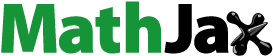
ABSTRACT
Hyperspectral images have been extensively employed to map alterations related to various ore deposits, particularly those associated with porphyry copper deposits. The present study aims to evaluate the efficiency of the PRISMA (Precursore Iper Spettraledella Missione Applicativa) satellite imagery, that is an Italian satellite, in identifying and discriminating alteration zones (Phyllic, Argillic, Propylitic) related to a well-known porphyry copper mineralization system in Iran. The chosen algorithms for performance evaluations involve selective principle component analysis (SPCA), spectral band ratios, mixture-tuned matched filtering (MTMF), and linear spectral unmixing (LSU). In this study, new band ratios are also proposed to improve the efficiency of alteration mapping. The results are validated by field data and geological-alteration map demonstrating the overall accuracy of detecting different alteration zones including the Phyllic, Argillic, and Propylitic zones equal to 71.87%, 87.50%, 81.25% and 71.87%, and kappa coefficients of 58.85%, 80.54%, 70.55% and 57.52% for SPCA, band ratio, MTMF, and LSU algorithms, respectively. In current research, it is found that using PRISMA data, the band ratio algorithm is superior when compared to other implemented algorithms in detecting hydrothermal alteration associated with porphyry copper deposits.
Introduction
The identification and classification of hydrothermal alteration minerals are crucial indicators in determining the occurrence of mineralization in the field of mineral exploration and preliminary surveying (Lowell & Gumbert, Citation1970; Rowan et al., Citation1977). A vast array of remote sensing techniques utilizing multispectral and hyperspectral images have extensively documented these invaluable insights (Loughlin, Citation1991, Rowan & Mars, Citation2003, Van der Meer et al., Citation2012, Chen et al., Citation2022;, Waske et al., Citation2009, Beiranvand Pour et al., Citation2013). An inner potassic assemblage, mainly featuring biotite and K-feldspar, exhibits a zonal distribution that expands outward and upward into phyllic, argillic, and propylitic zones. The phyllic zone often consists of rocks with sericite, illite, and pyrite, whereas the argillic zone contains kaolinite, dickite, and montmorillonites, and the outer propylitic zone showcases a mixture of minerals like chlorite-epidote and calcite (Atapour & Aftabi, Citation2007; Hosseinjanizadeh et al., Citation2014; Mars & Rowan, Citation2006). Hydrothermal alteration is observed in many valuable and base metal deposits, such as in Archean greenstone belts, volcanogenic massive sulfide (VMS) deposits, porphyries and intrusion-related orogenic gold mineralization.
Mineralization in these deposits is the consequence of hydrothermal to magmato-hydrothermal systems involving thin (centimeters) to widespread (hundreds of kilometers) alteration halos (Cooke et al., Citation2014; Eilu & Groves, Citation2001; Large & McGoldrick, Citation1998; Mathieu, Citation2018). Typical Porphyry Copper Deposits (PCDs) represent hydrothermal alteration zones, bearing indicator minerals. In addition, PCDs contain hematite, goethite, jarosite, and geochemical anomalies of Au, Cu, and Mo, which may have developed over pyritic shells (Atapour & Aftabi, Citation2007Hosseinjanizadeh et al., Citation2014). Several past and currently operating active and passive, hyperspectral and multispectral sensors have been used in remote sensing investigations for resources mapping and modeling (Melesse et al., Citation2007). Passive sensors, such as TM, ETM+, Aster, IKNOS, Quickbird, ALI, Hyperion and so on, rely on radiation from natural sources like reflected sunlight or emitted energy from objects on Earth. These satellites do not generate their own radiation. In contrast, active sensors, such as RADAR (Radio Detection and Ranging) and LIDAR (Light Detection and Ranging), have their own built-in sources of radiation (Jong et al., Citation2004). Detection of hydrothermal alteration zones and in some cases their lithological rock types (Shirazi et al., Citation2022) by various satellite images such as ASTER (Atif et al., Citation2022; Beiranvand Pour et al., Citation2020; Ishagh et al., Citation2021; Maleki et al., Citation2022; Sekandari et al., Citation2022; Shirazi et al., Citation2022), Hyperion (Yousefi et al., Citation2022), Landsat 7 (Aali et al., Citation2022), Landsat8 (Ishagh et al., Citation2021), HyMap (Hajaj et al., Citation2023), WorldView-3 (Sekandari et al., Citation2022), and sentinel-2 (Aali et al., Citation2022; Maleki et al., Citation2022) were reported in scientific papers.
Hyperspectral imageries are a powerful tool for extracting information to detect changes in the Earth’s surface (Eismann et al., Citation2009). The wide spectral information represented by hyperspectral data allows the discrimination of different materials spectrally (Eismann et al., Citation2009; Loizzo et al., Citation2018). These satellite images provide valuable data that can be practical in many science fields, such as lithological and alteration mapping in geoscience (Amer et al., Citation2010; Kamel et al., Citation2016; Yousefi et al., Citation2022).
PRISMA (Precursore Iper Spettraledella Missione Applicativa) is an Italian hyperspectral mission launched in 2018 (Loizzo et al., Citation2016). This satellite is a single Low Earth Orbit (620 km altitude) satellite with an expected operational lifetime of 5 years. The hyperspectral payload is composed of a Hyperspectral imager able to take images in a continuum of spectral bands ranging from 400 to 2500 nm, 240 continuous spectral bands, and optically integrated with a medium-resolution Panchromatic camera. PRISMA acquires data on areas of 30 km Swath width and with a Ground Sampling Distance (GSD) of 30 m (hyperspectral) and 5 m Panchromatic (PAN) (Loizzo et al., Citation2016; Loizzo et al., Citation2018). In full spectrums, bandwidth is crucial to pinpoint mineral absorption features. The narrowband sensors capture reflectance from a very specific spectrum area, providing a more detailed analysis. The reflectance properties of minerals in the VIS(Visible) and NIR (Near Infrared) regions can be related to their physicochemical properties, such as their physical structure or water content, which enhances their identification (Carmona Juarez, Citation2022). In geological fields, PRISMA data are used for mineral mapping (Bedini & Chen, Citation2020, Citation2022; Mishra et al., Citation2022); however, very few studies have investigated the applicability of PRISMA data to hydrothermal alteration mapping and must be promoted and demonstrated.
In the remote sensing field, according to various imageries proper techniques aptly have been utilized such as band rationing (Rowan & Mars, Citation2003), principal component analysis (PCA) (Crosta et al., Citation2003; Ranjbar et al., Citation2011), linear spectral unmixing (LSU) (Ishidoshiro et al., Citation2016), matched filtering (MF) (Mitchell & Glenn, Citation2009), and mixture tuned matched filtering (MTMF) (Hosseinjanizadeh et al., Citation2014). Hyperspectral images were utilized to map PCDs alterations (Ayoobi & Tangestani, Citation2018; Hosseinjanizadeh et al., Citation2014; Lotfi et al., Citation2020) by Area-based (Chen et al., Citation2022) and subpixel based (Chen et al., Citation2022; Hosseinjanizadeh et al., Citation2014) method in many remote sensing researches, while compared to the multispectral data, the hyperspectral data have more accurate ground object recognition.
