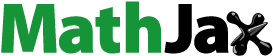
ABSTRACT
A methodology for classifying rainfed paddy fields based on their hydrological conditions is lacking. This study analysed the behaviour of synthetic aperture radar (SAR) backscatter coefficients at each polarisation and cross-polarisation ratio index in a rainfed rice paddy field with a partially waterlogged surface. The SAR polarisations used were the VH and VV of the C-band SAR Sentinel-1 and the HV and HH of the L-band SAR Palsar-2. The relationship between backscatter coefficient and terrains including topographic categories and local relative elevation, which affect waterlogging conditions in rainfed paddy fields, were evaluated. The VV of C-band, and HH and HV of L-band showed different patterns in the time series variation according to the topographic categories. We observed that the combination of L-band HH and HV could be used to assess waterlogging conditions because they differ depending on variations in local elevation. The study results suggest that future studies can evaluate the microtopography and associated local hydrological environment using the combination of L-band HH and HV backscatter coefficient.
Introduction
Numerous areas of rice cultivation worldwide lack irrigation measures. Rice yields in rainfed paddy fields are lower and considerably less stable than those in irrigated fields, as the duration of ponding during the cultivation period has a strong influence on the rice crop yield (Jearakongman et al., Citation1995). Southeast Asia, including the targeted area of this study, Northeast Thailand, has made effort to overcome the instability of rainfed rice cultivation for a long time. West Africa accounts for 56% of Africa’s rice paddies, 59% of which are rainfed; this proportion has been increasing since 1980 (Oteng & Sant’anna, Citation1999). Accurate quantification of the moisture conditions of rainfed paddy fields would be highly valuable in the evaluations of the stability of local food production, food planning, and farmland development.
Synthetic Aperture Radar (SAR) backscatter coefficients are widely used to estimate soil moisture and water content. For agricultural land, the backscatter coefficient is affected by soil surface roughness and vegetation conditions in addition to water content. Several methods have been developed to estimate soil water content by separating the effects of surface roughness (Fieuzal & Baup, Citation2016; Oh, Citation2004; Oh et al., Citation1992) and vegetation (Bhogapurapu et al., Citation2022). However, a different approach is required for paddy fields, where the moisture conditions should be evaluated based on the spatial cover ratio of water surface and its temporal duration as well as soil water content.
SAR is used to estimate growth conditions in rice paddies using scattering from rice vegetation and to extract paddy field areas using scattering from a combination of the water surface and vegetation. Paddy fields are extracted using the L- and C-bands of SAR, which have a high transparency to herbaceous vegetation relative to the X-band. In recent years, Sentinel-1 satellites, which provide 6- or 12-day period data, have been used to extract paddy fields and to evaluate rice growth using continuous time series Sentinel-1 backscatter coefficients (Keerthana et al., Citation2022; Minh et al., Citation2019; Nguyen et al., Citation2016; Singha et al., Citation2020; Wang et al., Citation2022; Zhan et al., Citation2021). These studies assumed almost constant waterlogged conditions spatially and were not intended to classify paddy fields according to the degree of wetness, such as the percentage or frequency of waterlogged surface area.
Backscatter coefficients of C-band Sentinel-1 and L-band PALSAR-2 have often been used to detect non-inundated paddy fields owing to artificial irrigation management during crop seasons, which affects the emission of methane from the soil (Arai et al., Citation2018; Nelson et al., Citation2022; Torbick et al., Citation2017). Inundated and non-inundated paddy fields can be distinguished from each other using components of backscattering, including single and double bounce and volume diffusion (Arai et al., Citation2018).
However, water logging in rainfed paddy fields ranges from fully flooded for most of the growing season to partially flooded for part of the season. Water logging varies less widely than rainfed paddy fields and is, therefore, easier to quantify. The backscatter coefficients are expected to show temporal and spatial variations, including waterlogging, depending on the vegetation and ground surface conditions. The possibility of using SAR to estimate waterlogging conditions in rainfed paddy fields has not been studied.
The objective of this study was to classify rain-fed paddy fields based on water logging conditions using multitemporal and multi-polarisation SAR backscatter coefficients. To accomplish this, we observed the time-series behaviours of the backscatter coefficient in each polarisation and the polarisation ratios of L-band PALSAR-2 and C-band Sentinel-1 in rain-fed paddy fields with various waterlogging conditions.
First, the time series of backscatter coefficients for the entire target area was observed in terms of vegetation and waterlogging conditions. Next, the time-series changes in backscatter coefficients at sample points arranged by terrain category were observed, and the characteristics of the backscatter coefficients in each terrain category were discussed. Finally, the backscatter coefficients in the cross sections of the characteristic landforms were observed to evaluate the relationship between the microreliefs, which affect local hydrological conditions. In addition, the backscatter coefficients were discussed.
