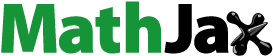
ABSTRACT
Soil moisture estimation is a key component in hydrological processes and irrigation amounts' estimation. The synergetic use of optical and radar data has been proven to retrieve the surface soil moisture at a field scale using the Water Cloud Model (WCM). In this work, we evaluate the impact of staellite-derived vegetation descriptors to estimate the surface soil moisture. Therefore, we used the Sentinel-1 data to test the polarization ratio () and the normalized polarization ratio (IN) and the frequently used optical Normalized Difference vegetation Index (NDVI) as vegetation descriptors. Synchronous with Sentinel-1 acquisitions, in situ soil moisture were collected over wheat fields in the Kairouan plain in the center of Tunisia. To avoid the bare soil roughness effect and the radar signal saturation in dense vegetation context, we considered the data where the NDVI values vary between 0.25 and 0.7. The soil moisture inversion using the WCM and NDVI as a vegetation descriptor was characterized by an RMSE value of 5.6 vol.%. A relatively close performance was obtained using IN and (
) with RMSE under 7. 5 vol.%. The results revealed the consistency of the radar-derived data in describing the vegetation for the retrieval of soil moisture.
Introduction
Water shortages threaten the Mediterranean basin, especially in the southern part, where the impacts of climate change are the most felt (Schilling et al., Citation2020; Tramblay & Somot, Citation2018). Therefore, the optimization of water resource management is essential for sustainable agriculture (Massari et al., Citation2021). Surface soil moisture is a key component in the hydrological processes that controls the amount of runoff and infiltrated water (Brocca et al., Citation2010, Citation2017; Koster et al., Citation2016). For soil water content estimation, several soil moisture monitoring techniques based on punctual soil sampling, such as the gravimetric method, and automated soil moisture measurement techniques, such as time domain reflectometry and Thetaprobe sensors, have been used. These methods are time-consuming and require substantial labor to assess the spatial heterogeneities and the temporal dynamics of the soil moisture (Peng et al., Citation2021; Walker et al., Citation2004).
To overcome these limitations, remote sensing products were used as an alternative to retrieve surface soil moisture. Owing to the lower sensitivity of microwave signals to weather conditions, operational soil moisture products are available with a spatial resolution varying between 25 and 35 km and a revisit time of up to one day and include the Soil Moisture and Ocean Salinity (SMOS) (Al Bitar et al., Citation2017; Kerr et al., Citation2001), Soil Moisture Active/Passive (SMAP) (Entekhabi et al., Citation2014) and Advanced Scatterometer (ASCAT) (Wagner et al., Citation2013). These products have been evaluated by a large number of studies (Albergel et al., Citation2009; Chen et al., Citation2018; El Hajj et al., Citation2018; Mohanty et al., Citation2017; Wagner et al., Citation2007; Kerr et al., Citation2010). The coarse resolution of these products is insufficient to cover soil moisture heterogeneities at the agricultural field scale. Therefore, a high spatial resolution of soil moisture is needed. In this context, several efforts were devoted to retrieving the soil moisture using synthetic radar aperture (SAR) data owing to its sensitivity to the geometric and dielectric properties of the soil. At the field scale, various approaches have been developed to retrieve surface soil moisture using available radar acquisitions in the X-band (Aubert et al., Citation2013; El Hajj et al., Citation2016; Fontanelli et al., Citation2013; Gorrab et al., Citation2015), C-band (Amazirh et al., Citation2018; Amri et al., Citation2012; Bousbih et al., Citation2017; Ezzahar et al., Citation2019; Gao et al., Citation2017; Kumar et al., Citation2019; Ouaadi et al., Citation2020; Wang et al., Citation2018, Citation2023) and L-band (Fascetti et al., Citation2017; Hamze et al., Citation2021; Hosseini & McNairn, Citation2017; Lievens & Verhoest, Citation2011; Shi et al., Citation1995, Citation1997; Zribi et al., Citation2019).
Due to the lack of available L-band data, most of the available works were developed using the X- and C-bands, especially after the launch of Sentinel-1 A and B on 3 April 2014 and 25 April 2016, respectively (ESA, Citation2012). These approaches are based on radar signal behavior modeling and inversion to estimate soil moisture. Over bare soil fields, various empirical models, semiempirical models such as Oh and Dubois and physical models such as the integral equation model (IEM), have been used (Baghdadi & Zribi, Citation2006; Baghdadi et al., Citation2011; Choker et al., Citation2017; Panciera et al., Citation2014; Tao et al., Citation2015). The main aim of these models is to relate the radar signal to ground data such as ground truth soil moisture measurements and geometric statistical parameters, including the root mean square of height (Hrms) and the correlation length (Lc).
The presence of vegetation impacts the radar signal behavior. Therefore, different models were proposed in this context to consider the crop contribution in the scattering process (Ulaby et al., Citation1990; Ulaby, Citation1975; Ulaby et al., Citation1984). The water cloud model (WCM) (Attema & Ulaby, Citation1978) has been extensively used in many works due to its simplicity. The total backscattering is the sum of the vegetation contribution, the attenuated bare soil contribution and the soil-vegetation interaction contribution. The interaction term is often neglected in the case of the C-band. The WCM may be coupled to the aforementioned bare soil models to calculate the soil contribution. The vegetation contribution and the attenuation term are calculated as a function of the crop descriptor. Notably, crop description is critical for the soil moisture estimation process.
The choice of the vegetation descriptor was extensively discussed and tested due to the complexity of the geometric structure and dynamics of vegetation. Some vegetation descriptors may be measured in situ, such as vegetation height, leaf area index, leaf water area index, aboveground biomass and vegetation water content (Chauhan et al., Citation2018; El Hajj et al., Citation2014; Kumar et al., Citation2015). Using optical data, crop descriptions may include indices such as the normalized difference vegetation index (NDVI) (Ayari et al., Citation2021; Baghdadi et al., Citation2017; Bousbih et al., Citation2018; Prakash et al., Citation2012) or be directly extracted from MODIS products such as NDVI, the leaf area index (LAI) and the enhanced vegetation index (EVI) (Wang et al., Citation2019). Several studies have estimated the descriptors using established empirical relationships between SAR data and vegetation variables (Ouaadi et al., Citation2021) or between optical data and variables (Liu & Shi, Citation2016; Ma et al., Citation2020). Periasamy (Citation2018) investigated the potential of C-band Sentinel-1 to estimate the Above Ground Biomass using the calculated SAR variables at i pixel: such as VH backscattering coefficients, the inverse dual polarization diagonal distance , and the vertical dual de-polarization index
. To retrieve the soil moisture at field-scale, Bhogapurapu et al. (Citation2022) used the copolarized purity parameter
, where q is the ratio parameter (
and X and Y are H or V polarizations, respectively, as the vegetation descriptor over croplands and shrublands. The aforementioned derived parameter already proved its pertinence to monitor the vegetation growth cycle in (Mandal et al., Citation2020).