Whereas remote sensing technology, in particular spectral resolution upgrades, simultaneously the expectation for more precise earth cover discrimination increases. Although previous hyperspectral satellites such as Hyperion imagery improved the detection and discrimination of hydrothermal alteration minerals, some deficiencies like the high number of noisy bands always exist. Not only this problem is alleviated in PRISMA imagery but the number of bands has increased. There are some researches related to PRISMA satellite imagery that evaluated PRISMA’s performance in different remote sensing fields. Vangi et al. analyzed the ability of the PRISMA hyperspectral sensor, compared to Sentinel-2 MSI for forest discrimination and the results show a 40% improvement in the discrimination between two understudy forests (Vangi et al., Citation2021). Bedini and Cheng analyzed PRISMA hyperspectral data in Cuprite, Nevada, USA for alteration mapping by adaptive coherence estimator (ACE) algorithm (Bedini & Chen, Citation2020). Again, Bedini and Cheng attempted to detect molybdenum, rare earth elements, gold, silver, and base metal mineralization in central east Greenland using PRISMA satellite hyperspectral imagery and the result shows the PRISMA satellite imageries’ potential for environmental applications (Bedini & Chen, Citation2022). Mishra et al compared the operation of AVIRIS-NG and spaceborne PRISMA hyperspectral data to mapping altered minerals in Jahazpur, Rajasthan (Mishra et al., Citation2022). Also, Pellegrino et al evaluated and reported the accuracy of the Level 2D PRISMA dataset products (Standard Reflectance Products) in visible and near-infrared (NIR) spectral regions over water bodies (Pellegrino et al., Citation2023).
Meanwhile, the new remote sensing imageries demand more investigation and development of applying methods. Due to the high spectral resolution of PRISMA data and spectral feature of alteration minerals in porphyry copper mineralization, the new band ratio identification has been developed in this paper to improve the efficiency of PRISMA data for alteration mapping. Due to the significance of PCDs as a source of industrial raw materials (Sillitoe, Citation1995), the primary objective of this study is to improve the ability to map the alteration zones related to Porphyry Copper Deposits (PCDs) using PRISMA satellite data, which represents the most recent and accessible hyperspectral data. To evaluate the efficiency of the PRISMA satellite images in mapping alteration (Phyllic, Argillic and Propylitic) zones, the alteration zones of the Kuhpanj porphyry system, as a case study, have been mapped using the proposed band ratio, selective PCA (SPCA), MTMF, and LSU methods. The main contrast between PCA and SPCA lies in the fact that SPCA chooses only a subset of bands depending on the desired objectives (Siljestrom et al., Citation1997). Ultimately, the alteration maps have been evaluated based on the field data, and the available geological map at a scale of 1:5000.
Material and methods
Datasets
Study area and geology
Kuhpanj district is part of the larger Urumieh-Dokhtar Magmatic Belt, an important geological formation in the region. This district is located in the southern segment of the UDMB, within the southeastern area of Kerman province. Its geographical coordinates span longitudinally between 56.05° and 55.08°E, while latitudinally it is located between 29.85° and 29.88°N. This region extends approximately 20 kilometers southeast of the Sarcheshmeh mine, which is recognized as the largest porphyry copper mine in Iran. This area is characterized by a semi-arid climate and exhibits limited vegetation cover. Significant porphyry copper deposits have been found in the UDMB as a result of the closure of the Neo-Tethys Ocean and the associated tectonic processes that occurred during the Tertiary period (Agard et al., Citation2005; Shafiei et al., Citation2009). Within the UDMB, porphyry copper mineralization is found in intrusive bodies that were formed during the Oligomiocene period. The Kerman Belt, which spans a width of 40 to 50 kilometers, follows the northwest to southeast direction of the UDMB along a total distance of 400 kilometers (Shafiei et al., Citation2009). The southeastern part of the UDMB is home to the Kerman Cenozoic Magmatic Arc (KCMA), also known as the Kerman Belt. This region is highly regarded as the most promising geological province for the occurrence of porphyry copper deposits in Iran (Aghazadeh et al., Citation2015). Significant crustal-scale dextral transform structures known as the Sarbizan and Naiband faults can be observed in the south-eastern end of the Kerman Belt. These faults are oriented in a northwest-southeast direction and cause displacement along the region (Shafiei, Citation2008). The Kerman Belt in Iran is characterized by two major phases of intrusions. The first phase, called “Jebal Barez”, occurred during the Eocene-Oligocene period. The second phase, known as “Kuhpanj”, took place during the mid-late Miocene. The Kuhpanj phase is located in the northwest and central regions of the Kerman Cenozoic Magmatic Arc (KCMA) and is associated with numerous porphyry copper deposits (Aghazadeh et al., Citation2015).
shows the 1:5000 geology map of the Kuhpanj area along with alteration assemblages and faults. Rock types include andesite, dacite, quartz diorite, andesite agglomerates, and tuffs. Different alteration zones are observed in the Kuhpanj porphyry copper district including propylitic, intermediate and advanced argillic, phyllic, and potassic (Tabatabaei et al., Citation2015). Microphotographs of these areas demonstrate that epidote and chlorite minerals were observed in samples from the propylitic alteration zone, while sericite (muscovite+illite) was observed in samples from the phyllic alteration zone (Babae, Citation2009; Najafian, Citation2010). The mineralization in the Kerman Belt often involves copper veins that are associated with Miocene quartz diorite subvolcanic activities. Hydrothermal solutions, derived from this magmatic activity, infiltrated through joints and fractures, primarily along major faults, allowing the formation of mineralized veins containing chalcopyrite and pyrite. Additionally, in the Kuhpanj area, there are occurrences of subsidiary galena mineralization, as well as scattered occurrences of malachite and azurite (Roshani et al., Citation2013). In many cases, the presence of iron oxides and hydroxyl minerals serves as a common indication of hydrothermal alteration and can be seen as surface expressions of mineralized zones. Iron oxide minerals, such as jarosite and hematite, are often observed in these areas. Additionally, two types of hydroxyl minerals are commonly considered: Al-OH minerals, including kaolinite and montmorillonite, and Mg-OH minerals, such as chlorite and epidote. These hydroxyl minerals contribute to the overall hydrothermal alteration patterns (Hosseinjanizadeh & Tangestani, Citation2011). According to Roshani et al, Cu and Mo are the commodity elements of the Kuhpanj mineralization that occurred in the north-western part of the region map extending south-easterly (Roshani et al., Citation2013).
Figure 1. Geological map of Kuhpanj copper mine (Tabatabaei et al, , Citation2015).

Hyperspectral image data
The Level 2D PRISMA dataset which corresponds to the top of atmosphere radiance radiometrically of this area has been recorded on the 1st of April 2022 and is downloaded from the Italian space agency. The main characteristics of PRISMA Payload are presented in . PRISMA products are divided into four levels, each of which has unique characteristics presented in . In this study, a level 2D PRISMA dataset has been employed which corresponds to Top-of-Atmosphere Radiance radiometrically corrected and calibrated in physical units. In this regard, the data from the surface does not need to be corrected for atmosphere interactions (R. et al., 2016). Although the SPCA and band ratio methods are not affected by the atmospheric noise-relevant bands, they will be a serious problem for the MTMF method. In this regard, noisy bands (with stripping effect) including 172, 92–94, and 46 bands, all in the SWIR region, covering 2490,1859– 1878, and 1394 nm should be removed.
Table 1. Main PRISMA payload characteristics (Loizzo et al., Citation2016).
Table 2. Description of PRISMA different level corrections (Loizzo et al., Citation2018).