Materials and methods
Study area
The study area is 14 km from east to west and 11 km from north to south around Nam phong District, Khon Kaen Province (N16.795, E102.741) in the north-eastern part of Thailand. shows the topography of the target area and the distribution of the rice paddies. The lowlands extend in a ring around the hills, and a network of river channels forms at the lowest part of the ring. The outer edge of the ring is an anticline, and the lowlands are surrounded by a concentric series of hills with a height difference of approximately 30 m. The gaps between the hills and lowlands are covered with small streams and paddy fields. A small stream flows from a gap of ridges to the lowland of the ring, forming a quasi-valley. The central part of the lowland ring is also covered by hills with a height difference of approximately 30 m. The flat area at the southern end of the eastern side is a marshland surrounding the swamp.
Figure 1. Terrain surrounding the study area. Streams and ponds/swamps are placed based on Sentinel-2 MSI on 21 May, 2020, while the elevation and contours are derived from the shuttle radar topography mission (SRTM) at 1-arc second. The contour interval is 5 m.

In the target area, farmlands, called rainfed paddy fields, are not directly dependent on rainfall alone but are recharged by runoff from the elevated land. Therefore, most paddy fields are located at the bottom of the circular lowlands, at the bottom of the slopes on either side of the lowlands, and at the bottom of shallow valleys. Some are also scattered in small valleys and basins on hillsides surrounded by lowlands.
Some rice paddies are wet and frequently flooded, whereas others are relatively dry. Within each catchment area, the lower the elevation, the more water was collected. Field observations carried out near the target area indicated that lower hillsides had saturated areas that were closer to the ground surface (Moroizumi et al., Citation2009). Even when there is a hard pan between the ground surface and the aquifer, the perched water table at or near the ground surface shows the same fluctuation trend as shown by the groundwater level (Tsubo et al., Citation2007). Therefore, the soil moisture is higher and water stagnation occurs more frequently at lower elevations within the same basin (Hoshikawa et al., Citation2018; Kaida et al., Citation1985; Miyagawa et al., Citation2006). Local farmers refer to wet paddy fields at lower elevations as “depression (lowland) paddy fields” and dry paddy fields at relatively higher slopes as “hill (highland) paddy fields”.
The average annual precipitation in the target area is approximately 1,000 mm. Northeast Thailand, including the targeted area, has distinct wet and dry seasons, with the annual rainfall mostly concentrated in the rainy season from April to November. Therefore, the moisture content of surface soils decreases to approximately 10% in early April at the beginning of the rainy season and displays a relatively simple increase toward the end of the rainy season around October (Goto et al., Citation2008; Moroizumi et al., Citation2009). The monthly rainfall from June to October exceeds 60 mm, which is the standard for humid tropical climates in the Köppen climate classification. Rice planting in rainfed rice paddies in the target area generally takes place from June to August, when the paddy fields are sufficiently wet, and harvesting takes place from November to December. shows the accumulated precipitation average of three stations – Nam Phong, Ubonrat, and Khaosuang Kwang – from 1 April to 31 October 2020 and 2021.
Methodology
Using the L-band PALSAR-2 and C-band Sentinel-1, we evaluated the relationship between the backscatter coefficient and condition of water logging at each wavelength and polarisation. In 2020, Sentinel-1 and PALSAR-2 observed the study area with dual polarisation mode, VV-VH and HH-HV, respectively.
In addition to the individual use of the backscatter coefficient from each polarisation, the behaviour of indices derived from the combination of cross- and co-polarisation C-band VH/VV and L-band HV/HH were observed. Smaller C-band VH/VV and larger double scatter of VV is observed when reflected from water surfaces and plants (Bangira et al., Citation2021). The L-band HV/HH ratio is highly sensitive to soil surface roughness, and the relationship between the ratio and roughness can be approximated using a single quadratic equation regardless of vegetation type, bare soil, sugar beet, winter wheat, or grassland (Koyama & Schneider, Citation2011). In this study, we evaluated the behaviour of the L-band HV/HH ratio on soil surfaces partially or totally covered by water.
Direct measurement of the moisture (i.e. soil water content and water surface area) of land surface over a wide area is impossible. Therefore, the local topographic relief, which is strongly correlated with wetness conditions, was used as a proxy indicator. The area encompasses a relatively low area, with surrounding hills, and shallow short valleys among the hills (). Rice is cultivated in the lower area, the bottom of shallow valleys, and some depressions in the hilly areas.
Three cross-sections with 30 points each were placed at paddy fields in areas with the three typical topographical features () to observe temporal changes in backscatter coefficients.
In addition, the relationship between local terrain and backscatter coefficients was evaluated using linear regression analysis. The entire area of interest was divided into 14 × 11 square plots each covering 1 km2, and the correlation between the elevation and the backscatter coefficient in paddy field pixels was calculated for 67 plots, for which the percentage of coverage by paddy fields was 30% or higher. Considering that local topographic elevation differences determine the moisture conditions, a correlation between the elevation and backscatter coefficient in each area should be observed. The backscatter coefficient in any polarisation or backscatter-derived index, which is strongly determined by the hydrological conditions of the ground surface, should have a high correlation with the local topographic elevation. Linear regressions were performed between the SAR backscatter coefficient and the digital elevation model (DEM) elevation for each pixel within each region, and the correlation coefficient and slope of the regression equation were determined and compared.
