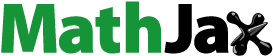
ABSTRACT
This article presents models to predict the time until mechanical failure of in-ground wooden test specimens resulting from fungal decay. Historical records of decay ratings were modelled by remotely sensed data from ERA5-Land. In total, 2,570 test specimens of 16 different wood species were exposed at 21 different test sites, representing three continents and climatic conditions from sub-polar to tropical, spanning a period from 1980 until 2022. To obtain specimen decay ratings over their exposure time, inspections were conducted in mostly annual and sometimes bi-annual intervals. For each specimen’s exposure period, a laboratory developed dose–response model was populated using remotely sensed soil moisture and temperature data retrieved from ERA5-Land. Wood specimens were grouped according to natural durability rankings to reduce the variability of in-ground wood decay rate between wood species. Non-linear, sigmoid-shaped models were then constructed to describe wood decay progression as a function of daily accumulated exposure to soil moisture and temperature conditions (dose). Dose, a mechanistic weighting of daily exposure conditions over time, generally performed better than exposure time alone as a predictor of in-ground wood decay progression. The open-access availability of remotely sensed soil-state data in combination with wood specimen data proved promising for in-ground wood decay predictions.
Introduction
From a climate change mitigation perspective, there is a strong interest to prioritize the use of renewable, wood-based (bio-based) building materials. However, while wood-based building materials may be attractive to end-users for their climate-conscious production footprint, they do unfortunately suffer in their longevity. This means that they are susceptible to natural deterioration over time by biotic and abiotic means.
The two greatest influences on the decay hazard of wood are temperature and moisture, and it has been recognized for centuries that exposed wood deteriorates more rapidly in warm, wet climates than in cold, dry climates (Lebow & Highley, Citation2008). Some well-known uses of wood in contact with soil (i.e. use class 4 according to EN 335 (Citation2013)) include railway sleepers (ties), transmission and fence poles, foundation pilings in unstable soils, as well as in-water constructions such as jetties, bridges, and quay/channel walls (Klaassen & Creemers, Citation2012; Marais et al., Citation2020). Wooden components exposed in-ground suffer severe exposure conditions due to the combination of potential attack from both insects and wood decaying fungi through suitable soil moisture and temperature conditions. Wood decay is a major contributor to failures of in-service wood; replacing decayed wood and wood-based materials accounts for 10% of the global lumber market (Morrell, Citation2018).
The recognition of regional variation in decay rate of in-ground wooden components, and its importance in predicting the usable lifespan (i.e. service life) of such components, has led to several efforts to develop maps delineating quantifiable hazard zones. These would assist wood users by providing a clear quantitative overview of the wood decay rate expected at a chosen location and where it might make sense to employ either a highly durable wood species or a chemical preservative treatment to enhance the durability of a non-durable wood species.
Available since 2007, efforts to develop models of regional decay hazards for Australia were published in technical manuals relevant to TimberLife, a timber service life design guide software package (MacKenzie et al., Citation2007). The estimation of the progress of decay (i.e. decay depth) considers the effects of the natural durability of wood, climate condition of a site, preservative treatment, and internal/external maintenance applications. Concerning the climate conditions of the site, the in-ground model was a function of average annual temperature and rainfall. To account for soil moisture, an additional factor was added for the number of months in a year in which rainfall was less than 5 mm (Wang et al., Citation2008). Following on from TimberLife, a dose–response concept sought to improve estimations of decay depth and therefore service life prediction with a sigmoid-shaped dose–response model, presented in Brischke and Rapp (Citation2008). The model describes the relationship between exposure conditions using wood moisture content and wood temperature (i.e. dose), and the resultant aboveground wood decay (i.e. response). Exposure conditions can be measured directly, or be inferred from weather data.
Recently, Marais et al. (Citation2021) developed a laboratory-based dose–response model to describe the effect of soil temperature, soil moisture content, and soil water-holding capacity (dose) on wood decay (response). These data are today readily available from remotely sensed sources, which makes connecting the laboratory-derived dose–response model to in-ground field trial specimens or even larger wooden infrastructure components possible.
Van Niekerk et al. (Citation2022) were able to successfully integrate remotely sensed soil moisture data and soil surface temperature data, with service life modelling approaches using dose (Marais et al., Citation2021). In this context, dose was calculated for the measuring period of March 2015 until October 2021, from which an average exposure year was constructed to present relative in-ground wood decay risk over Europe in the form of hazard maps. Therefore, these maps present average annual in-ground wood decay risk as a function of exposure to soil moisture and temperature conditions, which are understood to be prominent mechanistic drivers of in-ground wood decay.
Many studies investigating fungal wood decay have aimed to validate specific hypotheses, led by mechanistic understandings (i.e. models) focussed on the causality of input–output relationships. For example, the countless isolated studies investigating the effect of temperature, moisture, or fungal species on wood decay (A’Bear et al., Citation2014; Gonzales & Morrell, Citation2012; Hiscox et al., Citation2016; Morrell & Zabel, Citation1985; Morton & Eggins, Citation1977; Venugopal et al., Citation2016) – an exhaustive review can also be found in Marais et al. (Citation2020). As mentioned by Baker et al. (Citation2018), a mechanistic model’s purpose is to mimic real-life events through assumptions of the prominent underlying mechanisms. To this end, appropriate mechanistic models can be applied to larger, already existing datasets to evaluate their suitability to describe wood decay, specifically.
The objectives of the present study can be defined as the following:
To evaluate the suitability of a laboratory-derived dose–response model (Marais et al., Citation2021), as the predictor variable in modelling mean fungal decay progression of in-ground wooden specimens.
To illustrate the exploitation of already existing wood decay datasets by using remotely sensed data sources to retroactively populate in-situ soil-state data not originally captured at the time of exposure.
Materials and methods
In-situ measured wood decay progression
In-ground wood decay test data from the International Research Group on Wood Protection’s (IRG-WP) durability database (IRG/WP, Citation2013) were used. The records of wood specimens included dates of inspection and the measured state of decay progression, known as decay rating. At the time of our analysis (April 2022), the database contained a sum of 16,128 observations of in-ground wood decay, resulting from 2,570 individual untreated wood specimens, 16 different wood species, exposed at 21 different field test sites (). The observations were ordered chronologically spanning a time period from 1980 until 2022. The location coordinates of each test site can be found in Supplementary Table 1.