In the L-band, Wang et al. (Citation2021) tested four vegetation descriptors to retrieve the soil moisture, including the vegetation water content (VWC-NDVI) estimated using the empirical relationship between in situ measurements and the NDVI and the derived SAR data, such as the RVI (, the polarization ratio (
) and the VH backscattering coefficients. A similar accuracy was obtained by the VWC-NDVI and the polarization ratio as crop descriptors with RMSE values equal to 8.8 vol.% and 9.1 vol.%, respectively (Wang et al., Citation2021).
For grasslands, using X-band data, El Hajj et al. (Citation2016) investigated the potential of vegetation descriptors derived from optical data such as the fraction of absorbed active radiation, the fractional vegetation cover, the leaf area index and NDVI. For NDVI values fluctuating between 0.45 and 0.75, the RMSE value was 3.6 vol.% when the NDVI was used as the vegetation variable. When the NDVI exceeded 0.75, the RMSE increased to 6 vol.%. The soil moisture retrieval accuracy was similar for the rest of the tested descriptors. The use of the NDVI as vegetation descriptor is limited by the cloud presence and its saturation within dense vegetation context. Therefore, in the present work, as an alternative to NDVI, we investigate the potential of the derived Sentinel-1 C-band SAR data to incorporate the wheat cover effects when estimating surface soil in the semi-arid Kairouan plain in Tunisia. The used data and the adopted methodology are described in the second section. In the third section, we present the results and the discussion. In the final section, we draw the conclusions.
Materials and methodology
Study area and ground-based data
The study area is located in the plain of Kairouan in Tunisia (35°1′–35°55′ N, 9°23′–10°17′ E) as illustrated in . This alluvial flat landscape covers an area of 3000 km2 and is characterized by a semi-arid climate. The annual quantity of precipitation is approximately 300 mm with an irregular spatiotemporal distribution. The temperature varies from 11°C in the coldest months, namely, December, January and February, to 30°C during July and August, the hottest months. The land use mainly comprises cereals, olive trees, orchards and seasonal market garden crops (Amri et al., Citation2012; Shabou et al., Citation2015; Zribi et al., Citation2011).
In the present work, we used collected insitu data in several selected wheat fields in the plain of Kairouan during two agricultural years (2015/2016; 2016/2017) and the spring of 2022 (from April to mid-May). The wheat cycle in Kairouan starts with sowing events in December, vegetation development, and the heading approximately during the mid of March and senescence phases. It ends with the harvest extending from the end of May to the end of June. Over the wheat reference fields and during S-1 acquisitions, soil moisture measurements were collected at approximately 20 points by the handheld Thetaprobe in the first top layer of soil (first 5 cm). The used Theataprobes were already calibrated with gravimetric measurements over the same studied area in (Zribi et al., Citation2014). As detailed in , the soil moisture values vary from 7.5 to 41.2 vol.%.
Table 1. Characteristics of Sentinel-1 images and collected ground-based soil moisture during the agricultural years (2015–2016 and 2016–2017) and the spring of 2022 (due to the failure of the Sentinel-1B, the C-band images in 2022 were acquired every 12 days with Sentinel-1 A).
Satellite data
Sentinel-1 (S-1)
Since the launch of Sentinel-1A and B on 3 April 2014 and 25 April 2016, respectively, C-band synthetic aperture radar (SAR) data are available in a variety of modes, polarizations and resolutions. For the present study, we used Ground Range Detected S-1 images acquired in Interferometric Wide Swath (IW) mode at a frequency of 5.405 GHz in vertical-vertical (VV) and vertical-horizontal (VH) polarizations. For the study area, the selected images were characterized by a pixel spacing of 10 m × 10 m and an incidence angle between 39° and 40°, as described in .
The already processed S-1 images were downloaded from Google Earth Engine (https://developers.google.com/earth-engine/datasets/catalog/sentinel). For each S-1 scene, the backscattering coefficients were generated in dB scale in VV-polarization (and VH-polarization (
in each pixel were derived after five processing steps, namely, orbit file application, border and thermal noise removal, radiometric calibration and terrain correction. After converting each scene to the linear scale, the backscattering coefficients were averaged per field. The polarization ratio(
) in linear scale was computed for each field. The normalized index IN was computed from the polarization ratio(
) converted in dB scale for each reference field during each wheat growth season using the following equation in Rolle et al. (Citation2022):
The normalization of the polarization ratio aims to have a radar index comparable to the NDVI, where the values range from 0 (when to 1 (when
) and the
and
are calculated per reference fieldand for each wheat growth season.
Sentinel-2 (S-2)
The surface reflectance data were cloud-free selected and downloaded from the Theia site (https://www.theia-land.fr/donnees-satellitaires/). The Sentinel-2 data were corrected for atmospheric effects via the MAJA software (Hagolle et al., Citation2017), which detected clouds and their shadows and produced level 2A reflectance data. We used the red and infrared bands, B4 and B8, respectively, to calculate the normalized difference vegetation index (NDVI). For each wheat field and each date, the mean value of NDVI is calculated.
Water cloud model (WCM)
As developed by (Attema & Ulaby, Citation1978), the WCM considers the total backscattering as the sum of the vegetation contribution, the bare soil contribution attenuated by the vegetation and the contribution of soil-vegetation interactions. Due to the limited penetration depth of the C-band signal, the interaction term is often neglected. Consequently, the total backscattering coefficient at the field scale can be calculated using the following equations (2–4):
The bare soil backscattering was calculated using the integral equation model with the calibrated correlation length (IEM-B) as detailed in (Baghdadi et al., Citation2006). The bare soil properties included the measured soil moisture and constant geometrical parameter (Hrms) for all the wheat reference fields. Due to the lack of roughness measurements, we considered that Hrms is equal to 1 cm as measured in semi-arid Mediterranean regions in (Bousbih et al., Citation2017; Ouaadi et al., Citation2021) and tested in (Ouaadi et al., Citation2020).
