accuracy and the factors that may influence their uncertainties. We performed an accuracy analysis of the Topodata DEM in the hydrographic region of Uruguay (Brazil) assuming that its vertical accuracy may be related to terrain characteristics. Multiscale Geographically Weighted Regression (MGWR) was applied to investigate the spatial scales over which terrain characteristics affect local variations in altimetric errors. MGWR outperformed Ordinary Least Squares (OLS) and Geographically Weighted Regression (GWR). MGWR results also showed that aspect, curvature, and artificial areas operate at much smaller scales than elevation and have a higher influence in areas with high positive altimetric errors. The model explains about 41% of the total variation of the altimetric error of the Topodata DEM in the study area. Our findings enrich the understanding of the global and local processes affecting the accuracy of the Topodata DEM and shed light on the importance of local terrain characteristics in effective DEM product development.
HIGHLIGHTS
DEM products provide fundamental information for several research areas.
OLS, GWR and MGWR were applied to identify the factors explaining the altimetric error of a DEM.
MGWR investigated the spatial scales over which terrain characteristics affect local variations in altimetric errors.
MGWR outperformed OLS and GWR proving that terrain characteristics operate at different scales.
Introduction
Products extracted from Digital Elevation Models (DEM) are an important data source on the physical characteristics of the terrestrial surface since these data are the primary means of visualizing terrain texture and relief classification that should be observed in essential research topics (Xu et al., Citation2021). DEM products provide fundamental information for several approaches, such as precipitation estimation (Chen et al., Citation2021; Taheri et al., Citation2020; Xu et al., Citation2015), landslide susceptibility (Bui et al., Citation2016, Citation2020; Chen & Chen, Citation2021; Merghadi et al., Citation2020; Saleem et al., Citation2019), flood prediction (Sarma et al., Citation2020; Suliman et al., Citation2021; Vignesh et al., Citation2021) and geomorphometric analysis (Abdelkarim et al., Citation2020; Gorini & Mota, Citation2016; Lindsay, Citation2016; Pipaud et al., Citation2015; Sánchez-Guillamón et al., Citation2018). Furthermore, they are extremely important in risk assessment of natural disasters (Saleem et al., Citation2019).
Although traditional methods to generate DEM are expensive and time-consuming (Uysal et al., Citation2015), Earth’s surface analysis is becoming increasingly viable due to the rising availability of DEM products at different spatial resolutions (Drăguţ & Eisank, Citation2011). Moreover, due to the lack of more accurate three-dimensional data for geographic studies at a regional scale, freely available DEM are frequently used in several scientific applications which evidences the need to investigate the accuracy of DEM products (Liu et al., Citation2020). Approaches regarding the assessment of DEM quality are usually based on altimetric discrepancies between the DEM and the reference data, which must present reasonable density and be well-distributed in the study area (Polidori & El Hage, Citation2020). In addition, it is essential that these reference data have greater accuracy than the evaluated DEM to ensure a relevant statistical analysis as well as a spatial error analysis (Polidori & El Hage, Citation2020).
In recent decades, many technological advances in the creation and in the process of making available DEM products have been made, despite there are not enough specific guidelines yet regarding the assessment of the accuracy nor a perspective of suitability for the use of these products (Mesa-Mingorance & Ariza-López, Citation2020). In this sense, scale effects modelling has been considered an important research topic since there are not many studies dealing with the potential influence that such effects may have on some factors’ modelling related to hydrology, soil science and geomorphology (Chang et al., Citation2019; Drăguţ & Eisank, Citation2011). Moreover, mathematical modelling of the predicted DEM error as a function of the landscape characteristics is also a promising research path (Polidori & El Hage, Citation2020).
Many studies have addressed the vertical accuracy of DEM (Hawker et al., Citation2019; Hirano et al., Citation2003; Hladik & Alber, Citation2012; Wessel et al., Citation2018; Weydahl et al., Citation2007) through external and internal quality assessment, i.e. with or without reference data, respectively (Polidori & El Hage, Citation2020). The vertical accuracy is often assessed through the comparison of the elevation of the DEM product with a reference elevation collected from a greater accuracy source where parameters such as mean, standard deviation and root mean square error (RMSE) are obtained to analyse the elevation discrepancies (Mesa-Mingorance & Ariza-López, Citation2020; Wise, Citation2000).
The most common parameter to assess the vertical accuracy of a DEM is the RMSE (Mesa-Mingorance & Ariza-López, Citation2020), but it is also possible to use information entropy (Wise, Citation2012) as well as geomorphometric analyses to measure the quality of a DEM (Pipaud et al., Citation2015; Szypuła, Citation2019; Temme et al., Citation2009). Furthermore, some studies have addressed spatial statistics techniques such as Ordinary Least Squares (OLS) and Geographically Weighted Regression (GWR) to model errors in DEM (Carlisle, Citation2005; DeWitt et al., Citation2015; Erdoğan, Citation2010; Gallay et al., Citation2010).
OLS is a technique used to estimate the parameters of multiple linear regression models, which is based on the principle of minimizing the sum of squared differences between the observed dependent variable values and the predicted values (Brunsdon et al., Citation1996; Hutcheson & Hutcheson, Citation2011). OLS assumes that the relationship between variables is constant across the entire study area, disregarding any spatial variations. On the other hand, GWR (Brunsdon et al., Citation1996; Fotheringham et al., Citation2002) and MGWR – Multiscale Geographically Weighted Regression (Fotheringham et al., Citation2017) are both spatial regression techniques that explicitly account for spatial heterogeneity by estimating relationships locally, allowing for spatially varying coefficients. GWR operates at a local scale, where the relationships between variables are estimated for each individual location within the study area, while MGWR simultaneously estimates the relationships at different scales. This allows MGWR to capture variations in relationships at local, regional, and global scales. Thereby, MGWR can model the relationship between the dependent and the independent variables considering the geographic scale at which processes occur, allowing it to differentiate spatial homogeneous and heterogeneous relationships that may influence the dependent variable at different locations (Fotheringham et al., Citation2017). Hence, it is possible to identify if an explanatory factor of the altimetric error operates locally, regionally, or globally in the study area. However, there is still no evidence of studies that have modelled the pattern of the altimetric error through MGWR.
Our study performs a vertical accuracy analysis using MGWR, aiming to identify the local factors that may explain the altimetric error of the Topodata DEM in the hydrographic region of Uruguay (Brazil), accounting for possible different spatial scales in the relationship between such local factors and the altimetric error. Accordingly, this research study aims to investigate not only if the vertical accuracy of DEM products is related to local terrain characteristics but also if there are spatial variations in the magnitude of those relationships. Additionally, this study aims to determine if the relationships between the terrain characteristics and the altimetric error operate at different spatial scales. Besides MGWR, two additional models were used for comparison purposes, namely OLS and GWR.
Our findings are expected to provide a better understanding of the global and local processes influencing the quality of Topodata products and highlight the importance of terrain characteristics in effective DEM product development, besides shedding light on some limitations of regression modelling applications. Providing further understanding of the features influencing DEM vertical accuracy may also contribute to improving the applications that rely on the altimetric data extracted from DEM.
Materials
Study area
The Uruguay River watershed is approximately 385,000 km2 being 174,412 km2 of this area placed in the southern part of Brazil, covering 2% of the national territory, which is named in the hydrographic region of Uruguay (Brazil, Citation2006). The study area’s altimetry ranges from 32 to 1822 meters above sea level ().
Data and preprocessing
SRTMGL1v003 DEM and Topodata DEM
Shuttle Radar Topography Mission (SRTM) was released in 2000 onboard the Space Shuttle Endeavour aiming to generate a near-global DEM of the Earth through radar interferometry (NASA, Citation2013). Initially, SRTM data was made available with a resolution of 1 arc-second for the United States territory and with 3 arc-seconds for other regions of the world. In 2015, the data with full resolution (1 arc-second) was released globally (NASA, Citation2022). In this context, the Topodata project was developed by the Brazilian National Institute for Space Research (Instituto Nacional de Pesquisas Espaciais - INPE) to refine, through kriging techniques, SRTM data from the resolution of 3 arc-seconds (≈90 meters) into 1 arc-second (≈30 meters) over the Brazilian territory (Valeriano & Rossetti, Citation2012). This project also derived geomorphometric data from SRTM products providing information, such as slope, aspect, and curvatures, ready for use by the scientific community (Valeriano, Citation2008; Valeriano & Rossetti, Citation2008). Despite there being other DEM with better resolution, SRTM-90 (90-meter pixel) and Topodata are highly applied in research over the Brazilian territory (Silva et al., Citation2022). Moreover, Topodata DEM is still widely used due to the unavailability of cartographic products in suitable scales for some Brazilian regions (Ferreira & Cabral, Citation2022).