Spatial allocation of the points
Based on the three topographical characteristics above, paddy fields were broadly classified into three categories: lowland, valley, and hill. Ten sites were selected in each category for the analysis of temporal changes in backscatter coefficient (). Compared to the other two, paddy fields in lowland are the most diverse in terms of waterlogging conditions. The west side of the arc-shaped lowland has small rivers flowing through its branches (points L2, L3, L8, and L9), and water floods into the rice paddies during the rice-cropping season. The southeast side is the outer edge of a swampy area, where rice is cultivated during the dry season as well as rainy season (L6 and L7). The northern part of the arc (L4 and L5) is relatively drier than the rest of the arc. The border between the lowland and hilly area is not clear. Paddy fields between the two areas gradually become drier with elevation.
The valleys flow mainly from the periphery of the area to the lowlands (including V3-V9, V5, V4-V6-V1) except for short and shallow valleys on the hill (V10 and V7-V8). The difference in elevation between the valley bottom and ridges on its sides is less than 10 m in most cases. Rice paddies are cultivated on the valley bottom and surrounded by sugarcane fields on the surrounding hills.
Most of the hilly area is covered with sugarcane and cassava fields. Rice paddies are scattered in the depressions in the area (from H1 to H10).
Topographical features of the sections
shows the elevation based on Shuttle Radar Topography Mission (SRTM) along the three sections.
Section 1 crosses a shallow valley and a small stream at the bottom of the valley. Along the stream are strips of high bank (200 and 380 m) that appear to be natural levees. The tops of the natural levees are overgrown with trees, and rice paddies extend into the lowlands on both sides of the levees.
Section 2 extends from the upstream to the downstream of a short shallow valley that comprises a break in the range of hills surrounding the lowlands. The transect line is not at the bottom of the valley but rather on the left bank of the stream, parallel to a stream channel. The elevation along this line does not decrease monotonically; approximately 300–350 m from upstream, the elevation increases by approximately 2 m.
Section 3 is shallow and somewhat indistinct but extends for approximately 5 km; this section crosses the left bank of the outlet of the valley with a small stream. It begins approximately 20 m from the stream at the valley bottom and ends at the boundary between the valley and the hillslope. The valley floor and slopes on both sides can be clearly distinguished by differences in slope and land use.
The elevation along the stream is relatively high, and the lowest part of the section is approximately 80–100 m from the beginning of the section at the valley bottom.
Data
Synthetic aperture radar data
We used backscatter imagery data acquired by C-band Sentinel-1 from the end of March to October in 2020 and L-band PALSAR-2 on four days from March to October in 2020. presents an outline of the SAR data used in this study.
Table 1. Synthetic aperture radar (SAR) data used in this study.
Sentinel-1 was operated by two units, S1A and S1B, with a return period of 12 days for S1A. Sentinel-1 was observed in the interferometric wide (IW) swath mode for both its ascending and descending orbits around the target area; it acquires data with a 250-km swath at 5 × 20 m spatial resolution (single look). The maximum incident angles to the ellipsoid surface within the targeted area from the ascending orbits was 43.2°, which occurred at the western ends of the area. The boundary of the scene in the ascending orbit of S1A crosses the targeted area, which encompassed 19 scenes in total within the target period.
Level-1 GRD products downloaded from the Copernicus Open Access Hub, a website operated by the European Space Agency (ESA), were converted to a sigma naught backscatter coefficient using the ESA Sentinel Application Platform (SNAP). In addition to terrain correction, GRD border noise removal, thermal noise removal, and speckle noise filtering using the Lee – Sigma filter were conducted. Then the magnification values obtained by SNAP were converted to power decibel values at the pixel level using Eq. (1):
where σ0mag is the backscatter coefficient in magnification provided by the ESA SNAP and σ0dB is the decibel value.
The observation modes of PALSAR-2 in the target area and period were high-resolution SM3 (strip map 10 m) and wide-area WD1, respectively. In this study, we used data observed by SM3 on 1 July, 12 August, 9 September, and 21 October 2020 in ascending orbit. shows the dates of the PALSAR-2 observations used in this study. The DN of the Level 2.1 product with ortho rectification was converted to sigma naught using Eq. (2) provided by the Japan Aerospace Exploration Agency (JAXA, Accessed on, Citation2022):
where DN is the original pixel value and σ0dB the backscatter coefficient in its decibel value before terrain correction. CF1 is a calibration coefficient, −83.0, as of 28 March 2017.
Speckle noise filtering was not applied to the PALSAR-2 images, except for ensemble averaging during the DN conversion. The ensemble average is the value of the pixel in question and its eight neighbouring pixels, yielding nine pixels in total.
The resolution of all the data was maintained to minimise the influence of resampling. Sentinel-1 IW and PALSAR-2 SM3 Level 2.1 had 10 × 10 m and 6.25 × 6.25 m resolution, respectively, and both were in the WGS84 UTM-48 coordinate system.