Figure 1. Map of all 21 field test sites (points) where wood specimens were exposed in ground contact for use in this study. Some sites in Germany were located too close together to be clearly discerned at this map’s scale.

Specimens in the durability database were tested and rated according to two standards, EN 252 (Citation2015), shown in Supplementary Table 2, and AWPA E7–15 (Citation2015), shown in Supplementary Table 3. The European standard, EN 252 (Citation2015), uses a scale of decay rating (i.e. decay depth judged by a pick rating) from 0 to 4, while the North American standard, AWPA E7 (Citation2015), uses a scale from 10 to 0. To allow for common analysis, decay ratings according to AWPA E7 (Citation2015) were transformed to the EN 252 (Citation2015) standard, following the recoding shown in Supplementary Table 4.
Since it is understood that fungal wood decay is an irreversible chemical process, decay ratings should steadily increase over time. However, some instances of decreasing ratings (i.e. negative or “healing” ratings) over time were observed. Decay ratings are a measure of the depth in material surface softening, i.e. a measure of sound wood versus decayed wood. Therefore, numerous factors can influence a specimen’s decay rating ranging from material properties, such as surface hardness and moisture content, to season of inspection, assessor bias, and simply even mistaken or incorrect data entry. These decreasing ratings were corrected by the rule stating that each record needs to be larger or equal to the maximum of all previous records.
Once decreasing ratings were corrected, these synchronized values of wood decay representing individual wood specimens were then used to calculate mean decay ratings for wood specimen groups. The groups consisted of replicate wood specimens of the same wood species, installed on the same date at the same study site. The replicates also underwent subsequent decay rating inspections as a group on similar dates thereafter. This resulted in a total of 696 mean decay rating observations.
Wood species and anatomy (heartwood and sapwood) is known to influence wood decay rate. Early exploration of the full wood decay dataset showed high variability in mean decay progression over time, which in-part, could be attributed to differences in natural durability of the wood species. So, the incorporation of multiple wood species into the models developed in this work meant grouping the 16 different wood species according to similar natural durability classification and expected in-ground wood decay rate. This was achieved by using species-specific coefficients for relative in-ground wood decay rates (vrel), taken from Brischke et al. (Citation2021), shown in . These describe in-ground wood decay rate of a wood material relative to a reference wood material, irrespective of exposure conditions at the study site. The relative decay rates (vrel) in Brischke et al. (Citation2021) were calculated from the materials’ decay rate at the respective field sites relative to the decay rate of a reference wood material. Southern pine sapwood (Pinus spp.) and Norway spruce (Picea abies) were considered the reference species (vrel = 1.00), while species experiencing a higher in-ground decay rate have higher vrel values, and species experiencing a lower in-ground decay rate have lower vrel values. The various studies from which decay data were used to calculate these relative decay rates (vrel) are also listed in Brischke et al. (Citation2021). For the purposes of this study, the following three ranges in vrel were used to group wood species of similar performance for modelling in-ground wood decay rate as a function of exposure conditions over the respective sites: vrel >1.25; 1.25 > vrel >0.75; vrel <0.75.
Table 1. Species-specific coefficients for in-ground relative decay rates (vrel), taken from Brischke et al. (Citation2021), and used to group wood species according to natural durability in the modelling process. Wood material is assumed to consist of heartwood where no annotation of sapwood (sw) is given. Picea abies includes both heartwood and sapwood (hw & sw).
Specimen data were stored in the IRG-WP durability database (IRG/WP, Citation2013) in the form of PDF-files. Microsoft Excel (Citation2019, 2018) was used to transform these data into common spreadsheets. All subsequent steps of analysis, including merging the data using “dpylr” (Wickham et al., Citation2022), were done using the free programming language R, version 4.2.0 (R Core Team, Citation2022), interfaced with RStudio Team (Citation2022). The packages ggplot2 (Wickham, Citation2016) and ggpubr (Kassambara, Citation2020) were used to construct graphs of the modelling outputs.
In-ground dose–response model to describe soil exposure conditions
Soil moisture and temperature retrievals from ERA5-Land
Global grids of soil moisture content (“volumetric soil water layer 1”, SWC, [%]) and soil temperature (“soil temperature level 1”, Tsoil, [°C]) were downloaded from the ERA5-Land repository (European Commission, Copernicus, ECMWF, & Climate Change Service, Citation2022), spatial resolution grid of 9 km, 0–7 cm soil depth. Although ERA5-Land provides SWC and Tsoil grids at an hourly interval, we only used one grid per day (14:00 UTC), since the resultant temporal resolution was considered adequate for purposes of this study. The daily grids were transformed into a common raster, stacked, and used to extract a time series of SWC and Tsoil corresponding to the exposure period of each of the 2,570 wood specimens exposed at each of the 21 study sites. To make the retrievals of SWC (and Tsoil) conform to the input data requirements of the dose–response model by Marais et al. (Citation2021), entailed calculating variables for soil water-holding capacity (WHCsoil) and soil moisture content (MCsoil).
Following the procedure described by Salomón et al. (Citation2022), we defined the 95th percentile of SWC values at every study site as the WHCsoil at that site. SWC was expressed in percentage of WHCsoil [i.e. %WHCsoil], renamed as MCsoil, and calculated according to EquationEquation 1(1)
(1) .
To capture the time series between inspection intervals, cumulative total daily dose was calculated using the model by Marais et al. (Citation2021). In short, daily dose summarizes the relationship between Tsoil, MCsoil, and WHCsoil. The model is based on dose component values calculated using WHCsoil, and daily values of MCsoil and Tsoil. Marais et al. (Citation2021) used in-situ values of soil temperature and soil moisture to calculate daily dose values, which were accumulated for the period of exposure and compared to measured values of wood decay. In this work, we substituted the in-situ measurements of soil moisture and temperature with the ERA5-Land retrievals of MCsoil and Tsoil to calculate dose component values for moisture (DMC) and temperature (DT). The variable dose, D, was the product of DMC and DT for each day, and accumulated for the total exposure time of wood specimens on each study site, D(n). This meant that all the specimens belonging to the same wood species exposed for the same time period on the same study site, experienced the same exposure dose. That is to say, the same groupings used to calculate mean decay ratings from individual specimens (described above), were then assigned a calculated exposure dose. EquationEquation 2(2)
(2) - Equation5
(5)
(5) below describe the calculation of these exposure dose variables in more detail.