Topodata DEM was released in 2008 and had been revised regularly by INPE (INPE, Citation2008). SRTM datasets most recent version (SRTMGL1v003) eliminated voids that were present in previous versions of SRTM products by using data from ASTER Global Digital Elevation Model (GDEM) Version 2.0, the Global Multi-resolution Terrain Elevation Data 2010 (GMTED2010), and the National Elevation Dataset (NED) (NASA, Citation2013).
Brazilian Geodetic Network
The accuracy analysis in this study is based on official data from the Brazilian Geodetic System (IBGE, Citation2022a). The Brazilian Geodetic Network (BGN) contains geodesic stations implemented throughout the national territory with essential planimetric, altimetric and gravimetric information used as a reference in positioning activities as well as for correction and verification of Brazilian territory images (IBGE, Citation2019, Citation2022a). Most stations are materialized through concrete landmarks with a metal plate on the top, identifying their coordinates, altitudes and gravity obtained by using high-precision geodetic procedures and models (IBGE, Citation2022a). A total of 1068 reference points from the BGN are present in the hydrographic region of Uruguay () georeferenced to the Geocentric Reference System for the Americas (SIRGAS2000 - horizontal datum) and Imbituba (vertical datum). It is important to mention that the Imbituba datum is defined by the calculated middle level of the sea with data from a tide gauge station and then propagating it throughout the Brazilian territory by high-precision geometric levelling (IBGE, Citation2019). The main characteristics of BGN, SRTM and Topodata DEM are shown in .
Table 1. Main characteristics of BGN (IBGE, Citation2019), SRTM (NASA, Citation2013) and Topodata DEM (Garofalo & Liesenberg, Citation2015; Miceli et al., Citation2011; Valeriano, Citation2008).
Independent variables
Aiming to investigate the factors that may explain the altimetric error of Topodata DEM in the hydrographic region of Uruguay, the following candidate explanatory variables were chosen according to previous studies: altitude/elevation (Das et al., Citation2016; Gonzalez-Moradas & Viveen, Citation2020; Szypuła, Citation2019), aspect (Dong & Shortridge, Citation2019; Gorokhovich & Voustianiouk, Citation2006; Leon et al., Citation2014; Sharma et al., Citation2021; Szypuła, Citation2019), curvature (Leon et al., Citation2014; Sharma et al., Citation2021; Szypuła, Citation2019), distance to rivers (Yap et al., Citation2019), drainage density (Das et al., Citation2016), land use/land cover (LULC) (Dong & Shortridge, Citation2019; Leon et al., Citation2014; Satgé et al., Citation2015; Yap et al., Citation2019), roughness (Habib, Citation2021; Li et al., Citation2020; Schwendel & Milan, Citation2020), relative relief (Das et al., Citation2016), slope (Das et al., Citation2016; Dong & Shortridge, Citation2019; Gonzalez-Moradas & Viveen, Citation2020; Gorokhovich & Voustianiouk, Citation2006; Habib, Citation2021; Leon et al., Citation2014; Li et al., Citation2020; Satgé et al., Citation2015; Sharma et al., Citation2021; Szypuła, Citation2019; Yap et al., Citation2019), terrain ruggedness index (TRI) (Dong & Shortridge, Citation2019; Leon et al., Citation2014), topographic position index (TPI) (Dong & Shortridge, Citation2019; Leon et al., Citation2014) and vector ruggedness measure (VRM) (Leon et al., Citation2014; Pipaud et al., Citation2015).
The variables aspect, curvature, elevation, relative relief, roughness, slope, TPI, TRI and VRM were derived from the SRTM DEM (NASA, Citation2013) to ensure that their values are independent of the altimetric error derived from the Topodata DEM. If we used the same DEM to derive the explanatory variables, then their values would be a function of the values of the dependent variable. Hence, the regression model would not be correctly specified (i.e. it would be an inappropriate model). On the other hand, distance to rivers and drainage density were derived from the drainage data of the National Water and Sanitation Agency (Agência Nacional de Águas e Saneamento Básico – ANA) (ANA, Citation2022).
Aspect measures the orientation of the slope for each location on which the compass direction ranges from 0° to 360°Clockwise, where 0°, 90°, 180° and 270°Correspond to north, east, south, and west, respectively (Kaliraj et al., Citation2015; Lei et al., Citation2022). Curvature refers to a morphological measure of the terrain topography, where positive values mean that the surface is upwardly convex, negative values reveal an upwardly concave whereas zero values indicate that the surface is flat (Lee & Sambath, Citation2006).
The LULC mapping was performed by the Brazilian Institute of Geography and Statistics (Instituto Brasileiro de Geografia e Estatística – IBGE) based on images from the Moderate-Resolution Imaging Spectroradiometer (MODIS) sensor and from LANDSAT-5 and LANDSAT-7 satellites. In addition, the technical review process also involved the incorporation of polygons from the vegetation maps and auxiliary information from the Continuous Cartographic Base of Brazil at a scale of 1:250,000 (IBGE, Citation2017, Citation2022b). Since SRTM data were collected in year 2000, we use the LULC data from the same year trying to be as faithful as possible to the reality of the terrestrial surface at the mission’s time. The following LULC classes are found in the hydrographic region of Uruguay (IBGE, Citation2017):
Artificial area – characterized by urban use, structured by buildings and road systems, where non-agricultural artificial surfaces predominate.
Agricultural area – characterized by temporary and permanent crops, irrigated or not, with the land used for food production, fibre, and agribusiness commodities. It includes all cultivated land, which may be planted or fallow, and cultivated wetlands. It can be represented by heterogeneous agricultural zones or extensive areas of plantations.
Pasture – area intended for the grazing of cattle and other animals, with cultivated herbaceous vegetation or natural grassland vegetation, both presenting high-intensity anthropic interference.
Mosaic of agriculture and forest remnants – area characterized by the mixed occupation of agriculture, pasture and forestry associated with forest remnants. Other plant formations (herbaceous and shrubby) may occur to a lesser extent.
Forestry – area characterized by forest plantations of exotic and/or native species as monocultures.
Forest vegetation – area occupied by forests. Trees taller than 5 meters are considered forest formations, including areas of dense forest, open forest, and seasonal forest, in addition to the mixed ombrophiles forest.
Grassland – area characterized by natural grassland vegetation subject to grazing and other low-intensity anthropic interference.
Mosaic of anthropic areas and grassland – area characterized by the mixed occupation of agriculture, pasture and/or forestry with remnants of grassland vegetation. Arboreal plant formations may occur to a lesser extent proportion.
Water body - It includes all inland waters such as rivers, streams, canals, and other linear bodies of water. It also encompasses naturally closed bodies of water (natural lakes) and artificial reservoirs (artificial water dams built for irrigation, flood control, water supply and electricity generation). and show the spatial distribution of each LULC class.
Table 2. Spatial distribution of LULC classes in the hydrographic region of Uruguay.
To include the LULC classes in the regression modelling, it was necessary to consider an area around each reference point from the BGN. For this purpose, we considered the average distance parameter obtained through the “Calculate Distance Band from Neighbour Count” tool (ArcGIS Desktop software – version 10.8.2) which returns the average distance to the N nearest neighbour (Esri, Citation2022). Since this result was 5,438 meters, we defined the buffer radius value as 5,500 meters in this analysis. Then, the independent variables of the regression models were computed as the area of each LULC class inside the buffer at each location. This approach guaranteed that the scale of analysis used to compute the independent variables derived from the LULC classes was the same, regardless of the spatial distribution of the BGN points which are not regularly distributed.