The Integral Equation Model was funded by Fung et al., Citation1992 as function of the SAR sensor parameters (incidence angle, polarization and the frequency) as well as the surface properties such as the roughness and dielectric properties. The potential of the IEM to simulate the radar backscattering coefficients was examined in several works. The correlation length is one of the inputs of IEM which is difficult to estimate and the less accurate among the roughness measurements. Therefore, Baghdadi et al. (Citation2006), replaced the correlation length with a calibrated parameter expressed as function of the incidence angle and the Hrms values as the following equation for the C-band data in VV polarization and using the Gaussian correlation function:
In the present paper, our main concern is about correcting the vegetation cover effect in the soil moisture retrieve process using different descriptors calculated whether from optical or radar data. In this context, we avoided the analysis of bare soil (NDVI >0.25) to allow a precise estimation of each descriptor’s contribution.
Additionally, we used the threshold of NDVI under 0.7 to avoid dealing with very dense vegetation context. In fact, the C-band radar signal has already shown its limitation in correcting the effect of very dense vegetation for soil moisture retrieval.
In (Baghdadi et al., Citation2017), the Sentinel-1 signal simulation using the WCM and the NDVI as vegetation descriptor revealed that the vegetation contribution dominates the attenuated bare soil backscattering when NDVI is equal to 07 and the soil moisture is about 5 vol.%. At an incidence angle higher than 40°, the NDVI threshold is about 0.6.
By comparing the potential of L and C-bands data, the authors of (El Hajj et al., Citation2019) demonstrated that the penetration depth of the C-band signal is limited where the NDVI is equal to 0.7. Based on these conclusions, the operational products of surface soil moisture based on the synergy of Sentinel-1 and Sentinel-2 were limited to NDVI values lower than 0.75 (El Hajj et al., Citation2017). The main aim is to better estimate the contributions of each vegetation descriptor within the optimum inversion context. The vegetation contribution The vegetation contribution and the attenuation term
were calculated as functions of the vegetation descriptors (
and
) and the incidence angle
.
In the present study, we tested three vegetation descriptors =
NDVI),
=
polarization ratio in linear scale) and
=
IN).
and
are parameters that were calibrated and validated depending on the vegetation properties and the SAR sensor parameters. The A and B are two key parameters in the WCM simulations of the total backscattering over covered fields. As discussed in (Park et al., Citation2019), the accuracy of these parameters will improve the estimation of the attenuation and the volume scattering and consequently the accuracy of the surface soil moisture
Seventy percent of the entire dataset was used for the calibration (163 points), and 30% was devoted to the validation step (70 points).
Results
Radar sensitivity to surface soil moisture
In , we scatterplot the backscattering coefficients in VV and VH polarizations as a function of the in-situ measurements of the surface soil moisture. Using the copolarized data (, we observe better sensitivity to soil moisture with a value of 0.12 dB/vol.% compared to 0.04 dB/vol.% using the VH polarization (
. The correlation coefficient values vary between 0.56 and 0.12 for VV and VH, respectively. According to , some discrepancies were observed. These points are characterized by high NDVI values (NDVI >0.6), indicating the presence of dense vegetation combined with soil moisture values varying between 25 and 35 vol.%. The presence of vegetation introduces volume scattering in the backscattering process while the bare soil contribution decreases with the vegetation development. This effect is more clearly highlighted in the cross-polarization (VH) than in the copolarization (VV).
Figure 2. Scatterplots of the backscattering coefficients in VV and VH polarizations as a function of the in-situ measurements of soil moisture over the wheat reference fields in the Kairouan plain.

To examinate the vegetation impact on the soil moisture retrieval, in the backscattering coefficients in dB were analyzed as function of the measured soil moisture according to three classes of NDVI: 0.25 < NDVI ≤ 0.4, 0.4 < NDVI ≤ 0.55 and 0.55 < NDVI ≤ 0.7. The sensitivity of the VV-polarized data to the soil moisture (correlation coefficient) decreases from 0.18 dB/vol.% (r = 0.71) to 0.07 dB/vol.% (r = 0.43) when the NDVI values increase from 0.25 to 0.7. Using the VH-polarized C-band data, the sensitivity of radar signal to the soil moisture varies between 0.06 and 0.03 dB/vol.%. The intercomparison between the two polarizations highlights that the co-polarized data is more sensitive to soil moisture than the cross-polarized one.
Figure 3. Scatterplots of the Sentinel-1 backscattering coefficients as a function of the in-situ measurements of soil moisture over the wheat reference fields according to three classes of NDVI (0.25 < NDVI ≤ 0.4, 0.4 < NDVI ≤ 0.55 and 0.55 < NDVI ≤ 0.7).

In , the sensitivity of the backscattering coefficients is illustrated as function of the soil moisture according to the vegetation development phases: vegetative phase and productive phase. For the first phase which occurred from December to the mid of March, the vegetation is developed and marked finally by the heading event (the NDVI values increase to a maximum value). The second phase, which started after the heading event is marked by the presence of the vegetation volume and the grain filling until the harvest starting from the end of May (the NDVI values decrease to a maximum value).
During the first phase, we observe that the Sentinel-1 is more sensitive to the soil moisture in VV polarization (0.12 dB/vol.%) more than VH polarization (0.08 dB/vol.%). This may be induced from the sensitivity of VV polarization to the bare soil contribution in this first phase of wheat development. Regarding the second phase, when the vegetation covers the soil and its volume governs the scattering process, the sensitivity of the VV-polarized signal decreases to be in the same range of the VH-polarized data. Owing to this lower sensitivity of cross-polarized data to soil moisture, we selected only the copolarized data to estimate the soil moisture.
Empirical relationships between vegetation descriptors
In , we analyzed the NDVI as a function of the calculated radar variables such as the polarization ratio in dB and its normalization (IN). Positive correlations characterize the linear relationships between the polarization ratio and the NDVI. The correlation coefficients vary from 0.67 to 0.58 using the data during 2015/2016 and 2016/2017, respectively.
The lowest correlation coefficient, equal to 0.17, is observed in the scatterplot of the NDVI as a function of the polarization ratio during the spring of 2022, where the polarization ratio values are between − 6 and −4 dB with low NDVI value dynamics. The high values of the ratio indicate high VH backscattering in addition to a lower contribution of VV backscattering. Otherwise, well-developed vegetation, contributes by volume scattering within the total backscattering, regarding a decreased contribution from bare soil. This situation could be observed especially in the senescence phase of the wheat where the vegetation is present and mature with a yellow color and the maximum developed biomass. Therefore, the NDVI values are low regarding high ratio values, as identified by the red circles in , during 2016/2017. The same trends were observed for winter wheat using the polarization ratio (Ouaadi et al., Citation2020; Veloso et al., Citation2017). The same discrepancies were observed for the data between April and May 2022, which used the IN with an correlation coefficient equal to 0.2. These discrepancies confirmed the limited potential of radar data in case of dense vegetation especially after wheat maturation.