Moisture-induced component
EquationEquation 2(2)
(2) presents a polynomial function for the moisture-induced dose component of the laboratory-derived in-ground dose–response model developed for European beech wood specimens exposed to a reference soil consisting of a mixture of compost and quartz sand. Here, remotely sensed soil moisture values from ERA5-Land were used for WHCsoil and MCsoil, as described by EquationEquation 1
(1)
(1) . For purposes of compatibility with soils outside of the laboratory conditions that the model was developed from, the lower WHCsoil constraint was removed. The lower WHCsoil boundary in Marais et al. (Citation2021) was set to 30% to avoid the use of the model outside of its tested/developed parameters. However, once the model was applied to outdoor conditions (i.e. in this study), it became clear that wood decay still occurred in soils with WHCsoil lower than 30% and hence DMC still accumulated. Additionally, a new constraint had to be introduced to account for this modification of the model in cases where negative DMC values were produced (see EquationEquation 4
(4)
(4) ).
Where: is the moisture-induced dose component comprising a combination of soil water-holding capacity (
) and soil moisture content (
) for the considered day [%];
is the soil water-holding capacity [%];
is the soil moisture content [
];
;
;
;
Temperature-induced component
EquationEquation 3(3)
(3) presents a polynomial function describing the temperature-induced dose component of the in-ground dose–response model (Marais et al., Citation2021). Here, remotely sensed soil temperature values from ERA5-Land were used for Tsoil.
Where:
is the temperature-induced dose component;
is the mean soil temperature for the considered day [°C];
is the minimum allowable soil temperature for the considered day [°C];
is the maximum allowable soil temperature for the considered day [°C];
;
;
;
;
Cumulative total daily dose
EquationEquations 4(4)
(4) and Equation5
(5)
(5) present functions towards cumulative total daily dose, D(n), where the daily dose, D, consisting of the products of the moisture- and temperature-induced components, DMC and DT, are summed for a given exposure period (Marais et al., Citation2021).
Where:
D(n) is the cumulative total daily dose for the considered exposure period [days];
D is the daily dose [-];
DT is the temperature-induced dose component;
Tsoil is the mean soil temperature for the considered day [°C];
DMC is the moisture-induced component comprising of a combination of soil water-holding capacity (WHCsoil) and soil moisture content (MCsoil) for the considered day [%].
Non-linear model fitting
After mean decay ratings were calculated and grouped using vrel, (all described above), model fitting was conducted. A series of non-linear models were fitted using a sigmoidal function to evaluate the suitability of time of exposure of wood specimens [days] and cumulative total daily dose [days], to describe decay progression [mean decay rating]. The sigmoidal function used appeared as follows, Equation (6):
Where:
= mean decay rating;
= asymptote corresponding to mean decay rating of 4;
= independent input variable,
[days] or time of exposure [days];
and
= model coefficients.
EquationEquation 6(6)
(6) was chosen for its ability to start at zero (wood condition at start of exposure), with its asymptote set to 4 (maximum decay rating possible). Models were fitted using the built-in function “nls” in R, version 4.2.0 (R Core Team, Citation2022). Starting values for model coefficients
and
were separately optimized using a combination of visual model fitting and minimizing the sum of squares of residuals using the built-in “solver” function in Microsoft Excel. The result of the optimization of model coefficients was then used as starting values for the “nls” function in R.
Residual standard error (RSE) was used to evaluate the goodness-of-fit of the developed models against one another, EquationEquation 7(7)
(7) . Since the model fitting was non-linear, RSE was chosen over a coefficient-of-determination (R2), which is more suited to linear models to evaluate prediction accuracy. However, in observed vs. predicted plots, R2 was used as model performance metric and was calculated using the caret package (Kuhn, Citation2008) in R, version 4.2.0 (R Core Team, Citation2022).
Where:
= residual standard error;
= actual mean decay rating [0–4];
= predicted decay rating [0–4];
= number of mean decay rating observations.
Results
Exposure conditions of test sites
The 21 different exposure sites tested in this study presented a range in soil exposure conditions. shows characteristic average annual soil exposure data for each study site taken from ERA5-Land, calculated for the time period from 2012 until 2022. All but one of the study sites (Rotorua, New Zealand), were found in the northern hemisphere. Gainesville (USA) showed the highest mean annual temperature (21.4°C), while Maple Ridge (Canada) showed the lowest mean annual temperature (6.85°C). High standard deviation in mean annual soil temperature (4–8°C) was shown for all study sites, except Hawaiian sites (1–2°C), reflecting a high- and low-temperature seasonality, respectively. Sites showing the highest and lowest mean annual soil temperature also showed the highest and lowest annual accumulated temperature dose (218 and 87.9), reflecting the total number of days in an average year where soil temperature conditions suitable for fungal wood decay were achieved.
Table 2. Mean annual soil exposure conditions of the study sites calculated from ERA5-Land retrievals of soil moisture and soil temperature for the time period from 2012 to 2022. In this case, DMC(n) and DT(n) represent the cumulative sum of DMC and DT for an average year.
The test site in Tuusula (Finland) showed the highest WHCsoil (50.4%) and highest mean annual MCsoil (90 ± 8.14%). The Tuusula (Finland) test site also showed the lowest soil moisture dose conditions due to the dose–response model penalizing over-saturated soil moisture conditions, meaning moisture dose was not accumulated if the soil moisture conditions did not comply to the model’s ranges. A similar observation was noted for the test site in Maple Ridge (Canada) and Slovenian sites.
Rotorua (New Zealand) showed the lowest WHCsoil (28.1%), but Gainesville (USA), which showed the warmest mean annual soil temperature conditions, also showed the driest mean annual MCsoil conditions (70.2%). The test site in Saint-Trojan-les-Bains (France) showed the highest annual accumulated moisture dose conditions (73.1). Here, daily soil moisture conditions were moderate enough (i.e. not too wet or too dry) to accumulate a high number of total suitable moisture dose days in a year.