Methodology
To achieve the objectives of the current study the selective PCA, new proposed band ratio, MTMF, and LSU algorithms were employed to detect porphyry copper deposit alterations using PRISMA data. The most popular and effective techniques for alteration detection, which have been utilized in numerous research works including this study, are pixel-based methods such as MTMF and LSU and spectral-based methods such as SPCA and band ratioing (Hosseinjanizadeh & Tangestani, Citation2011; Hosseinjanizadeh et al., Citation2014; Mars & Rowan, Citation2006; Tangestani & Moore, Citation2001). In the subsequent sections, the aforementioned algorithms are introduced first followed by the results obtained by applying them to the data from the Kuhpanj porphyry copper deposit. The accuracy of the methods was evaluated by comparing the alteration map values with the field ground truth samples.
Selective Principal component analysis (SPCA)
In PCA, the uncorrelated linear combinations (eigenvector loadings) of variables are selected in a way that each successively extracted linear combination (principal component) represents a smaller variance (Loughlin, Citation1991; Singh & Harrison, Citation1985). The strong correlation between the bands of satellite imageries generally causes much data redundancy. There are many researches in which the dimensions of satellite images (I = number of spectral bands) are reduced using principal component analysis (PCA) which effectively involves creating uncorrelated principal components (PCs) based on the original image (J=number of components) (Chen et al., Citation2022; Crosta et al., Citation2003; Singh & Harrison, Citation1985). To utilize this approach, one only needs a basic comprehension of the spectral features of minerals and vegetation. It heavily relies on the principal component transform’s competency to convert escalating intricacies in data variability into subsequent components (Loughlin, Citation1991). While PCA uses all spectral bands as input, Selective PCA (SPCA) which is used in this research, utilizes a subset of multispectral bands (bands that represent goal characteristics) as input, leading to dimension reduction and minimizing unused components (Siljestrom et al., Citation1997; Tangestani & Moore, Citation2001). Moreover, this technique increases spectral dissimilarity between two different regions in the spectrum (Siljestrom et al., Citation1997). The SPCA method is implemented to PRISMA imagery of the study area using MATLAB software.
Band ratio
Band ratioing is a method in which the DN value of a specific band is divided by the DN value of another specific band. Band ratios can be beneficial for extracting certain features or materials that cannot be visible within the raw bands (Inzana et al., Citation2003). Multiple or single ratios are widely implemented in the spectral bands in the processing of satellite imagery (Ninomiya, Citation2003; Rowan & Mars, Citation2003; Volesky et al., Citation2003). This technique highlights the difference between selected bands and decreases the effect of topography by specifying proper bands for identifying target spectral features. Different proposed ASTER band ratios for the discrimination of rock units and alterations have been the most practical band ratios in the geoscience field for many years (Amer et al., Citation2010; Gabr et al., Citation2010; Rowan & Mars, Citation2003).
MTMF method
MTMF algorithm is based on both signal processing methodologies and linear mixture theory and consists of two phases: Firstly, it involves performing a matched filter (MF) calculation to estimate the abundance of the target. Secondly, it employs a mixture tuning calculation to efficiently identify and dismiss false positives (Boardman, Citation1998; Mundt et al., Citation2007). The MTMF algorithm is capable of determining the abundance of minerals even when there is an unknown mixture of background spectra present (Mundt et al., Citation2007).
LSU method
The linear spectral mixing technique is a well-known method that has been widely applied to calculate the fraction of several individual surface components contained in each pixel of satellite images (Volesky et al., Citation2003). By decomposing each observed spectrum into its pure components, the LSU method provides subpixel resolution (Boardman, Citation1998; Mundt et al., Citation2007). The utilization of subpixel unmixing algorithms for mapping alteration mineral assemblages holds great potential in identifying hydrothermal conduits. This, in turn, could ultimately facilitate the discovery of buried ores (Ayoobi & Tangestani, Citation2018; Hosseinjanizadeh et al., Citation2014).
Implementation
In this section, SPCA, MTMF, LSU, and proposed band ratio methods were implemented on the PRISMA satellite separately and the results were reported and visualized. Then, the results of these methods were compared and discussed; particularly, the performance of proposed band ratios was compared with other methods. The flow chart of the data processing workflow of the current study is shown in :
Selective principal component analysis (SPCA) implementation
Epidote and chlorite, known as representative minerals in propylitic alteration, have diagnostic spectral features that can be detected by PRISMA bands. shows the spectral profile of epidote and chlorite, revealing distinct absorption regions at approximately 2335 nm, 2255 nm, between 1945 and 1955 nm, between 1825 and 1835 nm, 1546 nm, around 2325 nm, and between 2245 and 2255 nm. These regions also exhibit noticeable reflectance, between 2418 and 2440 nm, around 2145 nm, and around 2418 nm. outlines the absorption and reflectance regions for epidote and chlorite, alongside their respective PRISMA bands. According to noted absorption and reflectance features a subset as an input of the SPCA method was defined. SPCA was applied to a subset of 19 PRISMA bands including bands 162–165, 148–150, 138–139, 102, 122 and 101–103 in the SWIR region (). Then the eigenvalue matrix of the subset was calculated to identify which PC involved target (defined minerals) information. As seen in the scree plot (. a), most of the information was integrated into the first three PCs, including the target PCs. In the next step, the average values of eigenvalues related to continuous absorption and reflectance regions were calculated () which made it possible to identify the PC containing propylitic zone (epidote and chlorite) information. Similarly, according to Loughlin (Citation1991), the PC containing the target spectral bands information must have the highest eigenvector loadings, but with opposite signs (+ or -) (Loughlin, Citation1991). Normalized values () illustrate that spectral bands after 2140 nm (b125) are more representative of argillic alteration than those before, so here columns 3,4, and 5 () were considered. According to , bands 125 and 161–165 and bands 138, 139, and 148–150 are related to reflectance and absorption, respectively. Furthermore, PC1 has a high negative loading from bands 125 and 161–165 and a low positive loading from bands 138–139, and 148–150. Comparison of the average eigenvalue loadings in PC1 for bands 45, 60, 88–89, and 101–103 (column 2) with band 125 (column 3) shows that, however, both present similar signs, but there is a distinct difference between mentioned average eigenvalue loadings (near 40%) which confirms choosing PC1 for propylitic alteration mapping.
Figure 3. Spectral profile of Epidote and chlorite (Kokaly et al., Citation2017).

Figure 4. a) Eigenvalues for 19 selected PRISMA bands related to propylitic alteration b) Eigenvalues for 22 selected PRISMA bands related to argillic alterations c) Eigenvalues for 14 selected prima bands related to phyllic alteration.

Table 3. Spectral reflectance and absorption for epidote and chlorite and their related PRISMA bands (Kokaly et al., Citation2017).
Table 4. Average eigenvalues of reflectance/absorption band for first three eigenvectors and 19 selected PRISMA bands related to propylitic alteration.