Radar signal modeling over wheat fields
The use of WCM requires the calibration and validation of and
parameters for each tested vegetation descriptor, namely, NDVI, polarization ratio and IN.
The values vary between 0.05 and 0.125, and the
values range from 0.55 to 1.18. The highest values of A and B, 0.1 and 1.18, respectively, are found using the polarization ratio. Over the same validation site, using the NDVI as a vegetation descriptor and the WCM coupled with the empirical model, the calibrated A and B parameters are equal to 0.06 and 0.42, respectively (Bousbih et al., Citation2017, Citation2018)
For the WCM coupled to IEM-B was tested in (Baghdadi et al., Citation2017) over the same study site of Kairouan plain, using the C-band data, the A parameter is equal to 0.095, and the B parameter is approximately 0.55. In , we scatterplot the modeled and S-1 observed backscattering coefficients with the respective calibrated coefficients A and B and the statistical parameters root mean square error (RMSE), bias and correlation coefficients (r). The RMSE and the bias values vary from 1.2 to 1.32 dB and from − 0.1 to −0.05 dB, respectively. The r values fluctuate between 0.59 and 0.69 using the three satellite-derived variables.
Figure 6. Scatterplots of calibrated backscattering coefficients and the VV-polarized S-1 data using the remote sensing data as vegetation descriptors (NDVI, IN and the polarization ratio in linear scale) with the corresponding statistical parameters (RMSE, bias and r).

After the WCM calibration using 70% of the dataset, we used the A and B parameters to simulate the signal over the wheat fields. shows the relationships between the modeled backscattering coefficients and the Sentinel-1 coefficients. Using the NDVI as the vegetation variable (V1=V2=NDVI), the RMSE and the bias values are equal to 1.26 dB and 0.02 dB, respectively. Based on the S-1 data, we notice almost the same RMSE values equal to 1.15 dB using the IN and 1.13 dB using the polarization ratio. According to the bias calculations, we observe low bias values between −0.06 dB and 0.02 dB. High correlation values characterize the linear relationships between the simulated radar signal and the Sentinel-1 signal for VV polarization varying between 0.61 and 0.7.
Soil moisture estimation
To retrieve the soil moisture, we performed an inversion of the WCM using the lookup table algorithm. In , we represent the estimated soil moisture as a function of the in-situ measurements and the statistical parameters (r, RMSE and bias). The inversion of soil moisture using the WCM model and the optical index as a vegetation descriptor is characterized by the lowest RMSE value of 5.58 vol.%.
A relatively close performance was obtained using IN and the polarization ratio with RMSE values equal to 6.7 and 7.45 vol.%, respectively. However, the correlation decreases significantly from 0.68 to 0.53 and 0.4 when using IN and the polarization ratio, respectively. Considering the polarization ratio, we observe a satisfactory level of accuracy, where the RMSE and bias values are approximately 7.45 vol.% and − 1.47 vol.%, respectively. A relatively close performance characterizes the use of the IN as a wheat descriptor in the soil moisture estimation with a mean underestimation of 2 vol.%.
Discussion
The incorporation of the SAR-derived variables as vegetation descriptor results produced a relatively close accuracy to the soil moisture estimations attained by the optical index. In terms of RMSE values, a difference of 10% between the IN and the polarization ratio characterizes the performance of the soil water content. This result may be due to the normalization of the polarization ratio, which is an index with a range of values between 0 and 1. Consequently, this normalization decreases the differences between the fields in terms of roughness effects and vegetation-induced backscattering.
The aforementioned results were in the same range of accuracy in previous works. Over the same study area, the authors of (Bousbih et al., Citation2018) estimated the soil moisture using Sentinel-1 in VV polarization and the NDVI as a wheat descriptor. The RMSE and correlation coefficient of the soil moisture content estimates were 6.4 vol.% and 0.63, respectively. Wang et al. (Citation2019) selected the NDVI as the optimum descriptor of vegetation compared with other normalized vegetation parameters, such as the enhanced vegetation index (EVI) and fraction of photosynthetically active radiation (FPAR). Based on the statistical metrics by Wang et al. (Citation2019), the inversion of WCM using the NDVI induced an RMSE value equal to 8.8 vol.% and a bias of − 0.2 vol.%. Bhogapurapu et al. (Citation2022) investigated the potential of the NDVI and the Sentinel-1 copolarized purity parameter () as crop descriptors to estimate the surface soil moisture. The use of
to estimate the soil moisture was characterized by RMSE value equal to 5.5 vol.% despite a value of 7.6 vol.% using the NDVI over croplands. In semi-arid region, Ouaadi et al. (Citation2020) estimated the aboveground biomass (AGB) and vegetation water content (VWC) using the empirical relationships between in situ measurements and the Sentinel-1 polarization ratio and coherence. The retrieved variables were used as vegetation descriptors in the WCM to estimate the surface soil moisture. By comparing the potential of the NDVI and the derived AGB and VWC from the polarization ratio over the Kairouan site, a similar accuracy of the soil water content estimation was observed. The RMSE and bias values were lower than 8 vol.% and 2 vol.%, respectively.
Despite the potential of the normalized index to detect sowing events in Rolle et al. (Citation2022), the integration of IN in soil moisture retrieval is characterized by a moderate RMSE value of 6.7 vol.% and a correlation coefficient of 0.53. This performance may be due to a characteristic of the normalized ratio, which reduced spatial vegetation variations between fields. Based on the aforementioned results, the relationships between the optical and SAR-derived information must be further investigated according to the wheat growth phases. This investigation focuses on the effect of vegetation volume scattering on the process of soil moisture estimation. In further steps, we aim to test the potential of other SAR-vegetation descriptors such as the interferometric coherence and the polarimetric parameters which can correct the vegetation canopy effects in the surface soil moisture retrieval using radar data. Additionally, one of the limitations of the developed approach is the consideration of constant roughness parameters to estimate the soil moisture. This assumption is derived from ground measurements and previous studies over wheat fields in semi-arid context (Bousbih et al., Citation2017; Ouaadi et al., Citation2020, Citation2021) which highlighted that the small variations in the range of Hrms during wheat growth seasons. Therefore, the developed method of surface soil moisture retrieval can be transposable in the southern Mediterranean regions over wheat fields.