Maple Ridge (Canada) showed the lowest annual accumulated dose (18.3), which reflects the cold and wet conditions that limit fungal wood decay. The same observation was noted for the Tuusula (Finland) site (20.1). Again, the test site in Saint-Trojan-les-Bains (France) showed the highest cumulative total daily dose (45.4), reflecting the moderate, yet suitable, daily soil moisture and temperature conditions over an average year of exposure.
Relationship between exposure time and in-ground wood decay of different wood species
shows the mean decay rating progression over time of exposure for all wood species used in the study. As expected, mean time of exposure until specimen failure (decay rating 4) varied across different wood species, and across different sites. Fagus sylvatica sapwood exhibited the shortest time until failure (2.3 years). Fagus sylvatica sapwood was the least durable wood species included in the study, while Larix sibirica heartwood, the most durable of the wood species tested, showed the longest mean time until failure (10.7 years). The commonly used reference wood species, Fagus sylvatica sapwood, Pinus sylvestris sapwood, and Picea abies, contributed the biggest share of mean decay rating observations to the dataset. Other material such as Pseudotsuga menziesii heartwood also made up a considerable share of the dataset. Differences in time until failure between wood specimen groups belonging to the same wood species can be attributed to differences in soil exposure conditions between exposure sites.
Figure 2. Relationship between mean decay rating progression and time of exposure for different wood species, irrespective of study site. For each wood species, different study sites are represented by the range of different geometric shapes on the decay progression curve. Each point on the decay progression curve of a given wood species represents the mean decay rating at one study site at a certain time of exposure (inspection interval). Wood material is assumed to consist of heartwood where no annotation of sapwood (sw) is given. Picea abies includes both heartwood and sapwood. The mean time until failure (mean decay rating 4) and standard deviation for each wood species is shown by the purple point and error bar.

Relationship between cumulative total daily dose and in-ground wood decay of different wood species
The graphs presented in show the relationship between mean decay ratings and dose (b and d), and mean decay ratings and time of exposure (a and c) to describe in-ground wood decay progression. The vrel metric was used to group mean decay ratings of different wood species into natural durability ranges to create three in-ground wood decay models.
Figure 3. Relationship between the time of exposure and mean decay ratings according to EN 252 (Citation2015) of 16 different wood species exposed across 21 different study sites (a). Relationship between dose and mean decay ratings (b), with mean decay ratings shaded from low (red) to high (black) natural durability classification using vrel. Relationship between mean decay ratings and time of exposure (c), and mean decay ratings and cumulative total daily dose (d). Each dot represents the mean decay rating at one study site for a given wood species at a certain time of exposure (n). Residual standard error (RSE) is given as model performance metric.

plots all mean decay ratings against time of exposure, irrespective of wood species. plots all mean decay ratings grouped according to a scale of low to high natural durability classification using vrel (Low: vrel >1.25; Med: 1.25 > vrel >0.75; High: vrel <0.75). shows models fitted to the plots of mean decay ratings against exposure time and cumulative total daily dose, respectively. The model performance metric RSE is also given in . Cumulative total daily dose, which is a combination of time of exposure and soil conditions linked to that exposure time, showed improved RSE performance over exposure time alone to describe in-ground wood decay progression. Although cumulative total daily dose improved RSE in all three cases, it also produced some new outliers. The full equations of the models in are given in .
Table 3. Non-linear model equations towards predicting mean in-ground wood decay rating [0–4] as a function of soil exposure conditions represented by cumulative total daily dose [days]. The models can accommodate a range of wood species by matching the natural durability metric (vrel) of the wooden material of interest to the appropriate model specification (vrel range). Model performance metrics, Bayesian information criterion (BIC) and residual standard error (RSE) for each model are also given. Degrees of freedom is denoted by df.
plots observed mean decay ratings against decay ratings predicted from models in where exposure period [days] was used to predict decay rating progression [0–4]. The coefficients of determination (R2) of the linear lines of best fit through the point clusters were satisfactory overall, with the specimens grouped according to high natural durability returning the lowest of the three R2 values. The slope and y-intercept of all three lines of best fit were close enough to the idealised 1:1 prediction line that no clear over- or underprediction pattern was observed.
Figure 4. Relationship between observed and predicted mean decay ratings of 16 different wood species exposed across 21 different study sites, evaluated according to EN 252 (Citation2015). Each dot represents the mean decay rating at one study site for a given wood species at a certain time of exposure. Predicted mean decay ratings were calculated using models shown in (not reported), which used exposure time as input.

plots observed mean decay ratings against decay ratings predicted from models in where cumulative total daily dose (D) was used as input (). The coefficients of determination (R2) of the linear lines of best fit were generally higher for cumulative total daily dose (D) than for exposure time. The R2 of dose models showed improved prediction accuracy compared to exposure time models. Again, the slope and y-intercept of the lines were close enough to the idealised 1:1 prediction line that no clear over- or underprediction pattern was observed, with the exception of the medium natural durability class line of best fit, which was strongly influenced by outlier points.
Figure 5. Relationship between observed and predicted mean decay ratings [0–4] of 16 different wood species exposed across 21 different study sites, evaluated according to EN 252 (Citation2015). Each dot represents the mean decay rating at one study site for a given wood species at a certain time of exposure. Predicted mean decay ratings were calculated according to models shown in , which used cumulative total daily dose (D) values calculated from ERA5-Land measurements of soil temperature and soil moisture as input.