Methods
SRTM and Topodata DEM are referenced to the EGM-96 geoid. So, we had to compute the geoidal undulation through the “Geoid Height Calculator” (UNAVCO, Citation2022) to transform their original geoidal into ellipsoidal altitudes (WGS-84) (Elkhrachy, Citation2018; Orlando, Citation2019). Then, it was necessary to convert these ellipsoidal altitudes into physical altitudes compatible with the Brazilian vertical datum (Imbituba) (Bettiol et al., Citation2021; Rodrigues et al., Citation2011) by using the hgeoHNOR2020 model (IBGE, Citation2019). We also had to convert the SRTM and Topodata horizontal data from WGS-84 into the Brazilian official horizontal datum (SIRGAS2000) through ArcGIS Desktop software – version 10.8.2.
To derive the altimetric errors, we followed the methodology applied by Satge et al. (Citation2016) and Shean et al. (Citation2016). Negative altimetric errors mean that the DEM analysed overestimates the elevation and positive errors underestimate the elevation at each verified point (Brasington et al., Citation2003; Holmes et al., Citation2000).
Terrain analysis was carried out by generating maps of the following input candidate explanatory variables: aspect, curvature, distance to rivers, drainage density, roughness, relative relief, slope, TPI, TRI and VRM. Then, statistical analysis was made through the accuracy indicators calculation (Moura et al., Citation2014; Satge et al., Citation2016; Shean et al., Citation2016), namely the altimetric error (AE) (1), mean error (ME) (2), mean absolute error (MAE) (3), and root mean square error (RMSE) (4).
where AREF is the altitude of the reference point from the BGN, ADEM is the altitude extracted from Topodata DEM, and n is the number of reference points.
The MAE is a statistical measure that computes the average absolute difference between the value considered the true (BGN) and the value extracted from the Topodata DEM. The RMSE measures the uncertainty in the computed values, defining the degree of correspondence between the reference values and those extracted from the DEM. Thus, lower MAE and RMSE values indicate better results (Rawat et al., Citation2019).
We performed the Exploratory Spatial Data Analysis (ESDA) through Voronoi tessellation, Hot Spot Analysis (Getis & Ord, Citation1992), and Local and Global Moran’s I statistics (Anselin, Citation1995; Moran, Citation1950). Finally, the altimetric error modelling was performed using OLS, GWR (Brunsdon et al., Citation1996, Citation1996; Fotheringham et al., Citation2002, Citation2002) and MGWR (Fotheringham et al., Citation2017, Citation2017) on the 1068 reference points from the BGN (). A 5% significance level was considered in all statistical tests, otherwise stated.
Voronoi tessellation is a concept proposed by Georgy Voronoi in 1907 based on a computational geometry data structure that has been applied in many scientific areas (Kastrisios & Tsoulos, Citation2018). Voronoi maps or Voronoi diagrams are built from polygons generated around a sample point. Each Voronoi polygon is obtained by intersecting perpendicular bisectors of adjacent points, where the nearest neighbour of any point inside the polygon is the sample point (i.e. the generator of the polygon) (Nene & Nayar, Citation1997; Safar, Citation2005).
Hot spot analysis is a spatial analysis technique used to identify statistically significant clusters of high (hot spots) or low values (cold spots) within a dataset using the Getis-Ord Gi* statistic (Getis & Ord, Citation1992).
The Global Moran’s I is an inferential statistic used to measure the spatial autocorrelation in a dataset and to test whether the observed spatial pattern in the dataset is randomly distributed (null hypothesis) or spatially autocorrelated (alternative hypothesis). A significant positive index reveals evidence of spatial clustering of similar values, and a negative one provides evidence of a dispersion pattern of dissimilar values (Moran, Citation1950; Prasannakumar et al., Citation2011). The Local Moran’s I examines the significance of local spatial autocorrelation by calculating the Moran’s I statistic for each location using neighbouring values to identify local clusters or spatial outliers (Anselin, Citation1995). Clusters with high or low values are defined as high-high (HH) or low-low (LL), respectively, and correspond to statistically significant positive spatial autocorrelation. A high-low (HL) outlier corresponds to a high value correlated with surrounding low values, and a low value correlated with surrounding high values is defined as a low-high (LH) outlier. Spatial outliers correspond to statistically significant negative spatial autocorrelation (Anselin et al., Citation2007).
OLS, GWR and MGWR are linear regression models, but each one operates at different spatial scales and make different assumptions regarding the spatial heterogeneity of the data set. OLS is a global model assuming a single coefficient to explain the relationship between each explanatory and the altimetric error in the whole study area, whereas GWR and MGWR are local regression models that allow the coefficients to vary in space. Moreover, MGWR also allows each independent variable to adopt a different spatial scale of analysis.
ArcGIS Desktop software (version 10.8.2) was used to run the ESDA and OLS analysis. We investigated all possible combinations of the 22 independent variables in 539,909 exploratory OLS models considering their statistical significance, the variance inflation factors (VIF), and the models’ Adjusted R2. The OLS model which has presented the highest Adjusted R2, without multicollinearity issues, included four significant variables: aspect (), curvature (
), elevation (
) and LULC_1 (artificial area) (
). These variables were then included in the GWR and MGWR models that were estimated using the MGWR 2.2 software (Oshan et al., Citation2019).
All variables in the MGWR model were standardized to increase the interpretability of the bandwidths of the spatial kernel. An adaptive bisquare kernel was applied in both GWR and MGWR models as the distance-weighting function to control the optimal number of nearest neighbours to be included in the local model fitting (5) (Fotheringham et al., Citation2017):
where is the weight between points i and j,
is the Euclidean distance between points i and j, and the bandwidth
is the distance from focal point i to its
nearest neighbor. The optimal number of neighbors (M) is determined by the lowest corrected Akaike’s Information Criterion (AICc) that is obtained from multiple comparisons. The MGWR model is formulated in Equation 6.
where denotes the specific optimal bandwidth used in the calibration of the intercept and
conditional relationship (
), and
are location coordinates for each focal point i (
). Model diagnostics and inference-related diagnostics are computed for local parameter estimates (Fotheringham et al., Citation2019; Yu et al., Citation2020).
In the GWR model, the notation was removed because the optimal bandwidth used is the same for the intercept and all
conditional relationships. That notation was also removed from the OLS model, as well as the
location coordinates, because the OLS (global) model is specified using a single regression equation: the
parameters (
) are the same for all locations and they are estimated using the whole data set. For further details see Fotheringham et al. (Citation2002).
Results
Statistical analysis
The altimetric errors of the Topodata DEM range from − 36.68 to 39.23 meters, and 75% of them are smaller than 1.22 meters (). There is very a strong correlation between the elevations extracted from SRTM and Topodata DEM when they are compared with the reference points from the BGN as the coefficient of determination (R2) of a simple linear regression is higher than 0.99 in both cases (Appendix A1/A2).
Table 3. Statistical accuracy indicators of the Topodata DEM.
The histogram indicates that the altimetric errors are negatively biased (overestimated) which is reinforced by the median value already shown in . Despite that, they present a distribution a little close to a normal curve (Appendix A3).
The boxplots of the elevation differences are shown in Appendix A4, where it is possible to notice that the SRTM and Topodata elevation are very similar to the BGN altitudes and that more than 50% of the altitudes are below 500 meters, an expected fact since Brazil is considered a low-lying country being 41% of its territory below 200 meters and barely 7% above 800 meters (Alvares et al., Citation2013). Even though it can be observed in Appendix A4 that almost 25% of the point altitudes are above 800 meters.
The altimetric errors are higher in areas with slopes above 30% (Appendix B1). Furthermore, we observe that the MAE and the RMSE increase as the slope also increases. Likewise, the altimetric errors are higher in places where the elevation is higher than 1,200 meters (Appendix B2), despite the altimetric errors being also higher in regions where elevation is between 900 and 1,200 meters when compared with places lower than 900 meters. Regarding the LULC, the classes that presented the highest altimetric errors were “forest vegetation” and “artificial area” (Appendix B3). The Pearson’s correlation coefficient between elevation and altimetric error is approximately 0.22. The correlation matrix of all the candidate explanatory variables (Appendix C) shows that some of them are significantly correlated with each other, such as relative relief, VRM, TPI.
Spatial effects in altimetric errors
The Voronoi map of the altimetric error shows that most samples (82%) present altimetric errors between − 6.46 and 6.46 meters and that they are evenly distributed (). There is no apparent trend of the altimetric error over the study area.