Conclusions
In this work, we retrieved the surface soil moisture at a depth of five cm over wheat fields in the Kairouan Plain. We used the Sentinel-1 data to calculate the backscattering coefficients, the polarization ratio and its normalization and the Sentinel-2 data to calculate the NDVI. To estimate the soil moisture, the WCM coupled with the modified integral equation model was calibrated using 70% of the dataset and validated by 30% of the dataset. We estimate the soil moisture using the look-up table. The results highlighted the importance of the derived SAR ratio in linear scale () and the normalized index IN as alternatives for the NDVI to retrieve the soil moisture at the field scale. The RMSE of soil moisture retrieval ranged between 5.58 and 7.45 vol.% using the different variables (NDVI,
and IN). In the case of the Kairouan Plain,
and IN can be considered a reliable tool to retrieve the soil moisture when the NDVI values are lower than 0.7 and higher than 0.25. These Sentinel-1-derived data may be a solution for vegetation description with full gap-free time series during cloud presence, especially in temperate and tropical climate regions. In the context of future missions such as NISAR and ROSE-L, SAR acquisitions in the L-band will be available. Hence, the description of vegetation volume scattering using L-band data will be further investigated. Consequently, the correction of the vegetation effect will be more precise and reliable using the L-band data than the C-band case.
Acknowledgments
This research has been supported by the European Space Agency Climate Change Initiative for Soil Moisture (grant 4000126684/19/I-NB), the Viana (ERA-Net ARIMNet) Project, the PHC Utique IPASS Project, the Chaams (ERANET3-602 CHAAMS) Project, the Irrigation+ ((ESA n◦4000129870/20/I-NB) Project; the TAPAS TOSCA/CNES project and H2020 ACCWA project. We would like to thank the ARTS program/IRD for awarding a PhD scholarship to Emna Ayari. We extend our warm thanks to the technical teams at the IRD and INAT (Institut National Agronomique de Tunisie) who participated in the ground truth measurement campaigns and data processing.
Disclosure statement
No potential conflict of interest was reported by the author(s).
Data availability statement
The data that support the findings of this study are available from the co-author, Mehrez Zribi, upon reasonable request.
References
- Albergel, C., Rüdiger, C., Carrer, D., Calvet, J. C., Fritz, N., Naeimi, V., Bartalis, Z., & Hasenauer, S. (2009). An evaluation of ASCAT surface soil moisture products with in-situ observations in Southwestern France. Hydrology and Earth System Sciences, 13(2), 115–13. https://doi.org/10.5194/hess-13-115-2009
- Al Bitar, A., Mialon, A., Kerr, Y. H., Cabot, F., Richaume, P., Jacquette, E., Quesney, A., Mahmoodi, A., Tarot, S., Parrens, M., Al-Yaari, A., Pellarin, T., Rodriguez-Fernandez, N., & Wigneron, J. P. (2017). The global SMOS level 3 daily soil moisture and brightness temperature maps. Earth System Science Data, 9(1), 293–315. https://doi.org/10.5194/essd-9-293-2017
- Amazirh, A., Merlin, O., Er-Raki, S., Gao, Q., Rivalland, V., Malbeteau, Y., Khabba, S., & Escorihuela, M. J. (2018). Retrieving surface soil moisture at high spatio-temporal resolution from a synergy between Sentinel-1 radar and Landsat thermal data: A study case over bare soil. Remote Sensing of Environment, 211, 321–337. https://doi.org/10.1016/j.rse.2018.04.013
- Amri, R., Zribi, M., Chabaane, Z. L., Wagner, W., Member, S., & Hasenauer, S. (2012). Analysis of C-Band scatterometer moisture estimations derived over a semiarid region. IEEE Transactions on Geoscience & Remote Sensing, 50(7), 2630–2638. https://doi.org/10.1109/TGRS.2012.2186458
- Attema, E. P. W., & Ulaby, F. T. (1978). Vegetation modeled as a water cloud. Radio Science, 13(2), 357–364. https://doi.org/10.1029/RS013i002p00357
- Aubert, M., Baghdadi, N. N., Zribi, M., Ose, K., el Hajj, M., Vaudour, E., & Gonzalez-Sosa, E. (2013). Toward an operational bare soil moisture mapping using terrasar-x data acquired over agricultural areas. IEEE Journal of Selected Topics in Applied Earth Observations & Remote Sensing, 6(2), 900–916. https://doi.org/10.1109/JSTARS.2012.2220124
- Ayari, E., Kassouk, Z., Lili-Chabaane, Z., Baghdadi, N., Bousbih, S., & Zribi, M. (2021). Cereal crops soil parameters retrieval using L-band ALOS-2 and C-band sentinel-1 sensors. Remote Sens (Basel), 13(7), 1393. https://doi.org/10.3390/rs13071393
- Baghdadi, N., Abou Chaaya, J., & Zribi, M. (2011). Semiempirical calibration of the integral equation model for SAR data in C-Band and cross polarization using radar images and field measurements. IEEE Geoscience and Remote Sensing Letters, 8(1), 14–18. https://doi.org/10.1109/LGRS.2010.2050054
- Baghdadi, N., El Hajj, M., Zribi, M., & Bousbih, S. (2017). Calibration of the water cloud model at C-Band for Winter crop fields and grasslands 1–13. Remote Sensing, 9(9), 969. https://doi.org/10.3390/rs9090969
- Baghdadi, N., Holah, N., & Zribi, M. (2006). Calibration of the integral equation model for SAR data in C-band and HH and VV polarizations. International Journal of Remote Sensing, 27(4), 805–816. https://doi.org/10.1080/01431160500212278
- Baghdadi, N., & Zribi, M. (2006). Evaluation of radar backscatter models IEM, OH and Dubois using experimental observations. International Journal of Remote Sensing, 27(18), 3831–3852. https://doi.org/10.1080/01431160600658123
- Bhogapurapu, N., Dey, S., Homayouni, S., Bhattacharya, A., & Rao, Y. S. (2022). Field-scale soil moisture estimation using sentinel-1 GRD SAR data. Advances in Space Research, 70(12), 3845–3858. https://doi.org/10.1016/j.asr.2022.03.019