![Figure 5. Relationship between observed and predicted mean decay ratings [0–4] of 16 different wood species exposed across 21 different study sites, evaluated according to EN 252 (Citation2015). Each dot represents the mean decay rating at one study site for a given wood species at a certain time of exposure. Predicted mean decay ratings were calculated according to models shown in Table 3, which used cumulative total daily dose (D) values calculated from ERA5-Land measurements of soil temperature and soil moisture as input.](/cms/asset/3c6b268a-c0d0-4cea-8835-3f415979bfd7/tejr_a_2264473_f0005_oc.jpg)
Discussion
Model performance
In models presented across , dose as a predictor variable generally performed better than exposure time alone to describe mean wood decay ratings. This can be seen from the lower RSE metrics in , and the higher R2 metrics from the observed vs predicted plots in and . Compared to exposure time alone, compound model error and measurement error were newly introduced by the dose modelling procedure. Nevertheless, the improved prediction accuracy of dose was expected since the dose–response concept, and therefore the weighting of moisture and temperature conditions in an exposure time series, has already been applied successfully to aboveground wood decay (Brischke & Rapp, Citation2008). Already in earlier studies, Viitanen (Citation1997) and Rapp et al. (Citation2000) postulated towards the application of a mechanistic weighting procedure (i.e. dose) through the identification of wood moisture content, temperature, and relative humidity ranges which influence aboveground fungal wood decay. A similar procedure was followed to identify soil moisture and temperature ranges that influence in-ground wood decay, captured in Marais et al. (Citation2021), and applied to this study.
The use of non-linear modelling techniques delivered three different models applicable to wood species spanning a range in natural durability (here based on vrel). The potential exists to incorporate the models developed in this study into the approach outlined in Brischke et al. (Citation2021) to predict the in-ground wood decay rate of wood species (including modified and treated wood materials) not included in this study.
Laboratory-derived dose–response model to describe soil exposure conditions
Modelling time-series data is complex, therefore the dose–response model employed in this study was seen as a mechanistic informer model, able to describe the exposure time as a function of exposure conditions. points out the benefits of using such a model to consolidate exposure time, where dose generally leads to better prediction accuracy of mean decay rating than exposure time alone. Dose was able to capture the interaction between daily moisture- and temperature conditions between inspection intervals of the specimens. The dose–response model captures the physiological requirements for proliferation of soil-inhabiting micro-organisms, with the assumption that the group of soft-rot fungi are predominantly present and therefore responsible for the observed wood decay. Of course, other fungi belonging to the brown-rot and white-rot groups can and have indeed been identified in countless in-ground wood decay test specimens. However, improvements to the physiological requirement curves of the dose–response model used in this study can still be implemented to improve its prediction accuracy. So-called “activation” or “set-back” functions could be implemented, where decay rate after physiological disruptions, such as excessively high or low temperature, or water-logging, and their inhibitory effect on wood decay rate could be captured (Isaksson et al., Citation2013; Viitanen et al., Citation2010).
Spatial resolution of remotely sensed site data
The application of open-access remotely sensed climate data to wood decay modelling was explored in this study. The use of remotely sensed site data to retroactively populate the laboratory-derived dose–response model (Marais et al., Citation2021) as a predictor variable to model in-ground wood decay progression was shown to be a suitable approach over using only exposure time as predictor variable. Therefore, the prediction of in-ground wood decay at study sites outside of those used in this study is possible, as long as the natural durability of the wood material of interest is known and data regarding soil exposure are available – either from remotely sensed sources or measured in-situ at the site. Some shortfalls of the spatial (and temporal) resolution of remotely sensed site data should, however, be mentioned.
The pros and cons of remotely sensed vs. in-situ measured climate data should be considered before any single data source is decided on. In-situ measurements can provide accurate measurements of local conditions, but their measurements can be sporadic, requiring interpolation where observations are missing (Mendelsohn et al., Citation2007). In-situ measurements are almost always located at a different distance from the site of interest, not to mention the differences in measuring devices and metrics, and the need for device calibration and calibration between datasets. Satellites might deliver problematic measurements for some ground phenomena such as precipitation, but they can provide complete spatial coverage of various parameters over a landscape (Mendelsohn et al., Citation2007). Their data is also distributed over regular grids and if biased, can be corrected systematically. While in-situ climate data is ideal (i.e. like the nature of the wood decay data in this study), this is not always possible at every study site, especially not retrospectively, which also makes remotely sensed datasets as presented in this study better suited to the modelling procedure in general. Nevertheless, it is possible to use in-situ measurements when available and remotely sensed data as a substitute for missing data, either due to sensor outage (to avoid interpolation) or due to a lack of measurements altogether. One source of data does not necessarily exclude another, where in the context of ERA5-Land, climate data are collected from numerous sources (i.e. satellite, aircraft, weather stations, ocean buoys, etc.) and combined into a temporally and spatially accurate data service through a process known as data assimilation and reanalysis. Here, the importance of numerous complementary data sources and measuring locations, all vetting one another, cannot be overemphasized.
The 9 km spatial resolution grid of the ERA5-Land data used in this study showed shortfalls when applied to sites close to bodies of water. The study site in Finland showed the lowest moisture dose, attributable to periods of waterlogging (saturated soil moisture conditions). These points were seen as outliers in both and . Temporal resolution aside, since only one mean soil moisture measurement value is delivered per 9 km grid, a high spatial density of water bodies in a grid can lead to an inflation of the soil moisture measurement value for that 9 km grid. In-ground wood decay test sites are typically placed a fair distance away from water bodies, but their associated remotely sensed data measurement can be skewed or unrealistic for a single non-water body point in that grid. A similar effect can be seen in mountainous regions, where high variation in altitude within a single grid can pose a similar anomaly to measurements of temperature (Brischke & Selter, Citation2020). In future however, the use of further disaggregation techniques such as “depth-to-water” or “topographic wetness index”, should be pursued to improve the spatial resolution of remotely sensed soil moisture observations (Ågren et al., Citation2021; Lidberg et al., Citation2020; Schönauer et al., Citation2022).
This study lacked wood decay sites in central and south America, Africa, and Australia, with the southern hemisphere generally under represented. Registered study sites in these regions, which form part of the IRG-WP durability database (IRG/WP, Citation2013) do exist, but most are also occupied by termites, where the role of fungi cannot be isolated. A full-scale validation of the models developed in this study would require more wood decay data over a broader site diversity.