Digital elevation model
SRTM3, with a 1-arc second resolution, was reprojected onto the WGS84 UTM-48 system at a spatial resolution of 10 m (Sentinel-1) and 6.25 m (PALSAR-2). The purpose of the SRTM project is to create data with an absolute vertical height accuracy of less than 16 m and a relative vertical height accuracy of less than 10 m. According to an accuracy assessment for SRTM at Phuket, Thailand, approximately 1000 km SSE of the study area, the average of absolute errors from GPS measurements at points with slopes of more than 10° was 12.37 ± 1.31, and 5.03 ± 0.41 at points with slopes not exceeding 10° (Gorokhovich & Voustianiouk, Citation2006). The slope at 97% of paddy fields in the study area is less than 10%.
Land cover and vegetation
The spatial distribution of paddy fields was extracted from land-use vector data based on L7018 1/50,000 produced by the Royal Thai Survey Department and normalised difference vegetation index (NDVI) derived from the atmospheric-corrected Sentinel-2 Multispectral Instrument (S2MSI2A) on 10 June 2020. The RTSD L7018 is based on ground-level information as of 2005. In recent years, however, sugarcane and cassava cultivations in rice paddies have expanded. The seasonal cultivation periods and plant structures of these crops differ from those of paddy rice, which may affect the backscatter coefficient. Therefore, pixels with NDVI > 0.3 on 10 June 2020, where the NDVI of the rice field is considerably smaller, were eliminated from the analysis.
shows the NDVI at four time points in the three transects. During the targeted period-two years of the rainy season – S2MSI images with little cloud cover were available for only 6 days close to the dates of observation using PALSAR-2.
Two photosensitive rice varieties, Khao Dok Mali (KDML)-105 and Rice Department (RD) 6, are mainly cultivated in the study area. There is no difference in phenology between these two varieties except that the harvest period of KDML-105 at the end of November is approximately 5–10 days later than that of RD6. The vegetative period of these rice varieties is approximately 60 days from late May to August. The reproductive growth (heading, flowering) period lasts 35 days from August to September. The ripening period lasts 25–35 days from September to the end of October. Finally, the mature grain stage occurs around the end of October (Boonhong, Citation2014; Rice Department in Thailand, Citation2008).
Results
Backscatter coefficients and cross-polarisation ratio for the entire area
show the backscatter coefficient and cross polarisation ratio of Sentinel-1A and PALSAR-2 in the target area. Images on six dates closest to each observation by PALSAR-2 were selected from the Sentinel-1A data.
Figure 4. Backscatter coefficient of C-band VV - VH polarisations and cross-polarisation ratio VH/VV for the entire study area.

Figure 5. Backscatter coefficient of L-band HH - HV polarisations and cross-polarisation ratio HV/HH for the entire study area.

For the entire area, the backscatter coefficients in March and May were smaller than those on other dates for all wavelengths and polarisations.
The C-band VH yielded lower values in paddy fields than in surrounding non-paddy fields over all six time points. The backscatter coefficient in paddy fields increased towards 28 October, with little difference observed between paddy and non-paddy fields on 28 October. The backscatter coefficient of the C-band VV in the paddy fields showed the most significant seasonal variation. The C-band-VV showed lower values than the surroundings until 30 June and then higher values on 17 August. As a result, the VH/VV ratio in the paddy fields was distinctly smaller than that of the surroundings on 17 August. On 30 March, there was no difference between the VH/VV ratios for the paddy and non-paddy fields, and the difference in the VH/VV ratio between paddy and non-paddy fields showed a significant seasonal variation.
L-band HV tended to yield lower backscatter coefficients in paddy fields than in surrounding non-paddy fields over the six time points. A slight difference was observed between the L-band HH for paddy and non-paddy fields except on 1 July and 12 August. The HV/HH ratio was consistently lower in paddy fields.
Backscatter coefficients at the points
shows the backscatter coefficient for each sensor, polarisation and cross polarisation ratio at each point, and the NDVI over time for each topographical category.
Figure 6. Time-series changes in backscatter coefficients and cross polarization ratio for each topographical categories, polarisation, and band.

The trends in the time-series of C-band VV backscatter coefficient in the three regions differed from each other. Backscatter coefficient in the lowland increased relatively quickly towards Day of Year (DOY) 230 (mid-August) and then began to decline. In the valley, there was no increase or a slight decline until around DOY 150 (end of May), then the values increased towards the peak around DOY 240 (end of August). Backscatter coefficient in the hilly region increased monotonically and reached a ceiling around DOY 240.
Cross-polarisation ratio HV/HH also showed different trends among the three categories. The ratio decreased until DOY 230 and then increased in the lowland region. In the valley category, the ratio decreased more slowly than in the lowland until DOY 240, whereas the ratio in the hilly region increased gradually.
For the L-band, the backscatter coefficients of HH and HV showed similar patterns of temporal change for each terrain category. In lowland, the HH did not change whereas HV showed a gradual decreasing trend until day 225 (12 August), after which both polarisations increased. In addition, L-band HH backscatter coefficient in the lowland showed a large point-to-point variation. Both HH and HV backscatter coefficients in the valley showed no change until DOY 225 (1 July) and then increased. In the hilly region, the backscatter coefficient for HH and HV showed an increasing trend after a clear decrease at DOY 126 (6 May).
The median HV/HH showed almost linear trends in all three categories due to the similarity between the HV and HH change patterns within the topographic categories.