- Bousbih, S., Zribi, M., Hajj, M. E., Baghdadi, N., Lili-Chabaane, Z., Gao, Q., & Fanise, P. (2018). Soil moisture and irrigation mapping in a semi-arid region, based on the Synergetic use of Sentinel-1. Remote Sens (Basel) 22. Remote Sensing, 10(12), 1953. https://doi.org/10.3390/rs10121953
- Bousbih, S., Zribi, M., Lili-Chabaane, Z., Baghdadi, N., El Hajj, M., Gao, Q., & Mougenot, B. (2017). Potential of sentinel-1 radar data for the assessment of soil and cereal cover parameters. Sensors (Switzerland), 17(11), 2617. https://doi.org/10.3390/s17112617
- Brocca, L., Ciabatta, L., Massari, C., Camici, S., & Tarpanelli, A. (2017). Soil moisture for hydrological applications: Open questions and new opportunities. Water, 9(2), 140. https://doi.org/10.3390/w9020140
- Brocca, L., Melone, F., Moramarco, T., Wagner, W., Naeimi, V., Bartalis, Z., & Hasenauer, S. (2010). Improving runoff prediction through the assimilation of the ASCAT soil moisture product. Hydrology and Earth System Sciences, 14(10), 1881–1893. https://doi.org/10.5194/hess-14-1881-2010
- Chauhan, S., Srivastava, H. S., & Patel, P. (2018). Wheat crop biophysical parameters retrieval using hybrid-polarized RISAT-1 SAR data. Remote Sensing of Environment, 216, 28–43. https://doi.org/10.1016/j.rse.2018.06.014
- Chen, F., Crow, W. T., Bindlish, R., Colliander, A., Burgin, M. S., Asanuma, J., & Aida, K. (2018). Global-scale evaluation of SMAP, SMOS and ASCAT soil moisture products using triple collocation. Remote Sensing of Environment, 214, 1–13. https://doi.org/10.1016/j.rse.2018.05.008
- Choker, M., Baghdadi, N., Zribi, M., el Hajj, M., Paloscia, S., Verhoest, N. E. C., Lievens, H., & Mattia, F. (2017). Evaluation of the oh, Dubois and IEM backscatter models using a large dataset of SAR data and experimental soil measurements. Water, 9(1), 38. https://doi.org/10.3390/w9010038
- El Hajj, M., Baghdadi, N., Belaud, G., Zribi, M., Cheviron, B., Courault, D., Hagolle, O., & Charron, F. (2014). Irrigated grassland monitoring using a time series of TerraSAR-X and COSMO-SkyMed X-Band SAR data. Remote Sensing, 6(10), 10002–10032. https://doi.org/10.3390/rs61010002
- El Hajj, M., Baghdadi, N., & Zribi, M. (2019). Comparative analysis of the accuracy of surface soil moisture estimation from the C- and L-bands. Int. J. Appl. Earth Obs.Geoinf, 82, 101888. https://doi.org/10.1016/j.jag.2019.05.021
- El Hajj, M., Baghdadi, N., Zribi, M., & Bazzi, H. (2017). Synergic use of Sentinel-1 and Sentinel-2 images for operational soil moisture mapping at high spatial resolution over agricultural areas. Remote Sensing, 9(12), 1–28. https://doi.org/10.3390/rs9121292
- El Hajj, M., Baghdadi, N., Zribi, M., Belaud, G., Cheviron, B., Courault, D., & Charron, F. (2016). Soil moisture retrieval over irrigated grassland using X-band SAR data. Remote Sensing of Environment, 176, 202–218. https://doi.org/10.1016/j.rse.2016.01.027
- El Hajj, M., Baghdadi, N., Zribi, M., Rodríguez-Fernández, N., Wigneron, J. P., Al-Yaari, A., Al Bitar, A., Albergel, C., & Calvet, J. C. (2018). Evaluation of SMOS, SMAP, ASCAT and Sentinel-1 soil moisture products at sites in Southwestern France. Remote Sensing, 10(4), 1–17. https://doi.org/10.3390/rs10040569
- Entekhabi, D., Yueh, S. H., O’neill, P. E., Kellogg, K. H., Allen, A. M., Bindlish, R., Brown, M., Chan, S. T., Colliander, A., Crow, W. T., Das, N. N., Lannoy, G. D., Dunbar, R. S., Edelstein, W. N., Entin, J. K., Escobar, V. M., Goodman, S. D., Jackson, T. J., & West, R. D. (2014). SMAP handbook. JPL Publication, JPL 400–1567.
- ESA. (2012). Sentinel-1: ESA’s radar observatory mission for GMES operational services. ESA Special Publication.
- Ezzahar, J., Ouaadi, N., Zribi, M., Elfarkh, J., Aouade, G., Khabba, S., Er-Raki, S., Chehbouni, A., & Jarlan, L. (2019). Evaluation of backscattering models and support vector machine for the retrieval of bare soil moisture from sentinel-1 data. Remote Sensing, 12(1), 1–20. https://doi.org/10.3390/RS12010072
- Fascetti, F., Pierdicca, N., & Pulvirenti, L. (2017). Empirical fitting of forward backscattering models for multitemporal retrieval of soil moisture from radar data at L-band. Journal of Applied Remote Sensing, 11(1), 016002. https://doi.org/10.1117/1.jrs.11.016002
- Fontanelli, G., Paloscia, S., Zribi, M., & Chahbi, A. (2013). Sensitivity analysis of X-band SAR to wheat and barley leaf area index in the Merguellil Basin. Remote Sensing Letters, 4(11), 1107–1116. https://doi.org/10.1080/2150704X.2013.842285
- Fung, A. K., Li, Z., & Chen, K. S. (1992). Backscattering from a randomly rough dielectric surface. IEEETransactions on Geoscience and Remote Sensing, 30(2), 356–369. https://doi.org/10.1109/36.134085
- Gao, Q., Zribi, M., Escorihuela, M. J., & Baghdadi, N. (2017). Synergetic use of sentinel-1 and sentinel-2 data for soil moisture mapping at 100 m resolution. Sensors (Switzerland), 17(9), 1966. https://doi.org/10.3390/s17091966
- Gorrab, A., Zribi, M., Baghdadi, N., Mougenot, B., Fanise, P., & Lili-Chabaane, Z. (2015). Retrieval of both soil moisture and texture using TerraSAR-X images. Remote Sensing, 7(8), 10098–10116. https://doi.org/10.3390/rs70810098
- Hagolle, O., Huc, M., Descardins, C., Auer, S., & Richter, R. (2017). MAJA Algorithm Theoretical Basis Document (1.0). Zenodo. https://zenodo.org/record/1209633
- Hamze, M., Baghdadi, N., el Hajj, M. M., Zribi, M., Bazzi, H., Cheviron, B., & Faour, G. (2021). Integration of L-Band derived soil roughness into a bare soil moisture retrieval approach from C-Band SAR data. Remote Sensing, 13(11), 2102. https://doi.org/10.3390/rs13112102