Real-world applications
In this article, the in-ground fungal decay of wooden test specimens exposed at numerous test locations for the period starting from 1980 until 2022 was modelled. These test specimens cannot directly describe wooden components of larger dimensions (e.g. transmission or fence poles). However, decay rating (or pick-rating), which relates to decay depth, can be considered the metric for service life, defined by the response variable “mean decay rating [0–4]”. According to EN 252 (Citation2015), a decay rating of 4 defines a mechanical impact failure of the wooden test specimen (dimension: 25 × 50× 500 mm3). To link decay rating to larger dimension wooden components would require a link between decay progress past a decay rating of 3 and an associated mechanical property loss of such larger components. Peer-reviewed articles (e.g. van de Kuilen, Citation2007; van de Kuilen & Gard, Citation2013), conference papers (van de Kuilen et al., Citation2021), industry standards (e.g. Welte & Refsnæs, Citation2010 - a review of transmission pole standards), and the in-ground component of the TimberLife software package (Wang et al., Citation2008), highlight links between decay depth, loss in bending strength and/or compressive strength, and loss in cross-sectional area. Depth of decay of a wooden specimen can therefore also reflect loss in cross-sectional area.
In Welte and Refsnæs (Citation2010), initial bending strength is assumed to be based on a 5th percentile of full-scale bending tests for logs of the same species and diameter intended for use as transmission poles. Stresses on the pole resulting from, for example, climatic loads due to wind and ice, the weight of the construction, and tension from the wire conductors, are calculated and compared to the pole’s strength which will decrease over time due to decay. At the same time, stresses in the pole will increase as soon as some part of the pole’s cross-section is subject to reduced modulus of elasticity stemming from decay pockets, woodpecker cavities, lean, and other damages. As decay progresses and causes softening of the cross-section, the sound regions will receive more loading. The point at which the material can no longer resist the maximum stress in the pole denotes failure; however, a major component of this prediction of failure lies in the pole’s loss in load-bearing capacity over time, where inaccurate measurements of remaining cross-section (i.e. remaining diameter and therefore remaining capacity) can cause errors in remaining service life estimation. This is where an accurate decay model could assist utility providers to achieve the longest possible service life from their pole assets (Salman et al., Citation2020). Currently, some utility companies are using resistance drilling measurements at ground level to assess cross-sectional area loss, however these are partially destructive and provide a means for further fungal ingress, not to mention the spatial shortfall of taking only one or two measurements per cross-section (Sharapov et al., Citation2019). Networks of utility poles can span over multiple regions having different soil conditions. The application of remotely sensed data for service life prediction will add information useful in the planning and maintenance on network scale. In addition, it can be used to make inference about whether additional protection is necessary, such as higher retentions of wood preservatives due to increased decay risk.
The developed models are envisioned to be incorporated into a type of service-life planning software, capable of predicting in-ground wood decay of various wood materials as a function of exposure to soil moisture and soil temperature conditions over time. The adoption of weighted daily exposure conditions (i.e. dose) has already been demonstrated in multiple aboveground service life planning works (Isaksson et al., Citation2014; Pousette et al., Citation2017; Thelandersson et al., Citation2011; van Niekerk et al., Citation2023). In this context, dose, rather than exposure time alone, enabled service life planning at practically any geographic location. This includes predicting service life and scheduling maintenance works on planned or already installed wooden components. Furthermore, the use of exposure conditions enables quantitative consideration of the effect of future climate changes. Van Niekerk et al. (Citation2022b) demonstrated the use of various Representative Concentration Pathway (RCP) scenarios to illustrate future aboveground wood decay hazard in the context of various climate change scenarios.
Conclusion
A need exists to incorporate easy-to-use wood decay models into the decision-making surrounding maintenance scheduling, and service life and obsolescence planning in sectors that make use of wood and wood-based components.
The core outcomes of this study can be summarized as follows:
Remotely sensed data from ERA5-Land was successfully integrated with a laboratory-derived dose–response model to (retroactively) analyze wood decay field-trial datasets. Existing wood decay datasets could be given new meaning.
Mean decay rating modelled from daily ERA5-Land retrievals of soil moisture and temperature conditions generally performed better than time of exposure alone as a predictor variable. The model uncertainty remains large, but serves as a starting point for further improvement.
Lacking model performance was observed in locations with high soil moisture conditions owing to the presence of water bodies. This was related to the spatial resolution of ERA5-Land retrievals and in future could be addressed by spatial disaggregation techniques.
Author contributions
Conceived and designed the analysis: B.N.M., M.S., and P.B.vN.
Collected the data: B.N.M., P.B.vN, and C.B.
Contributed data or analysis tools: B.N.M., M.S., P.B.vN, J.N., and C.B.
Performed the analysis: M.S., P.B.vN., and B.N.M.
Visualisation: M.S., B.N.M., and P.B.vN.
Wrote the paper: B.N.M., M.S., and P.B.vN.
All authors have read and agreed to the published version of the manuscript.
Supplemental Material
Download MS Word (21.1 KB)Supplemental Material
Download MS Word (16.4 KB)Acknowledgments
The authors acknowledge support by the Open Access Publication Funds of the University of Göttingen, the IRG-WP durability database and all of its contributors, as well as Joshua Rabke and Antonia Möller, for their role in converting wood decay data from the IRG-WP durability database into a common format.
Disclosure statement
No potential conflict of interest was reported by the author(s).
Data availability statement
The data that support the findings of this study are available from the corresponding author, B.N.M., upon reasonable request.
Supplementary material
Supplemental data for this article can be accessed online at https://doi.org/10.1080/22797254.2023.2264473.
Additional information
Funding
References
- A’Bear, A. D., Jones, T. H., Kandeler, E., & Boddy, L. (2014). Interactive effects of temperature and soil moisture on fungal-mediated wood decomposition and extracellular enzyme activity. Soil Biology and Biochemistry, 70, 151–14. https://doi.org/10.1016/j.soilbio.2013.12.017
- Ågren, A. M., Larson, J., Paul, S. S., Laudon, H., & Lidberg, W. (2021). Use of multiple LIDAR-derived digital terrain indices and machine learning for high-resolution national-scale soil moisture mapping of the Swedish forest landscape. Geoderma, 404, 115280. https://doi.org/10.1016/j.geoderma.2021.115280
- AWPA E7-15 Standard Field Test for Evaluation of Wood Preservatives to be Used in Ground Contact (UC4A, UC4B, UC4C). (2015) . Stake test. American Wood Protection Association (AWPA/WOOD).