The argillic alteration contains several representative minerals, including kaolinite, montmorillonite, and dickite. (). provides their spectral profile, revealing absorption (at wavelengths near 2386 nm, 2205 nm, 2165 nm, 1413 nm, 1393.5 nm, with a range between 2205 and 2215 nm, between 1905 and 1915 nm, and at wavelengths near 2386 nm and 2175 nm) and reflectance (within the range of 2235–2255 nm, near 2185 nm, near 1915 nm, near 1865 nm, near 2275 nm, between 2135 and 2165 nm, and near 2195 nm) spectral areas for these minerals, along with their corresponding PRISMA bands, as shown in (). 22 PRISMA bands including 45,46,50,97–99 in the VNIR, including 124–130,132–134,137–139,142,152,160 in the SWIR region, were selected for the SPCA method (). (). demonstrates the amount of information in each PC; accordingly, most of the information is collected in the first three PCs. In the same way, continuous spectral absorption or reflectance was averaged and the results are presented in (). There is no correct sign pattern for reflectance and absorption in PC2 and PC3 besides, according to normalized value, remarkable continuous spectral feature falls between 2235 and 2386 nm where bands 137, 138, 139, 142, 157, 160 exist. So, average eigenvalue loadings for bands 155 and 158 (noticeable absorption) and bands 137–139 and 142 (noticeable reflectance) are more considerable than the rest of the loadings. However, all eigenvalue loadings in PC1 are positive, but the eigenvalue loadings for bands 137, 138, 139, 142 are two times higher than the eigenvalue for bands 157 and 160. Additionally, another noticeable continuous spectral feature occurs between 1905 and 2165 nm where bands 97, 98, 124, 125, 126, and 128 exist. Where the average eigenvalue for bands 98, 124, 125, 126, 128 (related to reflectance) is 23 times higher than the eigenvalue for band 97 (related to absorption). It can be seen from PC1 that the positive and negative loadings are representative of reflectance and absorption, respectively, and this result is due to image transformation. According to all the aforementioned reasons, PC1 was selected for argillic alteration mapping.
Figure 5. Spectral profile of kaolinite, montmorillonite, and dickite (Kokaly et al., Citation2017).

Table 5. Spectral reflectance and absorption for kaolinite, montmorillonite, and dickite and their related PRISMA bands (Kokaly et al., Citation2017).
Muscovite and illite are considered as the most typical minerals in detecting phyllic alteration. (). displays the spectral profile of these minerals, revealing distinct absorption (at approximately 2440 nm, 2355 nm, within the range of 2205–2215 nm, near 1925 nm, 1413.5 nm, 2345 nm, 1905–1915 nm, and 1408.5–1413.5 nm) and reflection (at approximately 2285 nm, within the range of 18,550–18650 nm, 2275–2285 nm, and near 2145 nm) regions. (). represents absorption and reflectance spectral areas for these minerals and their related PRISMA bands. However, the SPCA should be applied to a subset of 16 PRISMA bands, including 47, 48, 91–93, 97, 99, 125, 133, 134, 143, 144, 151–153, and 166 (). in the preprocessing stage, bands 92 and 93 were removed as noisy bands. It should be noted that band 91 retrieves the removal of bands 92 and 93 and no huge data missing will occur. Similar to the case of propylitic and argillic alterations, in the scree plot of phyllic alteration, most of the information is integrated into the first three PCs (). In the same way, loadings with the same continuous spectral profile areas (absorption or reflectance) were averaged (). There are positive average loadings for bands 91, 125 and 143 (reflectance-related bands) and negative average loadings for the rest of the 14 selected bands in PC2. Besides, there is considerable continuous spectral difference between 2285 and 2440 nm in bands 143, 151–153 and 166 and the absolute eigenvalue loading for band 143 is about four times higher than those for bands 151–153 and 166. Therefore, sign patterns and clear differences between values in critical spectral regions verify PC2 for identifying phyllic alteration.
Figure 6. Spectral profile of muscovite and illite (Kokaly et al., Citation2017).

Table 6. Average eigenvalues of reflectance/absorption band for first three eigenvectors and 22 selected PRISMA bands related to argillic alteration.
Table 7. Spectral reflectance and absorption muscovite and illite and their related PRISMA bands (Kokaly et al., Citation2017).
Band ratio implementation
In the current study, three new band ratios are proposed to delineate different alteration zones in the study area, which can be implemented in other similar copper deposit areas. Typical propylitic alteration minerals (epidote and chlorite) have high absorption characteristics at 2090–2110 nm and 2325–2345 nm, and maximum reflection characteristics at 2400–2450 nm. According to the aforementioned spectral bands and (). a multiple-band ratio for PRISMA data is proposed as:
Also, typical argillic alteration minerals (kaolinite, montmorillonite, and dickite) have high absorption characteristics near 1400 nm and 2200 nm, and maximum reflection characteristics near 1600 nm. Regarding the mentioned spectral bands and ., a multiple band ratio for PRISMA data is suggested as:
In addition, typical phyllic alteration minerals (muscovite and illite) have high absorption characteristics near 2100 nm, and maximum reflection characteristics near 2280 nm. Based on the spectral bands and . a band ratio for PRISMA data can be arranged as:
Mixture tuned matched filtering (MTMF) implementation
As mentioned in section 3.2, noise-relevant bands (172, 91–94, and 46) have been eliminated, so the noise will not affect MTMF results. The next step is MNF transformation to minimize uncorrelated spatial noises (Green et al., Citation1988), which was implemented on the rest of the bands after noisy bands removal. Besides, based on a previous study (Yousefi et al., Citation2022), laboratory reflectance spectra of altered rock samples from the study area are available (). Here all argillic type groups (argillic, intermediate argillic, advanced argillic) and both propylitic type groups (argillic, propylitic and propylitic) are combined as one argillic unit and one propylitic unit. shows applied reference spectra in this research while both advanced argillic and argillic reference spectra are used as one alteration unit. There is an obvious resemblance in absorption and reflection area, between this spectrum (.) and USGS library spectra for under-study alteration indicator minerals in . These spectra are used as reference spectra in the MTMF method, with the aim of identifying three common alteration types in porphyry copper mineralization systems. Because eigenvalue loadings after the tenth MNF Band decreased sharply, it was evident that most variations among input data bands (more than 90%) are covered by the first ten MNF bands and the rest of the MNF bands are considered mostly noisy. Consequently, the MTMF algorithm was employed on the subset of 10 first MNF images that is the MNF bands with eigenvalues more than 7.9.
Figure 7. Reference spectra for a) propylitic alteration b) argillic alteration c) advanced argillic alteration d) phyllic alteration (Yousefi et al., Citation2022).

Linear spectral unmixing implementation
The reference spectra in part (2.3.3) are used as the reference for linear spectral unmixing method. Due to the extensive development of alteration zones in the study area, the target for the LSU process is propylitic, argillic, and phyllic alterations, which used all of the bands after removing noisy bands (172, 91–94 and 46) as a base image.
Results and discussion
The evaluation and discussion of the results obtained from the implemented methods on the PRISMA dataset should be conducted using appropriate criteria. In this case, the previously obtained ground truth samples (Babae, Citation2009; Najafian, Citation2010; Yousefi et al., Citation2022). The samples were collected based on their location representing different rock types and alterations. The quantity of samples taken from each alteration is proportionate to their surface area coverage. Each sample is precisely located and analyzed through petrographic examination, and microphotographs are available in certain instances. For detecting different rock types and their associated alterations in the study area, the Kuhpanj region can be utilized for calculating accuracy measures, such as the kappa coefficient, to assess the classification results.
Table 8. Average eigenvalues of reflectance/absorption band for first three eigenvectors and 14 selected PRISMA bands related to phyllic alteration.
Results
Based on the results obtained from the different methods, the following interpretations can be made:
The SPCA method presented in was able to identify propylitic, argillic, and phyllic alterations (, ). Bright DN values in PC1 for propylitic-related bands indicate high abundances of chlorite and epidote (). Bright DN values in PC1 for argillic-related bands confirm the presence of kaolinite, montmorillonite, and dickite (). Bright DN values in PC2 for phyllic-related bands are assumed to be associated with illite and muscovite phyllic alteration (). The bright DN value regions in the SPCA results correspond to the associated alterations in the geological map ().