The Global Moran’s I statistic revealed that the altimetric error did not have a significant spatial autocorrelation (index ≈0.02; p-value = 0.98), thus the altimetric error pattern does not appear to be significantly different from random.
Anselin’s Local Moran’s I, represented in , shows that 23 of the 1,068 points (≈2%) have significant positive (15 high-high and 3 low-low clusters) and negative (3 low-high and 2 high-low outliers) spatial autocorrelation. The high-high clusters (high altimetric errors surrounded by high altimetric errors) are in the upper watershed course, and 2 of the 3 low-low clusters (low altimetric errors surrounded by low altimetric errors) are in the middle watershed course. also shows that 3 low-high outliers (low altimetric errors surrounded by high altimetric errors) are in the upper watershed course whereas there is 1 high-low outlier in the upper watershed course and 1 in the middle watershed course.
Hot Spot Analysis (Getis – Ord Gi* statistic) reveals both high altimetric errors (hot spot) and low altimetric errors (cold spot) values clustered spatially (). In this analysis, results demonstrate the existence of 7 hot spots in the upper region, 5 in the middle and lower region of the watershed at the 1% significance level, 6 in the upper and 1 in the middle part of the watershed at the 5% significance level, and 2 in the upper region of the watershed at the 10% significance level. In addition, this analysis also shows cold spots in the upper region (3 points) and 1 in the middle region of the watershed at 1% and 5% significance levels, respectively ().
Models’ performance and diagnostics
OLS results
The summary of OLS results in shows that all variables have a statistically significant coefficient. Furthermore, the low VIF values indicate that there is no evidence of redundancy among the explanatory variables.
Table 4. Parameter estimates for the OLS model.
GWR and MGWR results
Results of the MGWR model executed with the same variables as the OLS and GWR models show an improvement of the proposed model since it had a higher Adjusted R2 (0.41) and a lower AICc (2,602.14) than the OLS and GWR models (). It was also noticed a decrease in the residual sum of squares (RSS).
Table 5. Metrics of OLS, GWR and MGWR models.
Given the spatially varying nature of MGWR, the map of the Local R2 () highlights the areas where the local regressions have a better goodness-of-fit and provides insights on the locations where important variables may be missing. The Local R2 ranges from 0.18 to 0.74 (). Low values of the Local R2 in the flatter regions located in the middle of the study domain indicate that other factors may affect the altimetric error besides aspect and elevation, which have significant coefficients in that region (). The Local R2 was higher than 0.5 in approximately 25% of the local regressions obtained with the MGWR model (). These areas, where the Local R2 ranges from 0.50 to 0.74, overlap with the areas where the curvature presents significant local coefficients (). Therefore, the inclusion of curvature contributed to improving the goodness-of-fit of the MGWR model in the northern and southern parts of the study domain.
Figure 6. Voronoi map of the spatial distribution of MGWR local coefficients: (a) aspect, (b) curvature, (c) elevation, (d) LULC (class 1 – artificial area).

Table 6. Frequency distribution of local R2 values of the MGWR model.
There is no evidence of spatial autocorrelation in the residuals of the MGWR model over the study area because the value of the Global Moran’s I statistic was equal to − 0.01, and it was not statistically different from zero (z-score = −0.01; p-value = 0.99). Hence, the spatial pattern of the residuals does not appear to be significantly different from random.
A Local Condition Number (Local_CN) greater than 30 indicates that there might be a multicollinearity problem in the model (Oshan et al., Citation2019). In this way, there is also no evidence of multicollinearity among the independent variables because the Local_CNs range from 1.14 to 2.67 ().
Spatial pattern analysis of the coefficients
The optimal bandwidths of the coefficients obtained from GWR and MGWR models are shown in . The GWR model presented a very restrictive bandwidth (173) compared with the bandwidth of the MGWR model since it is approximately half of the average bandwidth of the MGWR model (337). Furthermore, GWR assumes that the aspect, curvature, elevation and LULC_1 (artificial area) influence the altimetric error on the same scale, which is refuted by MGWR model results. The MGWR bandwidth of the curvature variable with 52 nearest neighbours indicates that this variable operates on a local scale. The MGWR bandwidths of the LULC_1 (artificial area) and aspect variables show that these variables operate on a regional scale. The MGWR bandwidth of the elevation variable reveals that this variable influences the altimetric error on a global scale because its bandwidth is exactly the possible maximum number of neighbours (1067).
Table 7. Summary of local regression results.
The MGWR bandwidth and the standard deviation parameter estimates of the variables are inversely related because a large bandwidth of a variable influences the dependent variable on a large scale which means small heterogeneity and, consequently, a small standard deviation of parameter estimates (Fotheringham et al., Citation2019). Likewise, a short bandwidth influences the dependent variable on a local scale where the standard deviation of the local parameter is large ().
MGWR local coefficients of each explanatory variable are shown in , where blank areas indicate that the coefficients are not significantly different from zero. Hence, the explanatory variable does not significantly affect the altimetric error in those locations. The significant coefficients of the aspect variable are all negative and appear in the upper and middle course of the hydrographic region of Uruguay (). On the other hand, the non-significant coefficients of this variable are mostly in the lower part of the study area. Therefore, the explanatory variable does not significantly correlate with the altimetric error in those locations. shows the variation of the curvature local parameters where the coefficients are all positive in parts of the upper and lower course of the watershed, considering only regions with significant values. reveals that the elevation variable significantly influences the altimetric error at a global scale and its coefficients are very similar across the whole study area, as they range from 0.42 to 0.45. All significant coefficients of the LULC_1 (artificial area) variable also presented positive values, and they are mainly in the lower course of the watershed ().
The percentage of locations with significant coefficients (p ≤ 0.05 of t-test) of aspect, curvature, elevation, and LULC_1 were 82%, 36%, 100%, and 18%, respectively (Appendix D).
Discussion
Despite covering 2% of Brazil territory, the area of the Brazilian part of the Uruguay River watershed is approximately equal to double the area of some countries such as Azerbaijan (86,600 km2), Hungary (93,030 km2) or Portugal (92,212 km2). Other regions of the Brazilian territory were discarded, and that area was chosen because of the reasonable coverage of points from the BGN, where we could identify 1068 reference points satisfactorily distributed over the study domain. Being the Topodata model exclusively developed for the Brazilian territory, it is expected that the altimetric range of the study area (32 to 1822 meters above sea level) may adequately represent the average of the national territory elevation.
The statistical analysis results demonstrated that the elevation and slope variables affect the accuracy of the Topodata DEM because higher places and steeper areas presented higher altimetric errors. In fact, the highest altimetric error residuals are related to the highest slope classes, and this result was also observed in similar studies (Gdulová et al., Citation2020; González-Moradas & Viveen, Citation2020; Gorokhovich & Voustianiouk, Citation2006; Mukherjee et al., Citation2013; Varga & Bašić, Citation2015). Additionally, altimetric errors are higher on higher-elevation surfaces, which was also found by Mukherjee et al. (Citation2013) and Pandey et al. (Citation2017). Nevertheless, some authors did not find a significant relationship between altimetric error and elevation (González-Moradas & Viveen, Citation2020; Varga & Bašić, Citation2015).
There is evidence of the influence of LULC on altimetric errors of the Topodata DEM since the artificial areas and forest vegetation presented higher altimetric errors when compared with the other classes as shown in the statistical analysis. Previous studies have already verified the LULC effect on altimetric error (Satgé et al., Citation2015; Yap et al., Citation2019), especially in vegetated areas (Dong & Shortridge, Citation2019; Gdulová et al., Citation2020; Leon et al., Citation2014) but such effect was also found in artificial areas (Dong & Shortridge, Citation2019; González-Moradas & Viveen, Citation2020), although smaller altimetric errors have been observed in built and homogeneous environments such as houses, roads and bare land (Leon et al., Citation2014). Despite the statistical analysis having shown some influence of the forest vegetation class on the altimetric error, this variable was not included in the local regression models because it was only significant in 0.02% of all the exploratory OLS investigated models. The best OLS regression model identified in this analysis included aspect, curvature, elevation and LULC_1 (artificial area) as explanatory variables of the altimetric error in the Topodata DEM. Nonetheless, Random Forest Regression (Breiman, Citation2001) could be considered in future studies for the selection of a reduced number of factors from a large set of potential explanatory variables.