The median NDVI increases sharply from around DOY 160 in all regions, but the change was more pronounced in the lowlands. The NDVI became nearly constant around DOY 200 in the lowlands and stabilised around DOY 250 in the other two regions. In both cases, a large point-to-point variation was observed within categories, and differences between categories were not pronounced.
Backscatter coefficients in the cross-sections
shows the backscatter coefficients of S1A and PALSAR-2 for each polarisation from the end of March to October 2020 for Sections 1–3, along with the SRTM elevations. Backscatter coefficient on six dates closest to each observation by PALSAR-2 were selected for Sentinel-1A. Overall, PALSAR-2 showed greater spatial variability than Sentinel-1. HH and VV showed larger intertemporal variations than HV and VH, respectively. shows correlation coefficients between elevation and backscatter coefficients along the three lines at each satellite observation. For Section 1, the correlation coefficient in the subsection from 170 to 350 m is indicated.
Figure 7. Backscatter coefficient of C-band VV - VH and L-band HH – HV along cross-sections. Vertical lines indicate the locations of transects between the ridges around each farm plot and section. Elevation is based on SRTM 1-arc second.

Figure 8. Correlations between elevation and backscatter coefficients and elevation and cross-polarization indices.

Section 1: High backscatter coefficients of VH polarisation appeared around 200, 350, 500, and 700 m, where the ground surface has peaks or steep slopes. However, the backscatter coefficient did not follow the general trend of undulations of the entire section (). Correlation coefficients between elevation and VH backscatter along the section vary from near zero to 0.63 throughout the period. Section 1 comprises three subsections divided by two streams at 170 and 350 m. Considerably higher correlation coefficients were determined between VH backscatter coefficient with elevation within each subsection. For example, the correlation coefficient for the subsection from 170 to 350 m varied in positive values from 0.25 to 0.88 ().
Significant temporal fluctuation was observed in the VV backscatter coefficient along the section (). The correlation coefficients between elevation and VV backscatter coefficient throughout the section varied largely from −0.36 to 0.34, and from −0.04 to 0.90 for subsection 170–350 m ().
HV backscatter coefficient showed more significant fluctuations along the cross-section (). Correlation coefficients between elevation and backscatter coefficient for the entire section were not more than 0.3 on any date. Correlation coefficients in the subsection from 170 to 350 m were 0.68, 0.81, and 0.71 on 6 May, 1 July, and 12 August. The correlation between HH and terrain was not as clear as that between HV and terrain because HH fluctuated substantially over time (). The correlation coefficient for the subsection 170–350 m fluctuated largely between −0.43 and 0.64 ().
Section 2: VV showed a large variation between observation points and did not have a notable relationship with the terrain (). VH and HV showed a change along the topography on most of the observation days (). Correlation coefficients between elevation and VH backscatter coefficient were positive on 14 of 19 observations (). HV showed a high correlation coefficient with elevation, 0.74, 0.54, and 0.64 on 1 July, 12 August, and 9 September, respectively. HH also showed a similar trend, although it was not as clear as that of HV (Figure (j)). Correlation coefficients for HH on the same dates were 0.33, 0.29, and 0.45 ().
Section 3: VV and VH backscatter coefficients showed similar relation with elevation (). The correlation coefficient between elevation and backscatter coefficients for the entire section ranged commonly from −0.8 to 0.9 and temporally fluctuated with similar trends ().
HH and HV showed different temporal and spatial trends along section 3 (). HV showed a positive correlation to elevation from 0.4 to 0.6 except for 25 March and 21 October, whereas HH showed a positive correlation only on 1 July ().
shows the cross-polarisation ratios along the three sections with HV/HH for PALSAR-2 and VH/VV for Sentinel-1 at the same time points as in .
Figure 9. Cross-polarisation ratio VH/VV and HV/HH along the cross-sections. Elevation is based on SRTM 1-arc second.

Both for Sentinel-1 and PALSAR-2, the cross-polarisation ratio followed a more detailed increase and decrease of elevation in all cross sections. However, correlation coefficients between VH/VV and elevation were considerably smaller than those between VH and elevation in Sections 1 and 3 (). Correlations between HV/HH ratios and terrain significantly exceeded those between HV backscatter coefficient and terrain on 1 July (section 2) () or 9 September (sections 1 and 3) () when the correlation of HH with terrain decreased significantly. In section 3, the HV/HH ratio showed higher correlation with terrain than HV except for 6 May ().
shows the backscatter coefficients (dB) of the two polarisations at three points in each transect, where characteristic changes in the backscatter coefficient were observed. The grey diagonal line shows a 1:1 slope for the co-polarisation/cross-polarisation relationship. Changes parallel to the diagonal were not accompanied by changes in the cross-polarisation ratio. The co-polarisation ratio is larger in the areas to the lower right and upper left of the line.
Figure 10. Temporal changes in the relationship between the co- and cross-polarisations of Sentinel-1A and PALSAR-2 at three selected points along the cross-sections during the observation period in 2020; a’, c’, and e’ indicate values for days close to the observations via PALSAR-2.