- Baker, R. E., Peña, J.-M., Jayamohan, J., & Jérusalem, A. (2018). Mechanistic models versus machine learning, a fight worth fighting for the biological community? Biology Letters, 14(5), 20170660. https://doi.org/10.1098/rsbl.2017.0660
- Brischke, C., Alfredsen, G., Humar, M., Conti, E., Cookson, L., Emmerich, L., Flæte, P. O., Fortino, S., Francis, L., Hundhausen, U., Irbe, I., Jacobs, K., Klamer, M., Kržišnik, D., Lesar, B., Melcher, E., Meyer-Veltrup, L., Morrell, J. J., Norton, J. Suttie, E. (2021). Modelling the material resistance of wood—part 3: Relative resistance in above- and in-ground situations—results of a Global survey. Forests, 12(5), 18. https://doi.org/10.3390/f12050590
- Brischke, C., & Rapp, A. O. (2008). Dose–response relationships between wood moisture content, wood temperature and fungal decay determined for 23 European field test sites. Wood Science and Technology, 42(6), 507–518. https://doi.org/10.1007/s00226-008-0191-8
- Brischke, C., & Selter, V. (2020). Mapping the decay hazard of wooden structures in topographically divergent regions. Forests, 11(5), 510. https://doi.org/10.3390/f11050510
- EN 252:2015. (2015) . Field test methods for determining the relative protective effectiveness of wood preservatives in ground contact. European Committee for Standardization (CEN).
- EN 335:2013. (2013) . Durability of wood and wood-based products – use classes: Definition, application to solid wood and wood-based products. European Committee for Standardization (CEN).
- European Commission, Copernicus, ECMWF, & Climate Change Service. (2022). Download data. ERA5-Land hourly data from 1950 to present. https://doi.org/10.24381/cds.e2161bac
- Gonzales, J. M., & Morrell, J. J. (2012). Effects of environmental factors on decay rates of selected white- and brown-rot fungi. Wood and Fiber Science, 44(4), 343–356.
- Hiscox, J., Clarkson, G., Savoury, M., Powell, G., Savva, I., Lloyd, M., Shipcott, J., Choimes, A., Amargant Cumbriu, X., & Boddy, L. (2016). Effects of pre-colonisation and temperature on interspecific fungal interactions in wood. Fungal Ecology, 21, 32–42. https://doi.org/10.1016/j.funeco.2016.01.011
- IRG/WP. (2013). International Research Group on Wood Protection (IRG/WP)—Durability Database. https://irg-wp.com/durability/index.html#step3
- Isaksson, T., Brischke, C., & Thelandersson, S. (2013). Development of decay performance models for outdoor timber structures. Materials and Structures, 46(7), 1209–1225. https://doi.org/10.1617/s11527-012-9965-4
- Isaksson, T., Thelandersson, S., Jermer, J., & Brischke, C. (2014). Beständighet för utomhusträ ovan mark. Research Institutes of Sweden (RISE).
- Kassambara, A. (2020). Ggpubr: ‘Ggplot2’ Based Publication Ready Plots. https://CRAN.R-project.org/package=ggpubr
- Klaassen, R. K. W. M., & Creemers, J. G. M. (2012). Wooden foundation piles and its underestimated relevance for cultural heritage. Journal of Cultural Heritage, 13(3), S123–S128. https://doi.org/10.1016/j.culher.2012.02.014
- Kuhn, M. (2008). Building predictive models in R using the caret package. Journal of Statistical Software, 28(5). https://doi.org/10.18637/jss.v028.i05
- Lebow, S. T., & Highley, T. (2008). Regional biodeterioration hazards in the United States. In T.P. Schultz, H. Militz, M.H. Freeman, B. Goodell, & D.D. Nicholas (Eds.), Development of commercial wood preservatives: Efficacy, environmental, and health issues (Vol. 982, pp. 120–141). American Chemical Society. https://doi.org/10.1021/bk-2008-0982.ch006
- Lidberg, W., Nilsson, M., & Ågren, A. (2020). Using machine learning to generate high-resolution wet area maps for planning forest management: A study in a boreal forest landscape. Ambio, 49(2), 475–486. https://doi.org/10.1007/s13280-019-01196-9
- MacKenzie, C. E., Wang, C.-H., Leicester, R. H., Foliente, G. C., & Nguyen, M. N. (2007). Timber service life design guide. ISBN. Forest and Wood Products Australia.
- Marais, B. N., Brischke, C., & Militz, H. (2020). Wood durability in terrestrial and aquatic environments – a review of biotic and abiotic influence factors. Wood Material Science & Engineering, 17(2), 82–105. https://doi.org/10.1080/17480272.2020.1779810
- Marais, B. N., van Niekerk, P. B., & Brischke, C. (2021). Studies into fungal decay of wood in ground contact—part 2: Development of a dose–response model to predict decay rate. Forests, 12(6), 698. https://doi.org/10.3390/f12060698
- Mendelsohn, R., Kurukulasuriya, P., Basist, A., Kogan, F., & Williams, C. (2007). Climate analysis with satellite versus weather station data. Climatic Change, 81(1), 71–83. https://doi.org/10.1007/s10584-006-9139-x
- Microsoft Excel 2019. (2018). [Computer software]. Microsoft Corporation. https://office.microsoft.com/excel
- Morrell, J. J. (2018). 14—Protection of wood-based materials. In M. Kutz Ed. Handbook of environmental degradation of materials Third, pp. 407–439. https://doi.org/10.1016/B978-1-4377-3455-3.00014-6.
- Morrell, J. J., & Zabel, R. A. (1985). Wood strength and weight losses caused by soft rot fungi isolated from treated southern pine utility poles. Wood and Fiber Science, 17(1), 132–143.
- Morton, L. H. G., & Eggins, H. O. W. (1977). The effect of constant, alternating & fluctuating temperatures on the growth on some wood inhabiting fungi. International Biodeterioration Bulletin, 13(4), 116–122.
- Pousette, A., Malo, K. A., Thelandersson, S., Fortino, S., Salokangas, L., & Wacker, J. (2017). Durable timber bridges final report and guidelines ( SP Rapport 2017:25; p. 178).