The analysis of spatial effects in altimetric errors highlighted the pattern of spatial heterogeneity of the altimetric error. Generally, GWR outperforms global regression models because of its capability to deal with spatial non-stationarity. Though, GWR uses a single optimized bandwidth for all independent variables to define the local neighbourhoods, thus assuming that all relationships vary at the same spatial scale across all covariates (Fotheringham et al., Citation2002, Citation2019). However, we assume that they may operate at different scales. In this sense, the MGWR approach is more appropriate since it computes an optimal bandwidth for each independent variable (Fotheringham et al., Citation2017). In fact, MGWR outperforms GWR because it allows examining the spatial scales in different processes by enabling the optimization of covariate‐specific bandwidths (Fotheringham et al., Citation2019; Yu et al., Citation2020).
GWR and MGWR models were estimated using the same covariates of the best OLS model previously identified. Regression analysis results based on the Adjusted R2 and AICc values proved that the MGWR outperforms the OLS and GWR models as expected. Moreover, our findings allow us to state that GWR may not be suitable for modelling the altimetric errors of a DEM because not all the explanatory variables influence the altimetric error on the same scale. Our results confirm the hypothesis that each explanatory variable operates on a different scale as the curvature variable affects the altimetric error on a local scale, the LULC_1 (artificial area) and aspect on a regional scale, and the elevation influences the dependent variable on a global scale.
MGWR local coefficients of different variables can be directly compared because the dependent and independent variables were standardized. MGWR coefficients analysis showed that high positive altimetric errors are mainly where the aspect and curvature variables coefficients are significant. Most of the highest positive altimetric errors (high-high clusters/hot spots) are placed in regions with the lowest coefficients of the aspect variable, which exhibits a negative relationship with the altimetric error. On the other hand, the curvature coefficients showed a positive relationship. Therefore, as the curvature values increase, so do the altimetric errors. However, it is possible to verify a (low-low) cluster of points with negative altimetric errors overlapping regions with the highest values of the curvature coefficients. Negative altimetric errors (low-low clusters) are mostly in the middle region of the study area, where only aspect and elevation variables have significant coefficients. Furthermore, negative errors occurred almost exclusively where the LULC_1 coefficients are not significant.
One of the limitations of this study is the use of the SRTM DEM to derive some explanatory variables as this product also has accuracy issues (Weydahl et al., Citation2007). Nevertheless, future work should consider the development of algorithms capable of dealing with the error arising from some of the explanatory variables addressed in this study aiming to reduce altimetric discrepancies in the DEM products.
Conclusion
This study performed a vertical accuracy analysis of the Topodata DEM in the hydrographic region of Uruguay assuming the hypothesis that its vertical accuracy would be related to terrain characteristics. The results of the statistical analysis showed that the MAE and RMSE values are sensitive to elevation, slope and some LULC classes, namely forest vegetation and artificial area. We performed a linear regression analysis through OLS, GWR and MGWR models to identify the factors that may explain the spatial patterns in the altimetric error of the Topodata DEM. The MGWR model showed better results than OLS and GWR because it models the relationship between the altimetric error and the factors influencing DEM vertical accuracy considering the geographic scale at which individual process occurs. The results show that different terrain characteristics operate at different scales and their relationships with altimetric error vary in space. The aspect, curvature, and artificial areas variables operate at much smaller scales than elevation which influences the altimetric error on a global scale. This implies that elevation is more relevant throughout the whole study area, whilst the other variables are relevant in certain areas since they operate on local or regional scales.
Our findings proved that different terrain characteristics operate at different scales and their relationships with altimetric error vary in space. In this way, this research provides a better understanding of the global and local processes influencing the quality of Topodata products and highlights the importance of terrain characteristics in effective DEM product development, besides shedding light on some limitations of regression modelling applications.
Disclosure statement
No potential conflict of interest was reported by the author(s).
Data availability statement
The data that support the findings of this study are available from the corresponding author, Z. Ferreira, upon reasonable request.
Additional information
Funding
References
- Abdelkarim, A., Al-Alola, S. S., Alogayell, H. M., Mohamed, S. A., Alkadi, I. I., & Youssef, I. Y. (2020). Mapping of gis-flood hazard using the geomorphometric-hazard model: Case study of the al-shamal train pathway in the city of qurayyat, kingdom of Saudi Arabia. Geosciences (Switzerland), 10(9), 1–20. https://doi.org/10.3390/geosciences10090333
- Alvares, C. A., Stape, J. L., Sentelhas, P. C., & de Moraes Gonçalves, J. L. (2013). Modeling monthly mean air temperature for Brazil. Theoretical and Applied Climatology, 113(3–4), 407–427. https://doi.org/10.1007/s00704-012-0796-6
- ANA. (2022). Agência Nacional de Águas e Saneamento Básico. Base Hidrográfica Ottocodificada da Bacia do Rio Uruguai. Retrieved March 12, 2022, from https://metadados.snirh.gov.br/geonetwork/srv/por/catalog.search#/metadata/3d6a7031-5b18-45ea-ab41-39911975e51a
- Anselin, L. (1995). Local indicators of spatial association—LISA. Geographical Analysis, 27(2), 93–115. https://doi.org/10.1111/j.1538-4632.1995.tb00338.x
- Anselin, L., Sridharan, S., & Gholston, S. (2007). Using exploratory spatial data analysis to leverage social indicator databases: The discovery of interesting patterns. Social Indicators Research, 82(2), 287–309. https://doi.org/10.1007/s11205-006-9034-x
- Bettiol, G. M., Ferreira, M. E., Motta, L. P., Cremon, É. H., & Sano, E. E. (2021). Conformity of the NASADEM_HGT and ALOS AW3D30 dem with the altitude from the Brazilian geodetic reference stations: A case study from Brazilian cerrado. Sensors, 21(9), 2935. https://doi.org/10.3390/s21092935
- Brasington, J., Langham, J., & Rumsby, B. (2003). Methodological sensitivity of morphometric estimates of coarse fluvial sediment transport. Geomorphology, 53(3–4), 299–316. https://doi.org/10.1016/S0169-555X(02)00320-3
- Brazil. (2006). Caderno da Região Hidrográfica do Uruguai. Ministério do Meio Ambiente, Secretaria de Recursos Hídricos. MMA, 2006
- Breiman, L. (2001). Random forests. Machine Learning, 45(1), 5–32. https://doi.org/10.1023/A:1010933404324
- Brunsdon, C., Fotheringham, A. S., & Charlton, M. E. (1996). Geographically weighted regression: A method for exploring spatial nonstationarity. Geographical Analysis, 28(4), 281–298. https://doi.org/10.1111/j.1538-4632.1996.tb00936.x
- Bui, D. T., Tsangaratos, P., Nguyen, V.-T., Liem, N. V., & Trinh, P. T. (2020). Comparing the prediction performance of a Deep learning Neural Network model with conventional machine learning models in landslide susceptibility assessment. Catena, 188, 188. https://doi.org/10.1016/j.catena.2019.104426
- Bui, D. T., Tuan, T. A., Klempe, H., Pradhan, B., & Revhaug, I. (2016). Spatial prediction models for shallow landslide hazards: A comparative assessment of the efficacy of support vector machines, artificial neural networks, kernel logistic regression, and logistic model tree. Landslides, 13(2), 361–378. https://doi.org/10.1007/s10346-015-0557-6
- Carlisle, B. H. (2005). Modelling the spatial distribution of DEM error. Transactions in GIS, 9(4), 521–540. https://doi.org/10.1111/j.1467-9671.2005.00233.x
- Chang, K.-T., Merghadi, A., Yunus, A. P., Pham, B. T., & Dou, J. (2019). Evaluating scale effects of topographic variables in landslide susceptibility models using GIS-based machine learning techniques. Scientific Reports, 9(1), 12296. https://doi.org/10.1038/s41598-019-48773-2
- Chen, X., & Chen, W. (2021). GIS-based landslide susceptibility assessment using optimized hybrid machine learning methods. Catena, 196, 196. https://doi.org/10.1016/j.catena.2020.104833
- Chen, C., Hu, B., & Li, Y. (2021). Easy-to-use spatial random-forest-based downscaling-calibration method for producing precipitation data with high resolution and high accuracy. Hydrology and Earth System Sciences, 25(11), 5667–5682. https://doi.org/10.5194/hess-25-5667-2021
- Das, S., Patel, P. P., & Sengupta, S. (2016). Evaluation of different digital elevation models for analyzing drainage morphometric parameters in a mountainous terrain: A case study of the supin–upper tons basin, Indian Himalayas. SpringerPlus, 5(1), 1–38. https://doi.org/10.1186/s40064-016-3207-0
- DeWitt, J., Warner, T., & Conley, J. (2015). Comparison of DEMS derived from USGS DLG, SRTM, a statewide photogrammetry program, ASTER GDEM and LiDAR: Implications for change detection. GIScience & Remote Sensing, 52(2), 179–197. https://doi.org/10.1080/15481603.2015.1019708
- Dong, Y., & Shortridge, A. M. (2019). A regional ASTER GDEM error model for the Chinese Loess Plateau. International Journal of Remote Sensing, 40(3), 1048–1065. https://doi.org/10.1080/01431161.2018.1524171
- Drăguţ, L., & Eisank, C. (2011). Object representations at multiple scales from digital elevation models. Geomorphology, 129(3–4), 183–189. https://doi.org/10.1016/j.geomorph.2011.03.003
- Elkhrachy, I. (2018). Vertical accuracy assessment for SRTM and ASTER digital elevation models: A case study of Najran city, Saudi Arabia. Ain Shams Engineering Journal, 9(4), 1807–1817. https://doi.org/10.1016/j.asej.2017.01.007
- Erdoğan, S. (2010). Modelling the spatial distribution of DEM error with geographically weighted regression: An experimental study. Computers & Geosciences, 36(1), 34–43. https://doi.org/10.1016/j.cageo.2009.06.005
- Esri. (2022). Geographic Information System Company. Calculate Distance Band from Neighbor Count (Spatial Statistics). https://pro.arcgis.com/en/pro-app/latest/tool-reference/spatial-statistics/calculate-distance-band-from-neighbor-count.htm
- Ferreira, Z. A., & Cabral, P. (2022). A comparative study about vertical accuracy of four freely available digital elevation models: A case study in the Balsas river watershed, Brazil. ISPRS International Journal of Geo-Information, 11(2), 106. https://doi.org/10.3390/ijgi11020106
- Fotheringham, A. S., Brunsdon, C., & Charlton, M. (2002). Geographically weighted regression: The analysis of spatially varying relationships. John Wiley & Sons.