- Hosseini, M., & McNairn, H. (2017). Using multi-polarization C- and L-band synthetic aperture radar to estimate biomass and soil moisture of wheat fields. International Journal of Applied Earth Observation and Geoinformation, 58, 50–64. https://doi.org/10.1016/j.jag.2017.01.006
- Kerr, Y. H., Waldteufel, P., Wigneron, J. -P., Delwart, S., Cabot, F., Boutin, J., Escorihuela, M. -J., Font, J. et al. 2010. The SMOS Mission : New Tool for Monitoring Key Elements of the Global Water Cycle. Proceedings of the IEEE, 98, 666–685. http://doi.org/10.1109/JPROC.2010.2043032
- Kerr, Y. H., Waldteufel, P., Wigneron, J.-P., Martinuzzi, J., Font, J., & Berger, M. (2001). Soil moisture retrieval from space: The soil moisture and Ocean Salinity (SMOS) mission. IEEE Transactions on Geoscience and Remote Sensing, 39(8), 1729–1735. https://doi.org/10.1109/36.942551
- Koster, R. D., Brocca, L., Crow, W. T., Burgin, M. S., & de Lannoy, G. J. M. (2016). Precipitation estimation using L-band and C-band soil moisture retrievals. Water Resources Research, 52(9), 7213–7225. https://doi.org/10.1002/2016WR019024
- Kumar, P., Prasad, R., Choudhary, A., Gupta, D. K., Mishra, V. N., Vishwakarma, A. K., Singh, A. K., & Srivastava, P. K. (2019). Comprehensive evaluation of soil moisture retrieval models under different crop cover types using C-band synthetic aperture radar data. Geocarto International, 34(9), 1022–1041. https://doi.org/10.1080/10106049.2018.1464601
- Kumar, K., Suryanarayana Rao, H. P., & Arora, M. K. (2015). Study of water cloud model vegetation descriptors in estimating soil moisture in Solani catchment. Hydrological Processes, 29(9), 2137–2148. https://doi.org/10.1002/hyp.10344
- Lievens, H., & Verhoest, N. E. C. (2011). On the retrieval of soil moisture in wheat fields from L-band SAR based on water cloud modeling, the IEM, and effective roughness parameters. IEEE Geoscience and Remote Sensing Letters, 8(4), 740–744. https://doi.org/10.1109/LGRS.2011.2106109
- Liu, C., & Shi, J. (2016). Estimation of vegetation parameters of water cloud model for Global soil moisture retrieval using time-series L-Band aquarius Observations. IEEE Journal of Selected Topics in Applied Earth Observations & Remote Sensing, 9(12), 5621–5633. https://doi.org/10.1109/JSTARS.2016.2596541
- Ma, C., Li, X., & McCabe, M. F. (2020). Retrieval of high-resolution soil moisture through combination of Sentinel-1 and Sentinel-2 data. Remote Sensing, 12(14), 12. https://doi.org/10.3390/rs12142303
- Mandal, D., Kumar, V., Ratha, D., Dey, S., Bhattacharya, A., Lopez-Sanchez, J. M., McNairn, H., & Rao, Y. S. (2020). Dual polarimetric radar vegetation index for crop growth monitoring using sentinel-1 SAR data. Remote Sensing of Environment, 247, 111954. https://doi.org/10.1016/j.rse.2020.111954
- Massari, C., Modanesi, S., Dari, J., Gruber, A., De Lannoy, G. J. M., Girotto, M., Quintana-Seguí, P., Le Page, M., Jarlan, L., Zribi, M., Ouaadi, N., Vreugdenhil, M., Zappa, L., Dorigo, W., Wagner, W., Brombacher, J., Pelgrum, H., Jaquot, P., Freeman, V. et al. (2021). A review of irrigation information retrievals from space and their utility for users. Remote Sensing, 13(20), 1–26. https://doi.org/10.3390/rs13204112
- Mohanty, B. P., Cosh, M. H., Lakshmi, V., & Montzka, C. (2017). Soil moisture Remote Sensing: State-of-the-Science. Vadose Zone Journal, 16(1), 1–9. vzj2016.10.0105. https://doi.org/10.2136/vzj2016.10.0105
- Ouaadi, N., Ezzahar, J., Khabba, S., Er-Raki, S., Chakir, A., Ait Hssaine, B., le Dantec, V., Rafi, Z., Beaumont, A., Kasbani, M., & Jarlan, L. (2021). C-band radar data and in situ measurements for the monitoring of wheat crops in a semi-arid area (center of Morocco). Earth System Science Data, 13(7), 3707–3731. https://doi.org/10.5194/essd-13-3707-2021
- Ouaadi, N., Jarlan, L., Ezzahar, J., Zribi, M., Khabba, S., Bouras, E., Bousbih, S., & Frison, P.-L. (2020). Monitoring of wheat crops using the backscattering coefficient and the interferometric coherence derived from Sentinel-1 in semi-arid areas. Remote Sensing of Environment, 251, 112050. https://doi.org/10.1016/j.rse.2020.112050
- Panciera, R., Tanase, M. A., Lowell, K., & Walker, J. P. (2014). Evaluation of IEM, Dubois, and oh radar backscatter models using airborne L-Band SAR. IEEE Transactions on Geoscience and Remote Sensing, 52(8), 4966–4979. https://doi.org/10.1109/TGRS.2013.2286203
- Park, S. E., Jung, Y. T., Cho, J. H., Moon, H., & Han, S. H. (2019). Theoretical evaluation of water cloud model vegetation parameters. Remote Sensing, 11(8), 894. 11(8. https://doi.org/10.3390/rs11080989
- Peng, J., Albergel, C., Balenzano, A., Brocca, L., Cartus, O., Cosh, M. H., Crow, W. T., Dabrowska-Zielinska, K., Dadson, S., Davidson, M. W. J., de Rosnay, P., Dorigo, W., Gruber, A., Hagemann, S., Hirschi, M., Kerr, Y. H., Lovergine, F., Mahecha, M. D. … Loew, A. (2021). A roadmap for high-resolution satellite soil moisture applications – confronting product characteristics with user requirements. Remote Sensing of Environment, 252, 112162. https://doi.org/10.1016/j.rse.2020.112162
- Periasamy, S. (2018). Significance of dual polarimetric synthetic aperture radar in biomass retrieval: An attempt on Sentinel-1. Remote Sensing of Environment, 217, 537–549. https://doi.org/10.1016/j.rse.2018.09.003
- Prakash, R., Singh, D., & Pathak, N. P. (2012). A fusion approach to retrieve soil moisture with SAR and optical data. IEEE Journal of Selected Topics in Applied Earth Observations & Remote Sensing, 5(1), 196–206. https://doi.org/10.1109/JSTARS.2011.2169236