Figure 8. A) PC1 of 19 selected bands related to propylitic alteration b) PC1 of 22 selected bands related to argillic alteration c) PC2 of 22 selected bands related to phyllic alteration.

The proposed band ratios for propylitic (Eqn.1), argillic (Eqn.2), and phyllic (Eqn.3) alterations effectively highlight alteration zones and the presence of specific minerals (). The band ratio for propylitic alteration highlights alteration zones and lithological units containing epidote and chlorite (.a). The band ratio for argillic alteration pinpoints alteration zones and lithological units containing kaolinite, montmorillonite, and dickite (.b). The band ratio for phyllic alteration delineates alteration zones and lithological units containing muscovite and illite (.c). The resulting band ratio images effectively delineate most of the alteration signatures in the study area ().
Figure 9. A) band ratio image using Equationeqn 1(1)
(1) , b) band ratio image using Equationeqn 2
(2)
(2) , and c) band ratio image using Equationeqn 3
(3)
(3) .

The alteration maps () generated by the MTMF algorithm for propylitic, argillic, and phyllic alterations display spatial coherence and are consistent with the geological map (). Moreover, the alteration maps obtained from the LSU method for propylitic, argillic, and phyllic alterations show similar patterns to the results of the MTMF method. The bright pixels in the target alteration zones () resemble the geological pattern observed in the geological map ().
Moreover, the results obtained from the various methods have been presented in the form of FCCs (False Color Composites) along with alteration boundaries of the geological map. This arrangement is meant to facilitate the comparison and interpretation of the findings, as shown in . While all four methods are capable of detecting some level of alteration, the band ratio and MTMF techniques demonstrate superior discrimination capabilities in argillic and propylitic regions. Additionally, the MTMF method effectively detects phyllic areas that more closely resemble the geological map. Overall, the results obtained from the different methods provide consistent and reliable identification and mapping of propylitic, argillic, and phyllic alterations in the study area. The MTMF and band ratio methods are effective in pinpointing alteration zones and identifying specific minerals associated with each alteration type respectively.
Discussion
The authors used ground truth samples to compare and verify their classification results. These samples were obtained from previous investigations in the study area (Babae, Citation2009; Najafian, Citation2010; Yousefi et al., Citation2022). A total of 32 samples were collected, with 10 samples from the propylitic alteration zone, 16 samples from the argillic alteration zone and 6 samples from the phyllic alteration zone. The sample locations are shown in , which is a false-color composite image of the Kuhpanj region. This image was chosen because it had the maximum Optimum Index Factor (OIF) value. In addition to visual inspection, thin sections of some samples were studied (). These thin sections showed the presence of specific minerals that are indicative of certain alteration zones. provides further details on the mineralogy observed in these thin sections.
Figure 13. Sample points location on the false-color composite image (38-8-7 in VNIR) of the Kuhpanj area.

Figure 14. Microphotographs of four sample points: a) sample of propylitic alteration with the presence of epidote (location: 56.076°E, 29.881°N) b) andesite sample with phyllic alteration and presence of sericite and pyrite veinlets (location: 56.066°E, 29.877°N) c) granodiorite sample with phyllic alteration and presence of crossed nickel veinlets (location: 56.067°E, 29.876°N) d) andesite sample with propylitic alteration and presence of epidote and chlorite (location: 56.055°E, 29.863°N) e) sample of argilic zone with presence of plagioclase and its replacement clay minerals (location: 56.061°E, 29.874°N) f) sample from advanced argillic with presence of plagioclase and its replacement by sericite and clay minerals (location: 56.068°E, 29.865°N) (Najafian, Citation2010 , Yousefi et al., Citation2022).

Figure 15. A) classified PC1 of 19 selected bands related to propylitic alteration b) classified PC1 of 22 selected bands related to argillic alteration c) classified PC2 of 22 selected bands related to phyllic alteration (Refer to ).

Table 9. Mineral occurrence in alteration zones of the Kuhpanj region (Yousefi et al., Citation2022(Babae, Citation2009) (Najafian, Citation2010).
The comparison between the results obtained from SPCA, Band ratioing, MTMF, and LSU and each field ground truth sample is represented in . Under specified conditions (DN > 102 as the target), the highest accuracy and kappa coefficient (87.50% and 80.54% respectively) could be observed in the band ratio method which are significantly in agreement with the alteration classification quality and accuracy results. The lowest accuracy (71.87%) and kappa coefficient (57.52%) are obtained from the linear spectral unmixing method. Although an acceptable range of accuracy values could be seen in the SPCA and LSU methods, they failed to appropriately classify the alteration zones. In the case of the MTMF method, accuracy and kappa coefficient values are 81.25% and 70.55% respectively, however, due to the presence of mixed regions and subsequently mixed pixels the efficiency of the MTMF subpixel method has decreased. Furthermore, considering the same reference spectra, the MTMF method operates more effectively than LSU alteration detection and discrimination. Although LSU and SPCA display similar accuracy, the Kappa coefficient for SPCA is slightly more than LSU, which means better classification by SPCA.
Table 10. Confusion matrix for all applied methods versus field data.
In this research work the results of applying band ratioing (Alimohammadi et al., Citation2015; Rowan & Mars, Citation2003) and MTMF (Chen et al., Citation2022; Hosseinjanizadeh et al., Citation2014) methods for hydrothermal alteration mapping using PRISMA imagery data are evaluated. Although alteration mapping in the same region using multi-spectral data such as Aster through applying MTMF and band ratioing methods have been reported in other papers previously, as was expected, the higher spectral resolution of PRISMA data has led to more accurate results in the current study.
One of the major limitations in this work is the presence of mixing alteration assemblages, which cause uncertainty and error in classification results. In fact, in most studied areas there are no pure propylitic, argillic, or phyllic assemblages with clear boundaries and our obtained classification results show close agreement to real ground truth which in this respect is very optimistic. Another limitation that should be considered is mineral changes in a certain alteration class, which may divide a class into two or more sub-classes mostly occurring in Argillic alteration, where we may observe advanced argillic and argillic alteration with different mineral aggregations. In this case, if the content of alteration mineral variation in a class is high, the number of expected classes would increase accordingly. In the current work, no subclasses were required; however, the mixing alteration zones may have affected the classification results to some extent which is reflected in the classification accuracy measures.
Figure 16. A) classified band ratio image using Equationeqn 1(1)
(1) , b) classified band ratio image using Equationeqn 2
(2)
(2) , and c) classified band ratio image using Equationeqn 3
(3)
(3) .

Conclusions
The main objective of this study was to determine if PRISMA satellite imagery can effectively identify hydrothermal alterations associated with porphyry copper mineralization. To achieve this, several popular spectral discrimination algorithms, such as SPCA, Band ratio, MTMF, and LSU, were used. Additionally, new band ratios were developed to improve the detection of specific alterations related to porphyry copper mineralization, such as propylitic, argillic, and phyllic assemblages.
Two evaluation methods were chosen: comparison of results with geological map alteration boundaries and numerical assessment. Accuracy and kappa coefficient are computed in the numerical assessment. To assess the accuracy of the algorithms, the results were compared with field ground truth sample data and geological maps. The overall accuracy percentages for SPCA, band ratioing, MTMF, and LSU algorithms were 71.87%, 87.50%, 81.25% and 71.87%, respectively. The corresponding kappa coefficients, which measure agreement beyond chance, were 58.85%, 80.54%, 70.55%, and 57.52%, respectively. The band ratioing method exhibits slightly better overall accuracy and kappa coefficient than the MTMF method (). Although visually the output of the MTMF method bears more resemblance to the alteration boundaries from the geological map than the band ratioing method (). The alteration boundaries for argillic and propylitic approximately match the output of both methods, while the MTMF method performs better in phyllic alteration mapping. Numerically, the band ratioing method shows the highest classification performance among the tested algorithms. However, its weakness in phyllic alteration mapping may derived from the presence of mixed zones.