- Fotheringham, A. S., Yang, W., & Kang, W. (2017). Multiscale Geographically weighted regression (MGWR). Annals of the American Association of Geographers, 107(6), 1247–1265. https://doi.org/10.1080/24694452.2017.1352480
- Fotheringham, A. S., Yue, H., & Li, Z. (2019). Examining the influences of air quality in China’s cities using multi-scale geographically weighted regression. Transactions in GIS, 23(6), 1444–1464. https://doi.org/10.1111/tgis.12580
- Gallay, M., Lloyd, C., & McKinley, J. (2010, July, 20-23). Using geographically weighted regression for analysing elevation error of high-resolution DEMs. (Ed.),^(eds.) Proceedings of the Ninth International Accuracy Symposium, Leicester, UK.
- Garofalo, D. F. T., & Liesenberg, V. (2015). Análise comparativa da informação altimétrica presente nos modelos digitais de elevação ASTER GDEM 1 e 2, SRTM e TOPODATA. Anais XVII Simpósio Brasileiro de Sensoriamento Remoto-SBSR. João Pessoa-PB:[sn], 2867–2875.
- Gdulová, K., Marešová, J., & Moudrý, V. (2020). Accuracy assessment of the global TanDEM-X digital elevation model in a mountain environment. Remote Sensing of Environment, 241, 111724. https://doi.org/10.1016/j.rse.2020.111724
- Getis, A., & Ord, J. K. (1992). The analysis of spatial association by the use of distance statistics. Geographical Analysis, 24(2), 189–206. https://doi.org/10.1111/j.1538-4632.1992.tb00261.x
- Gonzalez-Moradas, M. D. R., & Viveen, W. (2020). Evaluation of ASTER GDEM2, SRTMv3. 0, ALOS AW3D30 and TanDEM-X DEMs for the Peruvian Andes against highly accurate GNSS ground control points and geomorphological-hydrological metrics. Remote Sensing of Environment, 237, 111509. https://doi.org/10.1016/j.rse.2019.111509
- González-Moradas, M. D. R., & Viveen, W. (2020). Evaluation of ASTER GDEM2, SRTMv3. 0, ALOS AW3D30 and TanDEM-X DEMs for the Peruvian Andes against highly accurate GNSS ground control points and geomorphological-hydrological metrics. Remote Sensing of Environment, 237, 111509. https://doi.org/10.1016/j.rse.2019.111509
- Gorini, M. A. V., & Mota, G. L. A. (2016). Dealing with double vagueness in DEM morphometric analysis. International Journal of Geographical Information Science, 30(8), 1644–1666. https://doi.org/10.1080/13658816.2016.1150484
- Gorokhovich, Y., & Voustianiouk, A. (2006). Accuracy assessment of the processed SRTM-based elevation data by CGIAR using field data from USA and Thailand and its relation to the terrain characteristics. Remote Sensing of Environment, 104(4), 409–415. https://doi.org/10.1016/j.rse.2006.05.012
- Habib, M. (2021). Evaluation of DEM interpolation techniques for characterizing terrain roughness. Catena, 198, 105072. https://doi.org/10.1016/j.catena.2020.105072
- Hawker, L., Neal, J., & Bates, P. (2019). Accuracy assessment of the TanDEM-X 90 digital elevation model for selected floodplain sites. Remote Sensing of Environment, 232, 111319. https://doi.org/10.1016/j.rse.2019.111319
- Hirano, A., Welch, R., & Lang, H. (2003). Mapping from ASTER stereo image data: DEM validation and accuracy assessment. ISPRS Journal of Photogrammetry and Remote Sensing, 57(5), 356–370. https://doi.org/10.1016/S0924-2716(02)00164-8
- Hladik, C., & Alber, M. (2012). Accuracy assessment and correction of a LIDAR-derived salt marsh digital elevation model. Remote Sensing of Environment, 121, 224–235. https://doi.org/10.1016/j.rse.2012.01.018
- Holmes, K., Chadwick, O., & Kyriakidis, P. C. (2000). Error in a USGS 30-meter digital elevation model and its impact on terrain modeling. Journal of Hydrology, 233(1–4), 154–173. https://doi.org/10.1016/S0022-1694(00)00229-8
- Hutcheson, G. D., & Hutcheson, G. (2011). Ordinary least-squares regression. In L. Moutinho & G. D. Hutcheson (Eds.), The SAGE dictionary of quantitative management research (pp. 225–228). https://doi.org/10.4135/9781446251119.n67
- IBGE. (2017). Instituto Brasileiro de Geografia e Estatística. Monitoramento da cobertura e uso da terra do Brasil 2000 - 2010 - 2012 - 2014. Retrieved June 17, 2023, from https://biblioteca.ibge.gov.br/visualizacao/livros/liv101469.pdf
- IBGE. (2019). Instituto Brasileiro de Geografia e Estatística. Reajustamento da Rede Altimétrica com Números Geopotenciais (2nd ed.). Instituto Brasileiro de Geografia e Estatística - Coordenação de Geodésia. Retrieved July 14, 2020, from. https://biblioteca.ibge.gov.br/visualizacao/livros/liv101666.pdf
- IBGE. (2022a). Instituto Brasileiro de Geografia e Estatística. Banco de dados geodésicos. Retrieved March 19, 2022, from https://www.ibge.gov.br/geociencias/informacoes-sobre-posicionamento-geodesico/rede-geodesica/16327-banco-de-dados-geodesicos.html?=&t=o-que-e
- IBGE. (2022b). Instituto Brasileiro de Geografia e Estatística. Monitoramento da Cobertura e Uso da Terra. Retrieved March 26, 2022, from https://www.ibge.gov.br/geociencias/informacoes-ambientais/cobertura-e-uso-da-terra/15831-cobertura-e-uso-da-terra-do-brasil.html?=&t=notas-tecnicas
- INPE. (2008). Insitituto Nacional de Pesquisas Espaciais. TOPODATA - Banco de Dados Geomorfométricos do Brasil. Retrieved October 12, 2022, from http://www.dsr.inpe.br/topodata/index.php
- Kaliraj, S., Chandrasekar, N., & Magesh, N. (2015). Morphometric analysis of the River thamirabarani sub-basin in Kanyakumari District, South west coast of Tamil Nadu, India, using remote sensing and GIS. Environmental Earth Sciences, 73(11), 7375–7401. https://doi.org/10.1007/s12665-014-3914-1
- Kastrisios, C., & Tsoulos, L. (2018). Voronoi tessellation on the ellipsoidal earth for vector data. International Journal of Geographical Information Science, 32(8), 1541–1557. https://doi.org/10.1080/13658816.2018.1434890
- Lee, S., & Sambath, T. (2006). Landslide susceptibility mapping in the Damrei Romel area, Cambodia using frequency ratio and logistic regression models. Environmental Geology, 50(6), 847–855. https://doi.org/10.1007/s00254-006-0256-7
- Lei, X., Chen, X., Yang, Z., He, S., Zhu, L., & Liang, H. (2022). A simple and robust MPM framework for modelling granular flows over complex terrains. Computers and Geotechnics, 149, 104867. https://doi.org/10.1016/j.compgeo.2022.104867
- Leon, J. X., Heuvelink, G. B., Phinn, S. R., & Schumann, G. J. P. (2014). Incorporating DEM uncertainty in coastal inundation mapping. PLoS One, 9(9), e108727. https://doi.org/10.1371/journal.pone.0108727
- Lindsay, J. B. (2016). Whitebox GAT: A case study in geomorphometric analysis. Computers and Geosciences, 95, 75–84. https://doi.org/10.1016/j.cageo.2016.07.003