The C-band VV − VH (Sentinel-1), which was plotted on a smaller time step, shifted across the diagonal, such that VH increased while VV decreased ( 490 m) or only VH decreased () 490 m) or stagnated () 50 m and 110 m), which often occurred in the middle of the rainy season.
This trend is more pronounced in ( where the time steps are nearly aligned with the L-band HH−HV relation. The C-band VV−VH relation often move to the direction across the diagonal, whereas the L-band HH−HV relation moved parallel to it.
Spatial distribution of correlation coefficients
shows the correlation coefficients (R) between the backscatter coefficient in decibel value and elevation on the paddy fields for the 67 grids of 1 km squares with a paddy coverage of ≥ 30%.
Figure 11. Correlation coefficients of the linear regression between the elevation and backscatter coefficient within each square kilometre.

A high positive correlation was observed around the centre and lower left of the figures, where there is a slope from the hill to the valley bottom (), especially for cross-polarisations VH and HV. At the bottom of the wide valley surrounding the hill, almost no correlation was observed between the elevation and the backscatter coefficient for any polarisation and season. The spatial distribution of the correlation coefficient for VH was close to that for HV. HV showed higher positive correlation with elevation than VH in September and October.
The distributions for co-polarisations VV and HH differed significantly, whereas the similarity between the cross- and co-polarisation was low.
The VV polarisation had relatively large negative correlations at the bottom of the wide valley around the southwest corner of the target area, especially on 17 August, which differs from the results obtained using the other three polarisations. This indicates that the backscattering coefficients tended to be higher in wetter, lower-lying areas with higher water content and water surface ratios.
Discussion
Terrain categories and backscattering
The pattern of time-series changes of C-band VV backscatter coefficient in each topographic category can be characterised in two respects: the presence or absence of an increase towards around DOY 150 (end of May), and the presence and magnitude of a peak around DOY230–240 (mid to end of August) ().
The peaks of VV polarisation that appeared in August in the lowland and valley categories are likely because of double scattering by waterlogged surfaces and vegetation. The height of peaks may reflect the higher proportion of waterlogged areas in lowlands and valleys than in the hills. In the lowland and valley regions, VV began to decline during September. Similar increase and decrease of C-band VV of Sentinel-1 during the rice cultivation period have been previously reported in paddy fields in the Mekong Delta (Minh et al., Citation2019; Nguyen et al., Citation2016) and India (Keerthana et al., Citation2022). The decline on 26 October was particularly noticeable in the west, where small rivers are concentrated, and in the south-east on the edge of the swamp field (). This steep decline suggests that the height of surface water and the maturation of rice in paddy fields caused the decrease. The higher rate of increase in VV in the lowland region than other categories from the end of April (DOY 120), when NDVI is low, may be caused by a higher rate of increase in soil water content ().
No significant differences in the time series variation of VH polarisation were observed between terrain categories. As a result, the differences in the cross-polarisation ratio VH/VV between terrain categories were also not significantly higher than the differences in VV. However, the fact that VH/VV was significantly lower in August in the lowlands than in the surrounding area suggests that this ratio is useful for the identification of waterlogged paddy fields as well as flooded vegetation.
There were different patterns of temporal changes in the backscatter coefficient both for L-band HH and HV polarisation between topographical categories (). This suggests that L-band HH and HV are also useful for determining categories of paddy fields based on the waterlogging situation affected by terrain.
The backscatter coefficients of the L-band HH of PALSAR and PALSAR-2 increase with rice growth owing to double scattering (Arai et al., Citation2018; Wang et al., Citation2009). However, the low backscatter coefficients of L-band HH in paddy areas of lowland and valley were observed in August (), where C-band VH in lowland paddy fields showed a higher value than the surrounding area (). The increase after 9 September (DOY 240) was due to double scattering (). The high permeability of the L-band compared to the C-band to vegetation may have delayed the appearance of the effect of double scattering. The earlier rise in HH from DOY127 to 183 at hillside paddy fields, which should be dryer than lowland and valleys () may be due to the increase of soil water content in non-flooded areas.
Microrelief and backscattering
Cross-polarisations of both C-band VH and L-band HV brought higher positive correlations between elevation and backscatter coefficients than those of co-polarisations C-band VV and L-band HH (). L-band HV constantly showed high correlation coefficients in July and August, whereas the C-band correlation for VH fluctuated largely (). This difference in stability may be due to differences in transparency and behaviours toward the roughness of the ground surface, etc., depending on wavelength.
The high correlation between L-band HV/HH ratio and elevation along each section shown in indicates that HV/HH ratio or the use of a combination of HH and HV of the L-band are valuable for the evaluation of the waterlogging condition affected by local microrelief as well as classification into the three terrain categories. Identifying a relationship between C-band VH/VV and microtopography was challenging; however, it indicated the surface waterlogging situation through the rise of the ratio owing to double scattering (such as at approximately 90 m in section 3 on 16 October ().
L-band HH and HV are almost parallel to the diagonal. Differences in HV/HH among points are caused by differences in intersects or slopes of lines along which HH-HV moves (). In other words, differences in waterlogging conditions appear in the intersects and slopes of lines along which HH-HV moves.