Overall, in multispectral sensors such as ASTER and Landsat, low spectral resolution (Rowan & Mars, Citation2003; Rowan et al., Citation1977) can limit the ability to discriminate between different features. Similarly, some hyperspectral sensors, such as Hyperion, have high numbers of noisy bands (Hosseinjanizadeh et al., Citation2014; Lotfi et al., Citation2020) that can reduce the quality of discrimination. PRISMA imagery addresses these issues to some extent, providing improved discrimination quality for remote sensing applications. Band ratios have been previously suggested for detecting alteration in ASTER imagery (Rajendran & Nasir, Citation2019; Rowan & Mars, Citation2003; Volesky et al., Citation2003), with the ratios proposed by Rowan and Mars being the most well-known and successful for detecting three principal alteration zones in porphyry copper deposits (Rowan & Mars, Citation2003). Wide spectral windows which lead to the low spectral resolution were obvious problems which improved by presenting new band ratios through PRISMA imagery in this work. Furthermore, the quantity of bands producing noise is less in contrast to previously developed hyperspectral sensors such as Hyperion. The results of this study confirmed that PRISMA hyperspectral data can effectively improve the detection of various alteration minerals. Additionally, the newly proposed band ratio indicators show promising potential for future mapping of mineral alterations associated with porphyry copper mineralization systems using PRISMA data.
Disclosure statement
No potential conflict of interest was reported by the author(s).
Additional information
Funding
References
- Aali, A. A., Shirazy, A., Shirazi, A., Pour, A. B., Hezarkhani, A., Maghsoudi, A., Hashim, M., & Khakmardan, S. (2022). Fusion of remote sensing, magnetometric, and Geological Data to identify polymetallic mineral potential zones in Chakchak Region, Yazd, Iran. Remote Sensing, 14(23), 6018. https://doi.org/10.3390/rs14236018
- Agard, P., Omrani, J., Jolivet, L., & Mouthereau, F. (2005). Convergence history across Zagros (Iran): Constraints from collisional and earlier deformation. International Journal of Earth Sciences, 94(3), 401–19. https://doi.org/10.1007/s00531-005-0481-4
- Aghazadeh, M., Hou, Z., Badrzadeh, Z., & Zhou, L. (2015). Temporal–spatial distribution and tectonic setting of porphyry copper deposits in Iran: Constraints from zircon U–pb and molybdenite re–os geochronology. Ore Geology Reviews, 70, 385–406. https://doi.org/10.1016/j.oregeorev.2015.03.003
- Alimohammadi, M., Alirezaei, S., & Kontak, D. J. (2015). Application of ASTER data for exploration of porphyry copper deposits: A case study of daraloo–sarmeshk area, southern part of the Kerman copper belt, Iran. Ore Geology Reviews, 70, 290–304. https://doi.org/10.1016/j.oregeorev.2015.04.010
- Amer, R., Kusky, T., & Ghulam, A. (2010). Lithological mapping in the Central Eastern Desert of Egypt using ASTER data. Journal of African Earth Sciences, 56(2–3), 75–82. https://doi.org/10.1016/j.jafrearsci.2009.06.004
- Atapour, H., & Aftabi, A. (2007). The geochemistry of gossans associated with sarcheshmeh porphyry copper deposit for exploration and the environment. Journal of geochemical exploration, 93, 47–65.
- Atif, Y., Soulaimani, A., Ait Lamqadem, A., Pour, A. B., Pradhan, B., Nouamane, E. A., Abdelali, K., Muslim, A. M., & Hossain, M. S. (2022). Identifying hydrothermally altered rocks using ASTER satellite imageries in Eastern anti-atlas of Morocco: A case study from imiter silver mine. International Journal of Image and Data Fusion, 13(4), 337–361. https://doi.org/10.1080/19479832.2021.1958928
- Ayoobi, I., & Tangestani, M. H. (2018). Evaluation of subpixel unmixing algorithms in mapping the porphyry copper alterations using EO-1 Hyperion data, a case study from SE Iran. Remote Sensing Applications: Society & Environment, 10, 120–127. https://doi.org/10.1016/j.rsase.2018.03.009
- Babae, M. (2009). Integration of Geochemical, geophysical and remote sensing data for uranium indices by using GIS [MSc Thesis]. Shahid Bahonar University of Kerman.
- Bedini, E., & and Chen, J. (2020). Application of PRISMA satellite hyperspectral imagery to mineral alteration mapping at cuprite. Journal of hyperspectral remote sensing v, 10, 87–94.
- Bedini, E., & Chen, J. (2022). Prospection for economic mineralization using PRISMA satellite hyperspectral remote sensing imagery: An example from central East Greenland. Journal of Hyperspectral Remote Sensing, V12(3), 124–130. https://doi.org/10.29150/2237-2202.2022.253484
- Beiranvand Pour, A. B., Hashim, M., & van Genderen, J. (2013). Detection of hydrothermal alteration zones in a tropical region using satellite remote sensing data: Bau goldfield, Sarawak, Malaysia. Ore Geology Reviews, 54, 181–196. https://doi.org/10.1016/j.oregeorev.2013.03.010
- Beiranvand Pour, A. B., Sekandari, M., Rahmani, O., Crispini, L., Läufer, A., Park, Y., Hong, J. K., Pradhan, B., Hashim, M., Hossain, M. S., Muslim, A. M., & Mehranzamir, K. (2020). Identification of phyllosilicates in the antarctic environment using ASTER satellite data: Case study from the Mesa range, Campbell and Priestley Glaciers, Northern Victoria Land. Remote Sensing, 13(1), 38. https://doi.org/10.3390/rs13010038
- Boardman, J. W. (1998). Leveraging the high dimensionality of AVIRIS data for improved sub-pixel target unmixing and rejection of false positives: Mixture tuned matched filtering, Summaries of the Seventh JPL Airborne Geoscience Workshop, USA (Vol. 97, pp. 55–56). JPL Publication.
- Carmona Juarez, L. (2022). Mapping mineralogy in the Rodalquilar caldera, Spain, using PRISMA satellite images [Msc Thesis, Faculty of Geosciences of Utrecht University]. The Netherlands.
- Chen, Q., Zhao, Z., Zhou, J., Zhu, R., Xia, J., Sun, T., Zhao, X., & Jiangqin, C. (2022). ASTER and GF-5 Satellite Data for Mapping Hydrothermal Alteration Minerals in the Longtoushan Pb-Zn Deposit, SW China. Remote Sensing, 14(5), 1253. 1253. https://doi.org/10.3390/rs14051253
- Cooke, D. R., Baker, M., Hollings, P., Sweet, G., Chang, Z., Danyushevsky, L., Gilbert, S., Zhou, T., White, N. C., & Gemmell, J. B. (2014). New advances in detecting the distal geochemical footprints of porphyry systems—epidote mineral chemistry as a tool for vectoring and fertility assessments.