- Li, L., Nearing, M. A., Nichols, M. H., Polyakov, V. O., Guertin, D. P., & Cavanaugh, M. L. (2020). The effects of DEM interpolation on quantifying soil surface roughness using terrestrial LiDAR. Soil and Tillage Research, 198, 104520. https://doi.org/10.1016/j.still.2019.104520
- Liu, Z., Zhu, J., Fu, H., Zhou, C., & Zuo, T. (2020). Evaluation of the vertical accuracy of open global DEMs over steep terrain regions using ICESat data: A case study over Hunan Province, China. Sensors, 20(17), 4865. https://doi.org/10.3390/s20174865
- Merghadi, A., Yunus, A. P., Dou, J., Whiteley, J., ThaiPham, B., Bui, D. T., Avtar, R., & Abderrahmane, B. (2020). Machine learning methods for landslide susceptibility studies: A comparative overview of algorithm performance. Earth-Science Reviews, 207, 207. https://doi.org/10.1016/j.earscirev.2020.103225
- Mesa-Mingorance, J. L., & Ariza-López, F. J. (2020). Accuracy assessment of digital elevation models (DEMs): A critical review of practices of the past three decades. Remote Sensing, 12(16), 2630. https://doi.org/10.3390/rs12162630
- Miceli, B. S., Dias, F. D. M., Seabra, F. M., Santos, P., & Fernandes, M. D. C. (2011). Avaliação vertical de modelos digitais de elevação (MDEs) em diferentes configurações topográficas para médias e pequenas escalas. Revista Brasileira de Cartografia, 63(1), 191–201. https://doi.org/10.14393/rbcv63n1-43731
- Moran, P. A. (1950). Notes on continuous stochastic phenomena. Biometrika, 37(1/2), 17–23. https://doi.org/10.1093/biomet/37.1-2.17
- Moura, L. Z., Bias, E. D. S., & Brites, R. (2014). Avaliação da acurácia vertical de modelos digitais de elevação (MDEs) nas bacias do Paranoá e São Bartolomeu. Revista Brasileira de Cartografia, 66(1). https://doi.org/10.14393/rbcv66n1-43892
- Mukherjee, S., Joshi, P. K., Mukherjee, S., Ghosh, A., Garg, R. D., & Mukhopadhyay, A. (2013). Evaluation of vertical accuracy of open source digital elevation model (DEM). International Journal of Applied Earth Observation and Geoinformation, 21, 205–217. https://doi.org/10.1016/j.jag.2012.09.004
- NASA. (2013). National Aeronautics and space Administration. Shuttle Radar Topography Mission global 1 arc second. Version 3. In NASA EOSDIS land processes DAAC. USGS Earth Resources Observation and Science (EROS) Center. https://doi.org/10.5067/MEaSUREs/SRTM/SRTMGL1.003
- NASA. (2022). National Aeronautics and Space Administration. Shuttle Radar Topography Mission. Shuttle Radar Topography Mission. Retrieved July 15, 2022, from https://www2.jpl.nasa.gov/srtm/index.html
- Nene, S. A., & Nayar, S. K. (1997). A simple algorithm for nearest neighbor search in high dimensions. IEEE Transactions on Pattern Analysis and Machine Intelligence, 19(9), 989–1003. https://doi.org/10.1109/34.615448
- Orlando, F. C. (2019). Análise da potencialidade do uso de MDE global em trabalho de grande escala ao longo de todo o território brasileiro.
- Oshan, T. M., Li, Z., Kang, W., Wolf, L. J., & Fotheringham, A. S. (2019). MGWR: A python implementation of Multiscale Geographically weighted regression for investigating process spatial heterogeneity and scale. ISPRS International Journal of Geo-Information, 8(6), 269. https://doi.org/10.3390/ijgi8060269
- Pandey, P., Manickam, S., Bhattacharya, A., Ramanathan, A., Singh, G., & Venkataraman, G. (2017). Qualitative and quantitative assessment of TanDEM-X DEM over western Himalayan glaciated terrain. Geocarto International, 32(4), 442–454. https://doi.org/10.1080/10106049.2016.1155655
- Pipaud, I., Loibl, D., & Lehmkuhl, F. (2015). Evaluation of TanDEM-X elevation data for geomorphological mapping and interpretation in high mountain environments - a case study from SE Tibet, China. Geomorphology, 246, 232–254. https://doi.org/10.1016/j.geomorph.2015.06.025
- Polidori, L., & El Hage, M. (2020). Digital elevation model quality assessment methods: A critical review. Remote Sensing, 12(21), 3522. https://doi.org/10.3390/rs12213522
- Prasannakumar, V., Vijith, H., Charutha, R., & Geetha, N. (2011). Spatio-temporal clustering of road accidents: GIS based analysis and assessment. Procedia-Social and Behavioral Sciences, 21, 317–325. https://doi.org/10.1016/j.sbspro.2011.07.020
- Rawat, K. S., Singh, S. K., Singh, M. I., & Garg, B. (2019). Comparative evaluation of vertical accuracy of elevated points with ground control points from ASTERDEM and SRTMDEM with respect to CARTOSAT-1DEM. Remote Sensing Applications: Society & Environment, 13, 289–297. https://doi.org/10.1016/j.rsase.2018.11.005
- Rodrigues, T. G., Paradella, W. R., & Oliveira, C. G. (2011). Evaluation of the altimetry from SRTM-3 and planimetry from high-resolution PALSAR FBD data for semi-detailed topographic mapping in the Amazon region. Anais da Academia Brasileira de Ciências, 83(3), 953–966. https://doi.org/10.1590/S0001-37652011000300014
- Safar, M. (2005). K nearest neighbor search in navigation systems. Mobile Information Systems, 1(3), 207–224. https://doi.org/10.1155/2005/692568
- Saleem, N., Huq, M., Twumasi, N. Y. D., Javed, A., & Sajjad, A. (2019). Parameters derived from and/or used with digital elevation models (DEMs) for landslide susceptibility mapping and landslide risk assessment: A review. ISPRS International Journal of Geo-Information, 8(12), 545. https://doi.org/10.3390/ijgi8120545
- Sánchez-Guillamón, O., Fernández-Salas, L. M., Vázquez, J. T., Palomino, D., Medialdea, T., López-González, N., Somoza, L., & León, R. (2018). Shape and size complexity of deep seafloor mounds on the canary basin (west to canary islands, Eastern Atlantic): A DEM-based geomorphometric analysis of domes and volcanoes. Geosciences (Switzerland), 8(2), 37. https://doi.org/10.3390/geosciences8020037
- Sarma, C. P., Dey, A., & Krishna, A. M. (2020). Influence of digital elevation models on the simulation of rainfall-induced landslides in the hillslopes of Guwahati, India. Engineering Geology, 268, 105523. https://doi.org/10.1016/j.enggeo.2020.105523
- Satgé, F., Bonnet, M.-P., Timouk, F., Calmant, S., Pillco, R., Molina, J., Lavado-Casimiro, W., Arsen, A., Crétaux, J., & Garnier, J. (2015). Accuracy assessment of SRTM v4 and ASTER GDEM v2 over the altiplano watershed using ICESat/GLAS data. International Journal of Remote Sensing, 36(2), 465–488. https://doi.org/10.1080/01431161.2014.999166