- Rapp, A. O., Peek, R. D., & Sailer, M. (2000). Modelling the moisture induced risk of decay for treated and untreated wood above ground. Holzforschung, 54(2), 111–118. https://doi.org/10.1515/HF.2000.019
- R Core Team. (2022). R: A language and Environment for Statistical computing (4.2.0) [computer software]. R Foundation for Statistical Computing. https://www.R-project.org/
- RStudio Team. (2022). RStudio: Integrated Development Environment for R (2022.07.0) [computer software]. RStudio, PBC. http://www.rstudio.com/
- Salman, A. M., Salarieh, B., Bastidas-Arteaga, E., & Li, Y. (2020). Optimization of condition-based maintenance of wood utility pole Network Subjected to hurricane hazard and climate change. Frontiers in Built Environment, 6, 73. https://doi.org/10.3389/fbuil.2020.00073
- Salomón, R. L., Peters, R. L., Zweifel, R., Sass-Klaassen, U. G. W., Stegehuis, A. I., Smiljanic, M., Poyatos, R., Babst, F., Cienciala, E., Fonti, P., Lerink, B. J. W., Lindner, M., Martinez-Vilalta, J., Mencuccini, M., Nabuurs, G.-J., van der Maaten, E., von Arx, G., Bär, A., Akhmetzyanov, L. Steppe, K. (2022). The 2018 European heatwave led to stem dehydration but not to consistent growth reductions in forests. Nature Communications, 13(1), 28. https://doi.org/10.1038/s41467-021-27579-9
- Schönauer, M., Prinz, R., Väätäinen, K., Astrup, R., Pszenny, D., Lindeman, H., & Jaeger, D. (2022). Spatio-temporal prediction of soil moisture using soil maps, topographic indices and SMAP retrievals. International Journal of Applied Earth Observation and Geoinformation, 108, 102730. https://doi.org/10.1016/j.jag.2022.102730
- Sharapov, E., Brischke, C., & Militz, H. (2019). Assessment of preservative-treated wooden poles using drilling-resistance measurements. Forests, 11(1), 20. https://doi.org/10.3390/f11010020
- Stirling, R., Alfredsen, G., Brischke, C., De Windt, I., Francis, L. P., Frühwald Hansson, E., Humar, M., Jermer, J., Klamer, M., Laks, P. E., Le Bayon, I., Metsä-Kortelainen, S., Meyer-Veltrup, L., Morris, P. I., Norton, J., Singh, T., Van Acker, J., Van den Bulcke, J., Venås, T. M., and Wong, A. H. H. (2016). Global survey on durability variation – on the effect of the reference species. Proceedings IRG Annual Meeting, Lisbon, Portugal, IRG/WP 16-20573 (pp. 26).
- Thelandersson, S., Isaksson, T., Frühwald Hansson, E., Toratti, T., Viitanen, H., Grüll, G., Jermer, J., & Suttie, E. (2011). Service life of wood in outdoor above ground applications Engineering design guideline (TVBK 3060. Lund University. http://www.kstr.lth.se/fileadmin/kstr/pdf_files/Guideline/TVBK-3060JJversion.pdf
- van de Kuilen, J.-W. G. (2007). Service life modelling of timber structures. Materials and Structures, 40(1), 151–161. https://doi.org/10.1617/s11527-006-9158-0
- van de Kuilen, J. W., Beketova-Hummel, O., Pagella, G., Ravenshorst, G., & Gard, W. (2021). An integral approach for the assessment of timber pile foundations. World Conference on Timber Engineering, 9–12 August, Santiago, Chile.
- van de Kuilen, J.-W. G., & Gard, W. (2013). Damage assessment and Residual service life estimation of Cracked timber Beams. Advanced Materials Research, 778, 402–409. https://doi.org/10.4028/www.scientific.net/AMR.778.402
- van Niekerk, P. B., Marais, B. N., Brischke, C., Borges, L. M. S., Kutnik, M., Niklewski, J., Ansard, D., Humar, M., Cragg, S. M., & Militz, H. (2022b). Mapping the biotic degradation hazard of wood in Europe – biophysical background, engineering applications, and climate change-induced prospects. Holzforschung, 76(2), 188–210. https://doi.org/10.1515/hf-2021-0169
- van Niekerk, P. B., Niklewski, J., Hosseini, S. H., Marais, B. N., Frimannslund, I., Thiis, T. K., & Brischke, C. (2023). Simulations of microclimates for wood-decaying fungi in the built environment using environmental analysis. Proceedings IRG Annual Meeting (ISSN 2000-8953), Cairns, Australia, IRG/WP 23–20703.
- van Niekerk, P. B., Schönauer, M., Marais, B. N., & Brischke, C. (2022). Using satellite-retrieved soil moisture data to model the decay risk of in-ground timber IRG/WP 22-20689. 5.
- Venugopal, P., Junninen, K., Linnakoski, R., Edman, M., & Kouki, J. (2016). Climate and wood quality have decayer-specific effects on fungal wood decomposition. Forest Ecology and Management, 360, 341–351. https://doi.org/10.1016/j.foreco.2015.10.023
- Viitanen, H. A. (1997). Modelling the time Factor in the Development of brown rot decay in pine and spruce sapwood—the effect of critical humidity and temperature conditions. Holzforschung, 51(2), 99–106. https://doi.org/10.1515/hfsg.1997.51.2.99
- Viitanen, H., Toratti, T., Makkonen, L., Peuhkuri, R., Ojanen, T., Ruokolainen, L., & Räisänen, J. (2010). Towards modelling of decay risk of wooden materials. European Journal of Wood and Wood Products, 68(3), 303–313. https://doi.org/10.1007/s00107-010-0450-x
- Wang, C., Leicester, R. H., & Nguyen, M. N. (2008). Manual 3—decay in ground contact ( Manual USP2007/040). Timber Service Life Design Guide). CSIRO.
- Welte, T., & Refsnæs, S. (2010). Useful life extension of wood poles by better condition assessment. 13.
- Wickham, H. (2016). ggplot2: Elegant graphics for data analysis (2nd ed.). Springer-Verlag New York. https://doi.org/10.1007/978-3-319-24277-4
- Wickham, H., François, R., Henry, L., & Müller, K. (2022). Dplyr: A grammar of data manipulation [computer software]. https://dplyr.tidyverse.org