- Crosta, A. P., De Souza Filho, C. R., Azevedo, F., & Brodie, C. (2003). Targeting key alteration minerals in epithermal deposits in Patagonia, Argentina, using ASTER imagery and principal component analysis. International Journal of Remote Sensing, 24(21), 4233–4240. https://doi.org/10.1080/0143116031000152291
- Eilu, P., & Groves, D. I. (2001). Primary alteration and geochemical dispersion haloes of Archaean orogenic gold deposits in the Yilgarn Craton: The pre-weathering scenario. Geochemistry: Exploration, Environment, Analysis, 1(3), 183–200. https://doi.org/10.1144/geochem.1.3.183
- Eismann, M. T., Stocker, A. D., & Nasrabadi, N. M.2009. Automated hyperspectral cueing for civilian search and rescue. Proceedings of the IEEE, USA (Vol. 97, pp. 1031–1055).
- Gabr, S., Ghulam, A., & Kusky, T. (2010). Detecting areas of high-potential gold mineralization using ASTER data. Ore Geology Reviews, 38(1–2), 59–69. https://doi.org/10.1016/j.oregeorev.2010.05.007
- Green, A. A., Berman, M., Switzer, P., & Craig, M. D. (1988). A transformation for ordering multispectral data in terms of image quality with implications for noise removal. IEEE Transactions on Geoscience and Remote Sensing, 26(1), 65–74. https://doi.org/10.1109/36.3001
- Hajaj, S., El Harti, A., Jellouli, A., Pour, A. B., Himyari, S. M., Hamzaoui, A., Bensalah, M. K., Benaouiss, N., & Hashim, M. (2023). HyMap imagery for copper and manganese prospecting in the east of Ameln Valley shear zone (kerdous inlier, western anti-atlas, Morocco). Journal of Spatial Science, 1–22. https://doi.org/10.1080/14498596.2023.2172085
- Hosseinjanizadeh, M., & Tangestani, M. H. (2011). Mapping alteration minerals using sub-pixel unmixing of ASTER data in the Sarduiyeh area, SE Kerman, Iran. International Journal of Digital Earth, 4(6), 487–504. https://doi.org/10.1080/17538947.2010.550937
- Hosseinjanizadeh, M., Tangestani, M. H., Roldan, F. V., & Yusta, I. (2014). Sub-pixel mineral mapping of a porphyry copper belt using EO-1 hyperion data. Advances in Space Research, 53(3), 440–451. https://doi.org/10.1016/j.asr.2013.11.029
- Hosseinjanizadeh, M., Tangestani, M., Velasco Roldán, F., & Yusta, I.2014. Mineral exploration and alteration zone mapping using mixture tuned matched filtering approach on ASTER data at the central part of dehaj-sarduiyeh copper belt, SE Iran. IEEE JOURNAL OF SELECTED TOPICS IN APPLIED EARTH OBSERVATIONS AND REMOTE SENSING, 7, 284–289. https://doi.org/10.1109/JSTARS.2013.2261800
- Inzana, J., Kusky, T., Higgs, G., & Tucker, R. (2003). Supervised classifications of landsat TM band ratio images and landsat TM band ratio image with radar for geological interpretations of central Madagascar. Journal of African Earth Sciences, 37(1), 59–72. https://doi.org/10.1016/S0899-5362(03)00071-X
- Ishagh, M. M., Pour, A. B., Benali, H., Idriss, A. M., Reyoug, S. S., Muslim, A. M., & Hossain, M. S. (2021). Lithological and alteration mapping using landsat 8 and ASTER satellite data in the reguibat shield (West African Craton), north of Mauritania: Implications for uranium exploration. Arabian Journal of Geosciences, 14(23), 2576. https://doi.org/10.1007/s12517-021-08846-x
- Ishidoshiro, N., Yamaguchi, Y., Noda, S., Asano, Y., Kondo, T., Kawakami, Y., Mitsuishi, M., & Nakamura, H. (2016). Geological mapping by combining spectral unmixing and cluster analysis for hyperspectral data. The International Archives of the Photogrammetry, Remote Sensing and Spatial Information Sciences, 41, 431–435. https://doi.org/10.5194/isprsarchives-XLI-B8-431-2016
- Jong, S. M. D., Meer, F. D. V. D., & Clevers, J. G. (2004). Basics of remote sensing. In Remote sensing image analysis: including the spatial domain (pp. 1–15). Dordrecht: Springer Netherlands.
- Kamel, M., Youssef, M., Hassan, M., & Bagash, F. (2016). Utilization of ETM+ landsat data in geologic mapping of wadi ghadir-gabal zabara area, Central Eastern Desert, Egypt. The Egyptian Journal of Remote Sensing & Space Science, 19(2), 343–360. https://doi.org/10.1016/j.ejrs.2016.06.003
- Kokaly, R. F., Clark, R. N., Swayze, G. A., Livo, K. E., Hoefen, T. M., Pearson, N. C., Wise, R. A., Benzel, W. M., Lowers, H., & Driscoll, R. L. (2017). USGS spectral library version 7 data: Us geological survey data release. United States Geological Survey (USGS).
- Large, R. R., & McGoldrick, P. J. (1998). Lithogeochemical halos and geochemical vectors to stratiform sediment hosted zn–pb–ag deposits, 1. Lady Loretta Deposit, Queensland. Journal of Geochemical Exploration, 63(1), 37–56. https://doi.org/10.1016/S0375-6742(98)00013-2
- Loizzo, R., Ananasso, C., Guarini, R., Lopinto, E., Candela, L., & Pisani, A. R. 2016, May. The Prisma hyperspectral mission. In Proceedings of the Living Planet Symposium, ague, Czech Republic (pp. 9–13).
- Loizzo, R., Guarini, R., Longo, F., Scopa, T., Formaro, R., Facchinetti, C., & Varacalli, G., 2018, July. PRISMA: The Italian hyperspectral mission. In IGARSS 2018-2018 IEEE International Geoscience and Remote Sensing Symposium, Valencia, Spain (pp. 175–178). IEEE.
- Lotfi, M., Arefi, H., & Bahroudi, A. (2020). Investigating alteration zone mapping using EO-1 hyperion imagery and Airborne Geophysics Data. Journal of Mining & Environment, 11(2), 405–417.
- Loughlin, W. P. (1991). Principal component analysis for alteration mapping. Photogrammetric Engineering and Remote Sensing, 57(9), 1163–1169.
- Lowell, J. D., & Gumbert, J. M. (1970). Lateral and vertical alteration-mineralization zoning in porphyry ore deposits: Econ. Geol, 65(4), 373–408. https://doi.org/10.2113/gsecongeo.65.4.373
- Maleki, M., Niroomand, S., Rajabpour, S., Beiranvand Pour, A., & Ebrahimpour, S. (2022). Targeting local orogenic gold mineralization zones using data-driven evidential belief functions: The godarsorkh area, Central Iran. All Earth, 34(1), 259–278. https://doi.org/10.1080/27669645.2022.2129132
- Mars, J. C., & Rowan, L. C. (2006). Regional mapping of phyllic and argillic altered rocks in the Zagros magmatic arc, Iran, using advanced spaceborne thermal emission and reflection radiometer (ASTER) data and logical operator algorithms. Geosphere, 2(3), 161–186. https://doi.org/10.1130/GES00044.1
- Mathieu, L. (2018). Quantifying hydrothermal alteration: A review of methods. Geosciences, 8(7), 245. https://doi.org/10.3390/geosciences8070245
- Melesse, A. M., Weng, Q., & Thenkabail S. P, & Senay, G. B. (2007). Remote sensing sensors and applications in environmental resources mapping and modelling. Sensors, 7(12), 3209–3241. https://doi.org/10.3390/s7123209
- Mishra, G., Govil, H., Guha, A., Kumar, H., Kumar, S., & Mukherjee, S. (2022). Comparative evaluation of airborne AVIRIS-NG and spaceborne PRISMA hyperspectral data in identification and mapping of altered/weathered minerals in Jahazpur, Rajasthan. Advances in Space Research. https://doi.org/10.1016/j.asr.2022.09.047
- Mitchell, J. J., & Glenn, N. F. (2009). Subpixel abundance estimates in mixture-tuned matched filtering classifications of leafy spurge (Euphorbia esula L.). International Journal of Remote Sensing, 30(23), 6099–6119. https://doi.org/10.1080/01431160902810620
- Mundt, J. T., Streutker, D. R., & Glenn, N. F.2007. Partial unmixing of hyperspectral imagery: Theory and methods. Proceedings of the American Society of Photogrammetry and Remote Sensing, 2007.