- Satge, F., Denezine, M., Pillco, R., Timouk, F., Pinel, S., Molina, J., Garnier, J., Seyler, F., & Bonnet, M.-P. (2016). Absolute and relative height-pixel accuracy of SRTM-GL1 over the South American Andean Plateau. ISPRS Journal of Photogrammetry and Remote Sensing, 121, 157–166. https://doi.org/10.1016/j.isprsjprs.2016.09.003
- Schwendel, A. C., & Milan, D. J. (2020). Terrestrial structure-from-motion: Spatial error analysis of roughness and morphology. Geomorphology, 350, 106883. https://doi.org/10.1016/j.geomorph.2019.106883
- Sharma, M., Garg, R. D., Badenko, V., Fedotov, A., Min, L., & Yao, A. (2021). Potential of airborne LiDAR data for terrain parameters extraction. Quaternary International, 575, 317–327. https://doi.org/10.1016/j.quaint.2020.07.039
- Shean, D. E., Alexandrov, O., Moratto, Z. M., Smith, B. E., Joughin, I. R., Porter, C., & Morin, P. (2016). An automated, open-source pipeline for mass production of digital elevation models (DEMs) from very-high-resolution commercial stereo satellite imagery. ISPRS Journal of Photogrammetry and Remote Sensing, 116, 101–117. https://doi.org/10.1016/j.isprsjprs.2016.03.012
- Silva, J. F. D., Queiroga Miranda, R. D., & Candeias, A. L. B. (2022). Análise dos Modelos Digitais de Elevação (PE3D, SRTM-30, SRTM-90, ASTER GDEM, TOPODATA, TANDEM-X, ALOS PALSAR e ALOS AW3D30) e a necessidade da produção de dados altimétricos em excelência no Brasil. Revista Brasileira de Geografia Física, 15(3), 1543–1555. https://doi.org/10.26848/rbgf.v15.3.p1543-1555
- Suliman, A. H. A., Gumindoga, W., Awchi, T. A., & Katimon, A. (2021). DEM resolution influences on peak flow prediction: A comparison of two different based DEMs through various rescaling techniques. Geocarto International, 36(7), 803–819. https://doi.org/10.1080/10106049.2019.1622599
- Szypuła, B. (2019). Quality assessment of DEM derived from topographic maps for geomorphometric purposes. Open Geosciences, 11(1), 843–865. https://doi.org/10.1515/geo-2019-0066
- Taheri, M., Dolatabadi, N., Nasseri, M., Zahraie, B., Amini, Y., & Schoups, G. (2020). Localized linear regression methods for estimating monthly precipitation grids using elevation, rain gauge, and TRMM data. Theoretical and Applied Climatology, 142(1–2), 623–641. https://doi.org/10.1007/s00704-020-03320-2
- Temme, A., Heuvelink, G., Schoorl, J., & Claessens, L. (2009). Geostatistical simulation and error propagation in geomorphometry. Developments in Soil Science, 33, 121–140. https://doi.org/10.1016/S0166-2481(08)00005-6
- UNAVCO. (2022). EarthScope consortium. Geoid height calculator. Retrieved March 28, 2022, from https://www.unavco.org/software/geodetic-utilities/geoid-height-calculator/geoid-height-calculator.html
- Uysal, M., Toprak, A. S., & Polat, N. (2015). DEM generation with UAV Photogrammetry and accuracy analysis in Sahitler hill. Measurement, 73, 539–543. https://doi.org/10.1016/j.measurement.2015.06.010
- Valeriano M. D. M.2008. São José dos Campos: Instituto Nacional de Pesquisas Espaciais (INPE) (Report no. INPE-15318-RPQ/818). http://mtc-m16c.sid.inpe.br/col/sid.inpe.br/mtc-m18@80/2008/07.11.19.24/doc/publicacao.pdf
- Valeriano M. D. M., and Rossetti D. D. F. 2008. São José dos Campos (Report no. INPE-16701-RPQ/853). INPE. http://mtc-m16d.sid.inpe.br/col/sid.inpe.br/mtc-m19@80/2010/05.10.18.35/doc/publicacao.pdf
- Valeriano, M. D. M., & Rossetti, D. D. F. (2012). Topodata: Brazilian full coverage refinement of SRTM data. Applied Geography, 32(2), 300–309. https://doi.org/10.1016/j.apgeog.2011.05.004
- Varga, M., & Bašić, T. (2015). Accuracy validation and comparison of global digital elevation models over Croatia. International Journal of Remote Sensing, 36(1), 170–189. https://doi.org/10.1080/01431161.2014.994720
- Vignesh, K. S., Anandakumar, I., Ranjan, R., & Borah, D. (2021). Flood vulnerability assessment using an integrated approach of multi-criteria decision-making model and geospatial techniques. Modeling Earth Systems and Environment, 7(2), 767–781. https://doi.org/10.1007/s40808-020-00997-2
- Wessel, B., Huber, M., Wohlfart, C., Marschalk, U., Kosmann, D., & Roth, A. (2018). Accuracy assessment of the global TanDEM-X digital elevation model with GPS data. ISPRS Journal of Photogrammetry and Remote Sensing, 139, 171–182. https://doi.org/10.1016/j.isprsjprs.2018.02.017
- Weydahl, D. J., Sagstuen, J., Dick, O. B., & Ronning, H. (2007). SRTM DEM accuracy assessment over vegetated areas in Norway. International Journal of Remote Sensing, 28(16), 3513–3527. https://doi.org/10.1080/01431160600993447
- Wise, S. (2000). Assessing the quality for hydrological applications of digital elevation models derived from contours. Hydrological Processes, 14(11–12), 1909–1929. https://doi.org/10.1002/1099-1085(20000815/30)14:11/12<1909:AID-HYP45>3.0.CO;2-6
- Wise, S. (2012). Information entropy as a measure of DEM quality. Computers & Geosciences, 48, 102–110. https://doi.org/10.1016/j.cageo.2012.05.011
- Xu, S., Wu, C., Wang, L., Gonsamo, A., Shen, Y., & Niu, Z. (2015). A new satellite-based monthly precipitation downscaling algorithm with non-stationary relationship between precipitation and land surface characteristics. Remote Sensing of Environment, 162, 119–140. https://doi.org/10.1016/j.rse.2015.02.024
- Xu, Y., Zhu, H., Hu, C., Liu, H., & Cheng, Y. (2021). Deep learning of DEM image texture for landform classification in the Shandong area, China. Frontiers of Earth Science, 16(2), 352–367. https://doi.org/10.1007/s11707-021-0884-y
- Yap, L., Kandé, L. H., Nouayou, R., Kamguia, J., Ngouh, N. A., & Makuate, M. B. (2019). Vertical accuracy evaluation of freely available latest high-resolution (30 m) global digital elevation models over Cameroon (Central Africa) with GPS/leveling ground control points. International Journal of Digital Earth, 12(5), 500–524. https://doi.org/10.1080/17538947.2018.1458163
- Yu, H., Fotheringham, A. S., Li, Z., Oshan, T., Kang, W., & Wolf, L. J. (2020). Inference in Multiscale Geographically Weighted regression. Geographical Analysis, 52(1), 87–106. https://doi.org/10.1111/gean.12189
Appendix
Figure A1. Correlation between the elevation of the reference points (BGN) and the elevation extracted from SRTM (A1) and Topodata (A2)

Figure B1. Statistical analysis of the altimetric error regarding slope (B1), elevation (B2) and LULC class (B3).

Table D1. Statistics of significant coefficient estimates (p ≤ 0.05 of t-test) for the MGWR model of altimetric error.