- Rolle, M., Tamea, S., Claps, P., Ayari, E., Baghdadi, N., & Zribi, M. (2022). Analysis of maize sowing periods and cycle phases using Sentinel 1&2 data synergy. Remote Sensing, 14(15), 3712. https://doi.org/10.3390/rs14153712
- Schilling, J., Hertig, E., Tramblay, Y., & Scheffran, J. (2020). Climate change vulnerability, water resources and social implications in North Africa. Regional Environmental Change, 20(1). https://doi.org/10.1007/s10113-020-01597-7
- Shabou, M., Mougenot, B., Chabaane, Z. L., Walter, C., Boulet, G., Aissa, N. B., & Zribi, M. (2015). Soil clay content mapping using a time series of Landsat TM data in semi-arid lands. Remote Sensing, 7(5), 6059–6078. https://doi.org/10.3390/rs70506059
- Shi, J., Wang, J., Hsu, A., O’Neill, P., & Engman, E. T. (1995). Estimation of soil moisture and surface roughness parameters using L-band SAR measurements. International Geoscience and Remote Sensing Symposium (IGARSS), 1, 507–509. https://doi.org/10.1109/igarss.1995.520322
- Shi, J., Wang, J., Hsu, A. Y., O’Neill, P. E., & Engman, E. T. (1997). Estimation of bare surface soil moisture and surface roughness parameter using L-band SAR image data. IEEE Transactions on Geoscience and Remote Sensing, 35(5), 1254–1266. https://doi.org/10.1109/36.628792
- Tao, L., Li, J., Jiang, J., He, S., Cai, Q., & Chen, X. (2015). Evaluation of radar backscattering models using L- and C-band synthetic aperture radar data. Journal of Applied Remote Sensing, 9(1), 094091. https://doi.org/10.1117/1.jrs.9.094091
- Tramblay, Y., & Somot, S. (2018). Future evolution of extreme precipitation in the Mediterranean. Climatic Change, 151(2), 289–302. https://doi.org/10.1007/s10584-018-2300-5
- Ulaby, F. T. (1975). Radar response to vegetation. IEEE Transactions on Antennas & Propagation, 23(1), 36–45. https://doi.org/10.1109/TAP.1975.1140999
- Ulaby, F. T., Allen, C. T., Eger, G., & Kanemasu, E. (1984). Relating the microwave backscattering coefficient to leaf area index. Remote Sensing of Environment, 14(1–3), 113–133. https://doi.org/10.1016/0034-4257(84)90010-5
- Ulaby, F. T., Sarabandi, K., Mcdonald, K., Whitt, M., & Craig, M. (1990). Michigan Microwave Canopy Scattering Model. International Journal of Remote Sensing, 11(7), 1223–1253. https://doi.org/10.1080/01431169008955090
- Veloso, A., Mermoz, S., Bouvet, A., le Toan, T., Planells, M., Dejoux, J. F., & Ceschia, E. (2017). Understanding the temporal behavior of crops using Sentinel-1 and Sentinel-2-like data for agricultural applications. Remote Sensing of Environment, 199, 415–426. https://doi.org/10.1016/j.rse.2017.07.015
- Wagner, W., Blöschl, G., Pampaloni, P., Calvet, J. C., Bizzarri, B., Wigneron, J. P., & Kerr, Y. (2007). Operational readiness of microwave remote sensing of soil moisture for hydrologic applications. Hydrology Research, 38(1), 1–20. https://doi.org/10.2166/nh.2007.029
- Wagner, W., Hahn, S., Kidd, R., Melzer, T., Bartalis, Z., Hasenauer, S., Figa-Saldaña, J., de Rosnay, P., Jann, A., Schneider, S., Komma, J., Kubu, G., Brugger, K., Aubrecht, C., Züger, J., Gangkofner, U., Kienberger, S., Brocca, L. … Rubel, F. (2013). The ASCAT soil moisture product: A review of its specifications, validation results, and emerging applications. Meteorologische Zeitschrift, 22(1), 5–33. https://doi.org/10.1127/0941-2948/2013/0399
- Walker, J. P., Willgoose, G. R., & Kalma, J. D. (2004). In situ measurement of soil moisture: A comparison of techniques. Journal of Hydrology, 293(1–4), 85–99. https://doi.org/10.1016/j.jhydrol.2004.01.008
- Wang, L., He, B., Bai, X., & Xing, M. (2019). Assessment of different vegetation parameters for parameterizing the coupled water cloud model and advanced integral equation model for soil moisture retrieval using time series Sentinel-1A data. Photogrammetric Engineering & Remote Sensing, 85(1), 43–54. https://doi.org/10.14358/PERS.85.1.xxx
- Wang, H., Magagi, R., & Goïta, K. (2018). Potential of a two-component polarimetric decomposition at C-band for soil moisture retrieval over agricultural fields. Remote Sensing of Environment, 217, 38–51. https://doi.org/10.1016/j.rse.2018.08.003
- Wang, Z., Zhao, T., Qiu, J., Zhao, X., Li, R., & Wang, S. (2021). Microwave-based vegetation descriptors in the parameterization of water cloud model at L-band for soil moisture retrieval over croplands. GIScience & Remote Sensing, 58(1), 48–67. https://doi.org/10.1080/15481603.2020.1857123
- Wang, Z., Zhao, T., Shi, J., Wang, H., Ji, D., Yao, P., Zheng, J., Zhao, X., & Xu, X. (2023). 1-km soil moisture retrieval using multi-temporal dual-channel SAR data from Sentinel-1 A/B satellites in a semi-arid watershed. Remote Sensing of Environment, 284, 284. https://doi.org/10.1016/j.rse.2022.113334
- Zribi, M., Chahbi, A., Shabou, M., Lili-Chabaane, Z., Duchemin, B., Baghdadi, N., Amri, R., & Chehbouni, A. (2011). Soil surface moisture estimation over a semi-arid region using ENVISAT ASAR radar data for soil evaporation evaluation. Hydrology and Earth System Sciences, 15(1), 345–358. https://doi.org/10.5194/hess-15-345-2011
- Zribi, M., Gorrab, A., & Baghdadi, N. (2014). A new soil roughness parameter for the modelling of radar backscattering over bare soil. Remote Sensing of Environment, 152, 62–73. https://doi.org/10.1016/J.RSE.2014.05.009
- Zribi, M., Muddu, S., Bousbih, S., Al Bitar, A., Tomer, S. K., Baghdadi, N., & Bandyopadhyay, S. (2019). Analysis of L-band SAR data for soil moisture estimations over agricultural areas in the tropics. Remote Sensing, 11(9), 11. https://doi.org/10.3390/rs11091122