- Najafian, T. (2010). Discrimination and identification of hydrothermal alteration types related to porphyry copper deposits, Sarcheshmeh, Kerman Province. Shahid Bahonar University.
- Ninomiya, Y.2003. A stabilized vegetation index and several mineralogic indices defined for ASTER VNIR and SWIR data. IGARSS 2003. 2003 IEEE International Geoscience and Remote Sensing Symposium. Proceedings IEEE Cat, France. 1552–1554.
- Pellegrino, A., Fabbretto, A., Bresciani, M., Lima, T., Braga, F., Pahlevan, N., Brando, V., Kratzer, S., Gianinetto, M., & Giardino, C. (2023). Assessing the accuracy of PRISMA standard reflectance products in globally distributed aquatic sites. Remote Sensing, 15(8), 2163. https://doi.org/10.3390/rs15082163
- Rajendran, S., & Nasir, S. (2019). ASTER capability in mapping of mineral resources of arid region: A review on mapping of mineral resources of the sultanate of Oman. Ore Geology Reviews, 108(March 2017), 33–53. https://doi.org/10.1016/j.oregeorev.2018.04.014
- Ranjbar, H., Masoumi, F., & Carranza, E. J. M. (2011). Evaluation of geophysics and spaceborne multispectral data for alteration mapping in the Sar Cheshmeh mining area, Iran. International Journal of Remote Sensing, 32(12), 3309–3327. https://doi.org/10.1080/01431161003745665
- Roshani, P., Mokhtari, A. R., & Tabatabaei, S. H. (2013). Objective based geochemical anomaly detection—application of discriminant function analysis in anomaly delineation in the Kuh Panj porphyry cu mineralization (Iran). Journal of Geochemical Exploration, 130, 65–73. https://doi.org/10.1016/j.gexplo.2013.03.005
- Rowan, L. C., Goetz, A. F. H., & Ashley, R. P. (1977). Discrimination of hydrothermally altered and unaltered rocks in visible and near infrared multispectral images. Geophysics, 42(3), 522–535. https://doi.org/10.1190/1.1440723
- Rowan, L. C., & Mars, J. C. (2003). Lithologic mapping in the Mountain Pass, California area using advanced spaceborne thermal emission and reflection radiometer (ASTER) data. Remote Sensing of Environment, 84(3), 350–366. https://doi.org/10.1016/S0034-4257(02)00127-X
- Sekandari, M., Masoumi, I., Pour, A. B., Muslim, A. M., Hossain, M. S., & Misra, A. (2022). ASTER and WorldView-3 satellite data for mapping lithology and alteration minerals associated with pb-zn mineralization. Geocarto International, 37(6), 1782–1812. https://doi.org/10.1080/10106049.2020.1790676
- Shafiei, B.2008. Metallogenic Model of Kerman Porphyry Copper Belt and Its Exploratory Approaches. Ph. D. Thesis, Shaheed Bahonar University of Kerman.
- Shafiei, B., Haschke, M., & Shahabpour, J. (2009). Recycling of orogenic arc crust triggers porphyry cu mineralization in Kerman cenozoic arc rocks, southeastern Iran. Mineralium Deposita, 44(3), 265–283. https://doi.org/10.1007/s00126-008-0216-0
- Shirazi, A., Hezarkhani, A., Beiranvand Pour, A., Shirazy, A., & Hashim, M. (2022). Neuro-fuzzy-AHP (NFAHP) technique for copper exploration using advanced spaceborne thermal emission and reflection radiometer (ASTER) and geological datasets in the sahlabad mining area, East Iran. Remote Sensing, 14(21), 5562. https://doi.org/10.3390/rs14215562
- Siljestrom, P. A., Moreno, A., Vikgren, K., & Caceres, L. M. (1997). Technical note the application of selective principal components analysis (SPCA) to a thematic mapper (TM) image for the recognition of geomorphologic features configuration. International Journal of Remote Sensing, 18(18), 3843–3852. https://doi.org/10.1080/014311697216658
- Sillitoe, R. H. (1995). Exploration of porphyry copper lithocaps. Pacrim Congress 1995, Australia (pp. 527–532). Australasian Institute of Mining and Metallurgy.
- Singh, A., & Harrison, A. (1985). Standardized principal components. International Journal of Remote Sensing, 6(6), 883–896. https://doi.org/10.1080/01431168508948511
- Tabatabaei, S. H., Rodsari, P. R., & Mokhtari, A. R. (2015). Predicting Potential Mineralization Using Surface Geochemical Data and Multiple Linear Regression Model in the Kuh Panj Porphyry Cu Mineralization (Iran). The Arabian Journal for Science and Engineering, 40, 163–170.
- Tangestani, M. H., & Moore, F. (2001). Comparison of three principal component analysis techniques to porphyry copper alteration mapping: A case study, meiduk area, kerman, iran). Canadian Journal of Remote Sensing, 27(2), 176–182. https://doi.org/10.1080/07038992.2001.10854931
- Van der Meer, F. D., van der Werff, H. M. A., van Ruitenbeek, F. J. A., Hecker, C. A., Bakker, W. H., Noomen, M. F., van der Meijde, M., Carranza, E. J. M., de Smeth, J. B., & Woldai, T. (2012). Multi- and hyperspectral geologic remote sensing: A review. International Journal of Applied Earth Observation and Geoinformation, 14(1), 112–128. https://doi.org/10.1016/j.jag.2011.08.002
- Vangi, E., D’Amico, G., Francini, S., Giannetti, F., Lasserre, B., Marchetti, M., & Chirici, G. (2021). The new hyperspectral satellite PRISMA: Imagery for forest types discrimination. Sensors, 21(Issue 4). https://doi.org/10.3390/s21041182
- Volesky, J. C., Stern, R. J., & Johnson, P. R. (2003). Geological control of massive sulfide mineralization in the Neoproterozoic Wadi Bidah shear zone, southwestern Saudi Arabia, inferences from orbital remote sensing and field studies. Precambrian Research, 123(2–4), 235–247. https://doi.org/10.1016/S0301-9268(03)00070-6
- Waske, B., Benediktsson, J. A., Arnason, K., & Sveinsson, J. R. (2009). Mapping of hyperspectral AVIRIS data using machine-learning algorithms. Canadian Journal of Remote Sensing, 35(sup1), S106–S116. https://doi.org/10.5589/m09-018
- Yousefi, M., Tabatabaei, S. H., Rikhtehgaran, R., Pour, A. B., & Pradhan, B. (2022). Detection of alteration zones using the dirichlet process stick-breaking model-based clustering algorithm to hyperion data: The case study of Kuh-Panj porphyry copper deposits, Southern Iran. Geocarto International, 37(25), 9788–9816. https://doi.org/10.1080/10106049.2022.2025917