Conclusions
We analysed the behaviour of the VV and VH polarisation backscatter coefficients of the C-band Sentinel-1 and the HH and HV polarisation backscatter coefficients of the L-band PALSAR-2 on the surface of rice paddy fields in a rainfed rice-growing area with annual precipitation of 1000 mm, where waterlogging conditions in paddy fields are strongly affected by terrains.
Differences among the three major categories of lowlands, valleys, and hills were identified in time-series changes of backscatter coefficient for C-band VV and L-band HH and HV. These differences suggest that this combination of wavelengths and polarisations may clarify rain-fed paddy fields based on water logging conditions affected by the large topographic categories.
The L-band HV/HH ratio showed a high correlation with local microrelief elevation. This indicates that the combination of L-band HH and HV backscatter coefficient are useful for estimating slight differences in waterlogging situations within small spatial extent.
These findings allow for the classification and assessment of rainfed paddy fields based on diverse waterlogging conditions.
Author contributions
The first author was responsible for data analysis, construction of the theory, and the study design in detail. The second author was responsible for the field survey, data collection, and detailed information about the target area. The final author contributed to the selection of the target areas and the overall design of the study. All authors critically reviewed and revised the manuscript draft and approved the final version for submission.
Ethical approval
This study was approved by the National Research Council of Thailand under Research Permit Project ID:2008/092.
Acknowledgments
The authors thank the residents in the target area, university staff, and government officials who cooperated in the survey.
Disclosure statement
No potential conflict of interest was reported by the authors.
Data availability statement
Sentinel-1 and −2 data are accessible free of charge from the European Space Agency website (https://scihub.copernicus.eu/). PALSAR-2 data were sold by JAXA through an external provider.
Additional information
Funding
References
- Arai, H., Takeuchi, W., Oyoshi, K., Nguyen, L., & Inubushi, K. (2018). Estimation of methane emissions from rice paddies in the Mekong Delta based on land surface dynamics characterization with Remote Sensing. Remote Sensing, 10(9), 1438. Remote Sensing 10. https://doi.org/10.3390/rs10091438
- Bangira, T., Iannini, L., Menenti, M., van Niekerk, A., & Vekerdy, Z. (2021). Flood extent mapping in the Caprivi Floodplain using sentinel-1 time series. IEEE Journal of Selected Topics in Applied Earth Observations and Remote Sensing, 14, 5667–16. https://doi.org/10.1109/JSTARS.2021.3083517
- Bhogapurapu N, Dey S, Mandal D, Bhattacharya A, Karthikeyan L, McNairn H and Rao Y. (2022). Soil moisture retrieval over croplands using dual-pol L-band GRD SAR data. Remote Sensing of Environment, 271, 112900. https://doi.org/10.1016/j.rse.2022.112900
- Boonhong, J. (2014). Rice and production technology. (pp. 196). Pratum Thani, Thailand: Thammasart press. Thammasart University. ( in Thai).
- Fieuzal, R., & Baup, F. (2016). Improvement of bare soil semi-empirical radar backscattering models (Oh and Dubois) with SAR multi-spectral satellite data (X-, C- and L-Bands). Advances in Remote Sensing, 05(4), 296–314. https://doi.org/10.4236/ars.2016.54023
- Gorokhovich, Y., & Voustianiouk, A. (2006). Accuracy assessment of the processed SRTM-based elevation data by CGIAR using field data from USA and Thailand and its relation to the terrain characteristics. Remote Sensing of Environment, 104(4), 409–415. https://doi.org/10.1016/j.rse.2006.05.012
- Goto, S., Kuwagata, T., Konghakote, P., Polthanee, A., Ishigooka, Y., Toritani, H., & Hasegawa, T. (2008). Characteristics of water balance in a rainfed paddy field in Northeast Thailand. Paddy and Water Environment, 6(1), 153–157. https://doi.org/10.1007/s10333-007-0100-z
- Hoshikawa, K., Watanabe, K., Nagano, T., Kotera, A., & Fujihara, Y. (2018). Determination of patterns of rainfall history creating situations for accurate classification of rain-fed paddy fields with SAR backscatter coefficients. Remote Sensing Applications: Society & Environment, 9, 42–51. https://doi.org/10.1016/j.rsase.2017.11.004
- JAXA, Accessed on. (2022, October 26). Calibration result of ALOS-2_PALSAR-2 JAXA standard products. Japan Aerospace Exploration Agency. https://www.eorc.jaxa.jp/ALOS-2/en/calval/calval_index.htm
- Jearakongman, S., Rajatasereekul, S., Naklang, K., Romyen, P., Fukai, S., Skulkhu, E., Jumpaket, B., & Nathabutr, K. (1995). Growth and grain yield of contrasting rice cultivars grown under different conditions of water availability. Field Crops Research, 44(2–3), 139–150. https://doi.org/10.1016/0378-4290(95)00050-X
- Kaida, Y., Hoshikawa, K., & Kono, Y. (1985). Don Daeng village in Northeast Thailand: Instability of riceculture. Southeast Asian Studies, 23, 252–266. https://kyoto-seas.org/pdf/23/3/230304.pdf. (in Japanese).
- Keerthana, N., Salma, S., & Dodamani, B. M. (2022). Identifying rice crop flooding patterns using Sentinel-1 SAR data. Journal of the Indian Society of Remote Sensing, 50(8), 1569–1584. https://doi.org/10.1007/s12524-022-01553-4
- Koyama, C. N., & Schneider, K., 2011. Soil moisture retrieval under vegetation using dual polarized PALSAR data. 2011 IEEE International Geoscience and Remote Sensing Symposium, 24–29 July 2011, Vancouver, BC, Canada. (pp. 1059–1062).
- Minh, H. V. T., Avtar, R., Mohan, G., Misra, P., & Kurasaki, M. (2019). Monitoring and Mapping of rice cropping pattern in flooding area in the Vietnamese Mekong Delta using Sentinel-1A data: A case of an Giang Province. ISPRS International Journal of Geo-Information, 8(5), 211. https://doi.org/10.3390/ijgi8050211
- Miyagawa, S., Tsuji, T., Watanabe, K., & Hoshikawa, K. (2006). Long-term and spatial evaluation of rice crop performance of rain-fed paddy fields in a village of Northeast Thailand. Tropics, 15(1), 39–49. https://doi.org/10.3759/tropics.15.39
- Moroizumi, T., Hamada, H., Sukchan, S., & Ikemoto, M. (2009). Soil water content and water balance in rainfed fields in Northeast Thailand. Agricultural Water Management, 96(1), 160–166. https://doi.org/10.1016/j.agwat.2008.07.007
- Nelson, K., Sander, B. O., Yen, B. T., Yadav, S., & Laborte, A. G., 2022. Monitoring, reporting, and verification framework for rice production aligned with Paris Agreement transparency guidelines. IRRI Technical Guideline.
- Nguyen, D. B., Gruber, A., & Wagner, W. (2016). Mapping rice extent and cropping scheme in the Mekong Delta using Sentinel-1A data. Remote Sensing Letters, 7(12), 1209–1218. https://doi.org/10.1080/2150704X.2016.1225172
- Oh, Y. (2004). Quantitative retrieval of soil moisture content and surface roughness from multipolarized radar observations of bare soil surfaces. IEEE Transactions on Geoscience and Remote Sensing, 42(3), 596–601. https://doi.org/10.1109/TGRS.2003.821065
- Oh, Y., Sarabandi, K., & Ulaby, F. T. (1992). An empirical model and an inversion technique for radar scattering from bare soil surfaces. IEEE Transactions on Geoscience and Remote Sensing, 30(2), 370–381. https://doi.org/10.1109/36.134086
- Oteng, J. W., & Sant’anna, R. (1999). Rice production in Africa: Current situation and issues. International Rice Commission Newsletter. 48. https://www.fao.org/3/x2243t/x2243t05.htm
- Rice Department, Ministry of Agricultural Cooperatives, Thailand. (2008). Illustrations on rice growth and treatments at each stage. Open Educational Resources, Accessed 10 October 2023. https://oer.learn.in.th/search_detail/result/10105%20. ( Poster, in Thai).
- Singha, M., Dong, J., Sarmah, S., You, N., Zhou, Y., Zhang, G., Doughty, R., & Xiao, X. (2020). Identifying floods and flood-affected paddy rice fields in Bangladesh based on sentinel-1 imagery and Google earth engine. ISPRS Journal of Photogrammetry and Remote Sensing, 166, 278–293. https://doi.org/10.1016/j.isprsjprs.2020.06.011
- Torbick, N., Salas, W., Chowdhury, D., Ingraham, P., & Trinh, M. (2017). Mapping rice greenhouse gas emissions in the Red River Delta. Vietnam Carbon Management, 8(1), 99–108. https://doi.org/10.1080/17583004.2016.1275816
- Tsubo, M., Fukai, S., Basnayake, J., Tuong, T. P., Bouman, B., & Harnpichitvitaya, D. (2007). Effects of soil clay content on water balance and productivity in rainfed lowland rice ecosystem in northeast Thailand. Plant Production Science, 10(2), 232–241. https://doi.org/10.1626/pps.10.232
- Wang, M., Wang, J., Chen, L., & Du, Z. (2022). Mapping paddy rice and rice phenology with Sentinel-1 SAR time series using a unified dynamic programming framework. Open Geosciences, 14(1), 414–428. https://doi.org/10.1515/geo-2022-0369
- Wang, C., Wu, J., Zhang, Y., Pan, G., Qi, J., & Salas, W. A. (2009). Characterizing L-Band scattering of Paddy Rice in Southeast China with radiative transfer model and multitemporal ALOS/PALSAR imagery. IEEE Transactions on Geoscience and Remote Sensing, 47(4), 988–998. https://doi.org/10.1109/TGRS.2008.2008309
- Zhan, P., Zhu, W., & Li, N. (2021). An automated rice mapping method based on flooding signals in synthetic aperture radar time series. Remote Sensing of Environment, 252, 112112. https://doi.org/10.1016/j.rse.2020.112112